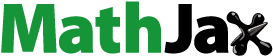
Abstract
With the objective of evaluating the accuracy of price index models, we adopt a series of techniques to compares the performances of the hedonic, repeat sales, and hybrid models based on the data from the Chinese most representative painter, Qi Baishi during the period from 2000 to 2016. When applying the mean squared error (MSE) technique, the repeat sales model outperforms alternative models. However, according to the correlations and width confidence intervals, the hybrid model provides the most reliable estimates of price indices. The study also shows that the repeat sales model obtains relatively a lower total return estimate as well as a higher volatility than other two models. Our findings have important implications in identifying the precision of index models and provide supplements to art investment studies..
Public Interest Statement
The rapid growth in the Chinese paintings market has attracted increasingly attention in recent years. Studies focusing on returns of Chinese artworks generally utilize different models and there has been no study comparing the results and no uniform conclusion on these issues. This study investigates the performances of the common models by using the data from the Chinese most influential painter, Qi Baishi during the period from 2000 to 2016. Notably, it is the first time to combine main index construction models to provide an overall perspective on their performances in the Chinese paintings market.
1. Introduction
The rapid growth in the Chinese paintings market has attracted increasingly attention in recent years. Until now, studies focusing on returns of Chinese artworks generally utilize the common hedonic or repeat sales models and there has been no study comparing the results and no uniform conclusion on these issues, thus creating confusion for investors. The aim of this study is to investigate the performances of the hedonic, hybrid along with repeat sales models in the Chinese paintings market in terms of (i) the accuracy of the various models and (ii) the influence on art return estimation using the data from the Chinese most influential painter, Qi Baishi during the period from 2000 to 2016. Notably, it is the first time to combine main index construction models to provide a overall perspective on their performances in the Chinese paintings market.
The reason we choose data from Qi Baishi as the representative of the Chinese paintings market is that he has been known as the renowned Chinese painter of the twentieth century and one of the top-selling artists. According to the 2016 market annual report from Artprice, he ranked the third of the top world 500 artists by auction revenue and the twentieth of the top 100 auction performance in 2016. It is widely acknowledged that the price behavior of Qi Baishi’s artworks exhibits consistent patterns of the Chinese paintings market and reveals useful aspects in terms of performance.
This paper, using both 8,218 hedonic data and 2,207 repeat sales data from Qi Baishi sold at China and global auction houses over the period 2000–2016, examines the performances of three common price index models: the hedonic, hybrid and the repeat sales models. In the hedonic model, all available transaction data are pooled. The logarithm of price is regressed as the dependent variable on a set of value-determining attributes and time dummies. One advantage of the hedonic model is that it makes efficient use of available data and may therefore give more reliable estimates of price indices (Renneboog & Spaenjers, Citation2013). However, it is criticized for disadvantages of implicit functional form and the change of item characteristics over time (Collins, Scorcu, & Roberto, Citation2009; Scorcu & Zanola, Citation2011; Wolverton & Senteza, Citation2000; Worthington & Higgs, Citation2004).
In contrast, by confining the analysis to a series of consecutive transactions of a given item at least twice during the sample period, the repeat sales model estimates a price index by regressing the price change of each item on a set of dummy variables. Researchers, such as Goetzmann (Citation1993), Mei and Moses (Citation2002), Pesando (Citation1993), and Pesando and Shum (Citation2008), employ this method to art investments. The main strength of the repeat sales model is that it does not require the specific information on item attributes. Nevertheless, since this approach is based on only a part of total sales observations and this subset may not represent the full sample, the weaknesses of using repeat sales model include the difficulty of controlling for the market trend during the sample period, as well as suffering from selection issues (Biey & Zanola, Citation2005; Kraeussl & Elsland, Citation2008; Renneboog & Spaenjers, Citation2013).
The hybrid model, first advanced by Case and Quigley (Citation1991) and then extended by Carter Hill, Knight, and Sirmans (Citation1997), constructs the index by combining features of both the hedonic and repeat sales models. This method goes beyond the previous techniques since it allows one to correct for the effects of heterogeneity and serial correlation (Biey & Zanola, Citation2005). However, the shortcoming of the hybrid model is that it needs an extensive data-set together with the details on the concerned observations. In addition, the requirement of complicated econometric techniques and implementation skills also makes it difficult for some people using this method. Actually, the hybrid model are mainly used in the house market and only a few applications exist on the art market due to the difficulties to apply it to art items (Chanel, Gérard-Varet, & Ginsburgh, Citation1996).
Our paper makes several important contributions to the analysis of performances of the hedonic, hybrid, and repeat sales models in the Chinese paintings market. First, this is the first time to apply the hybrid model to construct the price index of the Chinese paintings market. Secondly, we present an overall perspective on the comparison of these price index models by examining the results concerning the accuracy and their effects on return estimation. Finally, we examine some fundamental characteristics of the Chinese artworks and their interactions on return performance. Consequently, our findings are expected to be helpful to the evaluation of model construction and art investments.
The remainder of the paper is organized as follows. In Section 2 we provide a brief explanation of each model. Section 3 describes details on the data-set. Empirical results are analyzed in Section 4. In the final Section 5 we summarize the key conclusions.
2. The models
2.1. The hedonic model
Let i = 1, … , N be the set of selling artworks, define Pit as the logarithm price of the painting i at time t; Xit as a K × 1 vector of characteristics for the painting i and α as a K × 1 vector of implicit prices of Xit; δ as a T × 1 vector of index numbers and Dit as a T × 1 vector of time dummies taking a value of one if a transaction on the painting i occurs in time t and zero otherwise, and εit as an error term. The hedonic regression equation can be expressed as:(1)
(1)
2.2. The repeat sales model
Define Pjt+s as the logarithm price of the painting j at time t + s. The price difference between two sales of the painting j can be written as:(2a)
(2a)
where , and
(2b)
(2b)
2.3. The hybrid model
Separate the whole set N into the subset of artworks that were sold only once during the sample period w = 1, …, I and the subset of artworks that were transacted more than once j = 1, …, J. The error term ɛit of Equation (1) is now decomposed into two parts: a time dependent random error term eit and a time independent error term ηi. The hybrid model can then be represented as:(3a)
(3a)
(3b)
(3b)
(3c)
(3c)
(3d)
(3d)
where all other variables are as previously defined.
Estimations of the hybrid model involve a series of intermediate steps. Note that specification error term ηw in the hybrid model is directly analogous to the unobserved heterogeneity term in the random effects model outlined in Greene (Citation2003). Under this assumption, the covariance matrix associated with the hybrid model can be written as:(4)
(4)
Following Jones (Citation2010), we estimate Equation (3d) using all J observations to obtain the residuals as a consistent estimate of and then apply a degrees of freedom correction:
(5)
(5)
Analogously, a consistent estimate of is found by estimating Equation (1) using all W observations to obtain the residuals and then applying a degrees of freedom correction:
(6)
(6)
The covariance matrix could then be constructed using
and
. Theoretically,
can be found, and according to Cholesky decomposition, we could find the P matrix, where
, and use this P matrix to transform the data prior to estimation via least squares. Practically, finding
and P directly requires intensive computations, and it is not possible for large data sets. Fortunately, the structure of
is such that non-zero elements only exist on the on-diagonal of each block, and that the non-zero values within each block are identical. This means that the inverse of a proportionally much smaller version of
can be found to obtain the values and the location of the non-zero elements (Fogarty & Jones, Citation2011).
3. Data
The data employed in this study are obtained from Artron.net and span the period 2000 to 2016. The Artron database contains a number of relevant information of artworks sold at China and global major auctions, (e.g. sales record, artist’s name, title of the work, year of production, materials, sales date, prices, dimension, signed or not). Buyers and sellers’ transaction fees paid to auction houses are included in prices and are recorded in both renminbi (RMB) and local currencies.
Specifically, the explanatory variables Xit and Dit included in models are:
3.1. Dimension
The variable area is a product of both width and length, which is measured in square meters. To examine whether the price increases with a diminishing effect, the variable area2 is also included.
3.2. Medium
In our study, the Chinese artworks are categorized in two different mediums. The categories are paper and silk, with silk as the reference variable.
3.3. Mounting
Normally, the Chinese artworks can be mounted in several ways. We specify dummies album of sheets, folding fan, folding screen, framed, hand scroll, and hanging scroll to reflect in which type the painting is adopted, with album of sheets as the reference variable.
3.4. Type
Traditional Chinese artworks are done either in black ink or colored. In this case, two dummy variables ink (as the reference variable) and color are created to identify whether the painting is in ink or colored.
3.5. Auction houses
In this paper, the auction house dummies are specified by beijing hanhai, beijing kuangshi, christie, guardian, poly, and sotheby, which are main dominant auction houses in China and worldwide. We assign the dummy variable to take the value of one if the painting is classified into any of these six auction houses. Other auction houses are assigned to the references and take the value of zero.
3.6. Period
Both semiannual and annual period are applied in this paper. On the semiannual basis, a series of dummy variables, Dt, with t = 2000s, ..., 2016a, are introduced for each semiannual between the spring of 2000 and the autumn of 2016, regarding the spring of 2000 as the baseline variable and standardized to 100 in the price index. Similarly, t = 2000, ..., 2016 are introduced annually, considering 2000 as the baseline variable and standardized to 100 in the price index.
Table summarizes the descriptive statistics of artworks sold. The number of samples, means, and standard deviations are presented, along with the minimum and maximum. The average artwork price of Qi Baishi from 2000 to 2016 is 1,742,045 RMB, ranging from 330 RMB to 425,500,000 RMB. According to the dimension, the average area is 0.3501m2, with the standard deviation of 0.3068. There are 8,163 artworks which have been painted on paper, accounting for 99.33% of the total samples. Among all mounting types, hanging scroll is the most prevalent (5,115), followed by framed (2,320), whereas there are only 27 artworks mounted in hand scroll. Furthermore, the artworks in color (6,254) are approximately three times more than those in ink (1,964).
Table 1. Descriptive statistics
Table includes the statistics of artworks according to different auction houses as well. Of the six dominant auction houses, guardian is the most popular, with the number of 2,111. Relative to christie (608) and sotheby (495), poly (1,108), beijing hanhai (943) and beijing kuangshi (632) achieve higher proportions, suggesting that the majority of Chinese artworks of Qi Baishi has been sold in China.
4. Empirical results
4.1. Comparison of three price index models
The performances of three models are now evaluated. Table reports the coefficient estimates and corresponding standard errors for semiannual and annual periods using the White (Citation1980) heteroscedasticity-robust procedure. Columns (1) and (2) reveal the hedonic regression results. Results of the repeat sales method are shown on Columns (3) and (4). The hybrid model is estimated using the unrelated regression methodology and displayed in columns (5) and (6).
Table 2. Empirical results
To evaluate and compare price index models in depth, we then use three key measures to assess the precisions of three price index models:
(1) | The mean squared error (MSE) based on an out-of-sample forecast (Goh, Costello, & Schwann, Citation2012). | ||||
(2) | The squared correlation between the actual and predicted values (Case & Szymanoski, Citation1995). | ||||
(3) | The width of a 95% confidence interval (Case & Szymanoski, Citation1995). |
4.1.1. The mean squared error (MSE) based on an out-of-sample forecast
The first measure we adopt is the MSE based on an out-of-sample forecast. This procedure, proposed by Goh et al. (Citation2012), is simple to deal with and effective in examining the precisions of the price index models.
Specifically, for each price index model, we split its data-set into two sub-samples. The sub-sample 1 consists of 70% of the full sample and is used to compute the indices. The parameter estimates obtained in the first sub-sample are then applied to the sub-sample 2, which involves the remaining 30% of the full sample and is used to forecast prices for each observation. In this way, there are two sets of prices in the sub-sample 2: one is the forecast price, , and the other is the actual price yi. To evaluate their relative precisions, we then use the standard forecast analysis statistics in terms of the MSE for each price index model, which is represented by
. In this equation, n denotes the number of observations used to forecast the price,
is the forecast price for observation i, and yi is the actual price of observation i. Since the MSE is combined with both the bias and the variance, a lower MSE value for a particular price index generally indicates a better accuracy.
Table presents the estimated semiannual and annual MSEs for the three price index models, respectively. It reveals that at both levels of time frequency, the repeat sales model generates the lowest MSEs. However, Case and Szymanoski (Citation1995) note that the fact that standard deviation of the disturbance term in the repeat sales model smaller than those in the hedonic and hybrid models could be explained for two reasons. One reason is that the pure random noise component of the disturbance term is assumed to be positively related to the length of time elapsed between transactions, which is likely to be smaller for the repeat sales model than for other two model. The other reason is that the repeat sales model normally incorporates additional information on repeat transactions that are ignored in the hedonic model. Therefore, it is probably that the smaller estimated MSEs of the repeat sales model could be interpreted merely as a reflection of the smaller standard deviation of the disturbance term rather than an evidence that the repeat sales model is more precise than other two models.
Table 3. Mean squared error (MSE) of the three models
Additionally, although the hybrid model stands out as a relative accurate price index model in most housing literatures (Jones, Citation2010; Goh et al., Citation2012; Quigley, Citation1995), that is not the case in our study. Even if the hybrid model has the advantage of combing features of both the hedonic and repeat sales model, it yields the highest estimated MSEs at both levels of time frequency (1.5265 semiannual and 1.5554 annual), underperforming the other price index models. Biey and Zanola (Citation2005) also find that the standard deviation of the disturbance term of the hybrid model are higher than those of the hedonic and repeat sales models when investigating the performance of Picasso prints and attribute to the limited number of repeat sales in the sample. Similarly, our finding provides a certain support to Meese and Wallace (Citation1991), who argue that the hybrid model appears not to produce reliable estimates of the price indices. Figure depicts the MSEs of the three models based on an out-of-sample forecast at both semiannual and annual time frequencies, where the red line and the blue line represent the forecast value and the standard error, respectively.
4.1.2. Correlation between actual and predicted values
The second measure commonly used as means of assessing price index models is the correlation between actual and predicted values from econometric models, which provides a direct measure of the reliability. Normally, a high correlation can be interpreted as an indication of little error in the model, while a low correlation would indicate a large amount of error.
Table presents the squares of the estimated correlation coefficients between actual and predicted values based on the hedonic, hybrid, and repeat sales models (). As shown, the hybrid model generates overwhelming higher correlations than the corresponding hedonic and repeat sales models, demonstrating its more accurate prediction ability. Moreover, correlations of the three price index models on the basis of semiannual frequency are slightly higher than those on the basis of annual frequency, suggesting relative better predictions.
Table 4. Correlations between actual and predicted values of various models
4.1.3. Width of a 95% confidence interval
The third measure used is the width of a 95% confidence interval, which reflects both the estimated standard deviation of the disturbance term and the precision with which the individual parameters of the model. In view of this, we calculate the estimated semiannual and annual price indexes along with their corresponding 95% confidence intervals, which are reported in Table . Overall, the hedonic, hybrid, and repeat sales models show a similar trend in Table : there has been a noticeable increase from the year 2000 to 2011, and then followed by a strongly fluctuation till 2016. Such situation could also be demonstrated in Figure , which presents the indices constructed with three models at the annual and semiannual time levels, respectively. Figure reveals that in general, the patterns of three indices seem almost alike, although the repeat sales index always tracks below the hedonic and the hybrid indices at different time levels.
Table 5. Annual and semiannual prices indexes
Figure 2. Annual and semiannual hedonic, repeat sales and hybrid price indexes of Qi Baishi during the period from 2000 to 2016.
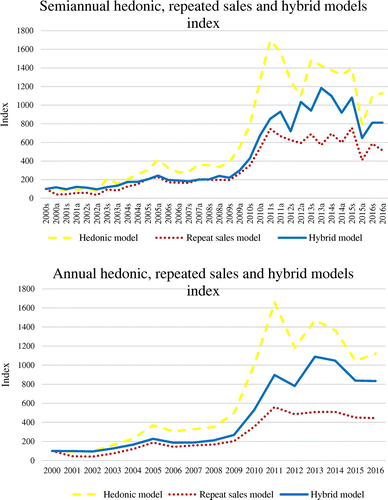
To make the estimate efficiency comparison across models much clearer, Figure plots the 95% confidence interval for each model around a constant index value of unity. The dashed lines, dotted lines, and continuous lines represent the 95% confidence intervals (the level of price volatility) estimated from the hedonic model, the repeat sales model and the hybrid model, respectively. In line with our expectation, for both time levels, the hybrid model provides the narrowest confidence interval among three models. This result, combined with the result of the correlation between actual and predicted values, suggests that the hybrid model to some extent provide the most efficient estimates, which confirms the findings by Biey and Zanola (Citation2005) and Fogarty and Jones (Citation2011).
4.2. Evaluations of three price index models
Besides the estimate efficiency, we also compare different returns evaluated by different models. In general, the estimated return on art varies with data, methodology, and the time period under consideration (Ashenfelter & Graddy, Citation2003). Specifically, an important difference between the repeat sales model and others is that the repeat sales model relies on only a subset of transactions and the utilized sample has been relatively small and selective, which may have a significant impact on the return estimates. Table presents the estimated returns for the three price index models during the period 2000 to 2016. The highest return has been achieved by the hedonic model, which yields 0.0734 semiannually and 0.1511 annually. The return differences between the hybrid model and the hedonic model are not distinct, which the former is on average 13% lower than the latter in both time levels. The repeat sales model performs the worst, obtaining the lowest estimated returns and the highest risk at both time levels. By contrast, the hybrid model provides the lowest estimated volatility even at different time levels, notably surpasses all other models. This result somewhat contradicts the finding of Renneboog and Spaenjers (Citation2013), which argues that, with respect to artworks, the hedonic model has found somewhat lower returns than the repeat sales model. Considering that only part of Qi baishi’s artworks appear in the art market more often, those artworks with high appreciation and esthetic value by the collectors may be transacted infrequently. For this reason, it is possible that the return estimates derived from the repeat sales model might be lower than those obtained using other models.
Table 6. Annual and semiannual returns
5. Conclusions
In this paper, we review both the advantages and disadvantages of the hedonic, hybrid and repeat sales models and apply a series of techniques to examine their performances in the Chinese paintings market by analyzing the artworks of Qi Baishi over the period 2000–2016. Applying the mean squared error (MSE) technique, the result shows that the repeat sales model outperforms alternative models. However, according to the correlations and width confidence intervals, the hybrid model provides the most efficient estimates, making it a more attractive approach for measuring price changes in the Chinese paintings market. Additionally, the empirical result reveals that, the repeat sales model gives the lowest estimated returns as well as the highest volatility among three models. Our findings, not only have important implications in identifying the accuracy of index models, but also provide a certain supplement to art investment studies.
It is worth noting that there exist some limitations in our paper. Although the hybrid model has the best explanatory power in terms of correlations and width confidence intervals, it ranks the worst in the MSE measure. In other words, none of the price index models seems to be consistently superior accurate to the others. Apart from the data used in our paper, absence of a convincing proxy for measuring index accuracy and pooling data together across time may also result in such inconsistency (Ansah, Citation2013). We fully expect that, in further studies, there would be a methodology demonstrated to provide more accurate and robust estimations in the price index construction and be applied in more general settings.
Funding
The authors received no direct funding for this research.
Additional information
Notes on contributors
Fang Wang
Fang Wang is a Doctoral candidate of Finance in the Department of Finance, Antai College of Economics and Management, Shanghai Jiao Tong University, Shanghai, China. Her researches are related to alternative investments, and specially focused on the investment in the Chinese art market.
References
- Ansah, A. O. (2013). Construction of property price indices. Property Management, 31(2), 115–131.10.1108/02637471311309427
- Ashenfelter, O., & Graddy, K. (2003). Auctions and the price of art. Journal of Economic Literature, 41(3), 763–787.10.1257/jel.41.3.763
- Biey, M. L., & Zanola, R. (2005). The market for picasso prints: A hybrid model approach. Journal of Cultural Economics, 29(2), 127–136.10.1007/s10824-005-8133-x
- Carter Hill, R., Knight, J. R., & Sirmans, C. F. (1997). Estimating capital asset price indexes. The Review of Economics and Statistics, 79(2), 226–233.10.1162/003465397556818
- Case, B., & Quigley, J. M. (1991). The dynamic of real estate prices. The Review of Economic and Statistics, 73(1), 50–58.10.2307/2109686
- Case, B., & Szymanoski, E. J. (1995). Precision in house price indices: Findings of a comparative study of house price index methods. Journal of Housing Research, 6(3), 483–496.
- Chanel, O., Gérard-Varet, L., & Ginsburgh, V. (1996). The relevance of hedonic price indices: The case of artworks. Journal of Cultural Economics, 20(1), 1–24.10.1007/s10824-005-1024-3
- Collins, A., Scorcu, A., & Roberto, Z. (2009). Reconsidering hedonic art price indexes. Economic Letters, 104(2), 57–60.10.1016/j.econlet.2009.03.025
- Fogarty, J. J., & Jones, C. (2011). Return to wine: A comparison of the hedonic, repeat sales and hybrid approaches. Australian Economic Papers, 50(4), 147–156.10.1111/aepa.2011.50.issue-4
- Goetzmann, W. (1993). Accounting for taste: Art and the financial markets over three centuries. American Economic Review, 83(5), 1370–1376.
- Goh, Y. M., Costello, G., & Schwann, G. (2012). Accuracy and robustness of house price index methods. Housing Studies, 27(5), 643–666.10.1080/02673037.2012.697551
- Greene, W. H. (2003). Econometric analysis (International 5th ed.). New Jersey: Prentice Hall.
- Jones, C. (2010). House price measurement: The hybrid hedonic repeat-sales method. The Economic Record, 86(272), 95–97.10.1111/ecor.2010.86.issue-272
- Kraeussl, R., & Elsland, N. (2008). Constructing the true art market index-A novel 2-step hedonic approach and its application to the German art market. CFS Working Paper, No. 2008/11. Retrieved from http://nbn-resolving.de/urn:nbn:de:hebis:30-54393
- Meese, R. A., & Wallace, N. E. (1991). Nonparametric estimation of dynamic hedonic price models and the construction of residential housing price indices. Journal of the American Real Estate and Urban Economics Association, 19, 308–332.10.1111/reec.1991.19.issue-3
- Mei, J., & Moses, M. (2002). Art as an investment and the underperformance of masterpieces. American Economic Review, 92(5), 1656–1668.10.1257/000282802762024719
- Pesando, J. E. (1993). Art as an investment: The market for modern prints. American Economic Review, 83(5), 1075–1089.
- Pesando, J. E., & Shum, P. M. (2008). The auction market for modern prints: Confirmations, contradictions, and new puzzles. Economic Inquiry, 46(2), 149–159.10.1111/ecin.2008.46.issue-2
- Quigley, J. M. (1995). A simple hybrid model for estimating property price indices. Journal of Housing Economics, 4(1), 1–12.
- Renneboog, L., & Spaenjers, C. (2013). Buying beauty: On prices and returns in the art market. Management Science, 59(1), 36–53.10.1287/mnsc.1120.1580
- Scorcu, A., & Zanola, R. (2011). The right price for collectibles: A quantile hedonic regression investigation of Picasso artworks. Journal of Alternative Investments, 14(2), 89–99.10.3905/jai.2011.14.2.089
- White, H. (1980). A heteroscedasticity-consistent covariance matrix estimator and a direct test for heteroscedasticity. Econometrica, 48(4), 817–838.10.2307/1912934
- Wolverton, M. L., & Senteza, J. (2000). Hedonic estimates of regional constant quality house prices. Journal of Real Estate Research, 19(3), 235–253.
- Worthington, A. C., & Higgs, H. (2004). Art as an investment: Risk, return and portfolio diversification in major painting markets. Accounting and Finance, 44(2), 257–271.10.1111/acfi.2004.44.issue-2