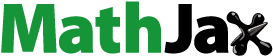
Abstract
In this paper, we examine the dynamic nature of equity market integration for the South Asian countries. The daily data for local equity indices are used from 6 January 2004 to 31 March 2015. Copula GARCH models and Diebold and Yilmaz methodology have been employed to study the inter-temporal process of equity market integration. Empirical results show that the sample countries of the region exhibit very little or no levels of integration between them. Equity portfolio flows within the South Asian region reconfirms this trend for low integration in the region. Further, trend analysis of the fundamental determinants of financial integration for the SAARC countries was performed and the same was compared with its neighbouring regional economic bloc in Asia i.e. ASEAN + 6. It indicated that SAARC countries have to show sincere political commitment and require collaboration in efforts of policy realignment to work on their governance parameters, improve on their trade linkages and trade tariffs and develop their equity market infrastructure to achieve higher levels of financial integration. The paper contributes to the International Finance literature, especially dealing with regional economic blocs and has important implications for policy-makers, portfolio managers and academia.
Public Interest Statement
Regional integration reinforces ties amongst a set of diverse economies so as to provide avenues for economic opportunities, sustainable growth, financial stability and inclusive development. South Asia, a diverse region of heterogeneous countries, formally initiated regional integration process with the formation of South Asian Association of Regional Cooperation (SAARC) in 1985. Until late 1970s, these countries followed high protectionist trade policies and maintained strict capital controls. But with the changing global economic landscape, SAARC countries have embraced upon outward oriented strategies and have increasingly acknowledged that regional approaches are quintessential to accomplish their developmental challenges. Ever since the formation of SAARC, South Asia has remained one of the least integrated regions in the world. In this context, we examine the dynamic nature of equity market integration for the South Asian countries. Empirical results show that the sample countries of the region exhibit very little levels of integration between them.
1. Introduction
The Association of South-east Nations (ASEAN)Footnote1 grouping is the first of the regional integration initiative in Asia which helped the member countries in achieving sustained economic growth since the early 1980’s, leading to an increased share in the global gross domestic product and a rise in their per capita income. International financial market liberalization along with outward-oriented strategies have significantly increased the degree of capital mobility in the Asian countries since 1990s (Guillaumin, Citation2009). The Asian countries have come out of the Asian Financial Crises of 1998 by strengthening their macroeconomic fundamentals and improving their fiscal conditions thereby promoting cross-border trade through export-led growth model leading to improved efficiency and higher productivity. In contrast, until the late 1970s, high protectionist trade policies by maintaining tariff and non-tariff barriers, heavy state intervention in economic activity, strict exchange rate controls, discouragement of foreign capital were some of the few measures being practiced in the South AsianFootnote2 countries which led to a contraction in their economic growth. This heterogeneous and unique region encompassing small and big economies in different stages of their economic development was also embroiled in political conflicts which slowed its growth leading to high levels of poverty (home to 44% of world poor, Source: World Bank) amongst its large population and low levels of human development.
Post the 1990’s, the South Asian countries have embraced upon outward-oriented strategies and have increasingly acknowledged that regional approaches are quintessential to accomplish their developmental challenges. Regional cooperation was formally initiated in South Asia with the formation of a trade bloc named as South Asian Association of Regional Cooperation (SAARC) in 1985. With the ratification of the South Asian Free Trade Area (SAFTA) agreement in 2004, the trade integration has all but begun in South Asia though the pace of economic reforms is far from desirable. But as critical as trade integration is, the policy-makers of the region are convinced that an efficient, well-regulated and integrated capital markets are quintessential for facilitating savings, investments and economic growth within the region. A number of initiatives have been carried out under the SAARC umbrella fostering regional capital market integration SAARCFINANCE, South Asian Federation of Exchanges (SAFE) and South Asian Securities Regulators’ Forum (SASRF).Footnote3
Although SAARC comprises countries which share common history, heritage, culture and more importantly contiguity but political differences and increasing mistrust amongst member nations (most specifically between India and Pakistan) have not permitted SAARC initiatives to achieve desired results and are major impediments in the way for realizing stronger integration amongst the South Asian countries. Given the increasing emphasis on closer regional co-operation across the world, it is an opportune time for the political leadership of the SAARC countries to steer their way through their political standoffs and embrace an open regionalism and institutional change which can help make South Asia an active partner in Asian economic integration and global economy. Given the fact that the twenty-first century is termed as the “Asian Century”, ASEAN or the bigger ASEAN + 3/6 is another adjoining and competing regional economic bloc to SAARC in Asia with a wider participation base and long history. Thus, the very relevance of the SAARC bloc in the Asian context along with the process of equity market integration needs to be studied and compared with its adjoining regional bloc in Asia.
The study has implications for the various observer countries, particularly China who is continuously pitching in to become a full member state of SAARC so as to understand the dynamics of the integration process in this region. SAARC has dedicated the decade 2010–2020 to the intra-regional connectivity which can help to propel growth, peace and prosperity in the region. India being the largest economy in terms of its economic growth, population and territorial size amongst the SAARC members, enjoys the most prominent position in the region bordering every SAARC country. India cannot aspire to become a regional and/or a global superpower unless it has a clear understanding of either having made SAARC functional in its objectives or leveraging the multilateral platform of ASEAN + 6 for its economic growth and development.
Stock market integration amongst the major economic blocs has received considerable attention from researchers over time. Researchers have documented varying degree of equity market integration for different regions. For the European Union; Fratzscher (Citation2001) employed GARCH model with time varying coefficients to analyse the impact of EMU on integration process of European equity markets and finds that European equity markets have become highly integrated only since 1996 by the drive towards EMU. Kim, Moshirian, and Wu (Citation2005) estimated the time varying correlation using DCC-EGARCH model to examine the influence of EMU on stock market integration and find shift in European stock market integration. Bley (Citation2009) examined the factor that determines the dynamics and contemporaneous interactions of Euro stock markets at the country and economic sector level and revealed the time varying nature of the financial market integration process. Mylonidis and Kollias (Citation2010) show that the introduction of the euro epitomizes European economic integration in the major European stock markets in the first euro-decade, with the German and French markets the ones with a highest degree of convergence. Using an asset pricing model, Hardouvelis, Malliaropulos, and Priestley (Citation2006) find full integration amongst the euro-area stock markets by the end of the 1990s. However, Morana and Beltratti (Citation2002), Fratzscher (Citation2002), Kim et al. (Citation2005) and Bartram, Taylor, and Wang (Citation2007) report that the integration in the region is evolving over time and far from being complete. Sehgal, Gupta, and Deisting (Citation2016) used a battery of price-based indicators to conform that there is segment of levels of equity market integration for EMU countries wherein important and prominent countries like Germany, France, Netherlands, Italy, Spain and Belgium exhibit strong financial integration while others show relatively low levels of integration.
With regards to Asian studies, Cavoli, Rajan, and Siregar (Citation2004) surveyed recent academic and empirical literature and show evidence of limited extent of financial market integration in East Asia. Huyghebaert and Wang (Citation2010) examined the stock market integration for East Asian economies (China, Hong Kong, Taiwan, Singapore, South Korea and Japan) for a data spanning from July 1992 to June 2003 and used co-integration and VAR analysis to confirm limited equity market integration. Boubakri and Guillaumin (Citation2015) studied the dynamics of equity market integration in the Asian region countries (China, South Korea, Hong Kong; China, Indonesia, Japan, Malaysia, Philippines, Singapore and Thailand) for the period January 1994 to November 2008. Results from the ICAPM methodology indicated that though the sample stock markets were partially segmented within the region. Sehgal, Pandey, and Deisting (Citation2016) examined equity market integration for the ASEAN + 6 countries from January 1999 to March 2015. Copula GARCH models confirmed that the sample countries exhibit varying degrees of integration with the Asian benchmark.
As for the NAFTA region, Gilmore and McManus (Citation2004) used Johnson co-integration and VECM technique to document that implementation of NAFTA has promoted greater equity market integration between the three North American countries. Lahrech and Sylwester (Citation2013) investigate the impact of NAFTA on U.S., Canadian and Mexican equity market linkages from December 1988 to July 2006. They employ a dynamic conditional correlation model to the stock markets of these three countries and confirmed linkages between the equity markets after the NAFTA agreement. Lahrech and Sylwester (Citation2011) examined Latin American equity markets of Argentina, Brazil, Chile and Mexico from 1994 to 2008 using DCC GARCH models and documented that these markets have become more integrated with the US equity market. Basher, Salem, and Hui (Citation2014) documented that the stock markets of the six GCC (Gulf Cooperation Council) countries have demonstrated significant integration over the past decade using copula models for data spanning 2004–2013. Yu and Hassan (Citation2008) investigate financial integration of MENA region to facilitate a more in-depth exploration of the structure of interdependence and transmission mechanism of stock returns and volatility between MENA and world stock markets and documented greater integration using GARCH models.
While the literature on equity market integration for major regional blocs is extensive, only a handful of studies have assessed regional stock integration of SAARC countries that also predominantly using Johnson Co-integration methodology. Using Johnson Co-integration, Narayan, Smyth, and Nandha (Citation2004), Perera and Wickramanayake (Citation2012), Latif, Rizvi, Mubin, and Iqbal (Citation2014) documented some linkages between the stock markets of Pakistan, Bangladesh, India and Sri Lanka, while Ali, Hussain, and Islam (Citation2014) and Singhania and Prakash (Citation2014) found out little or no association between sample stock markets.
This paper is based on two strands of literature. First set of literature is based on how to measure financial integration. Two broad categories of measures of financial integration have been in vogue: price-based and quantity-based measures.Footnote4 On the price-based measures, there is a heavy empirical literature on assessing the level of financial integration using the traditional beta and sigma convergence (Adam, Jappelli, Menichini, Padula, & Pagano, Citation2002; ECB, Citation2004; Rizavi & Naqvi, Citation2011). Early investigations into the linkages between the two markets used the VAR models to study co-movement of stock market returns. Contemporary literature has moved on to modern time series approaches such as dynamic co-integration approach (Mylonidis & Kollias, Citation2010) and MGARCH models (BEKK model, DCC models and its extensions mainly Asymmetric DCC-GARCH of Cappiello, Engle, & Sheppard, Citation2006 and Threshold DCC-GARCH of Pesaran & Pesaran, Citation2007) to study the dynamic process of integration.Footnote5 Recently, copula-based models have been used to study the asymmetric nature of dependence between financial market returns (Patton, Citation2006a; Rodriguez, Citation2007). The copula-GARCH (C-GARCH) model is a multidimensional GARCH process that uses a copula function to model the interlinkages. The application of the C-GARCH model has recently attracted increased academic attention (Basher et al., Citation2014; Peng & Ng, Citation2011; Sehgal, Pandey, et al., Citation2016; Yang & Hamori, Citation2013; Yang, Cai, Li, & Hamori, Citation2015). The quantity-based measures assess the degree of integration based on cross-country equity portfolio holding of assets and securities. The second strand of literature is related to the fundamental determinants of stock market integration.
Assets in financial markets tend to show asymmetric dependence as opined by Chollete, Heinen, and Valdesogo (Citation2009). Besides, data generation process of returns on financial market indices may be non-linear, non-stationary and/or heavy tailed. Moreover, the marginal distributions may be asymmetric, peaked and/or show conditional heteroskadasticity. GARCH family models have been used to address conditional heteroskadasticity and excess kurtosis as described by Engle (Citation1982) and Bollerslev (Citation1987) but mostly considering elliptical distributions. The use of copula-based models has become common practice in financial literature when it comes to studying asymmetric dependence structures between random variables. They do not require the assumption of joint normality and allow us to decompose a high-dimensional joint distribution into its marginal distributions (using GARCH models) and use a copula function to link them together.
In light of the above literature, the following objectives of the paper are set out: (a) To empirically evaluate the dynamic co-movements between the equity market indices of the member countries of the SAARC region using copula GARCH models and empirically examine the connectedness of these equity markets by Diebold and Yilmaz (Citation2009, Citation2012) methodology (price-based approaches) (b) To examine the state of equity market integration of the South Asian countries using quantity-based approaches (c) To study the trends of the fundamental determinants of financial integration for the SAARC member countries and compare the same with ASEAN + 6, its neighbouring regional economic bloc in Asia i.e. (d) Given the results, the paper provides policy suggestions for SAARC in general and India in particular.
The present study makes an important contribution to the existing literature which is very thin and limited in scope for the South Asia region by employing Copula GARCH models and Diebold and Yilmaz methodology on equity market price data to show that albeit after decades of formation of the SAARC regional bloc, the member countries exhibit little dependences with each other thereby confirming low regional integration. The data on equity portfolio flows reconfirms this trend for low integration in the region. From a comparative analysis with the adjoining and competing regional economic bloc in Asia i.e. ASEAN + 6, it was found out that equity market integration in the SAARC members lagged their ASEAN + 6 counterparts owing to poor governance quality, lower trade linkages, higher trade tariffs and less sophisticated equity markets with relatively weaker institutional and regulatory architecture. We believe the present study would be instructive and complementary to the existing literature on financial integration and provide strong arguments for the SAARC group members and specifically for India to come out of their political standoffs and collaborate in efforts of policy realignment to bring dynamism into this region thereby promoting regional integration.
The rest of the paper is organized as follows: Section 2 presents the Data, while Section 3 provides the Methodology related to Price-based and Quantity-based approach to evaluate equity market integration amongst the SAARC members. Section 4 discusses the empirical results of the econometric methodology used for the analysis, while Section 5 includes the discussion on the fundamental determinants of the equity market linkages amongst the SAARC members. Section 6 provides summary and policy suggestions for the policy-makers of the SAARC region to further strengthen regional integration.
2. Data descriptions and its sources
We begin our analysis on the daily stock market benchmark index closing prices retrieved from Bloomberg for SAARC member countries for the period from 6 January 2004 to 31 March 2015. The starting date has been selected keeping in mind the date when the SAFTA agreement was reached (6 January 2004) which was a landmark event in the process of regional cooperation in South Asia. Benchmark indices so selected for the analysis are provided in Table .
Table 1. Description about benchmark equity indices of SAARC Countries
Dhaka Index was discontinued on 31 July 2013 and the benchmark index now used in empirical literature for Bangladesh is Dhaka Stock Exchange Broad Index (DSEX) data for which is available from 28 July 2013. Hence, we perform the splicing process to get the values of the Dhaka Index till 31 March 2015. The values of the closing prices of Sri Lankan benchmark index are available from 17 December 2004. Thus, we have covered six South Asian countries in our study of the total eight who constitute the SAARC as no data were available for Afghanistan and Bhutan. As returns of the local equity indices would have been impacted by local currency movements against the US dollar, hence to capture the true market movements, all price indices are denominated in the local currency. The MSCI USA is included as a proxy for global factor as in prior research (Baele et al., Citation2004; Bartram et al., Citation2007; Gupta et al., Citation2015). We take the natural logarithm of the daily closing values. Daily returns are then computed as the first difference of the log-transformed series.
For the quantity-based measures, we construct the annual portfolio equity inflows for each SAARC member countries from the SAARC region as well as ASEAN + 6 region for the period 2004–2014. Similarly, we construct portfolio outflows from each SAARC member country to the SAARC and the AEAN + 6 regions. The portfolio flow data were sourced from IMF-CPIS (International Monetary Fund-Coordinated Portfolio Investment Survey) database. The quantity-based measures have been constructed for the same six countries (Bangladesh, India, Maldives, Nepal, Pakistan and Sri Lanka) that were a part of the empirical analysis performed using the price-based measures as no data were available for Afghanistan and Bhutan. Finally, for the fundamental determinants of equity market integration, a comprehensive set of factors was constructed (refer Table ) after an exhaustive review of literature belonging to three broad set of categories i.e. Macroeconomic, Trade and Market-based category. The data for these factors were sourced for the SAARC member countries subject to their data availability from 2004 to 2015. These variables were predominantly of annual frequency except for volatility of forex rate, volatility of domestic market index return, domestic growth opportunities and local stock market conditions which were of daily frequency. The daily data were averaged over the year and it was not annualized as even a common scaling factor of 250 trading days would not have led to a change in its relative position across the sample.
Table 2. Fundamental determinants of equity market integration
3. Methodology
3.1. Price-based measures
3.1.1. Copula GARCH Approach
Multivariate normality assumption is not suitable for measuring the association of equity market returns between two countries, especially with respect to their asymmetric co-movements or contagion effects (Longin & Solnik, Citation2001; Poon, Rockinger, & Tawn, Citation2004). Copula model have been preferred over multivariate GARCH models to model the dependence as the dependence structure estimated via copulas (which captures non-linear dependence) is more robust because it separates the dependence structure from the choice of margins (Da Silva Filho, Ziegelmann, & Dueker, Citation2012). Also, based on Sklar’s Theorem (Sklar, Citation1959) which helps in separating the marginal and joint distributions, the copula method makes the estimation process more flexible. Traditional multivariate GARCH models (DCC GARCH, ADCC GARCH, etc.) require that all random variables have same marginal distribution and also some assumptions of the margins which restraint their usage. Also for multivariate GARCH models, there are many parameters which make the model less flexible and parameter estimations more difficult. Other advantages of using copula models is that tail dependence measured by copulas allows investors to measure the probability of simultaneous extreme losses (Ning, Citation2010). Furthermore, copulas are invariant to increasing and continuous transformations and the results are the same for the asset returns as for the logarithm of the returns (Reboredo, Citation2011). At the same time, the copula function can provide us not only the degree of the dependence, but also the structure of the dependence between financial assets (Ning, Citation2010). Patton (Citation2006a) extended this methodology by adding a time varying specification to capture the dependence over time.
Let Rcountry i,t and Rcountry j,t be random variables that denote country i’s stock index returns and country j’s stock index returns, respectively, at period t, with marginal conditional cumulative distribution function Ucountry i,t = Gcountry i,t (Rcountry i,t|ɸt − 1) and Ucountry j,t = Gcountry j,t (Rcountry j,t|ɸt − 1), where ɸt − 1 denotes past information. Then, the conditional copula function Ct (Ucountry i,t, Ucountry j,t |ɸt − 1) can be written using the two time varying cumulative distributive functions. Extending Sklar’s theorem, bivariate conditional cumulative distributive function of random variable Rcountry i,t and Rcountry j,t is:(1)
(1)
3.1.1.1. Marginal specification
We investigate the integration of each sample country’s stock index returns with each other by combining the copula functions, described above, with a GARCH-type model of conditional heteroscedasticity. In this paper, we adopt EGARCH(1, 1) model (Nelson,Citation1991) for modelling of conditional volatility to capture the asymmetric impacts of shocks or innovations on volatilities and to avoid imposing non-negativity restrictions on the values of GARCH parameters. EGARCH(1, 1) model is chosen as the preferred model in the interest of parsimony of parameters (see Kim & Wang, Citation2006). The mean equation used for country i’s returns and Asian benchmark returns is as follows:
If εt = σtzt where zt is standard Gaussian,
Here, Rusa is the return of the USA index and is used as a global factor. It is well known that many financial time series are non-normal and tend to have a fat tail behaviour (Mandelbrot, Citation1963). Thus, a GARCH is formulated after factoring in the mean spillover effects from the US which proxies for global effects (see Dungey, Fry, & Martin, Citation2003; Samitas & Kenourgios, Citation2007; Sehgal, Gupta, et al., Citation2016). The lagged US returns proxy for global factor and capture the global mean spillover effects. In order to better capture the skewed and fat tails characteristic, Bollerslev (Citation1987) suggests the use of the conditional Student t distribution for the error term. We further obtain the standardized residuals from this marginal specification.
3.1.1.2. Copula models for dependence
Having estimated each of the marginal distribution, we now apply the probability integral transform to convert the standardized residuals of the marginal distribution into Uniform (0, 1) distributions. To describe the symmetric and asymmetric dependence structure between the uniform distributions of each sample country returns with that of the other, the study uses two Elliptical (Gaussian and Student t) and two Archimedean’s (Clayton and Gumbel) family of copula models. The Gaussian copula is symmetric and has no tail dependence whereas Student t copula can capture extreme dependence between variables. The Gumbel copula exhibits greater dependence in the upper tail than in the lower, whereas the Clayton copula exhibits greater dependence in the negative tail than in the positive tail. The Elliptical copula models (Gaussian and Student t) are most popular in Finance literature due to the ease with which they can be implemented. Because the nature of copulas can be either time invariant or time-variant, hence we include both for our empirical analysis. A detailed exposition of these copulas are provided in Appendix A for reference.
3.1.1.3. Estimation of copula parameters
Parameters are normally estimated in two steps, first for the marginal and second for the copula. The approach is similar to the Inference function for Margin (IFM) method. IFM method is superior to Exact Maximum Likelihood (EML) as the latter needs expensive computation more so for higher dimensions. In IFM method, parameters of the marginal distributions can be estimated before those of the copula functions whereas the EML method requires both to be estimated simultaneously.
3.1.1.4. Goodness of fit tests
Besides the log likelihood ratios, in order to check the quality of the overall fit of the family of copula models, goodness of fit test of (Genest, Rémillard, & Beaudoin, Citation2009) which is based on a comparison of the distance between the estimated and the empirical copula:(3)
(3)
The test statistic is based on Cramer–Von Mises distances defined as:(4)
(4)
Large values of the statistic Sn leads to rejection of the null hypothesis that the Copula C belongs to the class C0. A multiplier approach is used to find the p-values associated with the test statistics (Kojadinovic & Yan, Citation2011). The highest p-value indicates that the distance between the empirical and the estimated copulas is the smallest and that the copula in use provides the best fit (Aloui, Hammoudeh, & Nguyen, Citation2013).
3.1.2. Diebold and Yilmaz approach
We examine the connectedness of the South Asian equity markets by quantifying the contribution of shocks from each member country to other member countries’ at different points in time. The daily data of equity returns have been used to examine the connectedness of the stock markets of the region so as to provide support to the copula results. While copulas provide only the estimates of conditional correlation, this methodology allows us to quantify the cross-market directional returns spillovers (pairwise spillovers) thereby providing further insights into the equity market linkages for the member countries. This methodology is superior to VAR methodology earlier proposed by Sims, in 1980, as it can be used to measure the return spillovers both within and across countries, revealing spillover trends, cycles, bursts, etc. To assess the degree of connectedness in regional equity markets we use Diebold and Yilmaz (Citation2009, Citation2012) methodology which is based on dynamic variance decompositions from vector autoregression (VAR) applied to equity returns. A detailed exposition of Diebold and Yilmaz approach is provided in Appendix B for reference.
The results for connectedness of equity markets of the region are based on vector autoregression lag specification of order 4 and generalized variance decomposition of 10 day ahead forecast returns (Following Diebold & Yilmaz, Citation2009; total spillover plot is not sensitive to the choice of the order of VAR or to the choice of the forecast horizon). Directional SpilloverFootnote6 is a measure of the connectedness that captures the shocks received by vector i from all other vectors j and vice versa. Finally, we have Net Spillover (see note 6) which one can obtain by the difference between the returns shocks transmitted to and returns shocks transmitted from all other markets. It is also of interest to examine net pairwise return spillovers between markets i and j, which is simply the difference between gross return shocks transmitted from market i to j and gross return shocks transmitted from j to i.
3.2. Quantity-based measures
Quantity-based measures supplement the price-based measures for assessing and monitoring the changes and trends in the equity market integration. Thus, we examine the cross-border equity holdings of the sample countries to assess whether there is a shift to investment within/from SAARC region or the broad ASEAN + 6 region.
4. Empirical results
4.1. Preliminary analysis
The summary statistics of sample index return series are presented in Table below. The annualized mean returnsFootnote7 were positive for all sample countries with the highest being for Bangladesh as 42.5%. The annualized volatility (see note 7) in these returns ranged between 17.23% (Nepal) and 50.44% (Maldives). Majority of the sample markets were negatively skewed and all of them show an excess kurtosis thereby implying fat tails and sharp peaks. The Jarque–Bera statistic for all markets is statistically significant, thereby indicating that the return distributions are non-normal. The results of Ljung–Box Q-statistics in levels and ARCH-LM tests clearly state the presence of serial correlation and heteroscedasticity, respectively, for the sample series.
Table 3. Descriptive Statistics of sample return series
As a pre-cursor to the time-series analysis for modelling the same, we also conducted the unit root tests for stationarity. The Augmented Dickey and Fuller (Citation1979), Kwiatkowski, Phillips, Schmidt, & Shin, (Citation1992) as well as the Phillips and Perron, (Citation1988) test resultsFootnote8 suggest that all sample series are integrated to order 1 i.e. I(1).
4.2. Price-based measures
4.2.1. Copula-based results
Table presents the results of the marginal specification of the return series for each sample country. The coefficient of the lagged US returns acting as a proxy for global factor to capture mean spillover effect was found to be significant for only India and Maldives. This indicates the interlinkages of the equity market movements of USA index returns with these countries. The EGARCH (1, 1) estimation shows that long run volatility persistence (as measured by β coefficient) was statistically significant for all the sample countries. The asymmetric effect of news on volatility factor (measured by ϒ coefficient) is negative for all countries and even statistically significant (for India, Maldives and Pakistan) thereby justifying the use of EGARCH (1, 1) model. The Auto Correlation Function (ACFs) of the standardized residuals and squared standardized residuals, obtained from the mean equation (not presented due to brevity of space), indicate that the standardized residuals are approximately i.i.d (identically and independently distributed).
Table 4. Results of the marginal specification of return series of sample countries
We turn to estimate the copula functions wherein, firstly, we consider the standardized residuals from the mean equation and transform it into vector of uniform variates using cumulative distributive function.
Results from the time invariant copula models (refer Table ) suggest that the dependence parameter between sample equity market returns are around 0 implying almost no association. The biggest economy of the region i.e. India has the highest dependence parameter of 0.032 with Nepal, while the lowest parameter of −0.023 with Sri Lanka. The highest dependence parameter in the SAARC region is between the equity markets of Pakistan and Sri Lanka (0.082), while the lowest is for Nepal and Maldives (−0.0413).
Table 5. Estimation results of time invariant copula models of dependence amongst sample equity markets
The low values of the dependence parameters in the SAARC region can be attributed to its poor regional trade intensity (total trade within SAARC members/total trade with the world). It can be clearly seen that the regional trade intensity (refer Figure ) is the lowest for SAARC member group (hovering around 0.05%) compared to other regional economic blocs and has rather remained stagnant over the period of study.
Figure 1. Regional trade intensity of major regional blocs of the world.
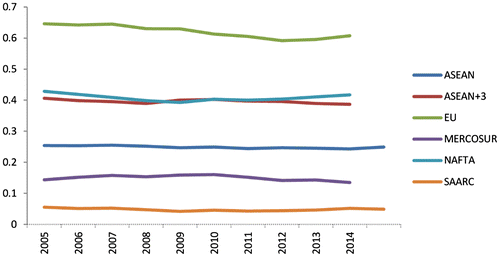
For the time invariant copula models, T copula seems to be the best fit (lowest negative log likelihood values, refer Table ) amongst the copula family of models for majority of the sample country pairs. Figure depicts the results relating to the time varying copula models which show dynamic dependence measure between each sample country’s benchmark equity index returns with each other. We have charted the best fit time varying copula model selected from the goodness of fit test (Refer Table ) against its corresponding time invariant copula parameter (shown in each graph as red line) to study its inter-temporal dependence.
Figure 2. Time Varying Copula Models Dependences between sample country’s benchmark equity returns.
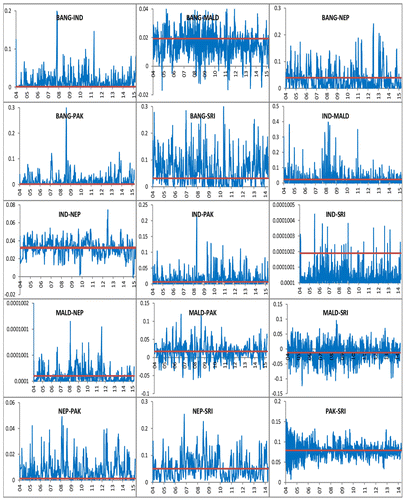
Table 6. Goodness of Fit test for time varying copula models of dependence amongst sample equity markets
The goodness of fit test selects clayton copula model amongst the different family of copula models used in this study for majority of the sample country pairs (except Bangladesh and Maldives; India and Nepal; Maldives and Pakistan; Maldives and Sri Lanka; and Pakistan and Sri Lanka). Clayton copula models the lower tails dependence and the parameter ranges from 0 to . Within the SAARC region, the highest time varying dependence amongst the country pairs using the clayton model (as selected by goodness of fit test) is shown by equity market indices of Sri Lanka and Nepal, while the lowest time varying dependence is shown between India and Sri Lanka. Amongst the Elliptical family of selected copula models based on goodness of the fit test, the time varying dependences between Pakistan and Sri Lanka seem to be the highest. Stronger co-movements between the two sample equity markets reflect greater levels of integration and hence, an increase in dependence parameter (as a measure of co-movement) amongst financial markets may signal increased convergence (Kuper & Lestano, Citation2007). In this regards, the levels of dependences are around 0 for all the pair of sample countries implying little or no integration between them. The goodness of fit tests in case of equity market linkages for the SAARC region predominantly selects Clayton copula model unlike the Elliptical copula model selected in case of equity market integration for the ASEAN + 6 equity markets (refer Sehgal, Pandey, et al., Citation2016). This indicates that equity markets in this region are not fairly mature and well-developed in comparison to their other Asian counterparts, with the exception of India. Also the selection of Clayton copula models show that equity markets of the region have a greater association of their left tails which implies potential asymmetry and some element of contagion.
Malevergne and Sornette (Citation2003) suggest that the dependence structure of a copula is not the same for raw returns and filtered returns (residuals). Hence, the robustness of copula results would be confirmed when the choice of copula models is the same when one uses the raw returns and the filtered returns. The goodness of fit test which was run on the raw returns (results not presented here due to brevity of space but can be available on request by the authors) also selected the same copula model as was selected when the filtered returns (residuals) were used. Even though our results are not insensitive to raw returns, we think that the analysis with filtered returns provides more accurate marginal distributions (as raw returns data were exposed to autocorrelation and ARCH effects and stochastic property of filtered returns are better as they are i.i.d). The copula parameter estimation and testing methodology would not be suffering from statistical biases when the i.i.d variable is used than otherwise (Roch & Alegre, Citation2006).
4.2.2. Diebold and Yilmaz-based results
Table presents the time aggregated equity returns spillover matrix for six South Asian countries. It can be clearly seen from Table that for each of the South Asian country, much of the contribution to its forecast error variance is coming from shocks given to its own equity returns and the cross-market spillovers (off diagonal elements of the table) are very small. The Indian equity market dominates the equity markets of South Asia region as can be seen from the result of net directional returns spillover, where the largest spillover transfer are from India to others (6.6–5.70 = 0.9%) whereas the other countries in the study (with the exception of Nepal and Sri Lanka) are net receiver of returns spillovers. The total returns spillover which appears in the lower right corner of Table , indicates that on the average 3.1% of the forecast error variance in all the six markets comes from spillovers which confirm that connectedness of the regional equity markets is low.
Table 7. Return spillovers across equity markets of South Asian Countries
Though the full sample spillover table and spillover index constructed earlier, provide useful summary of average return spillover behaviour, it likely misses the dynamic evolution of this behaviour as the equity markets are subject to financial linkages. To address this issue, we now estimate returns spillovers using 200-day rolling samples, and we assess the extent and the nature of spillover variation over time by the total spillover plot of Figure . The total return spillover graph for most of time fluctuates between 10 and 22%, which shows low linkages between equity markets of sample countries over time.
Both the chartsFootnote9 of time varying net return spillover for the sample countries from all other countries and dynamic net pairwise return spillover between sample countries indicate that the equity markets in the South Asian region are less interconnected with each other indicating low equity market integration for the region.
4.3. Quantity-based measures
Figure below shows the annual values of portfolio equity inflows from the SAARC and ASEAN + 6 member groups into the sample countries. Not surprisingly, the quantum of portfolio equity inflows seems to be attracted to the largest economy of the SAARC region i.e. India for the period under study. This trend has all but increased over time and has only strengthened post the global economic crises of 2008, which implies that there is mutual confidence in the macroeconomic strength of India. However, these equity investments into India are emanating from the larger ASEAN + 6 region as the same from the SAARC group countries is zero across the period. As for the remaining SAARC countries individually, portfolio equity investments from SAARC region remains muted and the bulk of the flows is dominated by the larger ASEAN + 6 member countries. India seems to predominantly be the only source of equity portfolio inflows into Nepal and Maldives even when we consider the bigger ASEAN + 6 region. As for the remaining three sample countries in the South Asia region, Sri Lanka seems to be attracting the equity portfolio flows from the SAARC region predominantly from India both before and after the global crises periods. Sri Lanka also received heavy portfolio investments from Pakistan in the pre crises periods which virtually dried out during recent years. Pakistan also received portfolio equity inflows from Bangladesh only in 2014.
Figure 4. Portfolio Equity Inflows into SAARC Countries from SAARC and ASEAN + 6 Groups.
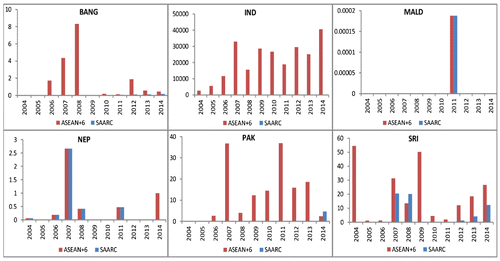
Figure below shows the annual values of portfolio equity outflows from sample countries to the SAARC and ASEAN + 6 member groups. Regarding the portfolio equity outflows, there are no outflows to the SAARC or the larger ASEAN + 6 region from Sri Lanka, Maldives and Nepal over the period under study which can be attributed to the internal instability in these countries which lead to their tepid economic growth and development. Pakistan seems to be heavily invested in SAARC region (though only in Sri Lanka) before the global crises of 2008 but has shifted its investments to larger ASEAN + 6 region for few years after the crises. Bangladesh seems to be the latest member to invest in the larger ASEAN + 6 region in all these years by investing in Sri Lanka and Pakistan in 2014. Though the portfolio equity outflows from India are considerably higher to larger ASEAN + 6 group region but India also seems to be invested in SAARC in predominantly all members’ countries except Pakistan across the study period which can be attributed to its political standoffs with Pakistan.
Figure 5. Portfolio Equity Outflows from sample SAARC countries to SAARC and ASEAN + 6 groups.
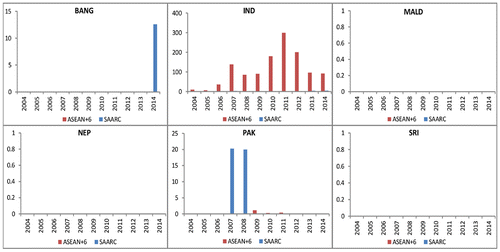
The results from the price-based analysis on the basis of time invariant copula models show that albeit after decades of its formation, the SAARC regional bloc has not come out of its political standoffs and embraced regionalism to bring dynamism into this region. The dependence parameter between the SAARC member nations are virtually 0 confirming little equity market integration in the region. Even after operationalization of SAFTA agreement in 2006, regional trade intensity is the lowest for SAARC group compared to major regional economic blocs of the world for the period under study. The charts from the time varying copula models support the preliminary findings of the time invariant models as the dependence parameter seem to be peaking towards the time of the great economic crises in 2008 but the co-movements are very low between all the country pairs. Results based on Diebold and Yilmaz methodology also indicate that connectedness of the equity markets for the South Asian region is low. The results based on quantitative indicators reconfirm that the quantum of equity portfolio inflows into SAARC members individually are from larger ASEAN + 6 region. While India receives no equity inflows from SAARC region, Srilanka seems to be attracting the maximum inflows from the SAARC region (predominantly India). With the exception of portfolio equity outflow to SAARC region from Bangladesh in 2014, there seems to be no portfolio flows from Bangladesh, Maldives, Nepal and Sri Lanka into even larger ASEAN + 6 region for the period under study which can be attributed to the state of their economy due to political conflicts. Therefore, SAARC member countries require collaboration in efforts and to build mutual trust and commitment to make their alliance viable enough to steadily progress towards regional integration to catch up with major regional blocs like European Union, NAFTA and neighbouring ASEAN.
5. Fundamental determinants of equity market integration
5.1. Selection of explanatory variables
After estimating the time varying dependence measure for the sample SAARC countries, in this section we investigate the fundamental determinants of stock market integration. We consider the exhaustive list of potential determinants of financial integration that have been covered by prior studies which were summarized in Table . These fundamental determinants are primarily divided into Macroeconomic, Trade and Market-based categories. These determinants (variables) potentially play an important role in explaining the intertemporal behaviour of equity market integration measured by the time varying copula parameter provided by the best fit copula model selected on goodness of fit test.
5.2. Measurement and rationale for selecting explanatory variables
These variables have been compiled after an exhaustive survey of literature and are operationalized as can be seen from Table . Academic literature (Guesmi, Nguyen, & Teulon, Citation2013; Ng, Solnik, Wu, & Zhang, Citation2013; Vo & Daly, Citation2007) suggests that growth and development (as measured by GDP growth rate, GDP per capita and IIP) is intricately linked to international financial integration as economic growth acts as a stimulant for private capital flows into the economies thereby fostering higher integration. We expect on a priori ground that a favourable External Position as measured by Current account balance (Guesmi et al., Citation2013) and Fiscal Position (proxied by budget balance, govt. debt and govt. tax revenues) will contribute to equity market integration (Arfaoui & Abaoub, Citation2010; Vo & Daly, Citation2007). The Monetary Policy variables (inflation rate and short term interest rates) act as proxies for confidence shocks on consumption and investment opportunities and thus, contribute to valuations of the firms in the equity market (Guesmi et al., Citation2013). A stable and favourable monetary policy would promote equity market integration. International financial integration is very closely associated with development of a sound institutional, legal and investment environment reflected by the Governance factor (Vo & Daly, Citation2007). Academic literature suggests that higher investment flows (as measured by FII and FDI) can significantly reduce the cost of capital in capital-poor economies leading to higher investment which can be a major engine for economic growth thereby contributing positively to financial integration The Risk factor (measured by volatility of the forex rate and liquidity risk) is specified in the international asset-pricing models where it has been recognized as a factor for financial disintegration (Huoy & Goh, Citation2007). Integration in equity markets is likely to be driven by increase in Trade Linkages and Trade Openness as measured by trade tariffs, total trade and trade intensity (Huoy & Goh, Citation2007; Ng et al., Citation2013).
A developed and well-performing financial market (Market Sophistication which includes market size and stock market efficiency and Market Performance constituted by volatility of market return, domestic growth opportunities and local stock market conditions) helps to attract foreign investors to diversify their portfolio and increase portfolio investment inflows, thus, contributing to financial integration (Vo & Daly, Citation2007).
5.3. Analysis
Since our equity market dependences are close to 0 between SAARC member countries, hence we are unable to perform the panel data analysis (as absence of financial integration implies no dependent variable to be explained by explanatory variables) to confirm the role of significant variables driving integration in this region. We would rather analyse the trends for these variables for the SAARC countries individually and compare the same with the member countries comprising the larger ASEAN group i.e. ASEAN + 6. ASEAN group is selected as it is the neighbouring regional economic bloc in Asia with a much longer history (created in 1967) and having a wider membership base (10 members ASEAN which extends to ASEAN + 3/ASEAN + 6). Sehgal et al. (Citation2016) observe dependences of the ASEN + 6 bloc members with the Asian benchmark thereby confirming varying degree of equity market integration of the sample countries. Thus, analysing and comparing the trends in these fundamental determinants for the two regional blocs will help to show how important these variables are for SAARC, which is a test group and ASEAN + 6 which is used as control group.
For majority of these determinants, the values are not much different for the SAARC and ASEAN + 6 member nations. We present here the trend of only those variables for which there was found an observable difference between the two groups. For the Macroeconomic category, important difference is observed for the trends in the governance indicator (refer Figure ) for SAARC member countries viz-a-viz ASEAN + 6 member countries.
Figure 6. Trends in Governance Indicators of SAARC and ASEAN + 6 Countries.
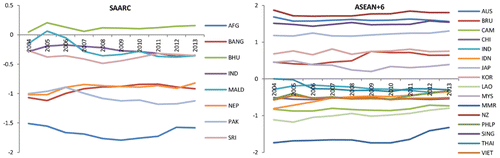
The governance indicators are all in negative for the SAARC member countries (except Bhutan) but the same for ASEAN + 6 members (Singapore, Malaysia, Japan, South Korea, Australia, New Zealand) which have high integration with the Asian benchmark (Sehgal et al., Citation2016) are positive.
For the Trade category, the trend analysis for the total trade as a % of GDP variable (refer Figure ) shows that except for Maldives and Bhutan, all South Asian countries have the value as less than 100% in comparison to many ASEAN + 6 countries which have a large total trade position (Singapore, Malaysia, Thailand, Vietnam). For the trade tariff variable (refer Figure ), except for the concerted trade policy commitment and contingency measures undertaken by World Trade organization (WTO) in 2009 to arrest the contraction in total trade due to great economic recession of 2008, the levels of trade tariffs (as %duty) is around 10–20% for SAARC member nations. In contrast, low tariff rates (less than 10%) are prevalent amongst majority of the ASEAN + 6 countries which help in facilitating intra-regional trade thereby promoting integration.
Figure 7. Trends in Total Trade (as percentage of GDP) of SAARC and ASEAN + 6 Countries.
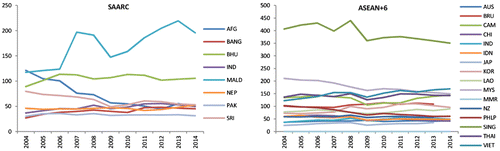
Figure 8 Trends in Trade Tariff (as percentage of duty) of SAARC and ASEAN + 6 Countries.
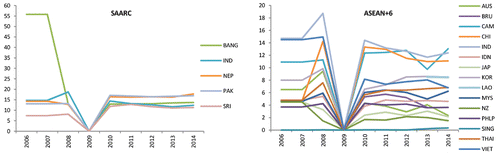
For the Market category, the trend analysis for market capitalization as a % of GDP (refer Figure ) shows that except for India, sample SAARC countries have values less than 50% while majority of ASEAN + 6 member countries have corresponding values close to 100%.
Figure 9. Trends in Market Capitalization (as percentage of GDP) of SAARC and ASEAN + 6 Countries.
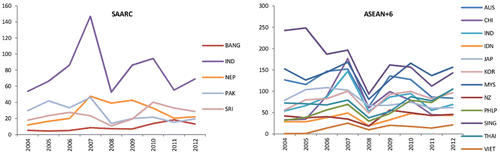
This shows that the SAARC member states have to work on their governance parameters, improve on their trade linkages and trade tariffs and develop their equity market infrastructure to achieve higher levels of financial integration. The findings are in line with Bekaert, Harvey, and Ng (Citation2005) and Goetzmann, Li, and Rouwenhorst (Citation2005) who claimed that capital market integration and increased trade are embedded with predictions about the association between markets.
India enjoys a unique place in the South Asian region as its GDP is eight times (Source: IMF, 2014) the size of Pakistan (second biggest economy in South Asia) and the only country in the region which shares common borders with all other nations except Afghanistan. Hence, the regional cooperation in South Asia will not succeed without the active participation and sincere commitment of India. Figure shows the trade intensity of India with respect to other country (total trade of countryi with India/total trade of India) for the SAARC and ASEAN + 6 countries.
Figure 10. Trade Intensity of India (ratio of total trade with India to total trade of India).
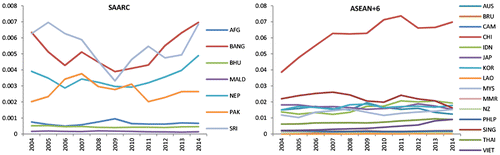
It clearly shows the fault lines as India trades higher with the ASEAN + 6 members than the SAARC members (roughly 10 times higher, refer Figure ). This is counter-intuitive as India provides overland transit to Bangladesh, Nepal and Bhutan for their bilateral trade and maritime transit to Nepal and Bhutan for its international trade.
6. Summary and policy suggestions
In this paper, we examine the extent of financial integration amongst the equity market segments of the SAARC member countries. Daily stock market index closing price data were used for the six of the eight SAARC member countries for the period of 6 January 2004–31 March 2015 as data for Afghanistan and Bhutan were not available. Our empirical analysis is based on Copula GARCH approach to capture the dynamic process of financial integration by investigating the return co-movement of the sample country’s equity index with each other. The results obtained from both time invariant and time varying copula models show that the dependences between the equity markets of SAARC member countries are close to 0 indicating very low level of financial integration in the region. This can be attributed to the low regional trade intensity in the SAARC region which is in fact the lowest amongst all the major regional economic blocs of the world. Results obtained from Diebold and Yilmaz methodology confirmed low connectedness between equity markets of member countries and thereby supported the copula-based findings.
The results based on quantity-based indicators reconfirm that the quantum of equity portfolio inflows for individual SAARC members are much greater from ASEAN + 6 group than from the SAARC group. Though India seems to receive equity portfolio flows only from the larger ASEAN + 6 group and none whatsoever from the SAARC region but it is itself invested in the SAARC group members. This can be attributed to the fact that contrary to groupings in other regional blocs, India is the largest country in the region, not only in terms of geographical territory and population, but also in terms of size of the economy (third largest economy in terms of PPP in the world, Source: World Bank). With the exception of Pakistan prior to the global financial crises of 2008 and Bangladesh in 2014, none of the other South Asian countries (Bangladesh, Maldives, Nepal and Sri Lanka) act as a source of equity portfolio investment into even the larger ASEAN + 6 member group. This may be attributed to their small size of the economy, hostile internal political situations and increasing mistrust with each other. The trends of the fundamental determinants of equity market integration of the SAARC member countries were compared with its neighbouring regional economic bloc in Asia which has a much longer history and a wider membership base i.e. ASEAN + 6. From a comparative analysis, one could see that the equity market integration in the SAARC members lagged their ASEAN + 6 counterparts owing to poor governance quality, lower trade linkages, higher trade tariffs and less sophisticated equity markets with relatively weaker institutional and regulatory architecture.
Regional integration will be bolstered as the policy-makers of the region prepare to improve their macroeconomic fundamentals, strengthen the regional institutional frameworks, enhance trade policy cooperation, undertake capital market development and effectively manage cross-border portfolio investments. The SAARC member countries may be at first expected to foster a stable political environment to ensure clean and transparent governance, while taking due consideration for each other’s security concerns. The SAARC members must coordinate to allay the mistrust between them by encouraging more people to people contact to help them develop social cohesion and imbibe shared cultural ethos which is unique to this region.
As demonstrated by Capannelli, Jong, and Peter (Citation2010) in order to increase financial integration within the region, it will be necessary to develop and strengthen the regional institutions. In this regards, creation of a South Asian Economic Union (SAEU) in the future would prove to be a milestone for regional cooperation efforts. Trimming of the SAARC sensitive list outside of SAFTA and removing of non-tariff barriers will boost intra SAARC trade which will act as a key for an integrated region. High transaction costs vitiate South Asian intra-regional trade and good payment systems (cash in advance i.e. WIRE transfer; credit cards, Documentary Collection and Letters of Credit) are one way of facilitating such trade. India, being the largest country of SAARC, should take a lead by reducing the high customs duty, facilitating port clearance, improving communication and transportation and removing regulatory constraints at the borders thereby creating an enabling connectivity infrastructure and trade facilitation regime. Further SAARC members could work towards strengthening the South Asia Regional Standards Organization (SARSO) to promote and harmonize standards on common products of regional interest to enhance flow of goods and services.
With regards to the equity markets development, SAARC members can come up with an Integrated Equity Markets Platform where companies of the member countries could list their shares. India, which has the most sophisticated equity market infrastructure with effective regulation and compliance in the SAARC region, can provide its financial support and technical expertise for building and operating equity market infrastructure in other SAARC member countries. The members can coordinate to subsequently formulate a SAARC Disclosure Standards Scheme and a SAARC Corporate Governance Scorecard to further enhance cooperation in the integration of equity market segments. The former would help in the cross-border offerings of securities while the later would ensure better transparency. A federation of market intermediaries (brokers of member states) association, South Asian Securities Association could be set up to help formalize and institutionalize a process of interaction amongst members to know each other and share best practices. Though sincere efforts should be made for regional cooperation amongst member nations and specifically by India, until SAARC region comes up as a vibrant regional economic bloc, Indian policy-makers can look towards the strengthening of the multilateral forum of ASEAN + 6 to act as the engine of growth and development of Asia.
The study has important implications for the policy-makers, portfolio managers and academia. For the policy-makers, the study is of particular relevance in the light of growing number of regional co-operation initiatives worldwide for achieving political influence, enhanced linkages amongst economies as well as developing a coordinated response to global risks including financial contagion. As the global economic gravity shifts to the east and the twenty-first century is believed to be the “Asian century”, the study would provide policy directions to Indian policy-makers who want to project India as a strategic player in the region having geopolitical ambitions. The global portfolio managers can treat SAARC region as a heterogeneous bloc providing diversification opportunities within the region (risk adjusted return is highest for Bangladesh and Nepal viz-a-viz India inspite of them being uncorrelated). From the academic point of view, firstly the literature is very limited on the regional financial integration in the South Asian region and hence this study brings out a comprehensive analysis of the state of equity market integration in this region. Secondly, the study highlights the dynamic process of financial integration in the region and further identifies its key determinants wherein concerted efforts of policy realignment from SAARC members are quintessential to enhance financial integration. The paper contributes to the literature by measuring the progress of financial integration in the equity market segments for the SAARC members’ countries and outlines the thrust areas which will improve the level of regional integration.
Funding
This manuscript is part of a sponsored research project entitled “Financial Integration in the SAARC Region: Empirical Analysis and Policy Issues”. The authors would like to acknowledge the financial support provided by Indian Council of Social Sciences Research (ICSSR).
Acknowledgments
The authors sincerely thank the anonymous reviewer and the journal editor for their valuable inputs and suggestions which have helped in refining the manuscript.
This manuscript is part of a sponsored research project entitled “Financial Integration in the SAARC Region: Empirical Analysis and Policy Issues”. The authors would like to acknowledge the financial support provided by Indian Council of Social Sciences Research (ICSSR). We would also like to thank Ms. Sakshi Saini, Research Assistant working under this project, for her research support in preparing this manuscript.
Additional information
Notes on contributors
Sanjay Sehgal
Sanjay Sehgal is currently a professor of Finance and Head, Department of Financial Studies, University of Delhi. He got his PhD from Delhi School of Economics, University of Delhi and has done his post doctorate from Department of Finance, London School of Economics, UK. He has a teaching experience of about 27 years in the field of investment management, financial derivatives, corporate finance and financial econometrics.
Piyush Pandey
Piyush Pandey is working as assistant professor in Finance and Accounting area at FORE School of Management, New Delhi. He was a PhD student at the Department of Financial Studies, University of Delhi under the supervision of Sanjay Sehgal and has submitted his doctoral thesis titled “Financial Market Integration in the East Asia Economic Community Region- An Empirical Study”.
Florent Deisting
Florent Diesting is currently a professor of Economics at ESC Pau, France. His research areas include Financial Economics, Risk Management, Insurance and Econometrics.
Notes
1. ASEAN was created with the signing of Bangkok Declaration in 1967, by Indonesia, Malaysia, Philippines, Singapore and Thailand. Subsequently the ASEAN bloc grew with the addition of Brunei, Vietnam, Laos, Myanmar and Cambodia (in the order of their entry). The 10 ASEAN members plus the three members i.e. Japan, China and South Korea constitutes the ASEAN + 3 bloc. The second East Asian Summit (EAS) was held Cebu in January 2007, wherein 10 ASEAN members and six countries including China, Japan, South Korea, India, Australia and New Zealand participated. Japan regards this ASEAN + 6 (EAS group) as an appropriate group for East Asia’s trade and investment cooperation.
2. In this paper, South Asia refers to the eight member countries of the South Asia Association for Regional Cooperation (SAARC)—Bangladesh, Bhutan, India, Nepal, Pakistan, Sri Lanka and the Maldives. Afghanistan later became the eighth member in 2007.
3. SAARCFINANCE is a regional network of SAARC Central Bank Governors and Finance Secretaries formed in 1998, to foster cooperation in macroeconomic policies; synchronize banking legislations and practices; collaborate amongst central banks and finance ministries; share ideas, information and experiences; undertake monetary and exchange cooperation; investigate global financial developments; and promote research on economic and financial issues amongst member countries. South Asian Federation of Exchanges (SAFE) was established in 2000, for development of securities markets, encouraging cross-border listings and achieving greater harmonization while South Asian Securities Regulators’ Forum (SASRF) was formed in 2005, to further enhance the cooperation and exchange of information amongst the regulators of these countries.
4. For a survey of the literatures and various indicators, see (Baele, Ferrando, Hordahl, Krylova, & Monnet, Citation2004; Cavoli et al., Citation2004; Poonpatpibul, Tanboon, & Leelapornchai, Citation2006).
5. For survey of literature using these indicators, see (Gupta, Sehgal, & Deisting, Citation2015; Yu, Fung, & Tam, Citation2010).
6. The equations for Total Connectedness, Directional Spillover and Net Spillover are the same as used by Filho & Hong (Citation2016).
7. Annualization has been done assuming 250 trading days in an year.
8. Unit root test results are not reported due to brevity of space. These are available on request from the authors.
9. The two charts are not reported due to brevity of space. These are available on request from the authors.
References
- Abad, P., & Chuli´a, H. (2014). European government bond market integration in turbulent times ( IREA Working Paper 2014/24). University of Barcelona.
- Adam, K., Jappelli, T., Menichini, A., Padula, M., & Pagano, M. (2002, January 28). Analyse, compare, and apply alternative indicators and monitoring methodologies to measure the evolution of capital market integration in the European Union. Centre for Studies in Economics and Finance, University of Salerno.
- Ali, G., Hussain, H., & Islam, T. (2014). Interdependence of South Asian Equity Markets. Research Journal of Applied Sciences, Engineering and Technology, 7(13), 2762–2771.10.19026/rjaset.7.598
- Aloui, R., Hammoudeh, S., & Nguyen, D. K. (2013). A time-varying copula to oil and stock market dependence: The case of transition economies. Energy Economics, 39, 208–221.10.1016/j.eneco.2013.04.012
- Arfaoui, M., & Abaoub, E. (2010). On the determinants of international financial integration in the global business area. Journal of Applied Economic Sciences, 5(3), 153–172.
- Baele, L., Ferrando, A., Hordahl, P., Krylova, E., & Monnet, C. (2004). Measuring financial integration in the Euro area ( European Central Bank (Frankfurt, Germany) Occasional Paper No. 14).
- Bartram, S., Taylor, S. J., & Wang, Y. (2007). The Euro and European financial market dependence. Journal of Banking and Finance, 51(5), 1461–1481.10.1016/j.jbankfin.2006.07.014
- Basher, S. A., Salem, N., & Hui, Z. (2014). Dependence patterns across Gulf Arab stock markets: A copula approach. Journal of Multinational Financial Management, 25–26, 30–50.10.1016/j.mulfin.2014.06.008
- Bekaert, G., Harvey, C. R., & Ng, A. (2005). Market integration and contagion. The Journal of Business, 78, 39–69.10.1086/jb.2005.78.issue-1
- Bley, J. (2009). European stock market integration: Fact or fiction? Journal of International Financial Markets, Institutions and Money, 19, 759–776.10.1016/j.intfin.2009.02.002
- Bollerslev, T. (1987). A conditionally heteroskedastic time series model for speculative prices and rates of return. The Review of Economics and Statistics, 69, 542–547.10.2307/1925546
- Boubakri, S., & Guillaumin, C. (2015). Regional integration of the East Asian stock markets: An empirical assessment. Journal of International Money and Finance, 57, 136–160.10.1016/j.jimonfin.2015.07.011
- Brunnermeier, M. K., Nagel, S., & Pedersen, L. H. (2008). Carry trades and currency crashes. NBER Macroeconomics Annual, 23, 313–347.
- Büttner, D., & Hayo, B. (2011). Determinants of European stock market integration. Economic Systems, 35(4), 574–585.10.1016/j.ecosys.2010.10.004
- Capannelli, G., Jong, W. L., & Peter, A. P. (2010). Economic interdependence in Asia: Developing indicators for regional integration and cooperation. The Singapore Economic Review, 55(01), 125–161.10.1142/S021759081000364X
- Cappiello, L., Engle, R. H., & Sheppard, K. (2006). Asymmetric dynamics in the correlations of global equity and bond returns. Journal of Financial Econometrics, 4(4), 537–572.10.1093/jjfinec/nbl005
- Cavoli, T., Rajan, R. S., & Siregar, R. (2004). A survey of financial integration in East Asia: How far? How much further to go (Discussion paper, p. 0401). University of Adelaide.
- Chollete, L., Heinen, A., & Valdesogo, A. (2009). Modeling international financial returns with a multivariate regime-switching copula. Journal of Financial Econometrics, 7(4), 437–480.10.1093/jjfinec/nbp014
- Clayton, D. G. (1978). A model for association in bivariate life tables and its application in epidemiological studies of familial tendency in chronic disease incidence. Biometrika, 65, 141–151.10.1093/biomet/65.1.141
- Da Silva Filho, O. C., Ziegelmann, F. A., & Dueker, M. J. (2012). Modeling dependence dynamics through copulas with regime switching. Insurance: Mathematics and Economics, 50, 346–356.
- Dickey, D. A., & Fuller, W. A. (1979). Distribution of the estimators for autoregressive time series with a unit root. Journal of the American Statistical Association, 74, 427–431.
- Diebold, F. X., & Yilmaz, K. (2009, January). Measuring financial asset return and volatility spillovers with application to Global Equity Markets. The Economic Journal, 119(534), 158–171.10.1111/ecoj.2008.119.issue-534
- Diebold, F. X., & Yilmaz, K. (2012). Better to give than to receive: Predictive directional measurement of volatility spillovers. International Journal of Forecasting, 28(1), 57–66.10.1016/j.ijforecast.2011.02.006
- Dungey, M., Fry, R., & Martin, V. (2003). Equity transmission mechanisms from Asia to Australia: Interdependence or contagion? Australian Journal of Management, 28(2), 157–182.10.1177/031289620302800203
- ECB. (2004). Measuring financial integration in Euro-Area. Frankefurt: European Central Bank (ECB).
- Engle, R. F. (1982). Autoregressive conditional heteroskedasticity with estimates of the variance of U.K. inflation. Econometrica, 50, 987–1008.
- Fernandez, V. (2008). Copula-based measures of dependence structure in assets returns. Physica A: Statistical Mechanics and its Applications, 387(14), 3615–3628.10.1016/j.physa.2008.02.055
- Filho, R. G., & Hong, G. H. (2016). Dynamic connectedness of Asian equity markets ( IMF Working Paper, WP/16/57).
- Fratzscher, M. (2001). Financial market integration in Europe: On the effects of EMU on stock markets ( Working Paper Series 0048). European Central Bank.
- Fratzscher, M. (2002). Financial market integration in Europe: On the effects of EMU on stock markets. International Journal of Finance & Economics, 7(3), 165–193.
- Genest, C., Rémillard, B., & Beaudoin, D. (2009). Goodness-of-fit tests for copulas: A review and a power study. Insurance: Mathematics and Economics, 44, 199–213.
- Gill, I., Sugawara, N., & Zalduendo, J. (2014). The center still holds: Financial integration in the Euro Area. Comparative Economic Studies, 56, 351–375.
- Gilmore, C. G., & McManus, G. M. (2004). The impact of NAFTA on the integration of the Canadian, Mexican and U.S. equity markets. In A. M. Rugman (ed.), North American economic and financial integration (Research in Global Strategic Management) (Vol. 10, pp. 137–151). Bingley: Emerald Group Publishing Limited.
- Goetzmann, W. N., Li, L., & Rouwenhorst, K. G. (2005). Long-term global market correlations. Journal of Business, 78, 1–37.
- Guesmi, K., Nguyen, D.-K., & Teulon, F. (2013). Further evidence on the determinants of regional stock markets integration in Latin America. European Journal of Comparative Economics, 34(1), 397–413.
- Guillaumin, C. (2009). Financial integration in East Asia: Evidence from panel unit root and panel cointegration tests. Journal of Asian Economics, 20, 314–326.10.1016/j.asieco.2009.02.002
- Gumbel, E. J. (1960). Bivariate exponential distributions. Journal of the American Statistical Association, 55, 698–707.10.1080/01621459.1960.10483368
- Gupta, P., Sehgal, S., & Deisting, F. (2015). Time-varying bond market integration integration in EMU. Journal of Economic Integration, 30(4), 708–760.10.11130/jei.2015.30.4.708
- Hardouvelis, G., Malliaropulos, D., & Priestley, R. (2006). EMU and European stock market integrations. The Journal of Business, 79, 365–392.10.1086/jb.2006.79.issue-1
- Huoy, C. W., & Goh, K. L. (2007). The Determinants of Stock Market Integration: A Panel Data Investigation. In 15th Annual Conference on Pacific Basin Finance, Economics, Accounting and Management, Ho Chi, Minh City, Vietnam, 20 & 21 July.
- Huyghebaert, N., & Wang, L. (2010). The co-movement of stock markets in East Asia: Did the 1997–1998 Asian financial crisis really strengthen stock markets integration? China Economic Review, 21, 98–112.10.1016/j.chieco.2009.11.001
- Kim, S. J., Moshirian, F., & Wu, E. (2005, October). Dynamic stock market integration driven by the European Monetary Union: An empirical analysis. Journal of Banking & Finance, 29(10), 2475–2502.
- Kim, M. S., & Wang, S. (2006). On the application of stochastic volatility models. Computational Statistics & Data Analysis, 51(4), 2210–2217.
- Kojadinovic, I., & Yan, J. (2011). A goodness-of-fit test for multivariate multiparameter copulas based on multiplier central limit theorems. Statistics and Computing, 21(1), 17–30.10.1007/s11222-009-9142-y
- Koop, G., Pesaran, M. H., & Potter, S. M. (1996). Impulse response analysis in nonlinear multivariate models. Journal of Econometrics, 74(1), 119–147.10.1016/0304-4076(95)01753-4
- Kuper, G. H., & Lestano. (2007). Dynamic conditional correlation analysis of financial market interdependence: An application to Thailand and Indonesia. Journal of Asian Economics, 18, 670–684.10.1016/j.asieco.2007.03.007
- Kwiatkowski, D., Phillips, P. C. D., Schmidt, P., & Shin, Y. (1992). Testing the null hypothesis of stationarity against the alternative of a unit root: How sure are we that economic time series have a unit root? Journal of Econometrics, 54, 159–178.10.1016/0304-4076(92)90104-Y
- Lahrech, A., & Sylwester, K. (2011). U.S. and Latin American stock market linkages. Journal of International Money and Finance, 30, 1341–1357.10.1016/j.jimonfin.2011.07.004
- Lahrech, A., & Sylwester, K. (2013, August). The impact of NAFTA on North American stock market linkages. The North American Journal of Economics and Finance, 25, 94–108.
- Latif, S., Rizvi, S. M., Mubin, M., & Iqbal, N. (2014). Financial Market Integration: Empirical Evidence from the Economic Cooperation of India and Pakistan. Journal of Economics and Sustainable Development, 5(3), 2014.
- Longin, F., & Solnik, B. (2001). Extreme correlation of international equity market. The Journal of Finance, 56, 649–676.10.1111/0022-1082.00340
- Malevergne, Y., & Sornette, D. (2003). Testing the Gaussian copula hypothesis for financial assets dependences. Quantitative Finance, 3, 231–250.10.1088/1469-7688/3/4/301
- Mandelbrot, B. B. (1963). The variation of certain speculative prices. Journal of Business, XXXVI, 392–417.
- Morana, C., & Beltratti, A. (2002). The effects of the introduction of the euro on the volatility of European stock markets. Journal of Banking and Finance, 26(10), 2047–2064.10.1016/S0378-4266(01)00182-0
- Mylonidis, N., & Kollias, C. (2010). Dynamic European stock market convergence: Evidence from rolling cointegration analysis in the first euro-decade. Journal of Banking & Finance, 2056–2064.10.1016/j.jbankfin.2010.01.012
- Narayan, P., Smyth, R., & Nandha, M. (2004). Interdependence and dynamic linkages between the emerging stock markets of South Asia. Accounting & Finance, 44(3), 419–439.10.1111/acfi.2004.44.issue-3
- Narayan, S., Sriananthakumar, S., & Islam, S. Z. (2014). Stock market integration of emerging Asian economies: Patterns and causes. Economic Modelling, 39, 19–31.10.1016/j.econmod.2014.02.012
- Nelson, D. B. (1991). Conditional heteroskedasticity in asset returns: A new approach. Econometrica, 59, 347–370.
- Ng, L., Solnik, B., Wu, E., & Zhang, B. (2013). Characterizing global financial and economic integration using cash flow expectations ( Working paper of University of Wisconsin- Milwaukee).
- Ning, C. (2010). Dependence structure between the equity market and the foreign exchange market–A copula approach. Journal of International Money and Finance, 29, 743–759.10.1016/j.jimonfin.2009.12.002
- Patton, A. J. (2006a). Modelling asymmetric exchange rate dependence. International Economic Review, 47(2), 527–556.10.1111/iere.2006.47.issue-2
- Patton, A. J. (2006b). Estimation of multivariate models for time series of possibly different lengths. Journal of Applied Econometrics, 21(2), 147–173.10.1002/(ISSN)1099-1255
- Peng, Y., & Ng, W. (2011). Analysing financial contagion and asymmetric market dependence with volatility indices via copulas. Annals of Finance, 1–26.
- Perera, A., & Wickramanayake, J. (2012). Financial integration in selected South Asian countries. South Asian Journal of Global Business Research, 1(2), 210–237.10.1108/20454451211252741
- Pesaran, B., & Pesaran, M. H. (2007). Modelling volatilities and conditional correlations in futures markets with a multivariate t distribution ( IZA Discussion Papers No. 2906). Institute for the Study of Labor.
- Pesaran, H. H., & Shin, Y. (1998). Generalized impulse response analysis in linear multivariate models. Economics Letters, 58, 17–29.10.1016/S0165-1765(97)00214-0
- Phillips, C. B. P., & Perron, P. (1988). Testing for a unit root in time series regression. Biometrika, 75(2), 335–346.10.1093/biomet/75.2.335
- Poon, S.-H., Rockinger, M., & Tawn, J. (2004). Extreme value dependence in financial markets: Diagnostics, models, and financial implications. Review of Financial Studies, 17(2), 581–610.10.1093/rfs/hhg058
- Poonpatpibul, C., Tanboon, S., & Leelapornchai, P. (2006). The Role of Financial Integration in East Asia in Promoting Regional Growth and Stability. Bank of Thailand: Mimeo.
- Reboredo, J. C. (2011). How do crude oil prices co-move?. Energy Economics, 33, 948–955.10.1016/j.eneco.2011.04.006
- Rizavi, S., & Naqvi, B. (2011). Global and regional financial integration for Asian stock markets. International Journal of Business and Social Sciences, 2(9), 82–93.
- Roch, O., & Alegre, A. (2006). Testing the bivariate distribution of daily equity returns using copulas. An application to the Spanish stock market. Computational Statistics & Data Analysis, 51(2), 1312–1329.10.1016/j.csda.2005.11.007
- Rodriguez, J. C. (2007). Measuring financial contagion: A Copula approach. Journal of Empirical Finance, 14(3), 401–423.10.1016/j.jempfin.2006.07.002
- Samitas, A., & Kenourgios, D. (2007). Macroeconomic factors’ influence on ‘new’ European countries’ stock returns: The case of four transition economies. International Journal of Financial Services Management, 2, 34–49.10.1504/IJFSM.2007.011670
- Sehgal, S., Gupta, P., & Deisting, F. (2016). Assessing time varying stock market integration in Economic and Monetary Union for normal and crisis periods. The European Journal of Finance. doi:10.1080/1351847X.2016.1158727
- Sehgal, S., Pandey, P., & Deisting, F. (2016). Stock market integration dynamics and its determinants in the East Asian Economic Community Region ( A Working Paper). Department of Financial Studies, University of Delhi. Retrieved SSRN from http://ssrn.com/abstract=2768106
- Singhania, M., & Prakash, S. (2014). Volatility and cross correlations of stock markets in SAARC nations. South Asian Journal of Global Business Research, 3(2), 154–169.10.1108/SAJGBR-04-2012-0056
- Sklar, A. (1959). Fonctions de répartition à n dimensions etleursmarges. Publications de l’Institut de Statistique de Paris, 8, 229–231.
- Vo, X. V., & Daly, K. J. (2007). Determinants of International financial integration (R. P. C. Leal, Ed.). Global Finance Conference. Coppead Graduate School of Business, Federal University of Rio de Janerio.
- Yang, L., Cai, X. J., Li, M., & Hamori, S. (2015). Modeling dependence structures among international stock markets: Evidence from hierarchical Archimedean copulas. Economic Modelling, 51, 308–314.10.1016/j.econmod.2015.08.017
- Yang, L., & Hamori, S. (2013). Dependence structure among international stock markets: A GARCH-copula analysis. Applied Financial Economics, 23, 1805–1817.10.1080/09603107.2013.854296
- Yu, I., Fung, L., & Tam, C. (2010). Assessing financial market integration in Asia—Equity markets. Journal of Banking and Finance, 34(12), 2874–2885.10.1016/j.jbankfin.2010.02.010
- Yu, J.-S., & Hassan, M. K. (2008). Global and regional integration of the Middle East and North African (MENA) stock markets. The Quarterly Review of Economics and Finance, 48, 482–504.10.1016/j.qref.2006.06.003
Appendix A
Copula Models for Dependence
Time Invariant Copula Models
(a) | Gaussian Copula: Following (Patton, Citation2006a), the dependence parameter of Gaussian process is: |
(1)
(1)
where u and v are cumulative distribution functions of standardized residuals, subjected to a uniform distribution between 0 and 1, rho is Pearson’s linear correlation, is the inverse cumulative distribution function of a standard normal distribution.
(b) | T-Copula: The dependence parameter of t copula follows from (Fernandez, Citation2008): |
(2)
(2)
where .
T is the student t distribution with degrees of freedom v and Pearson’s correlation ρ. In comparison to Gaussian copula, t copula captures the tail dependence.
(c) | Gumbel Copula: The dependence parameter (Gumbel, Citation1960) which can capture the upper tail dependence: |
(3)
(3)
where 1upper limit in Gumbel copula is ∞
(d) | Clayton Copula: The dependence parameter (Clayton, Citation1978) captures the lower tail dependence: |
(4)
(4)
where 0 ≤ θ < + ∞;
Time Varying Copula Models
Time varying copulas can be considered as dynamic generalization of a Pearson correlation or Kendall’s tau but it is difficult to find causal variables to explain such characteristics (Patton, Citation2006a, Citation2006b). In practice though, time varying copulas are operationalized to follow autoregressive moving average (ARIMA) (p, q) process.
(a) | Time varying Gaussian Copula (Patton, Citation2006a) uses a coefficient rhot to study the dependence dynamics defined as: |
(5)
(5)
where (x) is a logistic transformation which is defined as
(x) = (1 − e−x)(1 − e−x)−1
(b) | Time varying T copula (Patton, Citation2006b) uses the following to study the dependence process: | ||||
(c) |
where T−1is the inverse function of the student t-distribution with given degrees of freedom (DoF). | ||||
(d) | Time varying Gumbel copula studies the dependence using θt corresponding to τt = 1 − 1/θt defined as: |
(7)
(7)
where ⋀(x) = (1 + e−x)−1
(e) | Time varying Clayton copula uses θt corresponding to τt = θt/(2 + θt) to find the dependence defined as: |
(8)
(8)
Appendix B
Diebold & Yilmaz Methodology
For each asset i, in an N-variable VAR, the measure of connectedness is the sum of the shares of its forecast error variance coming from shocks to asset j represented as a percentage, for all j ≠ i. Here we follow the Diebold and Yilmaz (Citation2012), where the shocks are decomposed using generalized vector autoregressive framework of Koop, Pesaran, and Potter (Citation1996) and Pesaran and Shin (Citation1998) in order to avoid the sensitivity of the order of the variables. Consider a p order, N variable VAR model:
xt = where
is the variance of the error terms which are i.i.d. It can be also written in moving average form as xt =
where Ai =
and j = 1,…, p. The H step ahead forecast error variance decomposition (
is defined as follows:
(9)
(9)
where is the variance matrix for the error term of VAR, σij is the standard deviation of the error term for the ith equation and ei is the selection vector with one for the ith element and zero otherwise. Each forecast error variance decomposition is normalized by the row sum as:
(10)
(10)
Total Connectedness (see note 6) is the sum of cross-variance shares, which are the fraction of the H step ahead error variances in forecasting xi due to shocks to xj.