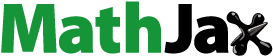
Abstract
The cyclical variation behavior of the mortgage spread has motivated some studies to investigate its relationship to economic activity. Indeed, recent empirical findings indicate that the mortgage spread is a determinant/predictor of economic activity. We define the mortgage spread as the difference between the 30-year mortgage and 10-year Treasury rates and ask whether the Blue Chip (consensus) forecasts of these series are accurate for 1988–2015. Our findings indicate that the Blue Chip forecasts of both the 30-year mortgage and 10-year Treasury rates are biased, fail to outperform the random walk benchmark, and are directionally inaccurate. However, the Blue Chip forecasts of the mortgage spread are generally unbiased, outperform the random walk benchmark, and are directionally accurate—thus of value to a user. Given such evidence, a natural extension for future research is to explore whether the predictive information content of Blue Chip forecasts of the mortgage spread is a better predictor of output growth and whether it helps improve Blue Chip forecasts of output growth.
Public Interest Statement
The literature indicates that the US mortgage spread is a determinant/predictor of economic activity. Motivated by such findings, we define mortgage spread as the difference between 30-year mortgage and 10-year Treasury rates and ask whether experts’ forecasts of these series are accurate for 1988–2015. Long-term interest rates are difficult to forecast. Both theory and empirical evidence suggest that the best forecast of future rate is today’s rate (a naïve forecast). In line with this, we show that experts’ forecasts of 30-year mortgage and 10-year Treasury rates fail to outperform naïve forecasts and are directionally inaccurate. However, experts’ forecasts of mortgage spread (calculated as the difference between experts’ forecasts of 30-year mortgage and 10-year Treasury rates) outperform naïve forecasts and are directionally accurate. Such evidence is important since accurate forecasts of mortgage spread have the potential to be a better predictor of economic activity than the actual spread.
1. Introduction
A strand of research asks whether financial spreads contain useful predictive information for output growth and inflation (Aretz & Peel, Citation2010; Estrella & Hardouvelis, Citation1991; Estrella & Mishkin, Citation1997; Stock & Watson, Citation2003). Estrella (Citation2005) constructs a formal macroeconomic model to provide the theoretical rationale for predictive information of the term spread (defined as the difference between yields on long and short maturity debt). A survey of the literature by Wheelock and Wohar (Citation2009) points to the term spread as a predictor of output growth and recessions, although the findings show variations across countries and time.Footnote1
More recent studies have focused on the mortgage spread as a determinant/predictor of economic activity. Walentin (Citation2014, p. 62) identifies three channels through which mortgage spreads can influence economic activity: “(i) house prices and residential investment through the user cost of housing, (ii) as one relevant rate in the consumption/savings decision and, (iii) the post-interest disposable income of any household with a mortgage.” He cites Hubbard and Mayer (Citation2009), Guerrieri and Lorenzoni (Citation2011) and Hall (Citation2011a, Citation2011b) who have alluded to the relationship and then goes on to add to the literature by providing empirical evidence indicating that mortgage spread shocks have economically and statistically significant impacts on economic activity. The study by Hännikäinen (Citation2016) provides further evidence by showing that the mortgage spread has useful predictive information for both real GDP and industrial production.
As noted by Darrat, Dickens, and Al-Khazali (Citation2006), the mortgage industry uses the 10-year Treasury rate as the benchmark for pricing fixed rate mortgages. As such, we follow Hännikäinen (Citation2016) and define the mortgage spread as the difference between the 30-year mortgage rate and the 10-year Treasury rate and ask whether the Blue Chip forecasts of these series are accurate for 1988–2015. Theory suggests that the best forecast of the long-term interest rate is the random walk forecast, defined as the most recent rate known at the time of the forecast (Pesando, Citation1979; Reichenstein, Citation2006). Consistent with this theory and related existing empirical evidence (Baghestani, Citation2006, Citation2008, Citation2009a; Brooks & Gray, Citation2004), our findings indicate that Blue Chip forecasts of both the 30-year mortgage rate and the 10-year Treasury rate are biased, fail to outperform the random walk benchmark, and are directionally inaccurate.Footnote2 However, Blue Chip forecasts of the mortgage spread are generally unbiased, outperform the random walk benchmark, and are directionally accurate. As noted by Schnader and Stekler (Citation1990, Citation1991), a forecast is of value if it accurately predicts directional change. Accordingly, our findings indicate that, unlike Blue Chip forecasts of 30-year mortgage and 10-year Treasury rates, Blue Chip forecasts of the mortgage spread are of value to a user since they are directionally accurate. Such results are generally consistent with existing evidence on survey forecasts of financial spreads (Baghestani Citation2005b, Citation2009b, Citation2010a).
Put together, our findings in this study contribute to the literature on the survey forecasts of financial spreads, with respect to comparing the accuracy of mortgage spread forecasts and the accuracy of the forecasts of the variables (mortgage rate and treasury rate) used to calculate the mortgage spread. Section 2 presents the methodology by first describing Blue Chip and random walk forecasts and then discussing the forecast evaluation test results. Section 3 concludes.
2. Methodology
As a long-running survey, Blue Chip asks approximately 50 analysts at major US banks, corporations, and consulting firms for their forecasts of major economic and financial indicators. The survey is conducted around the beginning of every month with the participants providing their forecasts for the current quarter and the following four quarters. Blue Chip utilizes the individual responses to calculate the consensus (mean) forecasts, which are then published in Blue Chip Financial Forecasts.Footnote3 Since the survey is conducted monthly, there are three sets of forecasts for each quarter. In this study, we focus on the one-, two-, three-, and four-quarter-ahead Blue Chip consensus forecasts of the 30-year mortgage rate and the 10-year Treasury rate made around the beginning of the second month of the quarter.
In generating the random walk forecasts of the 30-year mortgage rate (and the 10-year Treasury rate), we make use of the latest actual weekly rate available at the time of the survey published in Blue Chip Financial Forecasts along with the quarterly forecasts. The latest weekly rate for the surveys conducted in the second month of quarter t is the rate for the third week in the first month of quarter t. More specifically, let At + f be the actual rate in quarter t + f and Rt + f be the random walk forecast of At + f made in the second month of quarter t (with the forecast horizon f = 1, 2, 3, and 4 quarters).Footnote4 Then, the random walk forecast Rt + f = Awt (where Awt is the latest weekly rate known at the time of the survey).
As noted above, we follow Hännikäinen (Citation2016) and calculate the actual mortgage spread as the difference between the actual 30-year mortgage rate and the actual 10-year Treasury rate. We then calculate the Blue Chip (random walk) forecast of the mortgage spread as the difference between the Blue Chip (random walk) forecast of the 30-year mortgage rate and the Blue Chip (random walk) forecast of the 10-year Treasury rate.
In evaluating Blue Chip forecasts of the 30-year mortgage rate, 10-year Treasury rate, and mortgage spread, we focus on answering the following three questions:
(1) | Are Blue Chip forecasts unbiased? | ||||
(2) | Are Blue Chip forecasts more informative than the random walk benchmark? | ||||
(3) | Are Blue Chip forecasts directionally accurate? |
Figure plots the actual rates of the 30-year mortgage and 10-year Treasury for 1988Q1–2015Q4. As indicated, the 30-year mortgage has a mean rate of 6.77% with a high (low) rate of 10.82 (3.36), and the 10-year Treasury has a mean rate of 5.12% with a high (low) rate of 9.21 (1.64). Figure plots the actual mortgage spread for 1988Q1–2015Q4. Again, as indicated, the spread has a mean rate of 1.65% with a high (low) rate of 2.59 (1.23). We focus on Blue Chip and random walk forecasts of these series that are made in the first quarter of 1988 through the fourth quarter of 2014. As such, the sample periods for the one-, two-, three-, and four-quarter-ahead forecasts are, respectively, 1988Q2–2015Q1, 1988Q3–2015Q2, 1988Q4–2015Q3, and 1989Q1–2015Q4, with 108 observations for each forecast horizon.
2.1. Are Blue Chip forecasts unbiased?
We start with specifying the following test equations for unbiasedness,(1)
(1)
(2)
(2)
where At+f and At−1 are the actual series for, respectively, quarters t + f and t − 1, and Pt+f is the Blue Chip forecast of At+f made in quarter t. We subtract At-1 from both the actual and forecast series in Equation (1) in order to alleviate problems arising from the non-stationarity of the series (Fair & Shiller, Citation1990). The forecast is unbiased when the individual null hypotheses that α = 0, β = 1, and α′ = 0 cannot be rejected (Holden & Peel, Citation1990). With the forecasts made in quarter t, the error term ut+f follows an f-order moving-average process under the null hypothesis of unbiasedness. Since forecast errors are generally heteroscedastic, we utilize the Newey and West (Citation1987) procedure to estimate the above test equations in order to correct for both heteroscedasticity and serial correlation over f quarters.
Rows 1–4 of Table (Table ) report, respectively, the OLS estimates of Equations (1) and (2) with the correct standard errors for the one-, two-, three-, and four-quarter-ahead Blue Chip forecasts of the 30-year mortgage rate (10-year Treasury rate). As indicated by superscript “a,” these forecasts fail to be unbiased since we reject the individual null hypotheses that α = 0, β = 1, and α′ = 0.
Table 1. Forecast accuracy test results: 30-year mortgage rate
Table 2. Forecast accuracy test results: 10-year Treasury rate
Rows 1–4 of Table report, respectively, the OLS estimates of Equations (1) and (2) with the correct standard errors for the one-, two-, three-, and four-quarter-ahead Blue Chip forecasts of the mortgage spread. As can be seen, the one-, two-, and three-quarter-ahead forecasts are all unbiased since we cannot reject the individual null hypotheses that α = 0, β = 1, and α′ = 0. However, the four-quarter-ahead forecasts fail to be unbiased since we reject the null hypotheses that α = 0 and β = 1 both individually and jointly. Further inspection reveals that the one- through four-quarter-ahead Blue Chip forecasts of the mortgage spread in rows 1–4 are all free of systematic bias since we cannot reject the null hypothesis that α′ = 0. This conclusion is consistent with the mean forecast error (ME) and mean absolute forecast error (MAE) estimates reported in rows 1–4 of Table . That is, the ME estimates, ranging from 0.027 to 0.066, are small compared to the MAE estimates, ranging from 0.148 to 0.219.
Table 3. Forecast accuracy test results: Mortgage spread
2.2. Are Blue Chip forecasts more informative than the random walk benchmark?
In answering, we estimate the following encompassing test equation,(3)
(3)
where, again, At+f and At-1 are the actual series for, respectively, quarters t + f and t − 1, and Pt+f (Rt+f) is the Blue Chip (random walk) forecast of At+f made in quarter t. A significant and positive estimate of γB with an insignificant estimate of γR indicates that the Blue Chip forecast contains more predictive information than the random walk benchmark; the converse is also true. Blue Chip and random walk forecasts contain distinct predictive information when the estimates of γB and γR are both positive and significant. Blue Chip and random walk forecasts contain similar predictive information when the estimates of both γB and γR are insignificant.
Rows 5–8 of Table (Table ) report, respectively, the OLS estimates of Equation (3) with the correct standard errors for the one-, two-, three-, and four-quarter-ahead forecasts of the 30-year mortgage rate (10-year Treasury rate). As can be seen, for the one-, two-, and three-quarter-ahead forecasts, the estimates of γB are insignificant but the estimates of γR are both positive and significant, meaning that random walk forecasts are more informative than Blue Chip forecasts. For the four-quarter-ahead forecasts, the estimates of γB and γR are both insignificant, indicating that Blue Chip and random walk forecasts contain similar predictive information.
Rows 5–8 of Table report, respectively, the OLS estimates of Equation (3) with the correct standard errors for the one-, two-, three-, and four-quarter-ahead forecasts of the mortgage spread. As can be seen, the estimates of both γB and γR in row 5 are positive and significant, meaning that the one-quarter-ahead Blue Chip and random walk forecasts contain distinct predictive information. However, the estimates of γB in rows 6–8 are both positive and significant but the estimates of γR are insignificant, indicating that the two-, three-, and four-quarter-ahead Blue Chip forecasts contain more predictive information than the random walk benchmark.
2.3. Are Blue Chip forecasts directionally accurate?
We follow the literature and employ the test procedure first introduced by Merton (Citation1981) and Henriksson and Merton (Citation1981) and later refined by Schnader and Stekler (Citation1990), among others. Table presents the two-by-two contingency table in which the actual change is (At+f − Awt) and the predicted change is (Pt+f − Awt), with four elements representing the numbers of correct directional predictions (n1 and n2) and incorrect directional predictions (n3 and n4). As such, the overall directional accuracy rate is πAll = (n1 + n2)/n, with n representing the sample size. In addition, the proportion of correctly predicted upward moves is πUp = n1/(n1 + n3), and the proportion of correctly predicted downward moves is πDown = n2/(n2 + n4).
Table 4. Contingency table
Table reports these statistics for the Blue Chip forecasts of the 30-year mortgage rate in rows 1–4, the 10-year Treasury rate in rows 5–8, and the mortgage spread in rows 9–12. In testing the null hypothesis of no directional association between actual and predicted changes, we use Fisher’s exact test and the chi-square tests with and without Yate’s continuity correction (Sinclair, Stekler, & Kitzinger, Citation2010). As can be seen, we cannot reject this null hypothesis for the forecasts in rows 1–8, meaning that the one-, two-, three-, and four-quarter-ahead Blue Chip forecasts of the 30-year mortgage rate and the 10-year Treasury rate fail to be directionally accurate. These test results are consistent with the low overall accuracy rates (πAll), ranging from 0.41 to 0.55. Further results indicate that, for each forecast in rows 1–8, the proportion of correctly predicted upward moves (πUp), ranging from 0.59 to 0.86, is high, but the proportion of correctly predicted downward moves (πDown), ranging from 0.24 to 0.37, is quite low. As such, it appears that the directional inaccuracy of the Blue Chip forecasts of the 30-year mortgage rate and the 10-year Treasury rate is due to inaccurately predicting the downward moves. Baghestani and Kherfi (Citation2008) report a similar finding using the data from the Michigan Surveys of Consumers. They argue that “When interest rates are relatively stable, consumers may be more concerned with the explicit cost of borrowing since the declines in interest rates are perceived to be too small for the implicit cost to matter. Therefore, it becomes less important for them to accurately predict the downward moves in interest rates” (p. 727).
Table 5. Directional accuracy test results
For the one-, two-, three-, and four-quarter-ahead Blue Chip forecasts of the mortgage spread in rows 9–12, the overall accuracy rate (πAll), ranging from 0.60 to 0.69, happens to be high. As indicated by superscript “b,” we reject the null hypothesis of no directional association for these forecasts, meaning that they are directionally accurate and thus are of value to a user. Further results for every forecast in rows 9–12 indicate that the proportion of correctly predicted downward moves (πDown), ranging from 0.67 to 0.81, is much more than the proportion of correctly predicted upward moves (πAll), ranging from 0.43 to 0.61. In testing the null hypothesis of no asymmetry, which is defined as the proportion of incorrectly predicted upward moves (1 − πUp) equals the proportion of incorrectly predicted downward moves (1 − πDown), we use the chi-square test described in Berenson, Levine, and Rindskopf (Citation1988, sec. 11.4.1). With the chi-square test p-value of 0.570 greater than 0.10 in row 9, we cannot reject the null hypothesis of “no asymmetry,” meaning that the one-quarter-ahead Blue Chip forecast is of value to a user who assigns the same loss (cost) to both incorrect upward and downward moves in the mortgage spread. However, with the chi-square test p-values (in rows 10–12) lower than 0.10, we reject the null hypothesis of “no asymmetry.” As such, we conclude that the two-, three-, and four-quarter-ahead forecasts are of value to a user who assigns high (low) cost to incorrect downward (upward) moves in the mortgage spread.
3. Conclusions
Recent studies have focused on the mortgage spread as a determinant/predictor of economic activity. Walentin (Citation2014) shows that mortgage spread shocks have economically and statistically significant impacts on economic activity, and Hännikäinen (Citation2016) shows that the mortgage spread has useful predictive information for both real GDP and industrial production. We define the mortgage spread as the difference between the 30-year mortgage rate and the 10-year Treasury rate and ask whether the Blue Chip forecasts of these series are accurate for 1988–2015. Our findings indicate that Blue Chip forecasts of both 30-year mortgage and 10-year Treasury rates are biased, fail to outperform the random walk benchmark, and are directionally inaccurate. However, the Blue Chip forecasts of the mortgage spread are generally unbiased, outperform the random walk benchmark, and are directionally accurate – thus of value to a user.
A strand of research focuses on using survey data to generate accurate forecasts and/or improve professional forecasts of economic and financial indicators (Baghestani, Citation2005a, Citation2015; Baghestani & AbuAl-Foul, Citation2017; Christiansen, Eriksen, & Møller, Citation2014; Dees & Soares Brinca, Citation2013; Kellstedt, Linn, & Hannah, Citation2015; Lahiri, Monokroussos, & Zhao, Citation2016). Given the evidence presented in this study, a natural extension for future research is to explore whether Blue Chip forecasts of the mortgage spread are more useful than the actual mortgage spread in predicting output growth, and whether Blue Chip forecasts of the mortgage spread can help improve Blue Chip forecasts of output growth. Further future analysis may involve controlling for mortgage-related developments, such as those during the US recession of 2007–2009, and exploring the reason(s) behind the accuracy of mortgage spread forecasts and the inaccuracy of the forecasts of the variables used to compute the spread (i.e. mortgage rate and treasury rate).
Funding
The authors received no direct funding for this research.
Additional information
Notes on contributors
Hamid Baghestani
Hamid Baghestani has a 1982 PhD in Economics from the University of Colorado, Boulder. He is currently professor of Economics at the American University of Sharjah, UAE. His research interests include time-series analysis, macro-econometric modeling and forecasting, energy economics, monetary economics, and financial markets. He has published widely on these topics in internationally respected peer-reviewed journals such as Applied Economics, Energy Economics, Energy Policy, Journal of Business, Journal of Forecasting, Journal of Industrial Economics, Journal of Macroeconomics, and Oxford Bulletin of Economics and Statistics.
Notes
1. Term spreads have also been found useful in predicting directional change in home sales (Baghestani & Kaya, Citation2016). In another study, Baghestani and Toledo (Citation2017) show that the Blue Chip forecasts of the Australian–US (UK–US) term spread differential helps predict directional change in the Australian–US (UK–US) exchange rate.
2. There are studies which have produced more accurate forecasts than the random walk. For example, CitationBaghestani (2010b) utilizes the information in expected inflation to generate the 10-year Treasury rate forecasts that are superior to the random walk benchmark. Also, Baghestani (Citation2017) shows that consumer survey data on both mortgage interest rates and expected inflation can help produce more accurate 30-year mortgage rate forecasts than the random walk benchmark.
3. The historical Blue Chip Financial Forecasts were purchased from Aspen Publishers, Inc.
4. The actual quarterly rates of the 30-year mortgage and the 10-year Treasury are obtained from the Federal Reserve Bank of St. Louis database.
References
- Aretz, K., & Peel, D. A. (2010). Spreads versus professional forecasters as predictors of future output change. Journal of Forecasting, 29, 517–522.10.1002/for.v29:6
- Baghestani, H. (2005a). Improving the accuracy of recent survey forecasts of the T-bill rate. Business Economics, 40, 36–40.10.2145/20050204
- Baghestani, H. (2005b). On the rationality of professional forecasts of corporate bond yield spread. Applied Economics Letters, 12, 213–216.10.1080/1350485042000338635
- Baghestani, H. (2006). An evaluation of the professional forecasts of US long-term interest rates. Review of Financial Economics, 15, 177–191.10.1016/j.rfe.2005.06.001
- Baghestani, H. (2008). Consensus vs. time-series forecasts of U.S. 30-year mortgage rates. Journal of Property Research, 25, 45–60.10.1080/09599910802397073
- Baghestani, H. (2009a). Forecasting in efficient bond markets: Do experts know better? International Review of Economics and Finance, 18, 624–630.10.1016/j.iref.2008.10.007
- Baghestani, H. (2009b). Survey evidence on forecast accuracy of term spreads. Review of Financial Economics, 18, 156–162.10.1016/j.rfe.2009.02.001
- Baghestani, H. (2010a). How well do experts predict interbank loan rates and spreads? Economics Letters, 109(1), 4–6.10.1016/j.econlet.2010.07.004
- Baghestani, H. (2010b). Forecasting the ten-year U.S. Treasury rate. Journal of Forecasting, 29, 673–688.10.1002/for.1165
- Baghestani, H. (2015). Predicting gasoline prices using Michigan survey data. Energy Economics, 50, 27–32.10.1016/j.eneco.2015.04.015
- Baghestani, H. (2017) Do US consumer survey data help beat the random walk in forecasting mortgage rates? Cogent Economics & Finance, 5(1), 1343017. doi:10.1080/23322039.2017.1343017
- Baghestani, H., & AbuAl-Foul, B. (2017). Comparing federal reserve, blue chip, and time series forecasts of US output growth. Journal of Economics and Business, 89, 47–56.10.1016/j.jeconbus.2016.11.001
- Baghestani, H., & Kaya, I. (2016). Do financial indicators have directional predictability for US home sales? Applied Economics, 48, 1349–1360.10.1080/00036846.2015.1100253
- Baghestani, H., & Kherfi, S. (2008). How well do US consumers predict the direction of change in interest rates? The Quarterly Review of Economics and Finance, 48, 725–732.10.1016/j.qref.2007.06.002
- Baghestani, H., & Toledo, H. (2017). Do analysts’ forecasts of term spread differential help predict directional change in exchange rates? International Review of Economics and Finance, 47, 62–69.10.1016/j.iref.2016.10.003
- Berenson, M. L., Levine, D. M., & Rindskopf, D. (1988). Applied statistics: A first course. New York, NY: Prentice Hall.
- Brooks, R., & Gray, J. B. (2004). History of the forecasters: Naïve forecasts are better than the consensus WSJ forecast. The Journal of Portfolio Management, 31, 113–117.10.3905/jpm.2004.443329
- Christiansen, C., Eriksen, J. N., & Møller, S. V. (2014). Forecasting US recessions: The role of sentiment. Journal of Banking and Finance, 49, 459–468.10.1016/j.jbankfin.2014.06.017
- Darrat, A. F., Dickens, R. N., & Al-Khazali, O. (2006). Interactions between mortgage and other capital markets in the USA: Has financial deregulation made a difference? Applied Financial Economics, 16, 335–345.10.1080/09603100500186606
- Dees, S., & Soares Brinca, P. S. (2013). Consumer confidence as a predictor of consumption spending: Evidence for the United States and the Euro area. International Economics, 134, 1–14.10.1016/j.inteco.2013.05.001
- Estrella, A. (2005). Why does the yield curve predict output and inflation? The Economic Journal, 115, 722–744.10.1111/ecoj.2005.115.issue-505
- Estrella, A., & Hardouvelis, G. A. (1991). The term structure as a predictor of real economic activity. The Journal of Finance, 46, 555–576.10.1111/j.1540-6261.1991.tb02674.x
- Estrella, A., & Mishkin, F. S. (1997). The predictive power of the term structure of interest rates in Europe and the United States: Implications for the European Central Bank. European Economic Review, 41, 1375–1401.10.1016/S0014-2921(96)00050-5
- Fair, R. C., & Shiller, R. J. (1990). Comparing information in forecasts from econometric models. American Economic Review, 80, 375–389.
- Guerrieri, V., & G. Lorenzoni (2011) Credit crises, precautionary savings, and the liquidity trap. In 2011 Meeting Papers (No. 1414). Society for Economic Dynamics.
- Hall, R. (2011a). The long slump. American Economic Review, 101, 431–469.10.1257/aer.101.2.431
- Hall, R. (2011b). The high sensitivity of economic activity to financial frictions. The Economic Journal, 121, 351–378.10.1111/j.1468-0297.2011.02421.x
- Hännikäinen, J. (2016). The mortgage spread as a predictor of real-time economic activity. Applied Economics Letters, 23, 112–116.10.1080/13504851.2015.1054064
- Henriksson, R. D., & Merton, R. C. (1981). On market timing and investment performance. II. Statistical procedures for evaluation forecasting skills. The Journal of Business, 54, 513–533.10.1086/jb.1981.54.issue-4
- Holden, K., & Peel, D. A. (1990). On testing for Unbiasedness and efficiency of forecasts. The Manchester School, 58, 120–127.10.1111/j.1467-9957.1990.tb00413.x
- Hubbard, R.G., & C. J. Mayer (2009) The mortgage market meltdown and house prices. The BE Journal of Economic Analysis & Policy, 9(3). doi:10.2202/1935-1682.2223
- Kellstedt, P. M., Linn, S., & Hannah, A. L. (2015). The usefulness of consumer sentiment: Assessing construct and measurement. Public Opinion Quarterly, 79, 181–203.10.1093/poq/nfu056
- Lahiri, K., Monokroussos, G., & Zhao, Y. (2016). Forecasting consumption: The role of consumer confidence in real time with many predictors. Journal of Applied Econometrics, 31, 1254–1275.10.1002/jae.2494
- Merton, R. C. (1981). On market timing and investment performance. I. An equilibrium theory of value for market forecasts. The Journal of Business, 54, 363–406.10.1086/jb.1981.54.issue-3
- Newey, W. K., & West, K. D. (1987). A simple, positive semi-definite, heteroscedasticity and autocorrelation consistent covariance matrix. Econometrica, 55, 703–708.10.2307/1913610
- Pesando, J. E. (1979). On the random walk characteristics of short- and long-term interest rates in an efficient market. Journal of Money, Credit, and Banking, 11, 457–466.10.2307/1992047
- Reichenstein, W. (2006). Rationality of naïve forecasts of long-term rates. The Journal of Portfolio Management, 32, 116–119.10.3905/jpm.2006.611812
- Schnader, M. H., & Stekler, H. O. (1990). Evaluating predictions of change. The Journal of Business, 63, 99–107.10.1086/jb.1990.63.issue-1
- Schnader, M. H., & Stekler, H. O. (1991). Evaluating predictions of change: An application to inflation forecasts. Applied Financial Economics, 1, 135–137.
- Sinclair, T. M., Stekler, H. O., & Kitzinger, L. (2010). Directional forecasts of GDP and inflation: A joint evaluation with an application to Federal Reserve predictions. Applied Economics, 42, 2289–2297.10.1080/00036840701857978
- Stock, J. H., & Watson, M. W. (2003). Forecasting output and inflation: The role of asset prices. Journal of Economic Literature, 41, 788–829.10.1257/jel.41.3.788
- Walentin, K. (2014). Business cycle implications of mortgage spreads. Journal of Monetary Economics, 67, 62–77.10.1016/j.jmoneco.2014.07.005
- Wheelock, D. C., & Wohar, M. E. (2009). Can the term spread predict output growth and recessions? A survey of the literature. Federal Reserve Bank of St. Louis Review, 91, 419–440.