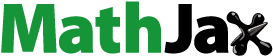
Abstract
This paper examines whether economic policy uncertainty (EPU) causes real housing returns in 8 emerging economies for which EPU data are available namely: Brazil, Chile, China, India, Ireland, Russia, South Africa and South Korea. Quarterly data were used for the analysis. The study uses cross-sample validation (CSV) Granger causality approach which obviates the need to partition the data into an in-sample and out-of-sample periods when limited data are available as in this study. Results based on the CSV full sample period indicate no evidence of economic policy uncertainty Granger causing real housing returns except for Chile and China. However, based on CSV rolling window results, there is evidence of time varying causality in all the countries except India. The implications of these findings are drawn.
PUBLIC INTEREST STATEMENT
The recent global crisis which stated in the housing market in the United States has raised the interest of all the stakeholders. Moreover, as the housing market is still struggling to recover from the aftermath of the crisis, it has become important to examine its drivers. Uncertainty surrounding economic policies is envisaged to be among these because such uncertainty could delay investment in the housing market as investors would either adopt a wait- and-see strategy or divert their investment to other portfolios. In this paper, the role of economic policy uncertainty (EPU) on real housing returns in 8 emerging markets was investigated using a robust analytical method. The findings show that EPU has significant causal effect on real housing returns. This effect varies over time. This should raise concern for investors, policymakers and researchers since development in the housing market could impact economic growth and welfare distribution.
1. Introduction
The 2007/2009 global economic and financial crisis which started as a subprime mortgage crisis had its root in the housing market. This has led to heightened interest in this market since the crisis given its susceptibility to shocks and its crucial role in the economy. According to Leamer (Citation2015), eight out of ten US post-war recessions were preceded by substantial problems in housing and consumer durables and these changes in the housing sector have had substantial effects on economic activity in the US. The recent crisis has highlighted deficiencies in the system of financial regulation. The policy dilemmas faced by central banks setting interest rates have been sharpened by deficiencies in financial regulation and fiscal policies. The causes and consequences of the operation of housing and credit markets have been misunderstood and this has contributed to the failure of governments to implement reforms that would stabilize their economies and financial systems and consequently reduce inequality and social exclusion (Muellbauer & Murphy, Citation2008). In this paper, economic policy uncertainty (EPU) is investigated as a driver or cause of housing returns.
The aftermath of the global crisis has increased both housing price volatility and economic uncertainty (Hirata, Kose, Otrok, & Terrones, Citation2013). Given the strategic position of the US in the world, any shock arising from there could be easily transmitted to other countries. Hence, this study focuses on emerging economies. Figure shows the historical evolution of housing returns and EPU in the eight emerging countries to be studied. The shaded bars indicate the USA recession. From these plots, it is observed that uncertainty has heightened since the last recession while housing returns has fallen or remained stagnant. The development in the housing markets is important for portfolio managers, investors, would-be homeowners, financial institutions and policymakers, as it helps to provide information on the probability of mortgage defaults, valuation of mortgage backed securities, and property tax revenue streams (Barros, Gil-Alana, & Payne, Citation2015). Housing prices provide indication as to where the economy is heading to (Aye, Balcilar, Bosch, & Gupta, Citation2014; Leamer, Citation2015). According to Muellbauer & Murphy (Citation2008), housing wealth is crucial for macroeconomic fluctuations and the distribution of welfare but this depends on the institutional framework governing property rights, access to credit, financial architecture and regulation, and trading costs. Therefore, understanding the role of EPU in the housing market is crucial for housing market development, economic growth and welfare.
Su, Li, Lobonţ, and Zhao (Citation2016) noted that attributing housing market instabilities to increased policy uncertainty is not uncommon. When there is uncertainty, policy authorities and investors are reluctant on the appropriate course of action. This may delay economic activity. Theoretically, uncertainty and the housing market should share a relationship. This is because EPU could delay investment decisions in the housing market as it increases the real-option value of waiting due to its potential to reduce demand for capital, and hence housing returns as well as the irreversible nature of housing investments (Burnside, Eichenbaum, & Rebelo, Citation2016; Calcagnini & Saltari, Citation2000; Cunningham, Citation2006; Hirata et al., Citation2013). Pástor and Veronesi (Citation2013) also argue that increased political uncertainty can lead to rising cost of financing housing projects. Also, the impact of uncertainty on income, mortgage rates, and credit conditions, may make households to reconsider their housing investment choices. Therefore, it is pertinent to empirically examine the causal link between economic uncertainty and housing returns.
A few studies as evidenced in the literature review section have investigated the relationship between economic uncertainty and housing prices and/or volatilities. Most of these studies barring André, Bonga-Bonga, Gupta, and Mwamba (Citation2017) and Christou, Gupta, and Hassapis (Citation2017) focused on in-sample predictability of economic uncertainty for the housing market. However, there is widespread evidence that in-sample predictive ability does not guarantee out-of-sample accuracy of forecasts (Rapach & Zhou, Citation2013). In-sample errors are likely to understate forecasting errors (Tashman, Citation2000). Ashley and Tsang (Citation2014) confirmed that the in-sample estimation of causality can be a poor approach to out-of-sample forecasting. It is a clear trend in recent finance literature to focus on the out-of-sample predictive ability as most models perform well in-sample but fail in out-of-sample forecasts (Aye, Deale, & Gupta, Citation2016; Campbell & Thompson, Citation2008; Welch & Goyal, Citation2008). Moreover, overfitting and structural changes may further aggravate the divergence between in-sample and post-sample performance (Tashman, Citation2000). This study contributes to this line of research by examining the causal link between EPU and housing returns using the cross-sample validation (CSV) Granger causality approach which avoids the need to partition the data a priori into an in-sample and out-of-sample periods (Ashley & Tsang, Citation2014). Ashley and Tsang (Citation2014) argued that the practice of a priori or arbitrarily partitioning of the data into in-sample period—used only for model specification/estimation- and out-of-sample period-used only for evaluating the model’s forecast ability is not feasible with samples of modest length (T ≤ 150) commonly seen in quarterly and sometimes monthly data sets. Thus, they proposed a CSV scheme whereby all of the available data are used at once in the testing procedure and every possible in-sample versus post-sample partitioning is examined. Thus, this preserves the power of in-sample testing. It also preserves most of the credibility of the out-of-sample testing by basing model forecast evaluation on data not used for estimating the particular model’s coefficients.
Moreover, in an empirical experiment, Hansen and Timmermann (Citation2012) demonstrated that out-of-sample forecast evaluation results can critically depend on how the sample split is determined. Overall, cross-validation is used instead of the conventional validation of partitioning the data into two to avoid losing significant modeling or testing capability especially when the data are not enough (Kyriazakou & Panagiotidis, Citation2017; Seni & Elder, Citation2010) as in the current study. Aside, the CSV approach allows one to determine the specific time periods for which causal relationship exists which is not the case with the approaches used by André et al. (Citation2017) and Christou et al. (Citation2017). In this study, eight emerging market economies namely Brazil, Chile, China, India, Ireland, Russia, South Africa and South Korea are considered based on the availability of EPU data. Aside South Korea which was included in the panel in Christou et al. (Citation2017), and China and India in Chow, Cunado, Gupta, and Wong (Citation2017), the relationship has not been previously examined for the remaining countries to the best of my knowledge. Moreover, given the above argument on in-sample versus out-of-sample, it is worthwhile to revisit the relationship for China and India since Chow et al. (Citation2017) utilized an in-sample approach.
Since uncertainty is a latent variable that needs to be measured, this study used the news-based measure of uncertainty, widely known as the economic policy uncertainty index (EPU) developed by Baker, Bloom, and Davis (Citation2016). Despite the availability of other measures of uncertainty such as historical volatility, stochastic volatility, implied volatility, realized volatility, conditional volatility, partisan conflict and macroeconomic uncertainty among others (Azzimonti, Citation2018; Fernández-Villaverde, Guerrón-Quintana, Kuester, & Rubio-Ramírez, Citation2015; Jurado, Ludvigson, & Ng, Citation2015; Rafiq et al., Citation2009; Rossi & Sekhposyan, Citation2015; Shoag & Veuger, Citation2016), EPU has received wide acceptance in empirical applications (André et al., Citation2017; Redl, Citation2015), due to the fact that it does not require any complicated model estimation for its generation and it has wider coverage beyond the US economy unlike many other uncertainty indicators. The results showed that there is time-varying causality from EPU to housing returns in all countries except India whereas the full-sample analysis detects causality for only Chile and China.
The remainder of the paper is organized as follows: the literature is presented in Section 2. The empirical model is presented in Section 3. Section 4 presents the data. Results are presented in Section 5 while Section 6 concludes the study.
2. Literature review
There may be many drivers of housing prices or returns such as income, the housing stock, demography, credit availability, interest rates, lagged appreciation, housing collateral, downpayment constraints and institutional variations (Muellbauer & Murphy, Citation2008). This review focuses on studies that have examined the relationship between EPU and the housing market.Footnote1 For instance, in a study by Sum and Brown (Citation2012) which was based on a VAR model and monthly data covering 1985–2011, there is no support for a significant causal link between EPU and Real Estate Investment Trust returns in the United States. Using data from January 1999 to June 2013 and volatility impulse response functions (VIRFs) introduced by Hafner and Herwartz (Citation2006) as well as variance causality test, Ajmi, Babalos, Economou, and Gupta (Citation2014) found a two-way transmission channel between US-listed REITs conditional volatility and macroeconomic uncertainty proxied by two indices namely EPU Index and the Equity Market Uncertainty Index.
Based on the Dynamic Conditional Correlation Generalized Autoregessive Conditional Heteroskedastic (DCC-GARCH) model, Antonakakis, Gupta, and André (Citation2015) found that the correlation between EPU and housing market returns in US is negative consistently, but with varying magnitude over time, peaking during the 2007/2009 financial crisis. Su et al. (Citation2016) used a bootstrap rolling window causality test developed by Balcilar, Ozdemir, and Arslanturk (Citation2010) and found that EPU has no impact on housing returns in Germany but the latter has significant effect on EPU for a limited time period. Housing returns do not have significant effect on EPU in most time periods. The causal relationship between EPU and real house prices in Canada, France, Germany, Italy, Spain, UK, and US was examined by El Montasser et al. (Citation2016) who employed quarterly data from 2001 to 2013 and a bootstrap panel VAR. Results showed that a bi-directional causality exists for France and Spain, while unidirectional causality was found for the remaining countries. In a study by Antonakakis, André, and Gupta (Citation2016), they found a time-varying volatility spillover effect from EPU to real housing returns in US based on a VAR-based approach.
For China and India, Chow et al. (Citation2017) used linear and nonlinear panel and time series models. Results based on linear model showed a unidirectional causality from EPU growth to housing returns in China but not in India. The nonlinear Granger causality tests found mostly unidirectional causality from EPU to housing returns in both countries. When the two countries were taken as a panel, both panel linear and panel nonlinear tests rejected the null of EPU not Granger causing housing returns. André et al. (Citation2017) used monthly data from 1953:1 to 2014:2 and a k-th order non-parametric Granger causality test. They split the whole sample into two equal parts of in-sample (1953:2–1983:8) and out-of-sample (1983:9–2014:1) periods. Their results showed that EPU predicts both real housing returns and its volatility in the United States. For ten OECD countries: Canada, France, Germany, Italy, Japan, the Netherlands, South Korea, Spain, UK, and US, Christou et al. (Citation2017) used quarterly data from 2003 to 2014 with an out-of-sample period of 2008:Q2–2014:Q4 and panel VAR models. They evaluated the point and density forecasts at one-, two-, four-, and eight-quarters-ahead and found a predictive power of EPU for housing returns. Aye, Clance, and Gupta (Citation2017) employed a hazard model to investigate the spill-over effect of economic uncertainty on the housing market cycles in 12 OECD countries (Australia, Canada, Chile, France, Germany, Ireland, Italy, Japan, The Netherlands, Spain, Sweden, United Kingdom and United States) and find that while higher economic uncertainty significantly affects the probability of exiting housing market busts it has no significant effect on the probability of leaving booms and normal times.
Apart from André et al. (Citation2017) and Christou et al. (Citation2017), the other studies focused on in-sample predictability (causality) of economic uncertainty for the housing market. However, it has been argued that “a sound and natural approach to testing predictive ability “must rely primarily on the out-of sample forecasting performance” (Ashley, Granger, & Schmalensee, Citation1980). Out-of-sample analysis based on partitioning the sample may lead to a loss of information and lower power in small samples giving rise to inaccurate conclusions. The current study therefore contributes in this regard by examining the predictive power of EPU for real housing returns for emerging economies most of which have not been previously examined using an out-of-sample cross-validation approach developed by Ashley and Tsang (Citation2014) to avoid the in-sample versus out-of-sample ad hoc data split and its consequences for our sample. This current paper also differs from André et al. (Citation2017) and Christou et al. (Citation2017) as it analyzed the explicit time-varying relationship between EPU and housing returns. In order words, specific time periods for which there are significant causal relationship are determined for each country.
3. Empirical model
This study employs the CSV test for Granger causality developed by Ashley and Tsang (Citation2014). The model for over the full (unrestricted) information set is given as
where is
vector of explanatory variables including the
putatively causative variables. The model for
over the restricted information set is given as:
where the array
is identical to
but omits the columns containing the data on the
putatively causative variables and where
omits the corresponding components. Additional explanatory variables may be included in
. In this study, only lagged values of real housing returns,
are included in
aside the lagged
putatively causative variable, EPU.
Assuming the sample of observations is split into two parts: the first
observations and the remaining
observations. Letting the first
observations be the “in-sample” period and the remaining
observations be the “post-sample” period, for any given sample-split
, the
can be computed as
where and
are an unrestricted and a restricted sum of
squared “out-of-sample” prediction errors respectively.Footnote2
would be potentially useful in testing the null hypothesis that the coefficients on all
putatively Granger-causing explanatory variables are zero. However,
depends on the (arbitrary) sample-split at period
. To avoid this dependence on the sample-split choice, the Granger-causality inference can be based on every possible value of
. This can be done by using a sample quantile of the observed values of
over all of the feasible values of
as the test statistic.
Letting denote the
sample quantile of the distribution from which the observations
are drawn, these sample order statistics can be expressed as:
where must lie in the interval
so that both
, the estimator of
in Equation (1) using only the first
observations, and
, the estimator of
in Equation (1) using only the last
observations are computable.
Granger causality tests based on are appropriately called “cross-sample validation” tests because they are based on applying the model coefficients estimated on one portion of the data to predicting the other portion of the data. Consequently,
, the sample median of
is denoted as the “
” statistic. Analogously,
, the sample third quartile of
is denoted as the “
” statistic, and so forth for the other values of
. These sample order statistics, by construction, do not depend on
. Granger causality inferences based on
are usually obtained using bootstrap methods as this ensures that the sizes of the CSV tests are reasonably accurate, even for the modest sample lengths. Ashley and Tsang (Citation2014) recommend using the “third-quartile” or CSV75 CSV test since it had the highest empirical power relative to the others (see also Kyriazakou & Panagiotidis, Citation2017; Plakandaras, Gupta, Gogas, & Papadimitriou, Citation2017). Hence, in the empirical section only CSV75 results will be reported.
Aside computing the usual in-sample F-test, the MSE-F statistic for post-sample (out-of-sample) tests is also computed as:
where is the number of post-sample periods,
is the one period ahead forecasting error made by the unrestricted model in period
,
is the one period ahead forecasting error made by the restricted model in period
.
4. Data
The data consist of two variables: real housing prices and EPU for 8 emerging countries namely Brazil, Chile, China, India, Ireland, Russia, South Africa and South Korea. Following Cesa-Bianchi, Cespedes, and Rebucci (Citation2015), the housing prices data were obtained from the OECD house price database, the BIS (Bank of International Settlement) property price dataset and the Federal Reserve of Dallas international house price database. The real house prices were obtained by deflating the nominal house prices with a country-specific consumer price index. The EPU indices were obtained from www.policyuncertainty.com. Month-by-month searches of leading newspapers in each country, for terms pertaining to uncertainty, the economy and policy were performed by Baker et al. (Citation2016). Although there are other measures of uncertainty as mentioned in the introductory section, EPU is widely used in empirical applications because it does not require any complex model estimation to construct it and it is available for a number of developed and emerging markets unlike other measures of uncertainty. Moreover, EPU incorporates different dimensions or categories of policy such as monetary, fiscal, regulatory and health policies amongst others. It has also been validated in terms of reliability, accuracy, bias, consistency as well as market-use value as commercial data providers such as Bloomberg, FRED, Haver and Reuters use these indices to meet demands from banks, hedge funds, corporates and policy makers (Baker et al., Citation2016). The original source documents the EPU data on monthly frequency. To be consistent with the real housing price data which are quarterly, the EPU data were converted into their quarterly frequency in this study by taking averages over 3 months comprising a quarter.
By definition, real housing returns is the first-difference of the natural log of real house price index. However, a preliminary analysis was conducted to determine the unit root property of the data. These include the Augmented Dickey–Fuller (ADF, Citation1979), Phillips–Perron (PP, Citation1988) and Ng and Perron (NP, Citation2001) unit root tests. In addition, the Zivot and Andrews (Citation1992) unit root test that allows for possibility of structural break is conducted. The results as presented in the Appendix A show that based on the tests without break, real housing prices are I(1) while EPU are I(0), with exception of EPU for India which is I(1). However, based on the test with break, unit root is rejected for only Chile, India and South Korea in the case of real housing prices and for Chile, Russia and South Africa in the case of EPU. All countries returns and EPU exhibit at least a break and this justifies the use of a model that accounts for the time varying nature of these series. Since there seems to be conflicting conclusion between the unit root tests with and without break especially for EPU and given the stationarity requirement, all variables are used in their log first-difference form (see also Balcilar, Gupta, & Segnon, Citation2016b; Chow et al., Citation2017; Li, Balcilar, Gupta, & Chang, Citation2016).Footnote3 Table reports key statistics for the log-difference of real house price and EPU for each country. The starting and ending dates are determined by data availability. Real house price returns is highest in India (1.68% per quarter) and lowest, in fact negative in South Korea (−0.27% per quarter). The housing market in Chile and Russia are more volatile than the rest of the economies while that of China is the least volatile. A normally distributed series should have skewness value of about zero and kurtosis of about 3. From Table , real housing returns are mostly negatively skewed with excess kurtosis. However, based on the Jarque–Bera test, the real housing returns in China, Ireland and South Africa are normally distributed while the rest are not. For EPU, the highest growth is witnessed in Brazil (2.27% per quarter) while the least growth is witnessed in India (0.05%). EPU are mostly positively skewed with kurtosis values that are closer to 3. The EPU growth series appear to be normally distributed in almost all the countries.
Table 1. Descriptive statistics
5. Results
The results for the usual in-sample F test, the standard post-sample MSE-F and CSV tests using full sample are presented in Table . The p-values of each test for the null hypothesis that EPU does not granger cause real housing returns are reported for each of the eight emerging countries. For the cross-validation test, only the third quartile or CSV75 test p-values are reported as this is consistent with suggestions by Ashley and Tsang (Citation2014) that this should be used in empirical applications given their power over the class of CSV tests. In all cases, the p-values were obtained via bootstrapping with M = 10,000 simulations. As determined by the SIC lag length criteria, two lags of real housing returns were included as explanatory variable for South Africa and Ireland while one lag was included for the remaining countries. Looking at the in-sample test result, it is observed that the null hypothesis can be rejected only for China at 5% level of significance. This implies that EPU Granger causes housing returns in China. Put in another words, EPU has an in-sample predictive power of housing returns in China. For the rest of the countries, EPU growth does not Granger cause housing returns. This implies that previous values of EPU do not have an in-sample predictive power for housing returns in these countries.
Table 2. In-sample, CSV and post-sample Granger causality tests
Moving to the CSV75 full sample or time invariant test result, the null hypothesis is rejected at 5% for Chile and China only. Again, this implies that based on the CSV75 time invariant test, China and Chile’s EPU past values can be used to predict the current value of housing returns above that which is predicted by the lagged value of housing returns in these two countries. While the CSV test supports the in-sample tests for China, and the rest of the countries, there is mixed evidence for Chile. The predictive power of EPU for China and Chile’s housing markets based on the CSV approach is however out-of-sample. The rejection p-values for the standard MSE-F tests are reported for post-sample periods of lengths 5 and 10 quarters. The null hypothesis is rejected for India, Russia and South Africa for the 5 quarter-ahead while it is not rejected for any of the countries for the 10- quarter ahead. Given the shortness of the sample data, the results of the standard post-sample MSE-F test should be interpreted with caution. This is where perhaps the advantage of the bootstrapping technique employed in the CSV approach becomes important.
Aside the results based on the full sample, this paper also presents the results from the rolling window CSV test with the rolling window size set to 20. These are presented for the various countries in Figures –. These figures graph the bootstrapped p-values for the CSV75 test as a function of the forecast windows; the horizontal line represents a p-value of 0.10 (10% significance level). Figure presents the CSV75 p-values for the null hypothesis that EPU growth does not Granger cause real housing returns in Brazil out-of-sample. The null hypothesis is rejected for Brazil in 2013Q3 only. This means that EPU has a very limited out-of-sample predictive power for housing returns in Brazil. In Figure , the null hypothesis is rejected for Chile during 2002Q4–2003Q3, 2007Q1, 2008Q4, 2009Q2–2011Q4 sub-periods. Hence, during these periods, Chile’s EPU has significant out-of-sample predictive ability for its real housing returns. These periods fall much within the recent global crisis and appear to connote that Chile’s housing market must have been seriously affected by high economic uncertainty during and after the crisis. For China as presented in Figure , EPU has significant causality for real housing returns in 2005Q1–2005Q2, 2005Q4 and 2011Q4–2015Q3 sub-periods. In Figure , the results show that there is clearly no causal relationship between EPU and real housing returns in India. Therefore, the null hypothesis of no Granger causality cannot be rejected for India based on the CSV rolling window approach.
Figure 2. The cross-sample validation Granger causality test for different rolling windows for Brazil.
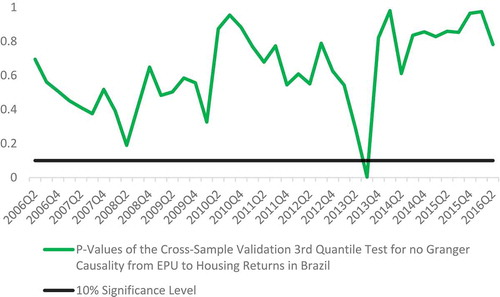
Figure 3. The cross-sample validation Granger causality test for different rolling windows for Chile.
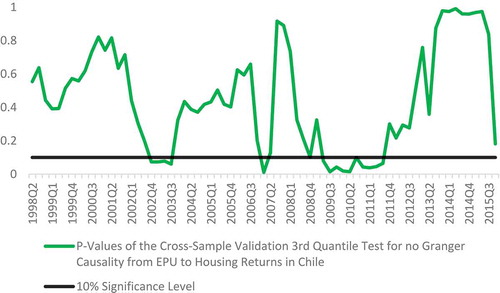
Figure 4. The cross-sample validation Granger causality test for different rolling windows for China.
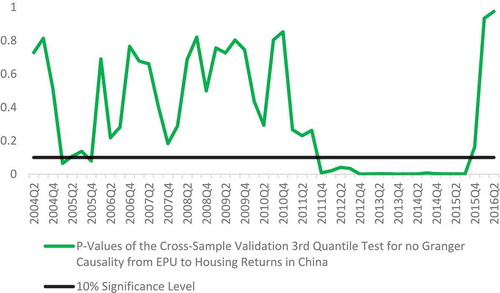
Figure 5. The cross-sample validation Granger causality test for different rolling windows for India.
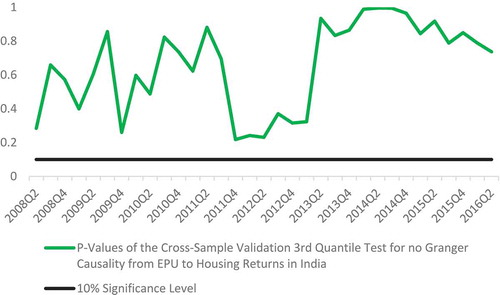
Figure 6. The cross-sample validation Granger causality test for different rolling windows for Ireland.
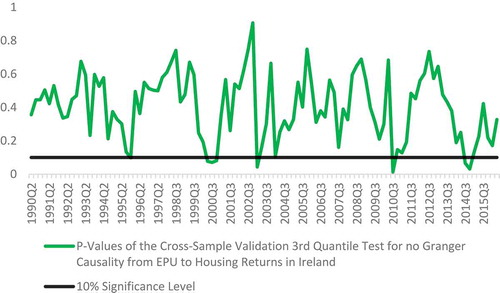
Figure 7. The cross-sample validation Granger causality test for different rolling windows for Russia.
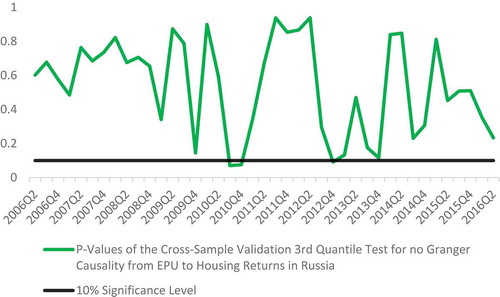
Figure 8. The cross-sample validation Granger causality test for different rolling windows for South Africa.
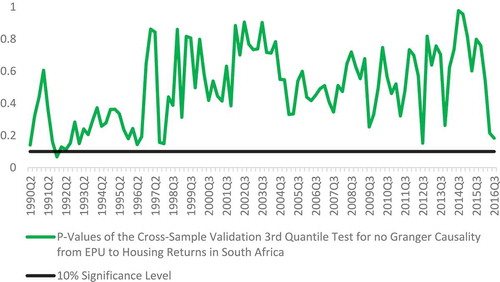
Figure 9. The cross-sample validation Granger causality test for different rolling windows for South Korea.
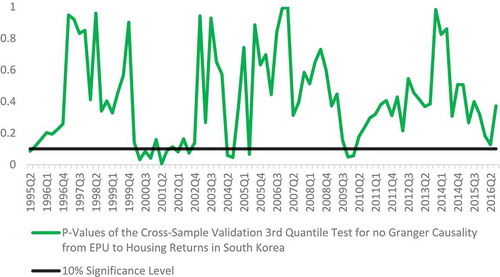
In Ireland as depicted in Figure , there seems to be some significant causal relationship between EPU and real housing returns at different periods but these do not seem to span for long. Specifically, there is evidence of EPU causing real housing return in the following periods: 1995Q4, 2002Q2–2002Q4, 2003Q1, 2010Q3 and 2014Q3–2014Q4. It can therefore be concluded that for these periods EPU has out-of-sample predictive power for housing returns in Ireland. In Figure , the results show that during 2010Q3–2010Q4 and 2012Q4, EPU has an out-of-sample predictive power for real housing returns in Russia. Therefore, we cannot reject the null hypothesis of non-Granger causality of EPU in Russia for these periods. Results based on Figure show that in South Africa, EPU has basically no predictive power for the housing market as its significance is felt only in 1991Q4. Finally for South Korea as shown in Figure 9, there is evidence of predictive power of EPU for real housing returns during 2000Q2–2000Q4, 2001Q2–2001Q3, 2002Q1, 2002Q3, 2004Q2–2004Q3, 2005Q2, 2009Q4–2010Q1 periods.
Overall, we find evidence of time-varying causality from EPU to housing returns in all countries except India whereas the full-sample analysis detects causality for only Chile and China. The findings are consistent with majority of the literature such as Chow et al. (Citation2017), Christou et al. (Citation2017) and André et al. (Citation2017) that find EPU as a causing variable for the housing market. However, it contrasts that of Su et al. (Citation2016) for Germany. This perhaps justifies country-specific analysis of these relationships.
As a robustness check, the Nishiyama, Hitomi, Kawasaki, and Jeong (Citation2011) k-order non-parametric test used in André et al. (Citation2017) is also used. The results are presented in Table . The null hypothesis of non-Granger causality in the mean (real housing return) is rejected for all the countries except Chile, India and Russia while it is not rejected in the second moment (real housing return volatility) for only Chile and India. In other words, EPU has predictive power for the mean housing returns in 5 out of 8 emerging countries studied. Similarly EPU has predictive ability for housing return volatility in 6 out of 8 countries. It can be concluded that when the assumption of linearity is relaxed, the dominant role of for real housing returns becomes very clear and this is robust to results from an alternative approach.
Table 3. Non-parametric Granger causality test
6. Conclusion
This study investigates the causal relationship between EPU and real housing returns for 8 emerging markets using the CSV causality test. The countries are Brazil, Chile, China, India, Ireland, Russia, South Africa and South Korea. Results show that EPU growth has predictive ability for Chile and China based on the full sample CSV test. However, with the rolling window CSV test, all countries barring India indicate evidence of EPU Granger causing real housing returns. The dominant role of EPU for real housing returns becomes very clear when the assumption of linearity is relaxed, and this is robust to results from an alternative approach. These findings have important implications. From a policy perspective, this results show that high EPU can weaken the impact of economic policies and hence calls for the respective policy makers to consciously seek for strategies for reducing uncertainty in these economies. The need for timely policy initiatives cannot be overstressed. Appropriate balance between fiscal adjustment and policy measures should be sought to reduce vulnerabilities. From investors’ perspective, heightened uncertainty may weigh on confidence, thereby restraining household and business spending. High policy uncertainty affects returns from real estate investment. This may lead to delayed business decisions such as delaying business expansion plans, putting off investment decisions which may stall company growth and considerations to invest offshore in perhaps more stable business environments. It also has implications for portfolio repositioning as every rational investor would want to reduce his/her holdings in unsafe stocks. Since heightened uncertainty about policy direction leads to weak investment growth in the housing sector, this will consequently affect economic growth given that housing has been shown to be a leading indicator. From academic perspective, the CSV test is feasible and provides credibility for post-sample testing when the sample data is scarce since the test does not depend on the decision on in-sample/post-sample split. Although, the countries studied are diverse in terms of geographical location, macroeconomic scenario and business environment, our results provide evidence that EPU affects the sustenance of the housing sector irrespective of these differences. It is therefore imperative that the development of the housing sector be paramount in any policy or regulation in all economies since housing markets play a key role in understanding regional evolutions and regional differentials in economic activity and living standards.
Additional information
Funding
Notes on contributors
Goodness C. Aye
Dr. Goodness C. Aye holds a PhD in Agricultural Economics from University of Pretoria. She obtained her Bachelors and Masters Degrees in the same field. She is a Senior Lecturer and Researcher with interest in agricultural and economic policy analysis, financial markets, development, energy and environmental economics as well as applied econometrics. She has over 100 publications covering these areas. She has presented papers in conferences in many continents of the world. She is a reviewer and editor for high ranking local and international journals. She teaches and supervises both undergraduate and graduate students. Above all, she is married and blessed with children whose love has enabled her to succeed in her career. The current research on the role of economic policy uncertainty on real housing returns in emerging economies demonstrates her quest for stable and effective fiscal, monetary and regulatory policies that would foster economic growth and welfare.
Notes
1. Please see Balcilar, Gupta, Kyei, and Wohar (Citation2016a) for a review of the relationship between uncertainty and other economic and financial variables.
2. More technical details can be found in Ashley and Tsang (Citation2014).
3. However, it is noted that when the underlying variables in levels are cointegrated, the model in first differences is mis-specified unless it allows for error-correction which then permits a long-run analysis. Therefore, the Johansen-based contegration is performed. Results as shown in the Appendix A indicate absence of cointegration at 5% level Therefore, the model with stationary series is not mis-specified.
References
- Ajmi, A. H., Babalos, V., Economou, F., & Gupta, R. (2014). Real estate market and uncertainty shocks: A novel variance causality approach. Frontiers in Finance and Economics, 2(2), 56–85.
- André, C., Bonga-Bonga, L., Gupta, R., & Mwamba, J. W. M. (2017). Economic policy uncertainty, US real housing returns and their volatility: A nonparametric approach. Journal of Real Estate Research, 39(4), 493–513.
- Antonakakis, N., André, C., & Gupta, R. (2016). Dynamic spillovers in the United States: Stock market, housing, uncertainty and the macroeconomy. Southern Economic Journal, 83(2), 609–624. doi:10.1002/soej.12149
- Antonakakis, N., Gupta, R., & André, C. (2015). Dynamic co-movements between economic policy uncertainty and housing market returns. Journal of Real Estate Portfolio Management, 21(1), 53–60.
- Ashley, R., Granger, C., & Schmalensee, R. (1980). Advertising and aggregate consumption: An analysis of causality. Econometrica, 48(5), 1149–1167. doi:10.2307/1912176
- Ashley, R. A., & Tsang, K. P. (2014). Credible Granger-causality inference with modest sample lengths: A cross-sample validation approach. Econometrics, 2, 72–91. doi:10.3390/econometrics2010072
- Aye, G. C., Balcilar, M., Bosch, A., & Gupta, R. (2014). Housing and the business cycle in South Africa. Journal of Policy Modelling, 36(3), 471–491. doi:10.1016/j.jpolmod.2014.03.001
- Aye, G. C., Clance, M. W., & Gupta, R. (2017). The effect of economic uncertainty on the housing market cycle (Working Paper No: 2017-57). Pretoria: Department of Economics, University of Pretoria.
- Aye, G. C., Deale, F. W., & Gupta, R. (2016). Does debt ceiling and government shutdown help in forecasting the US equity risk premium? Panoeconomicus, 63(3), 273–291. doi:10.2298/PAN1603273A
- Azzimonti, M. (2018). Partisan conflict and private investment. Journal of Monetary Economics, 93(2018), 114–131. doi:10.1016/j.jmoneco.2017.10.007
- Baker, S., Bloom, N., & Davis, S. (2016). Measuring economic policy uncertainty. The Quarterly Journal of Economics, 131, 1593–1636. doi:10.1093/qje/qjw024
- Balcilar, M., Gupta, R., Kyei, C., & Wohar, M. E. (2016a). Does economic policy uncertainty predict exchange rate returns and volatility? Evidence from a nonparametric causality-in-quantiles test. Open Economies Review, 27(2), 229–250. doi:10.1007/s11079-016-9388-x
- Balcilar, M., Gupta, R., & Segnon, M. (2016b). The role of economic policy uncertainty in predicting U.S. recessions: A mixed-frequency Markov-switching vector autoregressive approach. The Open-Access, Open-Assessment E-Journal, 10, 1–20.
- Balcilar, M., Ozdemir, Z. A., & Arslanturk, Y. (2010). Economic growth and energy consumption causal nexus viewed through a bootstrap rolling window. Energy Economics, 32(6), 1398–1410. doi:10.1016/j.eneco.2010.05.015
- Barros, C. P., Gil-Alana, L. A., & Payne, J. E. (2015). Modeling the long memory behavior in U.S. housing price volatility. Journal of Housing Research, 24(1), 87–106.
- Burnside, C., Eichenbaum, M., & Rebelo, S. (2016). Understanding booms and busts in housing markets. Journal of Political Economy, 124(4), 1088–1147. doi:10.1086/686732
- Calcagnini, G., & Saltari, E. (2000). Real and financial uncertainty and investment decisions. Journal of Macroeconomics, 22(3), 491–514. doi:10.1016/S0164-0704(00)00142-7
- Campbell, J. Y., & Thompson, S. B. (2008). Predicting excess stock returns out of sample: Can anything beat the historical average? Review of Financial Studies, 21(4), 1509–1531. doi:10.1093/rfs/hhm055
- Cesa-Bianchi, A., Cespedes, L. F., & Rebucci, A. (2015). Global liquidity, house prices, and the macroeconomy: Evidence from advanced and emerging economies. Journal of Money, Credit and Banking, 47(S1), 301–335. doi:10.1111/jmcb.2015.47.issue-S1
- Chow, S.-C., Cunado, J., Gupta, R., & Wong, W.-K. (2017). Causal relationships between economic policy uncertainty and housing market returns in China and India: Evidence from linear and nonlinear panel and time series models. Studies in Nonlinear Dynamics & Econometrics, 22. doi:10.1515/snde-2016-0121
- Christou, C., Gupta, R., & Hassapis, C. (2017). Does economic policy uncertainty forecast real housing returns in a panel of OECD countries? A Bayesian approach. The Quarterly Review of Economics and Finance, 65, 50–60. doi:10.1016/j.qref.2017.01.002
- Cunningham, C. R. (2006). House price uncertainty, timing of development, and vacant land prices: Evidence for real options in Seattle. Journal of Urban Economics, 59(1), 1–31. doi:10.1016/j.jue.2005.08.003
- Dickey, D. A., & Fuller, W. A. (1979). Distribution of the estimators for autoregressive time series with a unit root. Journal of the American Statistical Association, 74, 427–431.
- El Montasser, G., Ajmi, A. N., Chang, T., Simo-Kengne, B. D., Andre, C., & Gupta, R. (2016). Cross-country evidence on the causal relationship between policy uncertainty and house prices. Journal of Housing Research, 25(2), 195–211.
- Fernández-Villaverde, J., Guerrón-Quintana, P., Kuester, K., & Rubio-Ramírez, J. F. (2015). Fiscal volatility shocks and economic activity. American Economic Review, 105(11), 3352–3384. doi:10.1257/aer.20121236
- Hafner, C. M., & Herwartz, H. (2006). Volatility impulse responses for multivariate GARCH models: An exchange rate illustration. Journal of International Money and Finance, 25, 719–740. doi:10.1016/j.jimonfin.2006.04.006
- Hansen, P. R., & Timmermann, A. (2012). Choice of sample split in out-of-sample forecast evaluation (Economics Working Papers ECO2012/10). Florence: European University Institute.
- Hirata, H., Kose, M. A., Otrok, C., & Terrones, M. E. (2013). Global house price fluctuations: Synchronization and determinants. NBER International Seminar on Macroeconomics, University of Chicago Press, 9(1), 119–166. doi:10.1086/669585
- Jurado, K., Ludvigson, S., & Ng, S. (2015, March). Measuring uncertainty. American Economic Review, 105(3), 1177–1216. doi:10.1257/aer.20131193
- Kyriazakou, E., & Panagiotidis, T. (2017). Causality analysis of the Canadian city house price indices: A cross-sample validation approach. The Journal of Economic Asymmetries, 16, 42–52. doi:10.1016/j.jeca.2017.06.001
- Leamer, E. E. (2015). Housing really is the business cycle: What survives the lessons of 2008–09? Journal of Money, Credit and Banking, 47(1, supplementary), 43-50. doi.org/10.1111/jmcb.12189.
- Li, X.-L., Balcilar, M., Gupta, R., & Chang, T. (2016). The causal relationship between economic policy uncertainty and stock returns in China and India: Evidence from a bootstrap rolling-window approach. Emerging Markets Finance and Trade, 52(3), 674–689. doi:10.1080/1540496X.2014.998564
- Muellbauer, J., & Murphy, A. (2008). Housing markets and the economy: The assessment Oxford review of economic policy (Vol. 24, pp. 1–33). Oxford: Oxford University Press.
- Ng, S., & Perron, P. (2001). Lag length selection and the construction of unit root tests with good size and power. Econometrica, 69, 1519–1554. doi:10.1111/ecta.2001.69.issue-6
- Nishiyama, Y., Hitomi, K., Kawasaki, Y., & Jeong, K. (2011). A consistent nonparametric test for nonlinear causality—Specification in time series regression. Journal of Econometrics, 165, 112–127. doi:10.1016/j.jeconom.2011.05.010
- Pástor, Ľ., & Veronesi, P. (2013). Political uncertainty and risk premia. Journal of Financial Economics, 110(3), 520–545. doi:10.1016/j.jfineco.2013.08.007
- Phillips, P.C.B, & Perron, P. (1988). Testing for a unit root in time series regression. Biometrika, 75(2), 335-346. doi: 10.2307/2336182
- Plakandaras, V., Gupta, R., Gogas, P., & Papadimitriou, T. (2017). Macroeconomic uncertainty, growth and inflation in the eurozone: A causal approach. Applied Economics Letters. doi:10.1080/13504851.2017.1391996
- Rafiq, S, Salim, R, & Bloch, H. (2009). Impact of crude oil price volatility on economic activities: an empirical investigation in the Thai economy. Resources Policy, 34, 121–132. doi.org/10.1016/j.resourpol.2008.09.001
- Rapach, D., & Zhou, G. (2013). Forecasting stock returns, David E. Rapach and Guofu Zhou. In G. Elliott & A. Timmermann (Eds.), Handbook of economic forecasting (Vol. 2A, pp. 328–383). Amsterdam: Elsevier.
- Redl, C. (2015). Macroeconomic uncertainty in South Africa (ERSA Working Paper 509). Cape Town: Economic Research Southern Africa.
- Rossi, B., & Sekhposyan, T. (2015). Macroeconomic uncertainty indices based on nowcast and forecast error distributions. American Economic Review, 105(5), 650–655. doi:10.1257/aer.p20151124
- Seni, G., & Elder, J. F. (2010). Ensemble methods in data mining: Improving accuracy through combining predictions. Synthesis Lectures on Data Mining and Knowledge Discovery, 2(1), 1–126. doi:10.2200/S00240ED1V01Y200912DMK002
- Shoag, D., & Veuger, S. (2016). Uncertainty and the geography of the great recession. Journal of Monetary Economics, 84, 84–93. doi:10.1016/j.jmoneco.2016.11.002
- Su, D., Li, X., Lobonţ, O.-R., & Zhao, Y. (2016). Economic policy uncertainty and housing returns in Germany: Evidence from a bootstrap rolling window. Zbornik Radova Ekonomskog Fakulteta u Rijeci / Proceedings of Rijeka School of Economics, 34(1), 43–61.
- Sum, V., & Brown, K. (2012). Real estate sector response to economic policy uncertainty shocks. International Research Journal of Applied Finance, 3(12), 1739–1747.
- Tashman, L. J. (2000). Out-of-sample tests of forecasting accuracy: An analysis and review. International Journal of Forecasting, 16, 437–450. doi:10.1016/S0169-2070(00)00065-0
- Welch, I., & Goyal, A. (2008). A comprehensive look at the empirical performance of equity premium prediction. Review of Financial Studies, 21(4), 1455–1508. doi:10.1093/rfs/hhm014
- Zivot, E., & Andrews, D. W. K. (1992). Further evidence on the great crash, the oil-price shock, and the unit-root hypothesis. Journal of Business & Economic Statistics, 10, 251–270.
Appendix A
Table A1. Unit root tests with and without breakpoint
Table A2. Cointegration test