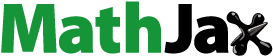
Abstract
This study is the first attempt to scrutinize the causal relationship between economic policy uncertainty (EPU) and innovation in the case of China, using the autoregressive distributed lag (ARDL) approach to co-integration approach of innovation accounting for causality analysis. The empirical findings show that EPU can negatively affect innovation. EPU indicates a significantly negative impact on innovation as well as on the gross domestic product (GDP) growth rate. The combined results based on ARDL, innovation accounting approach (IAA) (variance decompositions and impulse response functions), and fully modified ordinary least square (FMOLS) raise an important point that calls for attention. The point is relating to the causality running from EPU to innovation. The future of China is uncertain, so when the economic uncertainty is higher, it lowers the value of future activities of the economy of China.
PUBLIC INTEREST STATEMENT
This study is the first attempt to scrutinize the casual relationship between EPU and innovation in the case of China. Economic reforms of China after late 1978 have taken five different economic development phases. It is widespread incomplete legal, government regulating rules and market rules are the main characteristics of Chinese market. The incomplete market economy and its disposable resources of China are under the control of government. China is a transitional economy; different firms have its political connections and relationship between executives of firms and local government officials, thus high political uncertainty leads to less investment. This study observed that the future of China is uncertain; as the economic uncertainty is higher it lowers the value of future economic activities.
1. Introduction
Can innovation be affected by economic policy uncertainty (EPU) in China? In this study, we approach this main question by investigating the impact of Chinese EPU on the innovations. The significance of innovation in accelerating a sustainable economic growth and competitiveness of the nation has been properly acknowledged (Solow, Citation1957). Various empirical analysis by Bernstein (Citation2015); Dong and Gou (Citation2010); Faleye, Kovacs, and Venkateswaran (Citation2014); Lin, Lin, and Song (Citation2010); Lin, Lin, Song, and Li (Citation2011) estimates the correlation between market-based characteristics and firm innovation. However, few empirical analyses have discussed the influence of uncertainty on innovation and investment. Numerous empirical studies have discussed about the innovation and market-specific characteristics and examined how different institutional factors like political stability or instability determine technological innovations that are sparse. Political situation of the economy is also important because economic policies are determined by politics that can frequently change the economic condition in which innovative firms work, which ultimately impact an economy’s innovation growth. According to Global Innovation Index (Citation2013) Edition (Dutta and Lanvin, Citation2013), the two main indicators of this index are government effectiveness and political instability under the category of political environment. A total application of patent and economic policy uncertainty is inversely related to each other. The Figure shows the inverse relationship between innovation (total number of patents) and economic policy uncertainty.
Economic stability is highly correlated with internal and external economic balances explained by Keynesian school of economic thought. Over the last few decades, the important changes in economic policies and structures created economic uncertainty that decreases the investment and resource allocations, which create the external and internal imbalances. These imbalances are highly associated with the distortion of investment plans and directly affect the economic growth also (Ocampo, Citation2005).
In this study, we try to add our literature in existing empirical literature by properly examining the real effects of the political economic situation on innovation. Does policy uncertainty affect technological innovation in the mainland of China? Based on existing literature, in this study, we develop a hypothesis regarding the relative importance of policy uncertainty in determining innovation.
The hypothesis of policy uncertainty states that it is EPU that affects innovation. Various empirical analyses (e.g. prior study of Bernanke, Citation1983; than models of Chen and Funke (Citation2003)., Bloom, Bond, and Reenen (Citation2007)., and Bloom, Bond, and Reenen (Citation2016)) demonstrate that if different projects of investment are not profitable and reversible, then investment by firms hold back, and due to the uncertain economic condition, uncertainty enhances the value of the option to wait. Investment in R&D is highly affected by uncertainty and highly based on the value of option to wait; the innovation is discovering the new ideas, unknown approaches and novel methods (see Holmstrom, Citation1989; also Aghion & Tirole, Citation1994) that need significant investment especially in intangible assets. Political situation for innovation is particularly important because the value of the option to wait is also related to innovation as discussed before; the chance of success in exploration of innovation also depends on political leadership and their policies. The significance of the option to wait is associated with political uncertainty about which the government will be in power. Hence the hypothesis of policy uncertainty we develop argues that it is EPU that affects innovation.
This paper incorporates main contributions to the existing literature on the correlation between EPU and innovation. This study provides statistical evidence that the Chinese political system is a significant source of uncertainty and also related to innovational decision-making by policies. Our main variable proxy of uncertainty is established by Baker, Bloom, and Davis (Citation2012) as the uncertainty index of economic policy. Numerous studies mostly used EPU by Baker et al. (Citation2012); this is an appropriate proxy for real-world EPU (Wang, Chen, & Huang, Citation2014). Various recent empirical analyses used national election as a main indicator of time when a high level of political and economic uncertainty was found in a cross-country analysis. This proxy of uncertainty only captures election years that may be important in some countries but not in all. When studying the country-level cause of EPU on investment and innovation decision, the election years as a proxy of policy uncertainty strongly bias inferences. The study of Julio and Yook (Citation2012) used election year as dummy finds that there is no relation between EPU and corporate investment in the USA, which shows that investment is not significantly lower in the period of election versus non-election years in the USA. So, we agree that index of policy uncertainty of Baker et al. (Citation2012) is a significant measure of the policy uncertainty in the economy that shows substantial variation overtime (Gulen & Ion, Citation2015).
Numerous theoretical literature pays attention on the major effect of uncertainty on investment. However empirical literature also finds relationship between uncertainty and investment (Gulen & Ion, Citation2015; Julio & Yook, Citation2012; Wang et al., Citation2014). Based on Chinese cities data, An, Chen, Luo, and Zhang (Citation2016) check the effect of EPU on corporate investment and conclude that corporate investment is inversely related to political turnover. The relationship between market uncertainty and corporate R&D investment is examined by Czarnitzki and Toole (Citation2007, Citation2011), but according to my knowledge, there is no empirical study which discussed innovation and EPU relationship focus on China at macro-level and based on time series data. This study contributes to the existing literature by empirically evaluating the principal effect of policy uncertainty on innovation with controlling the effect of economic growth.
1.1. Theory and background
Chinese law of patent was established in 1985, the growth rate of patent application was reasonable until the end of the 1990s. But these applications have rushed dramatically since 2000. Domestic investors and their applications in particular increased at an annual rate of 30% from 1999 to 2009 (Chinese patent data from SIPO).
The real option theory explains that current investment level of the economy can be affected by uncertainty because higher value of the information achieved by waiting leads to reduced investment (see also Bernanke, Citation1983; Bloom et al., Citation2007; Dixit, Citation1989). Mostly investment related to R&D includes equipment purchases, employee remuneration, material expenses etc. These are mostly affected by the uncertain economic condition (Dixit & Pindyck, Citation1994). According to real option theory of investment, market uncertainty is not under consideration in this study, we try to investigate the influence of EPU on innovation and take time series data related to China. Similarly. strong association can be seen between corporate behavior and governments in emerging market economies (Shleifer & Vishny, Citation1994).
China faced most economic intervention by its government among all transitional market economies (see Fan, Huang, & Zhu, Citation2013). State-owned sectors, assets and economic activity in China are also affected by government policies (Chen, Sun, Tang, & Wu, Citation2011). Rapid growth of the Chinese economy in the past decade, but China as an emerging economy facing high EPU and still going far from a successful planned economy to a market-based economy (Wang et al., Citation2014). The central, provincial, county and city government prepares social and economic growth and development plans every 5 years to achieve the future industrial development targets. So innovation is also related to long-term investment, which can be significantly affected by long-term policies (monetary policy, fiscal policy and industrial policy) of government. So, EPU also plays an important role in the promotion of innovation (Wang et al., Citation2014).
The Chinese government launched patent subsidy program at the end of the 1990s to increase the local industries’ technological competitiveness after they became a WTO member. To encourage domestic firms in terms of “endogenous innovation,” and strengthen the awareness of intellectual property rights, the central government launched policy instructions named “Strengthen Technology Innovation, Develop High-Tech Industries, and Promote Industrialization (related to Inventions).” Although the policies and goal of the government are the same, the policy design is not same across regions and many governments have made significant revisions to their policies (Dang & Motohashi, Citation2015). The uncertain political conditions affect firms and investors because they are not sure about future economic policies by the government and firms have no idea about which sectors will be subsidies, developed by government and which can be imperfectly affected by government policies. The association between uncertainty and impact on the economy is an important query of long-standing anxiety to economists. Bernanke (Citation1983) and Dixit (Citation1989) investigated the connection between the real implications of uncertainty and its effects on the real economy (Handley, Citation2014) also explored impact on investment opportunities. The future of China is uncertain due to political instability, so when the economic uncertainty is higher it lowers the value of future activities of the economy of China.
The rest of the study is structured as follows. The second section summarizes the review of literature and the third section related to the empirical analysis and description of the variables. Fourth section describes the empirical results and finally draws conclusions from the analytical results.
2. Literature review
Various prior studies have discussed about uncertainty associated with political decisions, and these policies can significantly enhance the future uncertainty and also related to firm’s future profitability. These theoretical analyses have recommended mechanisms through which higher uncertain profits can reduce the level of investment. The first theory presented by Bernanke (Citation1983) explained the cyclical investment movements in terms of the irreversible choice theory under uncertainty, and Rodrik (Citation1991) also describes the relationship between uncertainty and about investment projects that cannot be usually reversible because uncertainty will raise the value of the option to wait until further knowledge about the profitability of the investments projects is revealed. Followed by this theory, many others have applied Bloom et al. (Citation2007), Bloom et al. (Citation2016); Chen and Funke (Citation2003) models to investigate the inverse relationship between uncertainty and investment projects. Innovation is a not a short-term process; it takes time by exploring different technology, methods of completion and implementation. Dixit and Pindyck (Citation1994) explained that EPU uplifting the value of the postponing opportunity of so many projects of the investment will be postponed, portraying a negative correlation between investment and uncertainty.
Bhattacharya, Hsu, and Tian, Xu (Citation2015) and studies of Gulen and Ion (Citation2015); Julio and Yook (Citation2012) investigate the significant inverse relationship between policy uncertainty and intangible investment. Economic activity especially in developing countries is affected by governments in terms of firm’s performance, shares, values and choices of firms (Firth, Gong Stephen, & Shan, Citation2013). Government has played a significant role in economic activity especially making industrial policy in the Republic of China. In case of uncertain political environment, the changed firms and investors are not sure about future economic policies by the government. Uncertainty of future policies is related to the industrial sector where firms have no idea about which sectors will be subsidies, developed by government and which can be badly affected by government policies. In this situation, when economy faces the problem of policy uncertainty, then the value of the option to wait to invest in R&D will be suffered. In the condition of innovation, irreversible investment mostly in intangible goods and assets and the value of R&D strongly depends on the government policies (Bhattacharya et al., Citation2015). So, growing EPU is negatively responding to firms to wait to make R&D investments.
Adner and Levinthal (Citation2004) and Dixit and Pindyck (Citation1994) examined that the decision of investment under uncertainty is discussed by real option approach and used this as a tool of decision analysis. Real option theory was criticized by many economists because of its limited use as a tool in the setting of originations Bowman and Moskowitz (Citation2001) also discussed by (Kogut & Kulatilaka, Citation2004). The real options theory proposes a reasoning logic which is related to description of uncertainty, flexibility and irreversibility inherent in decisions of innovation investment so as a strategic heuristic in decisions of organizational innovation, the logic of real option was usually applied (see Adner & Levinthal, Citation2004; Kogut (Citation2001).; also McGrath & Nerkar, Citation2004). McGrath (Citation1997) and McGrath and Nerkar (Citation2004) explained that various studies used the real options logic as an analytical framework for firms as “innovation investment, R&D investments” decisions where investment is related to technology innovation; these frame work of research are measured as strategic actions and flexibility in terms of amount and timing of investment by firms.
Economic reforms in China after late 1978 have taken five different economic development phases. The history of the market economy of western economies is more than 100 years old as compared to the Chinese market economy and widespread incomplete legal, government regulating rules et al and, market rules are the main characteristics of Chinese market. Usually, intervention of government mostly present in the market tries to make a transparent and fair market environment (Chen et al., Citation2011; Fan, Wong, & Zhang, Citation2007). The incomplete market economy and its disposable resources of China are currently under the control of government. China is the transitional economy; different firms have its political connection and relationship between executives of firms and local government officials (Fan et al., Citation2007).
High political uncertainty leads to less investment; so when firms are politically connected, the advantages of political connection may not be beneficial and An et al. (Citation2016) highlighted that politically related firms are badly affected by turnover of political parties. Consequently, the unrest political environment is significantly adverse effects of policy uncertainty on R&D investment. As empirical evidence based on EPU (EPU), Baker et al. (Citation2012) formulate an EPU index including various indicators like the frequency of newspaper references to EPU. Statistics of index of EPU indicate that uncertainty adversely effects on hiring and investment especially for different firms heavily dependent on government contracts. Based on macro-level analysis, they find that using vector autoregression (VAR) models, the EPU is a main indicator of declines in output, employment and investment. There is a considerable amount of time-varying volatility in tax and government spending as a share of output processes in the U.S. Fiscal volatility shocks reduces economic activity: aggregate output, consumption and investment. Most of the effect works through larger uncertainty about future tax rates on capital income. By taking fiscal volatility shock as proxy of uncertainty in their analysis influence inversely on economic activity in terms of reduction of investment, consumption, production and hours worked. EPU is negatively related to the firms and industrial investment in the USA examined by Gulen and Ion (Citation2015) in their analysis and also described that 32% drop in corporate investments examined during the period of 2007 to 2009 crisis can be attributed to economic policy associated with uncertainty.
3. Theory and econometric specification
The theoretical foundation for investment under the economic uncertainty is highlighted by the good news and bad news principle of the economy emphasized in the literature of Oi (Citation1961), Hartman (Citation1972) and also Abel (Citation1983), who explain the fact that firms and investors can increase to exploit good news and agreement to cover against bad news related to economy, making them potentially risk-seeking in an uncertain condition of the economy.
Recent empirical analysis in economics has revealed that economic political uncertainty has negative real effects on the economic activities. According to the theory of real options of investment irreversibility, it explains the basic argument is that economic uncertainty is inversely related to capital investment by increasing the value of being expected to invest. The adverse association found between various measures of EPU and investment has guided researchers to find that policymakers should be aware of the harmful effects that lengthy debates about policy may indict on the economy.
Usually EPU increases the value of the postponing option, so many projects of the investment will be postponed, depicting to an adverse investment and uncertainty relationship (McDonald and Siegel (Citation1986), Dixit and Pindyck (Citation1994)). The innovations are adversely affected by uncertainties and are more severe and problematic than on other types of investment. The real option theory stresses that partial irreversibility and adjustment costs may cause investors to defer innovation-based investment in the case of heightened uncertainty. Another way through which uncertainty potentially encourages research and development is discussed in the literature of Bloom and Van Reenen (Citation2002), who examine patents as options.
China is politically centralized country, so political instability can put inverse effects on innovation activities. Barro (Citation1991) and then Alesina and Perotti (Citation1996) explain that political unrest and government instability are significantly related with cross-country differences in investment and economic growth. An exploration of empirical literature depicted that there is limited empirical research on this topic respect to China. As, this study is interested in investigating the influence of EPU on innovations related to the Chinese economy, thus, it is based on the theoretical framework, primarily the following econometric model specification will be estimated. Our model specification follows the empirical equation of Gulen and Ion (Citation2015). Following equation shows the general empirical relation of innovation and uncertainty, with subscripts t, denoting a time series data analysis during different time period (t).
where log natural of innovation (gross domestic product (GDP) (
and et = error term.
4. Methodology and data
4.1. Construction of variables
This study uses three variables for analysis, namely, innovation, EPU and annual growth rate of GDP, which is used as control variable. The main objective of this paper is to investigate the influence of EPU on innovation in mainland of China applying time series data from 1995 to 2015 (according to data availability of uncertainty index from 1995).
4.1.1. Innovation
The data of patent are used to the proxy innovation so this study uses the number of patent applications (per million inhabitants) of China (Source: Procuratorial Yearbook of China and data is also available on the website of SIPO ((http://www.sipo.gov.cn/)). Due to limitation of data based on innovation as explained by (Keller, Citation2004), the patent data are the proxy of innovation mostly used. So this proxy has become more standard in the literature of innovation (like Audretsch and Fedman (Citation2004), Acharya and Subramanian (Citation2009), Bloom, Draca, and Van Reenen (Citation2011)), also explained by Nanda and Rhodes Kropf (Citation2013), similarly Hsu, Tian, and Xu (Citation2014).
Dang and Motohashi (Citation2015) also found that patent statistics is good and meaningful indicator in China.
4.1.2. Economic policy uncertainty
The main challenge of this research is solving the problem of an appropriate measure of EPU. Most studies are based on firm’s related policy uncertainty especially in China based on Chinese listed firms and their volatility of stock returns, input and output prices, related to their production, investment related uncertainty and dispersion in analyst forecasts. Prior studies mostly used EPU by Baker et al. (Citation2012); this is an appropriate proxy for real-world EPU (Wang et al., Citation2014). The variable of uncertainty is treating as exogenous interaction variable Aastveit et al (Citation2013).The uncertainty index of economic policy is established by Baker et al. (Citation2012). This uncertainty index includes some important economic aspects that are the frequency of newspaper related to uncertainty of economic policy, the numeral federal tax code provisions set to expire, and the degree of economic forecaster disagreement over future inflation rate and government purchases. Different studies related to Bloom (Citation2009)., Alexopoulos & Cohen (Citation2009) also explained by Fernandez-Villaverde et al. (Citation2013), Baker et al. (Citation2013), see Mumtaz and Surico (Citation2013), similarly by Caggiano et al. (Citation2014), Benati (Citation2014) and Nodari (Citation2014) along with various others explained that economic policies can put negative effect on employment, investment, industrial production and consumption. The index of EPU established by Baker et al. (Citation2015) also has small effects especially on various economic activity when comparatively compared with various macroeconomic measures of uncertainty by Jurado et al. (Citation2015). So in this study, the EPU index is used (taking averages of monthly data for making annual data) as the proxy of uncertainty as an independent variable measure from Baker et al. (Citation2012).
4.1.3. Gross domestic product (control variable)
The growth of GDP is the control variable in t time period and in this study we take lag of growth rate of GDP in percentage. The GDP growth shows as the role of macroeconomic variable on innovation. The effects of aggregate economic activities on innovation can be controlled by the growth rate because when GDP growth increases, related to economic prospective, the people may become more optimistic and they are ready to invest more in intangible assets. GDP growth rate as control variable is also used by Hsu et al. (Citation2014) in their study. The data of annual GDP growth rate of China are obtained from World Development Indicators (WDI). This is a key variable because this is likely to associate with subsequent innovation.
4.2. Methodology
This study examines the relationship between innovation and EPU related to within-country data of China using the following function:
So, this study contributes by taking an inclusive method to examine the relationship between innovation and EPU for China with the help of a theoretically justified model that has not been done so far. Literature of time series analysis proposes that one must test to determine whether a long-run correlation finds among the variables in the specific model. To find out the relationship between variables, numbers of econometric techniques are accessible in the published literature, but this study based on time series data used the autoregressive distributed lag model (ARDL) method to examine the main argument that is especially recommended by Pesaran, Shin, and Smith (Citation2001), because ARDL test has some significant advantages (see Laurenceson & Chai, Citation2003; Pesaran & Shin, Citation1999).Footnote1 The first step of the ARDL bounds testing method shows the existence of one or more long-run correlations between innovation and the remaining regressors are examined by calculating the F-statistic for testing the significance of the lagged levels of the variables in the error correction form in the model of ARDL (see also Hashem & Pesaran, Citation1997, pp. 304–305). So, this current study uses the ARDL bounds testing technique because of the several advantages it offers.
The ARDL method is more preferred than the method of Johansen’s (Citation1992) maximum likelihood and the method of Pesaran et al. (Citation2001). The ARDL method is used to test for existence of a relationship between innovation and EPU adopted for the following reasons. The ARDL method does not impose the most restrictive assumption, for example that all variables related to specific model should have the same order of integration, and it can be used whether the order of integration of variables are I(0), I(1), (Hashem & Pesaran, Citation1997). Besides this, ARDL estimators with its small sample properties are more superior to the co-integration techniques of Johansen and Juselius and long-run coefficients of estimators based on ARDL are greatly consistent in small sample sizes (as the advantage of small sample size properties of the ARDL method are far improved to that of the Johansen and Juselius’s technique of co-integration) (Pesaran & Shin, Citation1999). Moreover, endogeneity problem is less in the framework of ARDL method because it is free of residual correlation. The ARDL method overcomes the issues causing from non-stationary time series data (Laurenceson & Chai, Citation2003). Finally, the ARDL bonds technique help to generate dynamic error correction model without losing information about long-term time period through a simple linear transformation method. So, the ARDL test is used because its various well-documented advantages in the literature by many researchers are already mentioned above.
The time series data used for empirical analysis call for the checking of stationarity of all the given variables. Testing of stationarity of the data is an important prerequisite to avoid the key problem of spurious regression. The results of ordinary least square (OLS) regression results will be biased if data are non-stationary and used in level form. If the variables are non-stationary at a level, then different methods are available to search co-integration in non-stationary series, like Engle–Granger’s (Citation1987) test, two-step Johansen’s (Citation1992) maximum likelihood method, the models of Pesaran–Shin’s (Citation1999) and Pesaran et al. (Citation2001) that explain the ARDL models. Engle–Granger’s method does not support if greater than one co-integrating vector is present in the analysis (Seddighi, Lawler, & Katos, Citation2006). The method of ARDL bounds testing analysis to co-integration includes estimating the “unrestricted error correction method” (UECM) of the ARDL approach which is given below:
The null hypotheses :
=
= 0,
:
=
,
=
, while alternative hypothesis is
:
0,
:
,
Intercepts are drift component and
is error term and assumed to be white noise. To check the absence of serial correlation, we used Akaike Information Criterion (AIC) to choose the optimal lag length for the model.
The next step is to evaluate the F-statistic calculated with critical bounds value by Turner (Citation2006) to investigate the long-run (co-integration) relationship between variables exist or not. If calculated F-statistic value is greater than upper critical bound values, then it shows that long-run (co-integration) relationship exists. If computed F-statistics value is lower than the lower critical bound value, then there is no co-integration. The decision of co-integration is inconclusive when the value of F-statistic lies between lower and upper critical bounds.
After the estimation of the long-run association among variables, the other main objective is to find causality among variables. To investigate this causality, the method of innovation accounting approach (IAA) is used. Variance decomposition approach and impulse response function (IRF) method are considered in this approach. The contribution of individual variable in the variation occurred in a specific variable and can be detected by variance decomposition based on the sample period. Variation in one variable is significantly explained by the other variable, which shows that the one variable causes the other variable. This study applied the innovation accounting approach (IAA) method. This IAA method is based on Variance decomposition approach and impulse response function (IRF) method.
Table indicates the descriptive statistics for all variables. Table also shows the correlation matrix, and Jarque–Bera test is for normality checking and results show that all the variables are normally distributed. Results of correlation matrices indicate that data have no problem of multicollinearity.
Table 1. Descriptive statistics and correlation matrices
4.2.1. Unit root test
It is necessary to check all the variables for stationary otherwise the regression will be spurious. For this purpose, Augmented Dickey–Fuller (ADF) test is applied to detect the stationary problem that variables are stationary or non-stationary. ADF is also applied to establish the order of integration for all the variables.
4.3. Estimation results and discussion
Although the approach of ARDL related to co-integration is valid irrespective of whether the dependent or independent variables are integrated of order (0) or (1), before using ARDL approach, the pretest for non-stationarity is important because of the presence of different variables with I(2) or higher order of integration can complicate the F-statistics test, making the biased results (Ouattara, Citation2004). As a result, the test of ADF is performed to check the order of integration of the variables. Results of ADF test is shown in Table that uncertainty have no unit root problem and innovation and GDP have unit root problem and stationary at first difference level.
Table 2. The results of unit root tests
After the identification of the order of integration of all variables, our next task to find out whether or not, there is a long-run association among all these variables. However, before arranged to testing of co-integration analysis, a main step is to choose the optimal lag length of the selected variables. For this purpose, main conventional methods are used. Three optimal lag length criteria are according to conventional method. Followed the AIC, we selected the lag length, after this the ARDL bound testing technique to co-integration is used to examine the long-run association among all variables. Table shows the statistical results of the test.
Table 3. ARDL bounds testing to co-integration analysis
Table indicates that all the three ARDL equations are examined taking each variable as dependent variable, respectively. Table shows each equation and their respective lag length of dependent and all independent variables. The results propose that null hypothesis ( of no longer association between the variables is rejected at 5 and 10%, respectively, when innovation, EPU and GDP are treated as response variables. The estimated values of F-statistics are 5.99, 7.71 and 5.80 while the upper bound value is 5.06, 4.01 and 3.52 at 5% and 10% levels of significance (Designed by Pesaran et al., Citation2001and Narayan, Citation2005). These results indicate three co-integration vectors among innovation, EPU and GDP over the study period of 1995 to 2015 in case of Republic of China.
The optimal lag length structure is selected by AIC. F-statistics values are taken from Wald test after using ARDL approach. Lower and upper bonds values are taken from Pesaran et al. (Citation2001). The null hypothesis ( that there is no heteroskedasticity (ARCH), and no serial correlation (Breusch–Godfrey lm test). Results indicate that we accepted null hypothesis (which means long-run relationship exists). The
of Ramsey reset test indicates that the model is correctly specified, we also accept null hypothesis in case of Jarque Bera (J.B test), which shows that data are normally distributed. Null hypothesis indicates that the values are greater than 5% level of significance.
According to results of these variables, in case of innovation being dependent variable, long-run co-integration among innovation, EPU and GDP. These statistical results are achieved by applying the fully modified ordinary least square (FMOLS) approach. After concluding the existence of co-integration (long run) among these variables, Equation 2 has been calculated applying ARDL co-integration approach to find the long-run estimates. These results are shown in Table .
Table 4. Long-run results (is dependent variable)
The FMOLS approach is used to detect the relationship between innovation and EPU controlling the macroeconomic variable (GDP) in China. This research analysis was focused to observe the effect of EPU especially, is innovation highly correlated to the future uncertainty in China? Uncertainty is negatively related to innovation in China. Results indicate that if there is 1% increase in uncertainty, it will bring 0.10% decrease in innovation in China at 10% significant level. China’s modernization and developmental mission is continuing but this creates large mismatch and problems in China. The results of diagnostic tests are mentioned in the lower part of Table , which indicates the validity of data. Moreover, evaluations of model specification (FMOLS) also show the robustness of results.
After the discussion of long-run dynamics, the next step concern is to analyze the main direction of causality among all these variables. As we discussed before, the purpose of the ARDL bounds testing method to co-integration is only to investigate the existence of long correlation between the variable but direction of causality cannot be suggested by this approach. Morley (Citation2006) discussed that existence of long-run connection between the dependent and independent variables is only the order of necessary condition but not the order of sufficient condition to reject the non-causality hypothesis. The empirical result found in Table confirms the co-integration between innovation, EPU and GDP but it is not sufficient to recognize the direction of causality. However, this existence of long-run association between all variables does propose that there should be causality at least in one main direction. These grounds require the used of IAAFootnote2 including variance decompositions and also “impulse response functions” (see Wolde-Rufael, Citation2009). The results of variance decompositions approach are shown in Table and these results are based on the direction of causality. Table indicates those three blocks which is showing the variance decomposition of each other three variables separately. Variance decomposition of innovation, uncertainty and GDP is represented in first, second and third blocks, respectively.
Table 5. Variance decomposition approach
The results of Table in first block indicate the shock or impulse to uncertainty in start or short run contributes 0.11% fluctuation in innovation, it illustrates that the contribution of EPU (0.11%) to variation in innovation. But in the long run if we checked the 9th and 10th periods of time thaen we can check that the shock to EPU contributes 49.57% and 51.02% variation in innovation. Similarly, the shock to GDP in the long run contributes 12.5% fluctuation in innovation. Result shows that 51.02% variation in innovation is caused by the EPU. In these results, if we analyze the results of GDP, we can find that GDP growth is also affected by uncertainty and it is surprising that shock to uncertainty in start or short run contributes 74.2% fluctuation in GDP and in the long run if we analyzed 10th period of time than we can check that the shock to EPU contributes 76.2% variation in GDP. Based on variance decomposition results, it is concluded that there is unidirectional causality running from uncertainty to innovation and uncertainty to GDP growth also.
The impulse response function is the second method in IAA. The result of impulse response function is given in Figure , which validates the variance decomposition’s results mentioned in Table . It is found from Figure that innovation is highly responding by the EPU. Fluctuation can be seen (response of LNN to UNC) with ups and downs due to fluctuation in uncertainty. EPU affects innovation, and innovation is decreasing with the passage of time. Figure shows the response of innovation to the combined effects of EPU and GDP, graphical representation indicate that as uncertainty increase the innovation and GDP growth is also decreasing.
Figure 1. Total patent applications in SIPO (1995–2015).
Data source: Chinese invention patent statistics taken from SIPO.
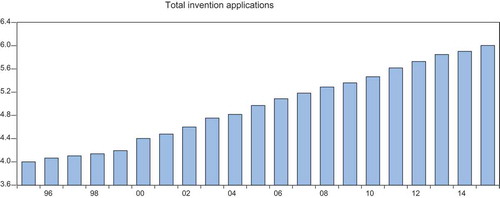
The combined results based on ARDL, IAA (variance decompositions and impulse response functions) and FMOLS raise an important point that calls for attention. The point is relating to the causality running from EPU to innovation. The future of China is uncertain, so when the economic uncertainty is higher, it lowers the value of future activities of the economy of China. So this study tries to analyze the EPU on innovation, which is neglected in many studies especially studies related to China (as China is a communist country). The proxy of uncertainty as EPU was applied in this research, which was also used by Kang and Ratti (Citation2015), they used EPU as measure of uncertainty for China was established based on Baker et al. (Citation2013), which indicates that diminution of uncertainty capacity to increase innovation. Uncertainty is significant and negatively related to innovation, representing that increase in uncertainty may distract the economic agents, and economic drivers focus their attention to the present so they decreased innovation to secure future. The Basu and Bundick (Citation2014, p. 12) examined in their empirical analysis that significant fall in investment is found because uncertainty shocks are significantly correlated with innovation especially in China because Chinese future is not clear so people prefer their present condition as compared with future. Reduction or abolition of uncertainty into the Chinese may be the best type of public policy to encourage innovation.
The main purpose of the underlying study was to scrutinize the causality between EPU and innovation. Using the ARDL approach to co-integration and the methods of innovation accounting for causality analysis, the study finds that innovation is highly affected by the uncertainty. EPU is the proxy of uncertainty used in this research which was also applied by Kang and Ratti (Citation2015), measure of uncertainty for China was established based on Baker et al. (Citation2012). The negative relationship between uncertainty and innovation is supported by the findings of Wang et al. (Citation2014) and Hsu et al. (Citation2014).
This paper concludes that there is a long-run relationship between EPU and innovation. The ARDL model describes the long-run effects of a country’s policy uncertainty on innovation and the methods of innovation accounting results indicate that there is short-run effects that exist but the degree of long-run effect is larger. Uncertain economic policy has a clear impact on innovation as well as inversely related to the GDP growth rate in China. The results suggest that government should adopt such economic policies which reduce the uncertainty related to economic policies to encourage innovational investment in China. The risk increases by uncertainty which encourages investors to postpone investment decisions. The government should also give more subsidies to investors related to innovational investment and activities.
This study concludes that EPU not only affects the current level of innovation, but also the decision of future innovational projects. This paper suggests that steady and sustainable economic policies by the government also minimize economic uncertainty and encourage the economic agents to increase economic activities in terms of investment. Our analysis also highlights that the strong relationship between innovation and political uncertainty depends on the nature of the political situation in the case of Republic of China. Unlike diminishing the innovation is stimulated by increasing economic political uncertainty. It is clear that the long-run consequence of political uncertainty is not clear and also a warning to policymakers especially in China about avoiding long debate about future policy is not entirely warranted.
Additional information
Funding
Notes on contributors
Hummera saleem
Hummera Saleem is, a Pakistani, doing PhD in Wuhan University department (China), school of economics and management. She won Higher Education Commission (HEC) scholarship for her PhD degree from China Republic. She focuses topics relating to economic growth and development and environmental degradation.
Wen Jiandong
Wen Jiandong is the honorable professor at school of economics and management in Wuhan University, China.
Muhammad Bilal Khan
Muhammad Bilal Khan is, a Pakistani, doing PhD in Wuhan University department (China), school of economics and management. The main area of interest is corporate finance and accounting.
Notes
1. Estimation procedure in detail can be obtained from Hashem and Pesaran (Citation1997).
2. Mostly economic literature is argued that the Granger-causality techniques such as “VECM Granger causality Test” have some limitations. The causality approach cannot show the relative strength of causal association between the variables beyond the given time period. This weak property of the reliability of causality resulted by the approach of VECM Granger (Wolde—Rufael, Citation2009).
References
- Aastveit, K. A., G. J. Natvik, and S. Sola (2013). “Economic Uncertainty and the Effectiveness of Monetary Policy”, Norges Bank Working Paper Series, 17.
- Abel, A. (1983). Optimal investment under uncertainty. American Economic Review, 73(1), 228233.
- Acharya, V. V., & Subramanian, K. V. (2009). Bankruptcy codes and innovation. Review of Financial Studies, 22, 4949–4988. doi:10.1093/rfs/hhp019
- Adner, R., & Levinthal, D. A. (2004). What is not a real option: Considering boundaries of the application for real options to business strategy. Academy of Management Review, 1(29), 74–85.
- Aghion, P., & Tirole, J. (1994). On the management of innovation. Quarterly Journal of Economics, 109, 1185–1209. doi:10.2307/2118360
- Alesina, A., & Perotti, R. (1996). Income distribution, political instability, and investment. European Economic Review, 40(6), 1203-1228.
- Alexopoulos, M. and J. Cohen (2009). Uncertain Times, Uncertain Measures, University of Toronto Department of Economics Working Paper, 352.
- An, H., Chen, Y., Luo, D., & Zhang, T. (2016). Political uncertainty and corporate investment: Evidence from China. Journal of Corporate Finance, 36, 147–189. doi:10.1016/j.jcorpfin.2015.11.003
- Audretsch, D. B., & Fedman, M. P. (2004). Knowledge spillovers and the geography of innovation. In J. V. Henderson & J. Thisse (Eds.), Handbook of urban and regional economics: Cities and geography (Vol. 4, pp. 2713–2739). Amsterdam: North Holland Publishing.
- Baker, S. R., Bloom, N., & Davis, S. J. (2012). Has economic policy uncertainty hampered the recovery?. Chapter 3 in L. E. Ohanian, J. B. Taylor, & I. J. Wright (Eds.), Government policies and the delayed economic recovery. Hoover Press, Hoover Institution, Stanford University.
- Baker, S., Bloom, N., Davis, S., (2013). Measuring economic policy uncertainty. Discussion Paper 13–02, Chicago Booth Research Paper.
- Baker, S.R. Bloom, N. and Davis, S.J., (2015). Measuring Economic Policy Uncertainty, NBER Working Paper No. 21633. Available at http://eprints.lse.ac.uk/64986/1/dp1379.pdf
- Barro, R. J. (1991). Economic growth in a cross-section of countries. Quarterly Journal of Economics, 106, 407–444. doi:10.2307/2937943
- Basu, S., & Bundick, B.,(2014). Uncertainty shocksina model of effective demand (WorkingPaperRWP14–15). Federal Reserve Bank of Kansas City.
- Bernanke, B. S. (1983). Irreversibility, uncertainty, and cyclical investment. The Quarterly Journal of Economics, 98, 14–31. doi:10.2307/1885568
- Bernstein, S. (2015). Does going public affect innovation? The Journal of Finance, 4, 1365–1403. doi:10.1111/jofi.12275
- Benati, L. (2014). “Do TFP and the Relative Price of Investment Share a Common I(1) Component?,” Journal of Economic Dynamics and Control, forthcoming..
- Bhattacharya, U., Hsu, P., Tian, X., & Xu, Y. (2015). What affects innovation more: Policy or policy uncertainty? Quarterly Journal of Economics, 98, 85–106.
- Bloom, N. (2009). The impact of uncertainty shocks. Econometrica, 77, 623–685. doi:10.3982/ECTA6248
- Bloom, N., Bond, S., & Reenen, J. V. (2016). Trade induced technical change? The impact of Chinese imports on innovation, IT and productivity. The Review of Economic Studies, 83, 87–117. doi:10.1093/restud/rdv039
- Bloom, N., Bond, S., & Reenen, J. V. (2007). Uncertainty and investment dynamics. Review of Economic Studies, 74, 391–415. doi:10.1111/roes.2007.74.issue-2
- Bloom, N., Draca, M., & Van Reenen, J. (2011). Trade induced technical change? The impact of Chinese imports on innovation, IT and productivity (National Bureau of Economic Research: Working paper No.16717).
- Bloom, N., & Van Reenen, J. (2002). Patents, real options, and firm performance. Economic Journal, 112(478), 97–116. doi:10.1111/1468-0297.00022
- Bowman, E. H., & Moskowitz, G. T. (2001). Real options analysis and strategic decision making. Organization Science, 12(6), 772–777. doi:10.1287/orsc.12.6.772.10080
- Caggiano, G., E. Castelnuovo, and N. Groshenny (2014). Uncertainty Shocks and Unemployment Dynamics: An Analysis of Post-WWII US Recessions, Journal of Monetary Economics, 67, 78–92.
- Chen, S., Sun, Z., Tang, S., & Wu, D. (2011). Government intervention and investment efficiency: Evidence from China. Journal of Corporate Finance, 17, 259–271. doi:10.1016/j.jcorpfin.2010.08.004
- Chen, Y.-F., & Funke, M. (2003). Option value, policy uncertainty, and the foreign direct investment decision. HWWA Discussion Papers 234, Hamburg Institute of International Economics (HWWA).
- Czarnitzki, D., & Toole, A. A. (2007). Business R & D and the interplay of R & D subsidies and product market uncertainty. Review of Industrial Organization, 31, 169–181. doi:10.1007/s11151-007-9152-x
- Czarnitzki, D., & Toole, A. A. (2011). Patent protection, market uncertainty, and R & D investment. The Review of Economics and Statistics, 93, 147–159. doi:10.1162/REST_a_00069
- Dang, J., & Motohashi, K. (2015). Patent statistics: A good indicator for innovation in China? Patent subsidy program impacts on patent quality. China Economic Review, 35(2015), 137–155. doi:10.1016/j.chieco.2015.03.012
- Dixit, A. K. (1989). Entry and exit decisions under uncertainty. Journal of Political Economy, 97, 620–638. doi:10.1086/261619
- Dixit, A. K., & Pindyck, R. S. (1994). Investment under uncertainty Princeton. NJ: Princeton University Press.
- Dong, J., & Gou, Y. N. (2010). Corporate governance structure, managerial discretion, and the R & D investment in China. International Review of Economics and Finance, 19(2), 180–188. doi:10.1016/j.iref.2009.10.001
- Duta, S., & Lanvin, B. (Eds). (2013). Global Innovation Index 2013, The Local Dynamics of Innovation. Johnson Cornell, NY: INSEAD, The business school of the world. WIPO.
- Engle, R. F., & Granger, C. W. J. (1987). Co integration and error correction representation: Estimation and testing. Econo- metrica, 55(2), 251–276. doi:10.2307/1913236
- Faleye, O., Kovacs, T., & Venkateswaran, A. (2014). Do better-connected CEOs innovate more. Journal of financial and Quantitative, 49, 1201–1225. doi:10.1017/S0022109014000714
- Fan, J., Huang, J., & Zhu, N. (2013). Institutions, ownership structures, and distress resolution in China. Journal of Corporate Finance, 23, 71–87. doi:10.1016/j.jcorpfin.2013.07.005
- Fan, J. P., Wong, T. J., & Zhang, T. (2007). Politically connected CEOs, corporate governance, and Post-IPO performance of China’s newly partially privatized firms. Journal of Financial Economics, 84, 330–357. doi:10.1016/j.jfineco.2006.03.008
- Fernandez-Villaverde, J., Guerron-Quintana, P., Kuester, K., & Rubio-Ramirez, J. (2013). Fiscal volatility shocks and economic activity (Working Paper). University of Pennsylvania.
- Firth, M., Gong Stephen, X., & Shan, L. (2013). Cost of government and firm value. Journal of Corporate Finance, 21, 136–152. doi:10.1016/j.jcorpfin.2013.01.008
- Gulen, H., & Ion, M. (2015). Policy uncertainty and corporate investment. The Review of Financial Studies, 29, 523–564.
- Handley, K. (2014). Exporting under trade policy uncertainty: Theory and evidence. Journal of International Economics, 94(1), 50-66.
- Hartman, R. (1972). The effects of price and cost uncertainty on investment. Journal of Economic Theory, 5(2), 258–266. doi:10.1016/0022-0531(72)90105-6
- Hashem, P. M., & Pesaran, B. (1997). Working with Microfit 4.0: Interactive econometric analysis. UK: Oxford University Press.
- Holmstrom, B. (1989). Agency costs and innovation. Journal of Economic Behavior and Organization, 12, 305–327. doi:10.1016/0167-2681(89)90025-5
- Hsu, P., Tian, X., & Xu, Y. (2014). Financial development and innovation: Cross- country evidence. Journal of Financial Economics, Forthcoming. doi:10.1016/j.jfineco.2013.12.002
- Johansen, S. (1992). A representation of vector autoregressive processes integrated of order 2. Econometric Theory, 8, 188–202.
- Julio, B., & Yook, Y. (2012). Policy uncertainty and corporate investment cycles. Journal of Finance, 67, 45–83. doi:10.1111/j.1540-6261.2011.01707.x
- Jurado, K., Ludvingson, S. C., & Ng, S. (2015). Measuring uncertainty. American Economic Review, 105, 1177-1216.
- Kang, W., & Ratti, R. A. (2015). Oil shocks and China’s policy uncertainty and stock returns, Economics of Transition 23, 657, 676.
- Keller, W. (2004). International technology diffusion. Journal of Economic Literature, 42(3), 752–782. doi:10.1257/0022051042177685
- Kogut, B. (2001). Capabilities as real options. Organization Science, 12(6), 744–758. doi:10.1287/orsc.12.6.744.10082
- Kogut, B., & Kulatilaka, N. (2004). Real options pricing and organizations: The contingent risks of extended theoretical domain. Academy of Management Review, 29, 1.
- Laurenceson, J., & Chai, J. C. H. (2003). Financial reform and economic development in China. advances in Chinese economic studies series. Cheltenham, UK: Edward Elgar.
- Lin, C., Lin, P., & Song, F. (2010). Property rights protection and corporate R & D: Evidence from China. Journal of Development Economics, 93, 49–62. doi:10.1016/j.jdeveco.2009.04.006
- Lin, C., Lin, P., Song, F., & Li, C. (2011). Managerial incentives, CEO characteristics and corporate innovation in China’s private sector. Journal of Comparative Economics, 39, 176190. doi:10.1016/j.jce.2009.12.001
- McDonald, R., & Siegel, L. D. (1986). The value of waiting to invest. Quarterly Journal of Economics, 101, 707–727. doi:10.2307/1884175
- McGrath, R. G. (1997). A real options logic for initiating technology positioning investments. Academy of Management Review, 22(4), 974–996. doi:10.5465/amr.1997.9711022113
- McGrath, R. G., & Nerkar, A. (2004). Real options reasoning and a new look at the R&D investment strategies of pharmaceutical firms. Strategic Management Journal, 25, 1–21. doi:10.1002/smj.358
- Morley, B. (2006). Causality between economic growth and immigration: An ARDL bounds testing approach. Econ. Lett., 90(1), 72–76. doi:10.1016/j.econlet.2005.07.008
- Mumtaz, H. and P. Surico (2013). Policy Uncertainty and Aggregate Fluctuations, Working Paper, School of Economics and Finance, Queen Mary, University of London.
- Nanda, R., & Rhodes-Kropf, M. (2013). Lnvestment cycles and startup innovation. Journal of Financial Economics, 110(2), 403-418.
- Nodari, G. (2014). Financial Regulation Policy Uncertainty and Credit Spreads in the U.S., Journal of Macroeconomics, Volume 41, September 2014, pp.122-132.
- Narayan, P. N. (2005). The saving and investment nexus for China: Evidence from cointegration tests. Appl. Econ., 37(17), 1979–1990. doi:10.1080/00036840500278103
- Ocampo, J. A. (2005). A broad view of macroeconomic stability. (DESA Working Paper No. 1. ST/ESA/2005/DWP/1.6)
- Oi, W. (1961). The desirability of price instability under perfect competition. Econometrica, 29(1), 58–64. doi:10.2307/1907687
- Ouattara, B. (2004). Foreign aid and fiscal policy in senegal. Manchester, UK: University of Manchester.
- Pesaran, M. H., & Shin, Y. (1999). An autoregressive distributed lag modeling approach to co integration analysis. Chapter 11. In S. Strom (Ed.), Econometrics and economic theory in the 20th century: The Ragnar Frisch Centennial Symposium. Cambridge: Cambridge University Press.
- Pesaran, M. H., Shin, Y., & Smith, R. (2001). Bounds testing approaches to the analysis of level relationships. J. Appl. Econom., 16(3), 289–326. doi:10.1002/jae.616
- Rodrik, D. (1991). Policy uncertainty and private investment in developing countries. Journal of Development Economics, 36(2), 229–242. doi:10.1016/0304-3878(91)90034-S
- Seddighi, H. R., Lawler, A., & Katos, A. V. (2006). Applied econometrics: A practical approach. London: Routledge.
- Shleifer, A., & Vishny, R. (1994). Politicians and firms. The Quarterly Journal of Economics, 109, 995–1025. doi:10.2307/2118354
- Solow, R. (1957). Technical change and the aggregate production function. Review of Economics and Statistics, 39, 312–320. doi:10.2307/1926047
- Turner, P. (2006). Response surfaces for an F-test for Co integration. Appl. Econ. Lett., 13(8), 479–482. doi:10.1080/13504850500401726
- Wang, Y. Z., Chen, C. R., & Huang, Y. S. (2014). Economic policy uncertainty and corporate investment: Evidence from China. Pacific-Basin Finance Journal, 26, 227–243. doi:10.1016/j.pacfin.2013.12.008
- Wolde - Rufael, Y. (2009). The defence spending-external debt nexus in Ethiopia. Def. Peace Econ., 20(5), 423–436. doi:10.1080/03066150902868171