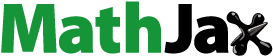
Abstract
This paper investigates the link between oil price uncertainty shocks and key macroeconomic indicators of a net oil importing country, South Africa. Monthly data covering the period 1990:01 to 2015:12 is used. The Structural Vector Autoregressive (SVAR) methodology is applied incorporating realized volatility as an indicator of oil price uncertainty to investigate the short run effects of oil price uncertainty. The Generalised Impulse Response Functions (GIRF) analysis reveals that for most variables, oil price uncertainty shock has an adverse and persistent effect over time. Consistent with GIRF, the Generalised Forecast Error Variance Decompositions (GFEVDs) analysis also points out that oil price uncertainty shocks contributes substantially to variations in real output, inflation and various macroeconomic variables of South Africa. Therefore, SVAR analysis reveals the significant role of exogenous oil prices on the economy of South Africa when price uncertainty shocks exist. The policy implications of these findings are drawn.
PUBLIC INTEREST STATEMENT
South Africa is an oil importing economy which highly depends on non-renewable energy. It continues to dominate the region’s consumption of both petroleum and total energy. The exogenously determined oil prices potentially generate uncertainties in the whole economy. The purpose of this paper is to characterize the impact of the oil price uncertainty shock on a small open economy like South Africa taking a holistic approach focusing on several macroeconomic variables. The study empirically tracks out how the oil price uncertainty shock affects economic activities in the economy using the Structural Vector Autoregressive (SVAR) model. It employs realised volatility as a proxy for uncertainty as volatility is known to display surges of uncertainty following major shocks. The transmission channels through which spill overs from policy specific shocks follow are also observed. The aim is to trigger conversations around the long-term energy policies of South Africa to mitigate economic uncertainties.
1. Introduction
The biggest risk facing companies in the oil and gas sector is uncertainty about energy prices. This makes it difficult to calculate the economic benefits of investments over a project’s life cycle and may hence impact on economic activities. The characteristic relationship between oil price and macroeconomic activity remains one of the key, debatable and inconclusive subjects in energy economics (Bashar, Wadud, & Ahmed, Citation2013). Since the 1970s and at least until recently the global fluctuations in oil prices have been considered as a major source of macroeconomic uncertainties around the world. Incidences of low growth, high inflation and unemployment, occurred in most developed economies in the early and late 1970s making oil price changes a significant cause of concern for policymakers (Blanchard & Gali, Citation2007; Rafiq, Salim, & Bloch, Citation2009). The oil price uncertainty created by oil price volatility causes adjustments in an economy besides the adjustments from the oil price in levels. Sharp oil price changes affect various economic activities depending on the degree of uncertainty created by oil price volatility, and the attitudes of economic agents towards uncertainty (Ebrahim, Inderwildi, & King, Citation2014). Most sources of oil price volatility are known to originate from wars, terrorist attacks, conflicts in the Middle East and political events involving OPEC members (Bloom, Citation2009; Guo & Kliesen, Citation2005). The resultant uncertainty after such major shocks for example, the OPEC 1 oil-price shock, stem from events exogenous to economic activity (Hamilton, Citation1983; Plante & Traum, Citation2012).
While oil demand is more observed in industrialised developed economies, the demand for oil in developing economies is also on an upward trend (Birol, Citation2007). The South African economy has a convincing track record of growth through privatisation and extensive trade liberalisation as compared to other African economies. The economy continues to dominate the region’s consumption of both petroleum and total energy (Nkomo, Citation2006). However, as a net oil importer South Africa continues to experience increased demand for energy and is expected to be susceptible to oil price shocks regardless of the phase of the business cycle (Kilian, Citation2008). Changes in oil prices impact real economic activities of oil importing countries on both the demand and supply side (Khan & Ahmed, Citation2011). From the consumer position, a rise in oil price causes a growth in energy bills, dwindling real money balances held by households and ultimately reducing the aggregate demand (Elder & Serletis, Citation2010). From the producer position, firms face an increase in production cost which leads to a decline in productivity which in turn negatively affects employment, core inflation, and investment (Lescaroux and Mignon, Citation2008). Since oil prices affects both production and consumption decisions, it has implications for the terms of trade for oil importing countries. The main interest in this paper is to characterize how uncertainty in oil price affects macroeconomic activity of a small open economy like South Africa.
Theoretical predictions about the effect of oil price uncertainty on economic activity are mixed. On one hand, higher uncertainty (example in oil prices) increases the option value of waiting on investment thus reducing growth (Bernanke, Citation1983; Bloom, Citation2009; Dixit, Citation1992; Elder & Serletis, Citation2010; Pindyck, Citation1991). In other words, higher oil price uncertainty will cause firms to postpone irreversible investment decisions, preferring to wait for more information if the cash flow from the investment is dependent upon the oil price. This will result in cyclical fluctuations in the economy (Jo, Citation2014). Similarly, the real options theory is applicable to durable consumption since durable good expenditures cannot be completely recovered once conducted (Kilian & Vigfusson, Citation2011a, Citation2011b). On the other hand, Plante and Traum (Citation2012) show that an increase in oil price volatility is likely to result in increased investment and higher real GDP in a general equilibrium model due to the heightened precautionary savings motives though durable spending decreases temporarily. Also Baskaya et al. (Citation2013) explain that higher uncertainty heightens precautionary savings, which in turn results in higher investment, in a scenario where agents have no access to alternative assets.
Our contribution in this study is to take a holistic approach by focusing on the effect of oil price uncertainty on several macroeconomic variables of South Africa namely: industrial production, inflation, interest rate, exchange rate, trade balance and money supply. This enables us to assess the overall performance of the economy under oil price uncertainty. In addition, this allows for dynamic interaction between the various variables and avoids any misspecification bias. This is lacking in most previous studies including the South African ones which focus on bivariate relationship (Aye, Dadam, Gupta, & Mamba, Citation2014; Aye, Citation2015; Dave & Aye, Citation2015; Elder & Serletis, Citation2010; Guo & Kliesen, Citation2005; Jo, Citation2014 among others). Ron, Kilian, and Vigfusson (Citation2013) and Kilian and Vigfusson (Citation2011b) observed that oil price uncertainty may not have been well captured by commonly used oil price volatility measures; hence some mixed theoretical and empirical findings. Therefore, since the previous South African studies relied on model based oil price volatility measure derived mainly from the GARCH process, the current study adds value by employing realized volatility which is nonparametric and hence does not depend on the model and/or distributional assumptions and also greatly improves the precision of the estimated uncertainty series (Andersen & Benzoni, Citation2009; Jo, Citation2014). Moreover, realized volatility measure provides empirical content to the latent variance variable and facilitate direct estimation of parametric models (Andersen & Benzoni, Citation2009). Although closely related to Rafiq et al. (Citation2009) on the Thai economy in terms of wider variable coverage, the current study differs in that it employs more recent data covering the recent global economic and financial crisis and uses higher frequency (monthly) data which is more amenable to uncertainty analysis (Dedi et al., Citation2016). Also we employ a theoretically founded model (Structural Vector Autoregressive (SVAR model) which allows us to make the necessary restrictions on the estimated reduced form model, required for identification of the underlying structural model, provided by economic theory unlike the traditional VAR employed in Rafiq et al. (Citation2009) which is an atheoretical approach. Moreover, the current study included additional variables such as money supply and the exchange rate series, the latter being an important variable for the transmission of oil price volatility given that South Africa is an oil importing country and hence integrated into the world economy. The results based on the SVAR model indicate that South African economy is adversely affected by the international oil price uncertainty shocks.
The rest of the paper is organized as follows: section two provides a review of empirical studies. The methodology is presented in section three. Results are discussed in section four. Section five concludes.
2. Literature review
Employing volatility to capture oil price uncertainty is quite visible in the literature (Elder & Serletis, Citation2010) and recent evidence documents evidence of volatility in oil prices (Baumeister & Kilian, Citation2016; Demirbas, Al-Sasi, & Nizami, Citation2017). This literature review focuses on studies that considered the effect of oil price uncertainty.
For instance, Guo and Kliesen (Citation2005) investigate the impact of oil price shocks on U.S macroeconomic activity over the period 1984–2004. With the realised volatility measure built from daily crude oil future prices traded on the New York Mercantile Exchange (NYMEX), the findings indicate a negative and significant effect of oil price volatility on key US macro-economic variables for instance fixed investment, consumption, employment and the unemployment rate. The authors also hypothesised asymmetric effect stating that large oil price volatility, in the short run may adversely affect aggregate output as it delays business investment by increasing uncertainty or encourage costly resource allocation. The results also suggest that variations in oil prices are less significant as compared to the uncertainty about future prices.
The Vector Autoregressive (VAR) model used in this study has been widely used in empirical literature to identify the purely exogenous shocks to trace out how the economy reacts, thus impulse response analysis. Jo (Citation2014) investigates how oil price uncertainty affects global real economic activity using quarterly data from 1958Q2 to 2008Q3. The study utilizes a VAR model with stochastic volatility, and it is to be noted that the study incorporated realized volatility as an additional uncertainty indicator. The results indicate that oil price uncertainty shock has significant negative effects on the world industrial production, the proxy used for economic activity.
Bredin, Elder, and Fountas (Citation2011) investigate the relationship between oil price uncertainty and industrial production for the G-7 countries using structural VAR adjusted for a multivariate GARCH-in-mean. The key result indicates that oil price uncertainty has a significant negative effect in four of the G7 countries, Canada, France, the United Kingdom and the United States. In their result, the impulse response analysis also suggests that in the short run, positive and negative oil shocks may be contractionary.
Bashar et al. (Citation2013) in the multivariate SVAR framework analyse the direct effects of oil price uncertainty shocks on macro economy of a net oil exporting country, Canada. The results obtained suggest that although the aggregate level of output is not affected by the shocks to oil price level, the oil price uncertainty contribute significant impacts on the economy of Canada. The results also indicate resemblance of an adverse demand shock attributable to higher oil price uncertainty, which is found to considerably decrease both output and price levels.
A study on China is quite interesting to look at considering the size of the country and the nature of the economy. Caporale, Ali, and Spagnolo (Citation2015) investigate the time-varying impact of oil price uncertainty on stock prices in China. The study covers the period 1997:01–2014:02 utilising weekly data and a bivariate GARCH-in-mean VAR estimation on ten sectoral indices. The results points out positive effects of oil price volatility on stock returns in phases characterised by demand-side shocks in all investigated sectors with the exception of the Financials, Consumer Services, and Oil and Gas. The latter two sectors are found to exhibit a negative response to oil price uncertainty during periods with supply-side shocks instead. In contrast, during periods with precautionary demand shocks, the oil price uncertainty effect appears to be insignificant.
Using a logistic transition based autoregressive model (LSTR), Ahmed and Wadud (Citation2017) investigate the effect of oil price volatility on the Australian equity market. They show that a one period increase or a shock in oil price volatility raises volatility of equity in consumer discretion, consumer staple, finance, industry, telecom and consumer staple sectors with equity volatility in industries exhibit a larger and prolonged positive response compared to the equity volatility response of other sectors. Similarly, Luo and Qin (Citation2017) examine the impact of realized volatility and the crude oil volatility index (OVX) on the Chinese stock market. They find that the OVX shocks have significant and negative effects while the impact of realized volatility shocks is negligible, especially after the recent financial crisis.
Elder (Citation2018) examine the effect of oil price volatility on disaggregated measures of industrial production namely indexes for industrial production excluding technology and motor vehicles, energy-related special aggregates and non-energy-related special aggregates. They find the effects of oil price volatility are concentrated in activities related to primary energy generation and oil and gas drilling relative to other energy-related market groups. In addition, oil price volatility affects a broad range of special aggregates among the non-energy-related market groups, including aggregates sorted by consumer goods and business equipment.
This analysis can also be viewed as part of the recent literature on the macroeconomic implications of oil price volatility on developing countries. Rafiq et al. (Citation2009) using monthly data from 1993:1 to 2006:4 examines the impact of oil price volatility on essential macroeconomic variables of Thailand in a VAR framework. Realized volatility measure was used to construct the oil price volatility data. As the structural break test points out breaks during the Asian financial crisis, the paper applies two different VAR structures, one for the entire period and the other for the post-crisis period. For the total period, causality tests, impulse response functions and variance decomposition tests show that oil price volatility has substantial effect on investment and unemployment. Conversely, the post-crisis period review indicates that the effect of oil price volatility is transferred to the budget deficit. Dutta, Nikkinen, and Rothovius (Citation2017) focused on analysing the impact of implied crude oil volatility index (OVX) on the realized volatility of Middle East and African stock markets using modified GARCH models. Their findings show that the oil market uncertainty has substantial effects on most of the markets.
With respect to South Africa, there is a growing literature on the impact of oil price shocks and uncertainty. Aye et al. (Citation2014) analyse the impact of oil price uncertainty on manufacturing production of South Africa utilising a bivariate GARCH-in-mean VAR model. The results indicate that oil price uncertainty have a significant negative effect on manufacturing production. Additionally, the paper also establishes that the reactions of manufacturing production to positive and negative shocks are asymmetric.
Another study by Aye (Citation2015) measures oil price uncertainty, utilising conditional standard deviation of the one-step-ahead forecast error for the change in oil price. The study investigates the effect of oil price uncertainty on South African stock returns using weekly data covering the period spanning from 1995:07:01 to 2014:08:30. In the analysis a bivariate GARCH-in-mean VAR framework is used. The results show a negative but marginally significant effect of oil price uncertainty on stock returns. In addition, there is evidence of asymmetric response of stock returns to negative and positive oil price uncertainty shocks.
Dave and Aye (Citation2015), using quarterly data from 1960 to 2014, in the GARCH-in-mean VAR model examines the impact of the volatility of oil prices on savings in South Africa. The study measures oil price uncertainty as the conditional standard deviation of the change in oil price one-step-ahead forecast error. The results show that oil price uncertainty negatively affects South Africa’s savings. In addition, in both direction and magnitude there are symmetric responses of savings to a positive and negative oil price shocks.
From the foregoing literature review, there is evidence that oil price uncertainty shocks have an impact on macroeconomic variables such as GDP, investment, savings and stock returns among others. The literature also suggests an asymmetric effect of oil price uncertainty shocks on the economy (See also Baumeister & Kilian, Citation2016; Charfeddine, Klein, & Walther, Citation2018; Hamilton, Citation1996, Citation2003; Kilian & Vigfusson, Citation2011a, Citation2013, Citation2017; on oil price shocks). In addition, there have been a few academic accomplishments made to analyse the impact of oil price uncertainty on economic activities in the context of developing countries. More importantly in South Africa, most of the studies focus on bivariate GARCH-in-mean VAR model and the conditional standard deviation of oil price as a proxy for oil price uncertainty. It would be important to apply the realised oil price variance as a proxy for uncertainty in a multivariate SVAR model apart from the bivariate models to examine the impact of oil price uncertainty shock. This is the gap this study aims to fill.
3. Methodology
3.1. Data and variables
The study uses time series data on a monthly frequency spanning from January 1990 to December 2015. Monthly data is used as it allows effective capturing of the oil price uncertainty shocks over the years. Admittedly, the span of years covered for the study is quite limited starting from the 90s as this may affect the robustness of the results. However, this choice is practical in order to allow an adequately larger set of variables as some variables could not be found on a monthly frequency prior to 1990. Moreover, monthly data is used instead of quarterly data these provide large number of observations which is an important issue with volatility analysis (Dedi et al., Citation2016).
Daily oil price is important in this study in determining the key variable of interest, which is the oil price uncertainty, integrated into the SVAR to analyse the effects of the global oil price uncertainty shocks on the South African economy. South Africa is a small net oil importing economy and usually for such smaller economies, the world oil prices are anticipated to be exogenous. The estimated model utilized West Texas Intermediate (WTI) daily crude oil prices from the US Energy Information Administration. The proxy for oil price uncertainty is the series of the realised oil price volatility built from daily crude oil prices.
Industrial production index obtained from OECD Statistics was used as a proxy for output as GDP is not available on monthly frequency. Inflation
is calculated as the percentage change in the consumer price index (CPI), where CPI is acquired from the Federal Reserve Economic Data. Also Trade balance
, found by subtracting the aggregate imports from the aggregate exports is collected from International Financial Statistics (IFS) as well as South Africa’s National Definitions of Money
currency. The data for real effective exchange rate
, short term interest rate
were sourced from Quantec Financial Conditions Index (QFC) Monthly Report.
Therefore, seven variables as shown in Table are incorporated in the analysis to capture the inter-relationship within a SVAR framework. However, there is no unison on the number of variables essential in any given VAR model to provide a credible analysis of the economy. In a study by Dungey and Pagan (Citation2000) their VAR model had 11 variables, whilst in another study by Kim and Roubini (Citation2000) the authors discuss that seven variables are enough. Rafiq et al. (Citation2009), suggests that most of these variables are appropriate for summarising the relevant dynamics in the macro economy. In the estimation, all the variables were expressed in logarithmic notation with the exception of the inflation.
3.2. Measuring uncertainty
Before discussing the effect of oil price uncertainty on the economy, it is crucial to initially discuss how oil price uncertainty is to be measured. This study employs realised volatility to capture the oil price uncertainty following Andersen, Bollerslev, Diebold, and Labys (Citation2001); (Citation2003) who suggests that, given proper conditions, realised volatility is a highly efficient unbiased estimator of returns volatility.
The proxy for oil price uncertainty is the realised volatility series constructed from high frequency daily prices of crude oil. In this study, other measures of volatility are not being used as they are difficult to adopt in a multivariate framework (Rafiq et al., Citation2009). The realised volatility utilised in this study follows Andersen, Bollerslev, Diebold, and Labys (Citation2003), Guo and Kliesen (Citation2005) and Rafiq et al. (Citation2009), but, applying daily price changes in a month.
Whereis the past realised oil variance.
, a positive integer is the aggregate working days in a month thus period of return daily
oil price return in a month
. The expression (
is the logarithmic change in
the closing crude oil prices in a day
and the day before, in month
. Realised volatility (RV) is backward looking, that is, it is an ex-post measure of uncertainty. The realised oil volatility is shown in Figure . The realised oil volatility series is the summation of daily squared oil returns in a month. In other words, RV is a sum of squared price changes when prices are sampled at high frequency based on daily price data. Hence, realised volatility of oil price on particular day captures uncertainty around the underlying daily closing oil price. This makes realised oil volatility quite a very useful measure of actual change in oil price uncertainty following a policy action. Looking at the recent portion of RV in Figure , there appears to be a spike around 2008 which may be associated with the recent global financial and economic crisis.
3.3. Empirical model
To address the effect of oil price uncertainty shock on the South African economy the study employs the Structural Vector Autoregressive (SVAR) approach. The model provides multivariate structure where variation in a specific variable relates to changes in its individual lags and to variations in other variables. The SVAR approach is one of the effective methods applied to test interdependence relationships among variables and is useful for structural inference. The SVAR model permits the identification of purely exogenous structural shocks with respect to fundamental economic theory to trace dynamic effects, and has better empirical suitability than other VAR classes (Khan & Ahmed, Citation2011), especially in a multivariate framework.
To conclude on the suitable number of lag length of the SVAR model, the Akaike Information Criterion (AIC) and Schwarz Bayesian Criterion (SBC) are used. For policy analysis an appropriate lag length assists in reflecting a long-term effect of variables on others and removing serial correlation and in addition make errors stationary (Sims, Citation1980; Sims, Stock, & Watson, Citation1990). Equally, it is crucial to avoid choosing longer lag length as it results in multi-collinearity hitches and will decrease the degrees of freedom; therefore for this nature of models, thus the SVAR multivariate models, the sequential modified Likelihood Ratio (LR) test propose that the best is lag order 1–3 (Wooldridge, Citation2006).
In the model no great concern is placed on the stationarity of the variables. The SVAR analysis mainly aims to determine an inter-relationship amongst variables not parameter estimates therefore, it may not be crucial to difference variables despite the fact the variables might contain unit root (Sims, Citation1980; Sims et al., Citation1990). Differencing variables might discard key signals relating to the co-movements found in the data (Enders, Citation2004). In addition, (Enders, Citation2004) also argues against detrending the data, particularly if the objective is to estimate the structural model, as the form of variables in the VAR ought to mimic the actual data generating process. In this case, the estimation of the SVAR in levels is fit on condition that the error terms of the separate VAR equations are stationary and serially uncorrelated (McCallum, Citation1993; Parrado, Citation2001). The estimated model could only be estimated in levels given the condition that the error terms in separate VAR equations are stationary and serially uncorrelated. However, as a robustness check we also estimated the model with variables in growth rates.
Following Amisano and Giannini, (Citation1997) and, Khan and Ahmed (Citation2011) the SVAR is specified as:
and
Where,
is a
vector of
variables included in the model,
is a
vector of intercept terms.
’s for all
are
coefficient matrices with
number of lags, which allows the capturing sufficient dynamic interactions between the
variables within the model. Structural error terms
are a
vector, assumed normally distributed with mean of zero plus mutually orthogonal thus diagonal variance covariance matrix
is an identity matrix.
is an invertible
vector of coefficients of contemporaneous relations between all endogenous variables comprising of ones on the diagonal axis and important in the identification process. Similarly,
is
vector of structural coefficients indicating effects of structural shocks.
However, the fundamental problem with equation (2) is that it cannot be estimated and observed directly to obtain specific values of and coefficients
and
. The standard form of equation 2 can be derived by pre-multiplying the structural VAR in equation 2 with
represented below.
Where, and
(
) represents the standard form VAR residuals vector uncorrelated with variables
. Forecast error
is normally and independently distributed and the residual variance covariance matrix
. Consequently, ordinary least squares (OLS) estimation provides consistent estimates of
and the estimates of
can be derived from the fitted residuals.
The VAR equation only specifies lagged terms on the right-hand side, therefore the standard form VAR in equation (4) is incapable of displaying the contemporaneous relationship between variables which causes cross correlation between residual series. Tang, Libo, and Zhang (Citation2010), specifies that while covariance matrix of residuals, does not affect the efficiency and unbiasedness characteristics of estimation, the contemporaneous link can have an impact on the impulse responses. Since equation (2) is not directly observable, the answer is acquired through an alternative relation between the structural VAR equation (2) and the reduced form VAR equation (4) as:
Applying the relations in (5), the structural coefficient in equation (2) can be recovered from the standard form equation (4). The residuals are presumed to be linearly linked to structural shocks, such that
, where forecast errors
are linear combinations of the structural shocks. To recover structural shocks and parameters it is crucial to impose identifying restrictions on matrix
and
, thus
based on economic intuition, using reduced form estimation. Given the
unknown elements in
and
, it is objective to impose
restrictions. In addition, Amisano and Giannini (Citation1997) specify that additional
restrictions on matrix
are required. In this case, there are seven variables in the model; therefore, 21 additional restrictions are necessary for the model to be estimated.
The reduced form VAR is multiplied by and
matrices to get the structural model shocks and the contemporaneous relations among variable. Thus
is equivalent to an identity matrix, as a result the structural parameters can be uncovered. Since industrial production and trade balance is not contemporaneously affected by financial variables, a lower triangular matrix appears to be more consistent with economic theory. Once convergence is obtained and the estimated output being displayed in the VAR window, there is need to compute an LR test to check over-identification.
Another way of analysing the role of uncertainty is by analysing the symmetry of the response functions to positive and negative oil price changes (Jo, Citation2014). The impulse-response analysis is conducted to evaluate the short-run dynamics of the variables by tracing out the effect of realized volatility shock on the economy. Variance decompositions are also analysed to track the transmission channels.
4. Results
The preliminary results of the VAR estimates indicate that the residuals are stationary as shown in Figure and also not serially correlated. The stability condition is also satisfied and no roots lie outside the unit circle.Footnote1 Although our preliminary VAR results show a significant negative effect of realised volatility on South Africa’s industrial production, and a rise in inflation and interest rates, a critical analysis in relation to the sign relationships and exactly how long the effect of oil price uncertainty shock remain effective through the system on macroeconomic variables is warranted.Footnote2 The variance decomposition and impulse response functions are very suitable in order to observe these relationships.
In relation to the impulse responses and the variance decompositions, it is frequently observed that the outcomes of these tests remain sensitive to the ordering of the variables. The ordering chosen in this paper is; realised volatility, industrial production, trade balance, M2, interest rate, exchange rate, and inflation. However, to avoid the problems that may come with ordering of variables the mean of Generalised Impulse Response Functions (GIRF) analysis and Generalised Forecast Error Variance Decompositions (GFEVDs) analysis are used in the SVAR analysis.
The SVAR model is estimated in levels of the variables, using 8 lags. On the basis of the Akaike Information Criteria (AIC) 3 lags were suggested but though could not be used in the model as it was observed to be too small resulting in residual autocorrelation in a number of equations. Therefore, following Bashar et al. (Citation2013) the study utilises lag order of 8 which is found to be smallest lag order that resolves residual correlation. About 21 restrictions are imposed to identify the model. The contemporaneous short run restrictions on SVAR on levels are imposed in-order to get the coefficients. The coefficients indicate that oil price uncertainty has a significant negative impact on industrial production and inflation. This is consistent with the theoretical expectations of disruption to the general price level and investments that may discourage firms to produce.Footnote3 The discussion that follows focuses on the two main tools of analysis consistent with existing literature on SVAR: impulse responses and the variance decompositions.
In examining the short run dynamics, the study employs the Generalised Impulse Response Functions suggested by Pesaran and Shin. (Citation1998). The GIRFs appears more suitable in contrast with Sims’s (Citation1980) orthogonalised impulse response functions, since they do not change with the ordering of the variables (Galesi & Lombardi, Citation2009). For each separate equation, a positive standard unit shock is applied on the realised volatility up to a limit of 24 months horizon. The GIRF of each variable to the monthly realised volatility, thus oil price uncertainty shock is displayed in Figure .
The paper only focuses on tracing out the dependent macroeconomic variables’ responsiveness to realised volatility shock in the SVAR; hence other impulses for the system were excluded. From Figure , output proxied by industrial production index, responds negatively to the oil price uncertainty shock and drops immediately after the shock, though still negative. It starts increasing in the second month and drops again in the third month then recovers slowly over the time lags. The impact remained negative, significant and persistent throughout the period. This may imply that when uncertainty exist around oil prices a decline in output is likely to occur due to discouraged and/or delayed investments. Under such uncertain condition, firms would prefer to adopt a wait and see option until perhaps they obtain more information about future cash flow. This is particularly important when these investments are irreversible making capital more risky. As firms delay their investment decisions, this will affect the level of output in the economy negatively.
As expected, inflation rose immediately after the oil price uncertainty shock, but returns to tendency after 3 months lags. It fluctuates a lot in the time lags then in the 13th month dies out slowly. This suggests that the oil price uncertainty shock may cause inflationary pressure on the South African economy. This is not surprising given that oil is one of the important factors of production and also used for distribution of goods and services. Hence uncertainty surrounding oil prices may lead to higher oil prices which will lead to higher production and distribution costs (Basnet & Upadhyaya, Citation2015). The increased costs will be transferred to the consumers in a form of higher consumer prices.
The response of short term interest rate indicates an immediate increase after the oil price uncertainty shock reaching a peak in month 3. It reverts slowly in the fifth month somehow indicating an insignificant response over time but however still indicating an increase on impact. This may be due to the reaction of the monetary policy authority to curb the rising inflation during uncertainty. The money balances (m2) response to oil price uncertainty shock observed is at first positive thus rising above levels of oil price shocks. After a 2 months lag the money balances rose but returns to its tendency. The impact fluctuates around the tendency and declines slowly after month 5 and continues to decline slowly after the 8th month lag. The response of M2 may hint how monetary authorities adversely respond to oil price shocks. Again this may be connected to the increasing inflation in the economy arising from oil price uncertainty shocks. Theoretically, the monetary authority will be expected to reduce the supply of money in such situation.
The immediate response of trade balance to a shock in oil price uncertainty is negative though this became positive within the first two periods which may be due to a temporary postponement of energy imports. The response fluctuates a lot and stabilises over time and after the 17 month lags and remains stagnant up to the 24 months. The immediate fall in trade balance is intuitive. This is because uncertainty arising from oil price volatility may reduce international trade flows since it increases the risks faced by both importers and exporters. Oil price fluctuations can increase uncertainty about the future path of the oil price, causing consumers to postpone irreversible purchases of consumer durable goods and also causing firms to postpone irreversible investments. The reduction in domestic consumption and investment expenditures implies a reduction in aggregate demand, and thus international trade (Bernanke, Citation1983; Bloom, Citation2009; Chen & Hsu, Citation2013). Also another intuitive explanation is that high fuel costs and fuel cost variability induce an increase in transport costs, which will reduce international trade directly (Chen & Hsu, Citation2013). As increase in oil prices increases the cost of imported raw materials and capital goods this will cause trade deficit and consequently trade balance will decline.
The real effective exchange rate appreciates instantaneously subsequent the shocks to realised volatility up to the fourth month. After the fifth month, it declines slowly and revert its tendency in the 7 month and depreciating over the next 24 months. This is in line with the reverting behaviour of real effective exchange rate as it is known to return to its pre shock levels once all prices and wages have adjusted. Moreover, the standard theory of exchange rate determination suggests that an increase in the oil price causes the currency of an oil-exporting country to appreciate as the demand for its currency increases in the foreign exchange market while an increase in oil price depreciates the currency of an oil-importing country because the supply of its domestic currency in the foreign exchange market increases (Basnet & Upadhyaya, Citation2015). It is then not surprising that the Rand depreciates in the long term following an oil price uncertainty shock. Overall, according to Figure , all variables had an immediate response with expected signs to the oil price uncertainty shock and the impulse responses indicate that for most of the variables, the oil price uncertainty shock appear to be persistent. Although, only output and inflation exhibit significant responses, the negative effect of oil price uncertainty on the macroeconomic variables of South Africa is of concern.
The Generalised Forecast Error Variance Decompositions (GFEVDs) analysis is very crucial in a multivariate framework. GFEVDs offer an analysis tool as it point out on the information each variable contributes in explaining the variations in the other variable. They provide the proportion of the movements in the dependent variables as a result of their own shocks, against shocks to the other variables (Rafiq et al., Citation2009), in other words the transmission channels of policy specific shocks spill overs. The results of the GFEVDs over a 24 months forecast horizon shocks are displayed in Table .
Table 1. Descriptive properties of the macroeconomic variables
Table 2. Generalised forecast error variance decomposition of realised volatility
There is a noteworthy contribution of oil price uncertainty shock to output of 5.01% over the 24 months horizon thus oil price uncertainty shock explains between 0.30 and 5.01% variations in domestic output. The same pattern is also observed for the all the variables. Similarly there is a notable contribution to money balances over the 24 months horizon where the oil price uncertainty shock explains between 0.37 and 5.05% variations in money balances. The variations in money supply are theoretically expected as increased volatility may result in reduced money balances over time for an oil importing country.
Moreover GFEVDs displays that inflation rate is significantly affected by the oil price uncertainty shocks. Similarly the contribution of oil price uncertainty shock to inflation is persistent over the months. The results propose that the contribution to inflation is quite significant between 2.77 and 6.25% between 2 month and 24 month forecast horizon. The observed influence of oil price uncertainty shock on inflation implies the quick adjustment of domestic prices in relation to international oil prices when uncertainty is present.
In general, the GFEVDs indicates that the oil price uncertainty shocks contribute substantially to variations in inflation, real output and various macroeconomic variables of South Africa, and persistent impact is observed over the longer forecast horizon. Therefore, SVAR analysis reveals the significant role of exogenous oil prices on the economy of South Africa when price uncertainty shocks exist.
Our results thus far indicate that the economy is significantly affected by the international oil price uncertainty shocks. To investigate the robustness of our results, we re-estimate the SVAR using alternative volatility measures, variable growth rates and incorporating an adjusted sample period. Given that RV seems to exhibit a break in 2008M12 we ran a robustness check using data from 2009 to 2015. These results are presented in Figure and confirm the adverse effect of oil price uncertainty shock. We also use the growth rates of all the variables as a second robustness check since the variables have unit root; for the full sample ranging from1990 to 2015. As seen in Figure , the conclusions are qualitatively similar to the case of level variables. As a third check we use the CBOE Crude Oil ETF Volatility Index (OVX) instead of the realized volatility also with sample ranging from 2009 to 2015. The results as depicted in Figure though not as precise as the realized oil price volatility measure seems to still support the adverse effect of oil price uncertainty shock. Overall, all the robustness results still indicate the expected immediate response to the oil price uncertainty shock for most of the variables.
5. Conclusion and policy implications
The study empirically examines the short run effects of oil price uncertainty shock on the macroeconomic variables of South Africa from January 1990 to December 2015. The SVAR methodology is applied incorporating realized volatility as an indicator of oil price uncertainty.
The generalised impulse response analysis reveals that for most of the variables, the oil price uncertainty shocks proxied by realised volatility respond persistently and the impact is significant for output and inflation. It is also visible in the results that oil price uncertainty shocks dampen output due to the postponement of irreversible investments by firms. The GFEVDs analysis also points out that oil price uncertainty significantly contributes to changes in real output and various macroeconomic variables of South Africa with minor impact on interest rate where a marginal contribution is realised. The results additionally suggest that oil price uncertainty shocks transmit mostly through industrial production, inflation, trade and money balances and the exchange rate channels creating significant pressure on South Africa’s economic activities.
The findings have important policy implications. South African industries greatly benefit from the locally mined coal which accounts for about 70% of the total energy whilst the imported crude oil accounts for about 15% of the aggregate energy used in South Africa (Department of Minerals and Energy, Citation2006). However, as a net crude oil importer the impact on output is quickly noticed because the country imports vast amounts of crude oil which creates a spill over effect on the economy. . Although the fluctuations in world oil prices cannot be controlled at domestic level, South Africa as a country should adopt such policies that can curb the adverse effects of oil price uncertainty shocks. Policy makers should always be alert on matters linked to oil price uncertainty so that they can be able to manage expectations of economic agents in order to steer expected outcomes of the South African economy. The expected outcomes include more emphasis in the prudent macroeconomic policy to deal with output boost when oil price uncertainty exists. Moreover, given the significant impact of oil price uncertainty shock on inflation, South African monetary authority should be more vigilant in their price stabilization policies to curb this effect. Furthermore, since South Africa depend more on non-renewable sources; renewable energy sources could possibly be an essential option to hedge oil price uncertainty shocks. The country for instance can increase developmental efforts towards hydropower, wind, solar and waste energy investments. Other policy decisions may include transitional arrangements and energy subsidy reforms. Regulations, standards and targets may be implemented more rigorously and effectively to enhance energy efficiency. The need for a policy coordination, not only the macroeconomic and regulatory but also social policy could help to reduce external shocks such as the oil price uncertainty shocks.
Additional information
Funding
Notes on contributors
Junior T. Chiweza
Junior Chiweza is a graduate with a Master’s degree in Economics from the University of Pretoria. She also holds an Honours degree in Economics and undergraduate degree in Business Management and Economics. Her overarching research interests are around Growth and Development, Monetary Theory and Policy, and Applied Econometrics. Recently, her focus has been around evaluating the impact of uncertainty examining context based interventions aimed at improving energy policies by exploring the challenges facing South Africa.
Goodness C. Aye
Dr. Goodness C. Aye holds a PhD in Agricultural Economics from University of Pretoria. She obtained her Bachelors and Master’s degree in the same field. She is a Senior Lecturer and Researcher with interest in agricultural and economic policy analysis, financial markets, development, energy and environmental economics. She has published widely, presented papers in conferences in many continents, a reviewer and editor for high ranking journals. She teaches and supervises both undergraduate and graduate students.
Notes
1. This justifies proceeding with the variables in levels. However, in section 4.3 robustness check is performed using the growth rates of variables.
2. These results are available from the authors upon request.
3. Results of the contemporaneous structural coefficients are available from authors on request.
References
- Ahmed, H. J. A., & Wadud, I. M. (2017). Oil price volatility and sectoral returns uncertainties: Evidence from a threshold based approach for the Australian equity market. The Journal of Developing Areas, 51(1), 329–342. doi:10.1353/jda.2017.0018
- Amisano, G., & Giannini, C. (1997). Topics in structural VAR econometrics (2nd ed.). Berlin: Springer.
- Andersen, T. G., Bollerslev, T., Diebold, F. X., & Labys, P. (2001). The distribution of realized exchange rate volatility. Journal of the American Statistical Association, 96(453), 42–55. doi:10.1198/016214501750332965
- Andersen, T. G., Bollerslev, T., Diebold, F. X., & Labys, P. (2003). Modeling and forecasting realized volatility. Econometrica, 71(2), 579–625. doi:10.1111/ecta.2003.71.issue-2
- Andersen, T. G., #x0026;Benzoni, L. (2009). Realized volatility. In T. G. Andersen, R. A. Davis, J. -P. Kreiss, Th. V. Mikosch (Eds.), Handbook of financial time series (pp. 555–575). Berlin, Heidelberg: Springer.
- Aye, G. C. (2015). Does oil price uncertainty matter for stock returns in South Africa? Stock returns. Investment Management and Financial Innovations, 12(1), 179.
- Aye, G. C., Dadam, V., Gupta, R., & Mamba, B. (2014). Oil price uncertainty and manufacturing production. Energy Economics, 43, 41–47. doi:10.1016/j.eneco.2014.02.004
- Bashar, O. H., Wadud, I. M., & Ahmed, H. J. A. (2013). Oil price uncertainty, monetary policy and the macroeconomy: The Canadian perspective. Economic Modelling, 35, 249–259. doi:10.1016/j.econmod.2013.07.007
- Başkaya, Y. S., Hülagü, T., & Küçük, H. (2013). Oil price uncertainty in a small open economy. IMF Economic Review, 61(1), 168–198. doi:10.1057/imfer.2013.5
- Basnet, H. C., & Upadhyaya, K. P. (2015). Impact of oil price shocks on output, inflation and the real exchange rate: Evidence from selected ASEAN countries. Applied Economics, 47(29), 3078–3091. doi:10.1080/00036846.2015.1011322
- Baumeister, C., & Kilian, L. (2016). Forty years of oil price fluctuations: Why the price of oil may still surprise us. Journal of Economic Perspectives, 30(1), 139–160. doi:10.1257/jep.30.1.139
- Bernanke, B. S. (1983). Irreversibility, uncertainty and cyclical investment. Quarterly Journal of Economics, 98(1), 85–106. doi:10.2307/1885568
- Birol, F. (2007). World energy prospects and challenges. Asia-Pacific Review, 14(1), 1–12. doi:10.1080/13439000701330528
- Blanchard, O. J., & Gali, J., (2007). The macroeconomic effects of oil price shocks: Why are the 2000s so different from the 1970s? National bureau of economic research (NBER Working Paper Series, No. 13368).
- Bloom, N. (2009). The impact of uncertainty shocks. Econometrica, 77(3), 623–685. doi:10.3982/ECTA6248
- Bredin, D., Elder, J., & Fountas, S. (2011). Oil volatility and the option value of waiting: An analysis of the G-7. Journal of Futures Markets, 31, 679–702. doi:10.1002/fut.v31.7
- Caporale, G. M., Ali, F. M., & Spagnolo, N. (2015). Oil price uncertainty and sectoral stock returns in China: A time-varying approach. China Economic Review, 34, 311–321. doi:10.1016/j.chieco.2014.09.008
- Charfeddine, L., Klein, T., & Walther, T. (2018). Oil price changes and U.S. Real GDP growth: Is this time different? (University of St.Gallen, School of Finance Research Paper No. 2018/18). doi:10.2139/ssrn.3222572.
- Chen, S. S., & Hsu, K.-W. (2013). Oil price volatility and bilateral trade. The Energy Journal, 34(1), 207–229. doi:10.5547/01956574.34.1.9
- Dave, D., & Aye, G. C. (2015). Oil price uncertainty and savings in South Africa. OPEC Energy Review, 39, 285–297. doi:10.1111/opec.2015.39.issue-3
- Dedi, L., Yavas, B. F., & McMillan, D. (2016). Return and volatility spillovers in equity markets: An investigation using various GARCH methodologies. Cogent Economics & Finance, 4, 1–18. doi:10.1080/23322039.2016.1266788
- Demirbas, A., Al-Sasi, B. O., & Nizami, A.-S. (2017). Recent volatility in the price of crude oil. Energy Sources, Part B: Economics, Planning, and Policy, 12(5), 408–414. doi:10.1080/15567249.2016.1153751
- Department of Minerals and Energy, (2006). Digest of South African energy statistics. Director: Energy Planning and Development, Pretoria. Retrieved November 14, 2016 from http://www.energy.gov.za/files/media/explained/2006%20Digest%20PDF%20version.pdf
- Dixit, A. (1992). Investment and hysteresis. The Journal of Economic Perspectives, 6(1), 107–132. doi:10.1257/jep.6.1.107
- Dungey, M., & Pagan, A. (2000). A structural VAR Model of the Australian economy. The Economic Record, 76, 321–342. doi:10.1111/ecor.2000.76.issue-235
- Dutta, A., Nikkinen, J., & Rothovius, T. (2017). Impact of oil price uncertainty on Middle East and African stock markets. Energy, 123, 189–197. doi:10.1016/j.energy.2017.01.126
- Ebrahim, Z., Inderwildi, O. R., & King, D. A. (2014). Macroeconomic impacts of oil price volatility: Mitigation and resilience. Frontiers in Energy, 8(1), 9–24. doi:10.1007/s11708-014-0303-0
- Elder, J. (2018). Oil price volatility: Industrial production and special aggregates. Macroeconomic Dynamics, 22(S3), 640-653.
- Elder, J., & Serletis, A. (2010). Oil price uncertainty. Journal of Money Credit and Banking, 42(6), 1137–1159. doi:10.1111/jmcb.2010.42.issue-6
- Enders, W. (2004). Applied econometric time series. New York, NY: John Wiley and Sons.
- Galesi, A., & Lombardi, M. J., (2009) External shocks and international inflation linkages, a global VAR analysis. European Central Bank. (Working Paper No. 1062).
- Guo, H., & Kliesen, K. L. (2005). Oil price volatility and U.S. macroeconomic activity. Federal Reserve Bank of St.Louis Review, 87(6), 669–683.
- Hamilton, J. D. (1983). Oil and the macroeconomy since World War II. Journal of Political Economy, 91, 228–248. doi:10.1086/261140
- Hamilton, J. D. (1996). This is what happened to the oil price-macroeconomy relationship. Journal of Monetary Economics, 38(2), 215–220. doi:10.1016/S0304-3932(96)01282-2
- Hamilton, J. D. (2003). What is an oil shock? Journal of Econometrics, 113(2), 363–398. doi:10.1016/S0304-4076(02)00207-5
- Jo, S. (2014). The effects of oil price uncertainty on global real economic activity. Journal of Money, Credit and Banking, 46(6), 1113–1135. doi:10.1111/jmcb.2014.46.issue-6
- Khan, M. A., & Ahmed, A. (2011). Macroeconomic effects of global food and oil price shocks to the Pakistan economy: A structural vector autoregressive (SVAR) analysis. The Pakistan Development Review, 50, 491–511. doi:10.30541/v50i4II
- Kilian, L. (2008). Exogenous oil supply shocks: How big are they and how much do they matter for the US economy? The Review of Economics and Statistics, 90(2), 216–240. doi:10.1162/rest.90.2.216
- Kilian, L., & Vigfusson, R. J. (2011a). Are the responses of the US economy asymmetric in energy price increases and decreases? Quantitative Economics, 2(3), 419–453. doi:10.3982/QE99
- Kilian, L., & Vigfusson, R. J. (2013). Do oil prices help forecast U.S. real GDP? The role of nonlinearities and asymmetries. Journal of Business & Economic Statistics, 31(1), 78–93. doi:10.1080/07350015.2012.740436
- Kilian, L., & Vigfusson, R. J. (2011b). Nonlinearities in the oil price-output relationship. Macroeconomic Dynamics, 15, 337–363. doi:10.1017/S1365100511000186
- Killian, L., & Vigfusson, R. J. (2017). The role of oil price shocks in causing U.S. Recessions. Forthcoming. Journal of Money, Credit, and Banking. doi:10.1111/jmcb.12430
- Kim, S., & Roubini, N. (2000). Exchange rate anomalies in the industrial countries: A solution with a structural VAR approach. Journal of Monetary Economics, 45, 561–586. doi:10.1016/S0304-3932(00)00010-6
- Lescarousx, F., & Mignon, V. (2008). On the influence of oil prices on economic activity and other macroeconomic and financial variables. OPEC Energy Review, 32, 343–380. doi:10.1111/j.1753-0237.2009.00157.x
- Luo, X., & Qin, S. (2017). Oil price uncertainty and Chinese stock returns: New evidence from the oil volatility index. Finance Research Letters, 20(C), 29–34. doi:10.1016/j.frl.2016.08.005
- McCallum, B. (1993). Unit roots in macroeconomic time series: Some critical issues. Federal Reserve Bank of Richmond EconomicQuarterly,79(2), 13-43.
- Nkomo, J. C. (2006). The impact of higher oil prices on Southern African countries. Journal of Energy Research in Southern Africa, 17(1), 10–17.
- Parrado, E. (2001) Effects of foreign and domestic monetary policy in a small open economy: The case of Chile. Central Bank of Chile. (Working Papers No. 109).
- Pesaran, M. H., & Shin., Y. (1998). Generalised impulse response analysis in linear multivariate models. Economics Letters, 58, 17–29. doi:10.1016/S0165-1765(97)00214-0
- Pindyck, R. S. (1991). Irreversibility, Uncertainty and Investment. Journal of Economic Literature, 29(3), 1110–1148.
- Plante, M., & Traum, N. (2012). Time-varying oil price volatility and macroeconomic aggregates (Center for Applied Economics and Policy Research Working Paper 2012-002).
- Rafiq, S., Salim, R., & Bloch, H. (2009). Impact of crude oil price volatility on economic activities: An empirical investigation in the Thai economy. Resources Policy, 34, 121–132. doi:10.1016/j.resourpol.2008.09.001
- Ron, A., Kilian, L., & Vigfusson, R. (2013). Forecasting the price of oil. In G. Elliott & A. Timmermann (Eds.), Handbook of economic forecasting (Vol. 2, pp. 427–507). Amsterdam: North-Holland.
- Sims, C. A. (1980). Macroeconomics and reality. Econometrica. Journal of the Econometric Society, 48, 1–48. doi:10.2307/1912017
- Sims, C. A., Stock, J. H., & Watson, M. W. (1990). Inference in linear time series models with some unit roots. Econometrica: Journal of the Econometric Society, 58, 113–144. doi:10.2307/2938337
- Tang, W., Libo, W., & Zhang, Z. (2010). Oil price shocks and their short- and long term effects on the Chinese economy. Energy Economics, 32, S3–S14. doi:10.1016/j.eneco.2010.01.002
- Wooldridge, J. (2006). Introductory econometrics: A modern approach. New York: Thomson South-Western.