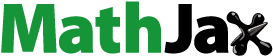
Abstract
This study is intended to identify the predictors of financial distress for the Pakistani firms. Variables used are the financial ratios representing profitability, liquidity, leverage, and cash flows, as well as two important market factors which are size and idiosyncratic standard deviation of each firm’s stock returns (SIG). The sample consists of 290 firms stretching from 2007 to 2016 and logit regression is applied to predict financial distress. The findings reveal that profitability, liquidity, leverage, cash flow ratios, and firm size are significant, while SIG is insignificant in predicting financial distress. Results of the estimated logit model I, model II, and holdout model reveal that the models perform consistently. This study contributes to the literature by testing the market variables in relation to financial distress as these variables were ignored by the previous studies in Pakistan. Findings of this study are precise as the study covers a longer time horizon and a larger sample size.
PUBLIC INTEREST STATEMENT
Financial distress refers to the vulnerable financial position of firms that may result bankruptcy. A large number of firms have shut their operations around the globe due to financial distress. Moreover, the situation is worst in developing countries. Therefore, it is necessary to predict financial distress on early stages. This early prediction may help managers in taking appropriate measures to avoid the potential bankruptcies. Various financial distress prediction models exist in literature that employ important financial ratios and firm-specific market variables to predict financial distress. Financial distress prediction models provide help for the credit rating agencies, debt providers and equity holders to analyze the financial health of the firms.
Competing interests
The author declares no competing interests.
1. Introduction
Predicting financial distress remains an important area of focus for researchers due to its vital importance for the firms and stakeholders including investors, lenders, and participants of capital markets in general. Moreover, the cost of financial distress is high, and it could result in business closure. In such a situation, the financial distress model can serve as a whistle-blowing mechanism to help the corporate managers to initiate remedial measures. Besides, the financial distress model can help the firms to avoid potential bankruptcy.
However, rapid changes in the economy, such as the collapse of the capital market, political instability, and law and order situation, are among the macroeconomic factors that lead firms toward financial distress. For instance, in 2008, 2012, 2014, and 2017, the Pakistan Stock Exchange experienced the worst stock market crisis due to the global financial and economic crises and a political downturn at the country level. The Pakistani equity market is volatile in nature and it results in an uncertain investment environment (Ghufran, Awan, Khakwani, & Qureshi, Citation2016). Therefore, in such an uncertain economic situation of the Pakistan Stock Exchange, predicting financial distress becomes a vital input for providing early signals to the firms.
Bankruptcy, financial distress, and default are the alternative terms used in the literature (Dichev, Citation1998). Financial distress usually refers to a situation where the cash inflows of the firm are not sufficient to meet the daily operational expenses. This situation results in the firm’s failure to meet its financial commitments in the long term. Definitions of financial distress vary across different countries owing to the different accounting treatments and rules. Notwithstanding the various models that exist in the literature to predict financial distress, Z-score and O-score models are more widely used in the financial literature (Adnan Aziz & Dar, Citation2006). Most of the studies on financial distress have been carried out in developed countries such as Australia (Agarwal & Taffler, Citation2008) and the US (Agarwal & Taffler, Citation2008; Altman, Citation1968; Beaver, Citation1966; Dichev, Citation1998; Ohlson, Citation1980; Shumway, Citation2001). It is important to note that the developed countries have different economic structure, clear bankruptcy procedures, and defined laws related to bankruptcy, while the developing and under-developed countries lack such bankruptcy laws and procedures. Hence, predicting financial distress by using the existing well-known Z-score of Altman (Citation1968) or O-score of Ohlson’s logit model (Ohlson, Citation1980) may not work for the developing countries as these models were developed for developed countries. Under such circumstances, as Pakistan is a developing country, it is very important to develop a financial distress prediction model using the data from Pakistan. Following Altman, Iwanicz‐Drozdowska, Laitinen, and Suvas (Citation2017), who stated that one global financial distress model cannot work globally, hence, there is an imperative need to develop a country-specific financial distress model.
Prior literature on financial distress and related theoretical underpinnings are elusive. Therefore, factors affecting financial distress are identified based on their significance in the previous literature. Adnan Aziz and Dar (Citation2006) carried out a meta-analysis on 98 financial distress predictions and argued the importance of financial ratios, namely profitability ratios, liquidity ratios, leverage ratios, and cash flow ratios in predicting financial distress. Shumway (Citation2001) added a few market factors, such as firm size, volatility, and standard deviation of past returns, to predict bankruptcy and found that these factors are significant. Despite the vital importance of market factors in predicting financial distress, previous studies in Pakistan such as by Ijaz, Hunjra, Hameed, and Maqbool (Citation2013) and Rashid and Abbas (Citation2011) had ignored these variables in predicting financial distress among Pakistani firms.
Therefore, this study is intended to fill the gap by incorporating the market factors along with financial ratios to predict financial distress for Pakistani firms. This study will help the corporate managers and other stakeholders to understand the importance of market factors in predicting financial distress. Moreover, this study considers a longer sample horizon of 10 years from 2007 to 2016, while the previous studies used a sample period between five and seven years. Furthermore, this study uses a larger sample of 290 non-financial firms listed on Pakistan Stock Exchange.
This study consists of five sections. In the literature review section, the pertinent literature on financial distress is reviewed to identify the significant predictors of financial distress. Section 3 elaborates the data and methodology for predicting financial distress and the measurement of different variables. This is followed by section reports, descriptive statistics, and empirical findings of the study. The final section is devoted to the conclusion and future research directions.
2. Literature review
Initial studies of financial distress predictions have used financial ratios to predict the financial health of the firms. These financial ratios are easy to access due to their availability in the financial statements of the firms, which are commonly available to the public. The use of financial ratios in discriminating distressed and healthy firms started in the mid-1930s with the work of Winakor and Smith (Citation1935), where they used financial ratios to evaluate the financial soundness of the firms. Even though financial ratios can reflect the financial health of the firm, the quality of these ratios is prone to some serious threats of their usefulness as this information can be manipulated (Beaver, Citation1966; Vladu, Amat, & Cuzdriorean, Citation2017). Beaver (Citation1966) used financial ratios to predict financial distress by using univariate analysis. Subsequently, the popular multivariate discriminant analysis (MDA) model evolved, and Altman (Citation1968) argued that financial ratios measuring profitability, liquidity, and solvency prove significant in predicting financial distress. MDA classifies firms into one of several groups depending on the characteristic of the individual firm. Afterwards, Altman (Citation1968, Citation1973, Citation1984), Altman, Haldeman, and Narayanan (Citation1977), and Altman and Loris (Citation1976) had used the MDA model and stressed the importance of financial ratios in predicting financial distress. Altman (Citation1968), Altman et al. (Citation2017), Beaver (Citation1966), Hill, Perry, and Andes (Citation1996), Ohlson (Citation1980), Shumway (Citation2001), and Xu, Xiao, Dang, Yang, and Yang (Citation2014) had also documented the importance of profitability ratios in predicting financial distress and argued that firms with high profitability face fewer chances of financial distress. Hence, literature designate a negative relationship between financial distress and profitability.
Liquid assets are often helpful for settling the liabilities of a firm. Liquidity refers to a firm’s ability to convert its assets into cash quickly and economically to pay off its financial obligations (Brealey, Myers, & Allen, Citation2011). Many different financial ratios have been used to measure the liquidity of the firm. Popular liquidity ratios are quick assets to current assets (Zavgren, Citation1985), current assets to total liabilities (Agarwal & Taffler, Citation2008), current assets to current liabilities (Yap, Yong, & Poon, Citation2010), and working capital to total assets (Bhunia, Khan, & MuKhuti, Citation2011). Past studies of Campbell, Hilscher, and Szilagyi (Citation2008), Chiaramonte and Casu (Citation2017), Ijaz et al. (Citation2013), Manab, Theng, and Md-Rus (Citation2015), and Zmijewski (Citation1984) articulated the significant importance of liquidity ratios in predicting financial distress and argued that a firm with a high level of liquidity faces less chance of financial distress.
Prior literature also highlighted the importance of the capital structure of the firm in forecasting the probability of financial distress. Beaver (Citation1966) articulated that the probability of financial distress increases as the debt of the firm increases. Firms have the choice to trade-off between the benefits of using debt financing and the cost associated with debt financing (Miller, Citation1977). On one side, the firm can increase the debt portion of their capital structure to minimize the cost of capital, and on the other hand, borrowing beyond an optimal point can increase the risk of bankruptcy as a firm with high debt ratios is more disposed to financial distress. Although various leverage ratios have been used by different researchers, total liabilities to total assets (Bauer & Agarwal, Citation2014), earnings before interest and tax (EBIT) to interest expenses (Her & Choe, Citation1999), and total equity to total liabilities (Altman et al., Citation2017) are among the popular ratios used for predicting financial distress. Altman (Citation1968), Bandyopadhyay (Citation2006), Shumway (Citation2001), and Xu et al. (Citation2014) showed a positive relationship between financial distress and leverage ratios.
Similarly, cash flows are important for the survival of the firm (Beaver, Citation1966). The cash flow theory suggests that a firm will be financially strong if it generates enough cash flow from its operations and will fail when it is unable to generate adequate cash inflow from its operations (Wruck, Citation1990). Many researchers such as Arlov, Rankov, and Kotlica (Citation2013), Beaver (Citation1966), Biddle, Ma, and Song (Citation2016), Gentry, Newbold, and Whitford (Citation1985), Jones and Peat (Citation2014), and Ohlson (Citation1980) stressed the importance of cash flow ratios in predicting financial distress and postulated a negative relationship between cash flows and financial distress.
Although prior literature presented a well-established role of financial ratios in predicting financial distress, a few market variables are important to predict financial distress. Shumway (Citation2001) argued that market-driven variables contain important information which is helpful for predicting financial distress. Shumway (Citation2001) considered three market variables, namely the size of the firm, past returns of the firm, and idiosyncratic standard deviation of past returns and argued that the model consisting of both the accounting ratios and market-driven variables performs well compared to the model that consists of only accounting ratios.
For this study, the financial ratios are chosen based on their popularity and significance in the previous studies. As such, this study chooses net income to total assets (Yap et al., Citation2010), retained earnings to total assets (Hu & Sathye, Citation2015), and EBIT to total asset (Altman et al., 2014) to represent the profitability ratios. Four liquidity ratios including quick assets to current assets, current assets to total liabilities, current assets to current liabilities, and working capital to total assets are also selected. Total liabilities to total assets, EBIT to interest expenses, total debt to total equity, and working capital to long-term debt ratios are used to represent leverage ratios. Moreover, cash flow from operations to total liabilities, cash flow from operations to sales, and cash flow from operations to total assets are used to represent cash flows. For market variables, following Shumway (Citation2001), firm size and idiosyncratic standard deviation of past returns are used.
Although identifying the predictors of financial distress is very important, identifying an appropriate statistical model to predict distress is also imperative. Several statistical models are available in the literature for predicting financial distress including Altman (Citation1968), who used the multiple discriminant analysis (MDA) technique to discriminate the healthy and distressed firms. MDA is a statistical technique used to classify observations into pre-defined groups based on dependent variable’s distinct characteristics. After establishing the groups, MDA drives a linear relationship among the characteristics that discriminate the groups. Altman (Citation1968) concluded that discriminant ratio analysis is a precise measure to differentiate between the bankrupt firms and healthy firms.
Later, Ohlson (Citation1980) criticized the MDA model’s assumption (see Ohlson, Citation1980) and suggested the use of probabilistic logit model to predict financial distress. Logit regression provides a probabilistic score which establishes a non-linear maximum likelihood function. Zmijewski (Citation1984) used the probit regression model to predict financial distress. These two probabilistic models (logit and probit) are widely used in the literature. For example, Bandyopadhyay (Citation2006), Bauer and Agarwal (Citation2014), Schmidt and Duda (Citation2010), and Shumway (Citation2001) used probabilistic regression to predict financial distress. Besides these models, recursive partitioning (decision tree) analysis, the neural network model, and linear probability model are also used to predict financial distress. However, the logit model has remained the most used model for bankruptcy prediction over the globe after 2000 (Adnan Aziz & Dar, Citation2006).
3. Methodology
This study considers the 10 years data from 2007 to 2016 for 290 non-financial firms listed on Pakistan Stock Exchange. The sample period of this study from 2007 to 2016 is selected to provide analysis based on the most recent dataset. Data are collected from the websites of the Securities Exchange Commission of Pakistan and the State Bank of Pakistan. In accordance with the State Bank of Pakistan, a distressed firm is defined as “a firm that has reported a negative value of equity for three consecutive years” (Azizullah, Citation2014). A similar definition was also used by Mateos-Ronco and Mas (Citation2011) and Waqas and Md-Rus (Citation2018) to identify the bankrupt firms. Based on this definition, 45 firms are identified as distressed firms and the other 245 firms are classified as healthy firms. Total annual observations are 2,889 firms-year consisting of 447 distressed firms and 2,442 healthy firms.
Following Altman and Hotchkiss (Citation2010), Bandyopadhyay (Citation2006), and Bunyaminu and Issah (Citation2012), the dataset is divided into two sets. The first set contains data from 2007 to 2013, comprising 2,029 annual observations and is used as the estimation. The second dataset contains data from 2014 to 2016, comprising 860 annual observations and is used as a holdout sample. Variables are used to estimate model I. In estimating model II, those variables that are insignificant in model I are excluded. This is to ensure that the preciseness in the estimation is achieved, as Jones (Citation1987) argued that using too many ratios in a model can actually make the model less useful.
Adnan Aziz and Dar (Citation2006) articulated that the logit regression model is the most used model in predicting financial distress after the year 2000. The logit model was first proposed by Ohlson (Citation1980) to predict financial distress in developed countries. Later, Abdullah, Halim, Ahmad, and Rus (Citation2008), Demirgüç-Kunt and Detragiache (Citation2005), Lin (Citation2009), and Waqas and Md-Rus (Citation2018) used the logit model to predict financial distress for the developing countries. The basic equation of logit regression as cited by Gujarati and Porter (Citation2009) is as follows:
In this equation, is the probability of distress,
is the financial ratio for the firm,
is error term, and
is the coefficient of the financial ratio.
The probability and likelihood function for the non-distressed firms can be defined as follows:
It can be rearranged as
where . Equation (2) represents the logit distribution function.
To estimate the financial distress prediction model, the weights of predictors are calculated by using equation (1). If represents the probability of non-distress which is given in Equation (2), then (
would be the probability of distress. Hereafter,
The following equation is estimated to apply the logit regression.
This study has selected 14 financial ratios and two market factors as the independent variables. These ratios have been found significant in other studies. Financial ratios and market variables, together with their measurements, are presented in Table .
Table 1. Variables used in the model
Since the logit model provides a score between 0 and 1, if the predicted probability is greater than 0.5, this study will classify this observation as distressed, but if the probabilistic score is less than 0.5, the observation will be classified as non-distressed (Gujarati & Porter, Citation2009).
4. Results and discussion
Table reports the mean, standard deviation, and significance of mean differences for the independent variables. The Mann–Whitney U test is applied to examine the significance of differences between the distressed and healthy firms.
Table 2. Means, standard deviations, and significance of differences between distressed and healthy firms
Table provides the mean averages, standard deviation, and significance differences between the means of the healthy and distressed firms. The table reveals that the profitability ratios (NITA, RETA, and ETA) for the healthy firms are higher than for the distressed firms. Liquidity ratios, which measure the firm’s ability to meet its short-term obligations with its current assets, are higher for the healthy firms except for the quick assets to current assets ratio. Leverage ratios are used to analyze the proportion of capital structure financed through debt. Results further show that mean averages of leverage ratios for distressed and non-distressed firms are significantly different. Moreover, the Mann–Whitney U test demonstrates that cash flow ratios of the healthy firms are significantly different from those of the distressed firms. It is revealed that the healthy firms are larger in size with slightly higher SIG. The Mann–Whitney U test demonstrates that all mean average ratios are significantly different for the distressed and healthy firms except for the quick assets to currents asset ratio.
Table provides the correlation analysis among the variables. Except for the correlation between ETA and NITA as well as WCTA and RETA, other variables are not highly correlated.
Table 3. Pairwise correlation for the logit model
To further strengthen the results of the correlation analysis, Variance Inflation Factor (VIF) is used to check for multicollinearity. Table presents the results of VIF.
Table 4. Variance inflation factor results
Gujarati and Porter (Citation2009) and Hair, Black, Babin, and Anderson (Citation2010) stressed that if the VIF values are above 10 (which correspond to tolerance value of less than 0.10), then it indicates the problem of multicollinearity in the data. However, results in Table illustrate that the VIF values for the independent variables are less than the critical value of 10 and this indicates the absence of multicollinearity in the data.
4.1. Results of logit regression for estimation model I
Table reports the results of logit regression (model I) in predicting financial distress.
Table 5. Logit regression results of estimation model I
4.2. Relationship of profitability ratios and financial distress
Table shows that profitability ratios are significant in predicting financial distress. Retained earnings to total assets and earnings before interest and taxes to total assets ratios show a negative relationship with the probability of financial distress. The significantly negative relationship of retained earnings to total assets ratio suggests that as the firm increases its retained earnings, the probability of financial distress decreases. Likewise, earnings before interest and tax expenses to total assets has a significantly negative relationship with the probability of financial distress. This finding suggests that as the EBIT against total assets increases, the probability of financial distress decreases. The net income to total asset ratio is also significant in predicting financial distress. However, the sign of the coefficient is positive, which is in contrast to the previous studies such as Anginer and Yildizhan (Citation2010), Campbell et al. (Citation2008), Cederburg and O’Doherty (Citation2015), Chava and Jarrow (Citation2004), and Lo (Citation1986) while in line with Begley, Ming, and Watts (Citation1996), Beaver (Citation1966), Deakin (Citation1972), Abdullah et al. (Citation2008), Lane, Looney, and Wansley (Citation1986), and Ohlson (Citation1980). This unexpected sign of NITA may arise due to some unusual patterns in the financial statements of the distressed firms as discussed by Lane et al. (Citation1986).
4.3. Relationship of liquidity ratios and financial distress
The results show that current assets to total liabilities (CATL) and working capital to total assets (WCTA) ratios are significant at the 5% level, while quick assets to current assets (QACA) and current assets to current liabilities (CACL) ratios are significant at the 10% level. The results illustrate significantly negative coefficients for working capital to total asset and quick assets to current asset ratios. The negative coefficients depict that a firm with a high liquidity faces fewer chances of financial distress. However, two liquidity ratios (current assets to current liabilities and current assets to total liabilities) exhibit a positive coefficient, which implies that a high liquidity position leads to a higher probability of distress. These results are not expected. Theoretically, this relationship should be negative as literature guides that a firm with a high level of liquidity faces less probability of financial distress. The findings of these two ratios are in contrast to the previous studies by Campbell et al. (Citation2008), Chiaramonte and Casu (Citation2017), and Rashid and Abbas (Citation2011). Both ratios (CATL and CACL) are calculated by using current assets, which comprise inventory along with cash and cash equivalents, accounts receivable, marketable securities, and prepaid expenses. An unnecessary stock of inventory may lead to a high liquidity ratio that can affect the results of the study. When inventory is excluded to calculate the quick assets ratio (quick assets to current assets), the ratio shows a negative sign with the probability of distress, which is in accordance with the past studies. This statement may indicate that the distressed firms possess an excessive amount of inventory, which shows the inefficiency of the firms. Prior studies by Ijaz et al. (Citation2013) and Rashid and Abbas (Citation2011) on Pakistani firms articulated the negative relationship between liquidity and financial distress. The finding of the study implies that the Pakistani firms with high liquidity ratios can face fewer chances of financial distress.
4.4. Relationship of leverage ratios and financial distress
This study has used four leverage ratios to predict financial distress. Results show that total equity to total liabilities (TETL) and working capital to long-term debt (WCLD) ratios are insignificant in predicting financial distress while total liabilities to total assets (TLTA) ratio is significant with a positive coefficient sign. Results further reveal that interest coverage ratio (INTC) has a significantly negative relationship with financial distress. This negative relationship illustrates that a firm with the ability to service its interest payments can reduce the probability of financial distress. This is similar to the findings by Asquith, Gertner, and Scharfstein (Citation1994), Avramov, Chordia, Jostova, and Philipov (Citation2013), Bunyaminu and Issah (Citation2012), and Turetsky and McEwen (Citation2001). Results illustrate that working capital to long-term debt and total equity to total liabilities ratios are irrelevant for the Pakistani firms.
4.5. Relationship of cash flow ratios and financial distress
The results in Table reveal that cash flow from operations to sales (CFOS) ratio is positive and significant. This result is not expected as it illustrates that as the cash flow against sales increases, the probability of distress also increases, which is contrary to the findings by Agarwal and Taffler (Citation2007) and Almamy, Aston, and Ngwa (Citation2016). The other two cash flow ratios, which are cash flow from operations to total liabilities (CFOTL) and cash flow from operations to total assets (CFOTA) ratios, are insignificant in predicting financial distress. These insignificant results raise a question on the usefulness of the cash flow ratios in predicting financial distress. Similar results were found by Casey and Bartczak (Citation1985), Charitou and Vafeas (Citation1998), and Charitou, Neophytou, and Charalambous (Citation2004).
4.6. Relationship of market variables and financial distress
The results demonstrate that the market variable, SIG (idiosyncratic standard deviation of each firm’s stock returns) is insignificant in predicting financial distress. This finding is similar to Aretz, Florackis, and Kostakis (Citation2017) but in contrast to Chava and Jarrow (Citation2004), who found a significant relationship of idiosyncratic standard deviation of each firm’s stock returns with financial distress. The results also illustrate a significantly negative relationship between size and financial distress. As a firm grows in size, it has less probability of defaults. The results are in line with Luqman, Ul Hassan, Tabasum, Khakwani, and Irshad (Citation2018) for Pakistan and Altman Iwanicz-Drozdowska, Laitinen, and Suvas (Citation2017), Ohlson (Citation1980), and Shumway (Citation2001). Results of this study on the market variables are similar to Charalambakis and Garrett (Citation2016), who found that market variables are insignificant predictors of financial distress for the developing countries except for the size of the firm.
Overall, net income to total assets (NITA), retained earnings to total assets (RETA), EBIT to total assets (ETA), current assets to total liabilities (CATL), working capital to total assets (WCTA), total liabilities to total assets (TLTA), interest coverage (INTC), cash flow from operations to sales (CFOS), and size of the firm are significant at the 5% level, while quick assets to current assets (QACA) and current assets to current liabilities are significant at the 10% level. The adjusted R2 of the model is 0.60, which shows that 60% of the variation is captured by the model.
4.7. Classification accuracy for model I
Classification accuracy analyzes the ability of the logit model to classify distressed firms as distressed and healthy firms as healthy with precision. Accuracy rate is calculated by using the following formula.
Classification accuracy for model I is presented in Table .
Table 6. Classification accuracy for model I
The classification accuracy table demonstrates that model I yields an overall classification accuracy of 92.2% for the sample firm. Model I predicts the healthy firms as healthy with an accuracy rate of 98.7% and the distressed firms as distressed with an accuracy rate of 57%. The overall accuracy of model I is greater than the 85.6% reported by Ohlson (Citation1980), 83% by Zmijewski (Citation1984), 75% by Shumway (Citation2001), 82.6% by Almamy et al. (Citation2016), and 85% by Wang and Wu (Citation2017).
4.8. Logit regression for model II
Model I shows that some of the variables including total equity to total liabilities, working capital to long-term debt, cash flow from operations to total liabilities, cash flow from operations to total assets, and volatility of returns are insignificant in predicting financial distress and therefore, are not useful in predicting financial distress.
Henceforth, to estimate another model, all insignificant variables are excluded as in the work by Lane et al. (Citation1986). Insignificant ratios are dropped to make the model more accurate and useful, as Jones (Citation1987) argued that using too many ratios can actually make the model less useful. Results of model II are presented in Table .
Table 7. Logit regression results of model II
Results in Table show that all ratios are significant as in model I and the signs of coefficients remain unchanged. The adjusted R2 of the model is 0.59, which is slightly lower than 0.60 of model I.
4.9. Classification accuracy for model II
Classification accuracy analyzes the ability of the logit model to predict distressed firms as distressed and healthy firms as healthy with precision. Classification accuracy for model II is presented in Table .
Table 8. Validation test results for model II
Table presents that the overall accuracy for model II is 92%, which is slightly lower than the 92.2% obtained in model I. Model II classifies healthy firms as healthy with an accuracy rate of 98.5% and distressed firms as distressed with an accuracy rate of 56.1%. Results of the analysis show that either model (I or II) can be used to predict financial distress as the models produce almost similar results. Following the suggestion by Lane et al. (Citation1986) to exclude insignificant ratios from the model to keep the model simple, this study uses model II which is estimated after excluding the insignificant ratios.
4.10. Classification accuracy for holdout model
The holdout sample for the study consists of the dataset from 2014 to 2016. Logit regression is applied to the holdout model to analyze the consistency of model I. Table illustrates the classification accuracy of the holdout sample.
Table 9. Validation test results for holdout model
Table demonstrates the accuracy of the model for the holdout sample. The model classifies 98.5% of the healthy firms as healthy, which is slightly lower than the accuracy rate of model 1 and similar to the classification accuracy rate of model II. Table further shows that the model classifies 59.4% of the distressed firms as distressed, which is slightly higher than the classification accuracy of model I and model II. The overall accuracy of the holdout model is slightly higher than the classification accuracy of model I and model II. Overall, the logit model has provided consistent results for all of the three models.
5. Findings and conclusion
Findings of the study provide that net income to total assets, retained earnings to total assets, and earnings before interest and tax to total assets are important profitability ratios in predicting financial distress. Results stress the importance of liquidity ratios and demonstrate that current assets to total liabilities, working capital to total assets, and current assets to current liabilities ratios are significant in predicting financial distress. Furthermore, total liabilities to total assets and interest coverage ratio are important leverage ratios in predicting financial distress. The results show that out of three cash flow ratios, only cash flow from operations to sales ratio is a significant predictor of financial distress, while market ratios are irrelevant in predicting financial distress except for firm size. Results provide that the big-size firms are less likely to face financial distress as compared to the small-size firms.
The logit model predicted the distressed firms as distressed with an accuracy rate of 56.1% and the healthy firms as healthy with an accuracy rate of 98.5% that corresponded to the overall accuracy rate of 92%. Results of the holdout model showed a slightly superior accuracy rate with an increase of 0.2%. The overall prediction accuracy rate remained almost similar for the holdout model that designated the consistency of our model. The accuracy rate of the current study is higher than the 76.9% reported by Rashid and Abbas (Citation2011) and slightly lower than the 95% reported by Ijaz et al. (Citation2013) for Pakistani firms.
There are certain limitations in this study. First, the sample period of this study is from 2007 to 2016 and the firms are identified as distressed according to the definition of default provided by the State Bank of Pakistan. Second, financial ratios are selected as predictors based on their popularity in the literature. Moreover, this study has applied the logit regression based on its popularity in the literature. Other models like the Hazard Model or Neural Network Model can be used in future studies to compare the accuracy of the models. For the future studies, macroeconomic variables such as political stability, law and order index, and corporate governance indicators may be considered for predicting financial distress.
Additional information
Funding
Notes on contributors
Hamid Waqas
Hamid Waqas is currently perusing PhD (Finance and Banking) at Universiti Utara Malaysia. Earlier, he completed his Masters in Finance. Afterward, He served as Project Manager in an NGO, Sangtani Women Rural Development Organization. Later, he served as Visiting Lecturer in Bahaudin Zakriya University (Layyah Campus). His research interests include financial distress, bankruptcy predicting, portfolio risk management, corporate governance, and financial economics.
Rohani Md-Rus
Rohani Md-Rusis is an Associate Professor of Finance at School of Economics, Finance and Banking, Universiti Utara Malaysia.She received her PhD in Corporate Finance from University of Manchester, UK in 2003. She is specialised in financial distress, corporate governance, and capital structure. She has published many articles in Scopus and international refereed journals and has over 25 years of teaching experience.
References
- Abdullah, N. A. H., Halim, A., Ahmad, H., & Md-Rus. (2008). Predicting corporate failure of Malaysia’s listed companies: Comparing multiple discriminant analysis, logistic regression and the hazard model. International Research Journal of Finance and Economics, 15(2008), 201-217.
- Abdullah, N. A. H., Halim, A., Ahmad, H., & Rus, R. M. (2008). Predicting corporate failure of Malaysia’s listed companies: Comparing multiple discriminant analysis, logistic regression and the hazard model. International Research Journal of Finance and Economics, 15(2008), 201–217.
- Adnan Aziz, M., & Dar, H. A. (2006). Predicting corporate bankruptcy: Where we stand? Corporate Governance: the International Journal of Business in Society, 6(1), 18–33. doi:10.1108/14720700610649436
- Agarwal, V., & Taffler, R. (2008). Comparing the performance of market-based and accounting-based bankruptcy prediction models. Journal of Banking & Finance, 32(8), 1541–1551. doi:10.1016/j.jbankfin.2007.07.014
- Agarwal, V., & Taffler, R. J. (2007). Twenty‐five years of the Taffler z‐score model: Does it really have predictive ability? Accounting and Business Research, 37(4), 285–300. doi:10.1080/00014788.2007.9663313
- Almamy, J., Aston, J., & Ngwa, L. N. (2016). An evaluation of Altman’s Z-score using cash flow ratio to predict corporate failure amid the recent financial crisis: Evidence from the UK. Journal of Corporate Finance, 36, 278–285. doi:10.1016/j.jcorpfin.2015.12.009
- Altman, E. I. (1968). Financial ratios, discriminant analysis and the prediction of corporate bankruptcy. The Journal of Finance, 23(4), 589–609. doi:10.1111/j.1540-6261.1968.tb00843.x
- Altman, E. I. (1973). Predicting railroad bankruptcies in America. The Bell Journal of Economics and Management Science, 4, 184–211. doi:10.2307/3003144
- Altman, E. I. (1984). A further empirical investigation of the bankruptcy cost question. The Journal of Finance, 39(4), 1067–1089. doi:10.1111/j.1540-6261.1984.tb03893.x
- Altman, E. I., Haldeman, R. G., & Narayanan, P. (1977). ZETATM analysis: A new model to identify bankruptcy risk of corporations. Journal of Banking & Finance, 1(1), 29–54. doi:10.1016/0378-4266(77)90017-6
- Altman, E. I., & Hotchkiss, E. (2010). Corporate financial distress and bankruptcy: Predict and avoid bankruptcy, analyze and invest in distressed debt (Vol. 289). Hoboken, NJ: John Wiley & Sons, Inc.
- Altman, E. I., Iwanicz‐Drozdowska, M., Laitinen, E. K., & Suvas, A. (2017). Financial distress prediction in an international context: A review and empirical analysis of Altman’s Z‐score model. Journal of International Financial Management & Accounting, 28(2), 131–171. doi:10.1111/jifm.12053
- Altman, E. I., & Loris, B. (1976). A financial early warning system for over‐the‐counter broker‐dealers. The Journal of Finance, 31(4), 1201–1217. doi:10.1111/j.1540-6261.1976.tb01969.x
- Anginer, D., & Yildizhan, C. (2010). Is there a distress risk anomaly? Corporate bond spread as a proxy for default risk. The World Bank. Development Research Group. Finance and Private Sector Development Team.
- Aretz, K., Florackis, C., & Kostakis, A. (2017). Do stock returns really decrease with default risk? New international evidence. Management Science, 18(2), 22.
- Arlov, O., Rankov, S., & Kotlica, S. (2013). Cash flow in predicting financial distress and bankruptcy. Advances in Environmental Science and Energy Planning, 42(2/3), 421–441.
- Asquith, P., Gertner, R., & Scharfstein, D. (1994). Anatomy of financial distress: An examination of junk-bond issuers. The Quarterly Journal of Economics, 109(3), 625–658. doi:10.2307/2118416
- Avramov, D., Chordia, T., Jostova, G., & Philipov, A. (2013). Anomalies and financial distress. Journal of Financial Economics, 108(1), 139–159. doi:10.1016/j.jfineco.2012.10.005
- Azizullah, K. (2014). Financial statements analysis of companies (non-financial) listed at Karachi Stock Exchange. Karachi: Pakistan Stock Exchange.
- Back, B., Laitinen, T., Sere, K., & van Wezel, M. (1996). Choosing bankruptcy predictors using discriminant analysis, logit analysis, and genetic algorithms. Turku Centre for Computer Science Technical Report, 40(2), 1–18.
- Bandyopadhyay, A. (2006). Predicting probability of default of Indian corporate bonds: Logistic and Z-score model approaches. The Journal of Risk Finance, 7(3), 255–272. doi:10.1108/15265940610664942
- Bauer, J., & Agarwal, V. (2014). Are hazard models superior to traditional bankruptcy prediction approaches? A comprehensive test. Journal of Banking & Finance, 40, 432–442. doi:10.1016/j.jbankfin.2013.12.013
- Beaver, W. H. (1966). Financial ratios as predictors of failure. Journal of Accounting Research, 4, 71–111. doi:10.2307/2490171
- Begley, J., Ming, J., & Watts, S. (1996). Bankruptcy classification errors in the 1980s: An empirical analysis of Altman’s and Ohlson’s models. Review of Accounting Studies, 1(4), 267–284. doi:10.1007/BF00570833
- Bhunia, A., Khan, I., & MuKhuti, S. (2011). A study of managing liquidity. Journal of Management Research, 3, 2. doi:10.5296/jmr.v3i2.574
- Biddle, G. C., Ma, M. L. Z., & Song, F. M. (2016). Accounting conservatism and bankruptcy risk. SSRN Electronic Journal, 52.
- Brealey, R. A., Myers, S. C., & Allen, F. (2011). Principles of corporate finance. (10th edn.) B. Gordon, Ed. New York: McGraw Hill.
- Bunyaminu, A., & Issah, M. (2012). Predicting corporate failure of UK’s listed companies: Comparing multiple discriminant analysis and logistic regression. International Research Journal of Finance and Economics, 94, 6–22.
- Campbell, J. Y., Hilscher, J., & Szilagyi, J. (2008). In search of distress risk. The Journal of Finance, 63(6), 2899–2939. doi:10.1111/j.1540-6261.2008.01416.x
- Campbell, J. Y., & Shiller, R. J. (1988). Stock prices, earnings, and expected dividends. The Journal of Finance, 43(3), 661–676. doi:10.1111/j.1540-6261.1988.tb04598.x
- Casey, C., & Bartczak, N. (1985). Using operating cash flow data to predict financial distress: Some extensions. Journal of Accounting Research, 23, 384–401. doi:10.2307/2490926
- Cederburg, S., & O’Doherty, M. S. (2015). Asset-pricing anomalies at the firm level. Journal of Econometrics, 186(1), 113–128. doi:10.1016/j.jeconom.2014.06.004
- Charalambakis, E. C., & Garrett, I. (2016). On the prediction of financial distress in developed and emerging markets: Does the choice of accounting and market information matter? A comparison of UK and Indian firms. Review of Quantitative Finance and Accounting, 47(1), 1-28.
- Charitou, A., Neophytou, E., & Charalambous, C. (2004). Predicting corporate failure: Empirical evidence for the UK. European Accounting Review, 13(3), 465–497. doi:10.1080/0963818042000216811
- Charitou, A., & Vafeas, N. (1998). The association between operating cash flows and dividend changes: An empirical investigation. Journal of Business Finance & Accounting, 25(1‐2), 225–249. doi:10.1111/1468-5957.00185
- Chava, S., & Jarrow, R. A. (2004). Bankruptcy prediction with industry effects. Review of Finance, 8(4), 537–569. doi:10.1093/rof/8.4.537
- Chiaramonte, L., & Casu, B. (2017). Capital and liquidity ratios and financial distress. Evidence from the European banking industry. The British Accounting Review, 49(2), 138–161. doi:10.1016/j.bar.2016.04.001
- Deakin, E. B. (1972). A discriminant analysis of predictors of business failure. Journal of Accounting Research, 10, 167–179. doi:10.2307/2490225
- Demirgüç-Kunt, A., & Detragiache, E. (2005). Cross-country empirical studies of systemic bank distress: A survey. Washington, DC: The World Bank.
- Dichev, I. D. (1998). Is the risk of bankruptcy a systematic risk? The Journal of Finance, 53(3), 1131–1147. doi:10.1111/0022-1082.00046
- Gentry, J. A., Newbold, P., & Whitford, D. T. (1985). Classifying bankrupt firms with funds flow components. Journal of Accounting Research, 23, 146–160. doi:10.2307/2490911
- Gombola, M. J., Haskins, M. E., Ketz, J. E., & Williams, D. D. (1987). Cash flow in bankruptcy prediction. Financial Management, 16, 55–65. doi:10.2307/3666109
- Gujarati, D. N., & Porter, D. C. (2009). Basic econometrics (5th ed.). New York: Douglas Reiner.
- Ghufran, B., Awan, H. M., Khakwani, A. K., & Qureshi, M. A. (2016). What causes stock market volatility in Pakistan? Evidence from the field. Economics Research International, 2016.
- Hair, J. F., Black, W. C., Babin, B. J., & Anderson, R. E. (2010). Multivariate data analysis. (7th ed). Prentice Hall. doi:10.1016/j.ijpharm.2011.02.019
- Her, Y. W., & Choe, C. (1999). A comparative study of Australian and Korean accounting data in business failure prediction models (No. 1999.07). 41–68.
- Hill, N. T., Perry, S. E., & Andes, S. (1996). Evaluating firms in financial distress: An event history analysis. Journal of Applied Business Research, 12, 60–71. doi:10.19030/jabr.v12i3.5804
- Hu, H., & Sathye, M. (2015). Predicting financial distress in the Hong Kong growth enterprises market from the perspective of financial sustainability. Sustainability, 7(2), 1186–1200. doi:10.3390/su7021186
- Ijaz, M. S., Hunjra, A. I., Hameed, Z., & Maqbool, A. (2013). Assessing the financial failure using Z-Score and current ratio: A case of sugar sector listed companies of Karachi Stock Exchange.
- Jones, F. L. (1987). Current techniques in bankruptcy prediction. Journal of Accounting Literature, 6(1), 131–164.
- Jones, S., & Peat, M. (2014). Predicting corporate bankruptcy risk in Australia: A latent class analysis. Journal of Applied Management Accounting Research, 12(1), 13-26.
- Lane, W. R., Looney, S. W., & Wansley, J. W. (1986). An application of the Cox proportional hazards model to bank failure. Journal of Banking & Finance, 10(4), 511–531. doi:10.1016/S0378-4266(86)80003-6
- Lin, T. H. (2009). A cross model study of corporate financial distress prediction in Taiwan: Multiple discriminant analysis, logit, probit and neural networks models. Neurocomputing, 72(16–18), 3507–3516. doi:10.1016/j.neucom.2009.02.018
- Lo, A. W. (1986). Logit versus discriminant analysis: A specification test and application to corporate bankruptcies. Journal of Econometrics, 31(2), 151–178. doi:10.1016/0304-4076(86)90046-1
- Luqman, R., Ul Hassan, M., Tabasum, S., Khakwani, M. S., & Irshad, S. (2018). Probability of financial distress and proposed adoption of corporate governance structures: Evidence from Pakistan. Cogent Business & Management, 5(1), 1–14. doi:10.1080/23311975.2018.1492869
- Manab, N. A., Theng, N. Y., & Md-Rus, R. (2015). The determinants of credit risk in Malaysia. Procedia-Social and Behavioral Sciences, 172, 301–308. doi:10.1016/j.sbspro.2015.01.368
- Mateos-Ronco, A., & Mas, Á. L. (2011). Developing a business failure prediction model for cooperatives: Results of an empirical study in Spain. African Journal of Business Management, 5(26), 10565–10576.
- Miller, M. H. (1977). Debt and taxes. The Journal of Finance, 32(2), 261–275.
- Ohlson, J. A. (1980). Financial ratios and the probabilistic prediction of bankruptcy. Journal of Accounting Research, 18, 109–131. doi:10.2307/2490395
- Rashid, A., & Abbas, Q. (2011). Predicting bankruptcy in Pakistan. Theoretical & Applied Economics, 18(9), 103-128.
- Schmidt, H., & Duda, M. (2010). Bankruptcy prediction: Static logit model versus discrete hazard models incorporating macroeconomic dependencies/Master Thesis. Lund University, School of Economics and management. Lund, Sweden.
- Shumway, T. (2001). Forecasting bankruptcy more accurately: A simple hazard model. The Journal of Business, 74(1), 101–124. doi:10.1086/jb.2001.74.issue-1
- Turetsky, H. F., & McEwen, R. A. (2001). An empirical investigation of firm longevity: A model of the ex ante predictors of financial distress. Review of Quantitative Finance and Accounting, 16(4), 323–343. doi:10.1023/A:1011291425075
- Uğurlu, M., & Aksoy, H. (2006). Prediction of corporate financial distress in an emerging market: The case of Turkey. Cross Cultural Management: An International Journal, 13(4), 277–295. doi:10.1108/13527600610713396
- Vladu, A. B., Amat, O., & Cuzdriorean, D. D. (2017). Truthfulness in accounting: How to discriminate accounting manipulators from non-manipulators. Journal of Business Ethics, 140(4), 633–648. doi:10.1007/s10551-016-3048-3
- Wang, L., & Wu, C. (2017). Business failure prediction based on two-stage selective ensemble with manifold learning algorithm and kernel-based fuzzy self-organizing map. Knowledge-Based Systems, 121, 99–110. doi:10.1016/j.knosys.2017.01.016
- Waqas, H., & Md-Rus, R. (2018). Predicting financial distress: Applicability of O-score and logit model for Pakistani firms. Business and Economic Horizons, 14(2), 389–401. doi:10.15208/beh.2018.28
- Winakor, A., & Smith, R. (1935). Changes in the financial structure of unsuccessful industrial corporations. Bulletin, 51, 44.
- Wruck, K. H. (1990). Financial distress, reorganization, and organizational efficiency. Journal of Financial Economics, 27(2), 419–444. doi:10.1016/0304-405X(90)90063-6
- Xu, W., Xiao, Z., Dang, X., Yang, D., & Yang, X. (2014). Financial ratio selection for business failure prediction using soft set theory. Knowledge-Based Systems, 63, 59–67. doi:10.1016/j.knosys.2014.03.007
- Yap, B. C. F., Yong, D. G. F., & Poon, W. C. (2010). How well do financial ratios and multiple discriminant analysis predict company failures in Malaysia. International Research Journal of Finance and Economics, 54(13), 166–175.
- Zavgren, C. V. (1985). Assessing the vulnerability to failure of American industrial firms: A logistic analysis. Journal of Business Finance & Accounting, 12(1), 19–45. doi:10.1111/j.1468-5957.1985.tb00077.x
- Zmijewski, M. E. (1984). Methodological issues related to the estimation of financial distress prediction models. Journal of Accounting Research, 22, 59–82. doi:10.2307/2490859