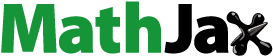
Abstract
This paper aims to examine the relation between idiosyncratic volatility (IVOL) and stock returns with full-sample and conditional alpha sub-samples in Vietnam stock market covering the period from January 2008 to December 2018. We test the IVOL effect on stock returns employing Fama-Macbeth regression method (firm-level analysis) and sorting portfolio method (portfolio-level analysis). In addition, we use different approaches to estimate IVOLs which are the standard deviation of the residuals estimated from regression based on capital asset pricing model (CAPM), Fama-French three-factor model and Carhart four-factor model. We find the IVOL effect which is considered as IVOL puzzle in positive alpha sub-samples. However, we do not discover any significant relation in full-sample and negative alpha sub-samples. Besides, these findings are not consistent with prospect theory. This paper also suggests IVOL opposite strategy for investors to generate significant returns by collecting stocks in positive alpha sub-samples.
PUBLIC INTEREST STATEMENT
In this paper we investigate the link between idiosyncratic volatility and stock returns in Vietnam stock market. We test for the idiosyncratic volatility effect using various approaches. We find that this effect only exists in positive alpha sub-samples. The finding does not support the prospect theory.
1. Introduction
Finance theories have early ignored the role of idiosyncratic risk as important factor in asset pricing model (Lintner, Citation1965; Mossin, Citation1966; Treynor, Citation1961 Citation2016). In addition, Fama and MacBeth (Citation1973) support that there is no relation between idiosyncratic risk and expected returns in efficient market. It also means that portfolios are separated according to idiosyncratic volatility (IOVL) display no difference in its average excess returns; that is defined as return of stocks minus risk-free rate.
By contrast, Merton (Citation1987) indicates that investors are not able to hold the market portfolio in their hand, then they will be noticeable with both total risk and market risk. Therefore, firms with larger total variance (or idiosyncratic risk) yield the higher stock returns to cover risk due to imperfect diversification. Moreover, Morck, Yeung, and Yu (Citation2000) theoretically support that the role of idiosyncratic risk in total risk is considered as a factor to measure effective market level and it has also been treated as an important factor in the research of diversified portfolio.
The IOVL-stock returns relation has attracted considerable attention which is varied based on different perspectives and findings. Until now, it still could be conflicting to indicate clearly whether IOVL tends to have a positive, negative, insignificant or conditional impact on stock returns. While some studies show a positive relation between IVOL and average returns (Drew, Marsden, & Veeraraghavan, Citation2007; Fu, Citation2009; Malkiel & Xu, Citation2002; Nartea, Ward, & Yao, Citation2011), several authors such as Ang, Hodrick, Xing, & Zhang, Citation2006, Citation2009; Chen, Jiang, Xu, & Yao, Citation2012; Guo and Savickas (Citation2006); Stambaugh, Yu, & Yuan, Citation2015 and indicate that high IVOL may tend to have low future average returns around world markets. Even a number of studies such as Bali & Cakici, Citation2008; Brockman and Yan (Citation2008); Fang, Wu, & Nguyen, Citation2017 have failed to find any significant IVOL-stock returns relation. More specifically, given the contradictory results related to the impact of IVOL on stock returns, Qu, Liu, and He (Citation2019) add more explanation that there is existence of negative IVOL-abnormal returns relation in portfolio with negative alpha and positive IVOL-abnormal return relation in portfolio with positive alpha, which could be explained under prospect theory by Wang, Yan, and Yu (Citation2017). In addition, Liu, Kong, Gu, and Guo (Citation2019) also give another perspectives of IVOL-stock returns relation by indicating that employing many calculating methods for IVOL could come to a conflicting conclusion for signs of IVOL-expected returns relation.
Our research is conducted to clarify the question about the direction of IVOL-stock returns relation in Vietnam stock market. First and foremost, our research is motivated due to the insufficient documented studies of IVOL-stock returns linkage in Vietnam market. Besides, recently empirical results have been primarily studied in some developed countries while there are missing ones in emerging market. Vietnam is an important emerging market with several unique characterisitcs both in finance and banking area (Vo & Bui Citation2016, Vo Citation2018). In addition, previous studies have found the relation between IVOL and stock returns for full-sample and they could unintentionally ignore the IVOL effect in sub-samples sorted according to abnormal return levels. If our study does not continue testing more in sub-samples, we could not also find significantly negative linkage in positive sub-samples based on alpha levels; that are abnormal returns computed from capital asset pricing model (CAPM) (Lintner, Citation1965; Mossin, Citation1966; Treynor, Citation2016), Fama-French three-factor (FF3) model (Fama & French, Citation1992) and Carhart four-factor (CH4) model (Carhart, Citation1997). Result implied from sorted sub-samples could be related to prospect theory (He & Zhou, Citation2014; Qu et al., Citation2019). This behavioural theory indicates that if investor could view expected returns based on sign of abnormal returns as the reference point, they become risk-averse investors in profit domain and risk-seeking ones in loss domain, which leads to positive IVOL-stock returns relation and negative IVOL-stock returns relation, respectively. Therefore, it is implied that behavioural rules rather than rational finance ones are possibly explain the abnormal return events. Last but not least, for arbitrage investors, they could decide suitable strategies to form portfolios following to IVOL strategy or opposite IVOL strategy to create significant returns from holding these portfolios. Employing both Fama-Macbeth cross-sectional regression method and sorted portfolios method over the period from January 2008 to December 2018 in Vietnam stock market, the result suggests that there is no relation between IVOL and stock returns in full-sample. However, there still has an existence of IVOL puzzle in sub-samples based on positive alpha levels. In other words, by separating full-sample into sub-samples depending on abnormal return levels, the different patterns are shown for negative IVOL-stock returns relation with significantly positive abnormal return levels and insignificantly positive IVOL-stock returns relation with negative abnormal return levels. While IVOL puzzle is consistent with Ang et al. (Citation2006), these results could not be explained by prospect theory (Qu et al., Citation2019; Wang et al., Citation2017). Thus, IVOL effect based on prospect theory is not workable in Vietnam market’s context.
Vietnam stock market is an interesting case to examine some implications of IVOL-stock returns relation. The relation between IVOL and stock returns could not be found due to some unique features of emerging market. In most these markets, systematic risks still play a crucial role when market risk and political risk might be powerful in estimating asset returns (Diamonte, Liew, & Stevens, Citation1996). Besides, systematic risk would also be dominated in the privatization’s progress of emerging stock market (Perotti & Van Oijen, Citation2001). Moreover, investors have ability to select favoured stocks into portfolios to diversify unsystematic risks after liberalization when the efficiency of market information could be improved (Huang, Wald, & Martell, Citation2013).
This research would make some profound contributions to existing literature for three ways. Firstly, we are not able to find any relation between IVOL and stock returns in full-sample for both portfolio method and Fama-Macbeth regression method. These findings support for Brockman and Yan (Citation2008), Bali and Cakici (Citation2008) and Fang et al. (Citation2017) that the IVOL could not be viewed as essential factor in priced asset model to estimate stock returns. Secondly, by dividing full-sample into sub-samples based on abnormal return levels, the finding shows that there is a significantly negative relation between IVOL and stock returns with sub-samples based on positive alpha level. This finding supports Ang et al. (Citation2006)’s theory that IVOL puzzle still remains, especially in sub-samples depending on positive abnormal levels. In addition, there are not supported evidences for prospect theory given in work of Wang et al. (Citation2017) and Qu et al. (Citation2019). Finally, investors could take advantage of acting opposite IVOL strategy to get returns of 0.9146%, 0.9733% and 1.0242% per month by using estimated IVOL from CAPM, FF3 model and CH4 model, respectively.
Our remainder of the paper is organized as following structure. Section 2 reviews literature summary relating to IVOL-return. Section 3 presents data used in our research. Section 4 describes methodology employed to test IVOL-stock returns relation. Section 5 shows the empirical findings about IVOL-stock returns in both portfolio-level and firm-level and section 6 comes to general conclusion of our study.
2. Literature review
Primary literature for investigating the impact of risk on return is mostly under traditional CAPM in the research of Treynor (Citation2016); Lintner (Citation1965) and Mossin (Citation1966). Underlying implications are that expected stock returns have a positive relation with its market betas and it could not be explained by other variables. To put it differently, idiosyncratic risk might not be considered as important factors in asset pricing model. Therefore, original theories show no linkage between idiosyncratic risk and stock returns in diversified portfolios. Nevertheless, these studies could not mention idiosyncratic risk under circumstance that it is difficult for investor to create diversified portfolio due to transaction cost.
Merton (Citation1987) develops asset pricing model related to idiosyncratic risk. Theory convinces that due to insufficient information, investors tend to spend their money into stocks they are likely to understand clearly about characteristics of risk and returns. In other words, investors might not hold many asset classes in their portfolio and they concern about total risk, not only market risk or idiosyncratic risk. Therefore, they could hold an undiversified portfolio and require risk premium covering idiosyncratic risk.
Malkiel and Xu (Citation2002) use data from both individual US stocks and Japanese stocks to study the role of idiosyncratic risk in asset pricing by investigating whether idiosyncratic risk has impact on asset returns. There is an empirical evidence that idiosyncratic risk would positively affect asset returns when investors could not hold the market portfolio. More specifically, the findings indicate that IOVL variable could be more powerful than either beta or size measures in generating stock returns.
To test the relation between idiosyncratic risk (or unique risk) and stock returns for New Zealand stock market, by replicating an approach of Fama and French (Citation1992) and Fama and French (Citation1993), Drew et al. (Citation2007) find the empirical result that portfolios with the highest IOVL tend to have higher average returns than portfolios with the lowest IOVL during the period from 1995 to 2002. Therefore, the findings point out that cross-sectional expected returns could be well explained by IOVL for New Zealand stocks.
In consistence with positive relation above, using stocks’ data listed on the New York Stock Exchange (NYSE), American Stock Exchange (AMEX) and NASDAQ covering the period from July 1963 to December 2006, Fu (Citation2009) examines the cross-sectional relation between average stock returns and the estimated conditional IOVL using the exponential GARCH models. The evidence shows that the estimated conditional idiosyncratic volatilities have an economically and statistically significant positive impact on the expected returns. More specifically, unlike some firm characteristics, the idiosyncratic volatilities would fluctuate through time. Moreover, the finding suggests that a sub-set of small firms tend to have high returns in the month of high IOVL but the high returns reverse in the subsequent month and lead to the result of negative abnormal returns.
Similarly, to examine the impact of idiosyncratic risk on cross-sectional stock returns, Nartea et al. (Citation2011) use the data of five ASEAN markets such as Malaysia, Singapore, Thailand, Indonesia and the Philippines covering the period up to November 2007. Empirical result indicates that there is existence of a positive relation between realized IOVL and 1-month ahead stock returns only for Malaysia, Singapore, Thailand and Indonesia stock market, but not in the Philippines. The findings also reveal that it is significant to make trading strategy of holding stocks with long high and short low LIV to create profits in Malaysia, Singapore, Thailand and Indonesia.
By contrast, Guo and Savickas (Citation2006) follow FF3 method to estimate IOVL and argue that the relation between expected future stock returns and IOVL is significantly negative by using stocks’ data in Center for Research on Security Prices (CRSP) stock files which span from July 1962 to December 2002.
Supporting this sign of IVOL-stock returns relation, Ang et al. (Citation2009) investigate the impact of IOVL on expected average returns in 23 developed markets (including G7 countries) covering the period from January 1980 to December 2003 for international markets and the period from July 1963 to December 2003 for more detailed study in US. IOVL is calculated for local, regional or world versions referred to the Fama and French (Citation1992) and Fama and French (Citation1993) factor model. After sorting data of 23 countries according to the past IOVL and controlling for market, size and book-to-market factors between stocks, the difference in alphas in the highest quintile of IOVL and stocks in the lowest quintile of IOVL is a high minus level per month. In other words, there is a negative relation between IOVL and expected average returns, which could be observed worldwide.
This negative sign of IVOL-stock returns relation could be supported by the study of Chen et al. (Citation2012). By using stocks’ data (containing common stocks and non-common stocks) in CRSP database covering the period from 1963 to 2010, the findings suggest that IVOL anomaly is an expected event of common stock that could be not display market micro-structure noise. Moreover, the coefficient of IVOL with stock returns is significantly negative when employing Fama-Macbeth regression.
To explain the negative IVOL-stock returns linkage viewed as a puzzle in the study of Ang et al. (Citation2009), Stambaugh et al. (Citation2015) suggest that higher IVOL leads to higher arbitrage risk and causes greater mispricing. Therefore, a negative (positive) IVOL-stock returns relation could be among over-priced (under-priced) stocks. In term of arbitrage asymmetry, the negative relation in over-priced stocks is stronger so the overall relation between IVOL and stock returns is negative.
In the research of Brockman and Yan (Citation2008), an insignificant relation between average IOVL and 1-month ahead excess market returns exists in the sample of 37-year holdout of returns covering the period 1926 to 1962. Bali and Cakici (Citation2008) also examine the relation between IOVL and expected stock returns using data of NYSE, AMEX and NASDAQ financial and non-financial firms during the period from July 1958 to December 2004. The result shows no linkage between IOVL and expected stock returns with monthly data for all breakpoints and weighted schemes.
In the study of Vietnam context, Fang et al. (Citation2017) examine the impact of IVOL on raw returns for both portfolio-level and firm-level. The result shows the insignificant relation between IVOL and stock returns after controlling for the 2008 financial crisis and other predictive variables such as size, BM, REV and MOM.
Perhaps most interesting, as studied by Qu et al. (Citation2019), when using sub-samples of all Chinese A-share firms in Shanghai and Shenzhen Stock Exchange ranked by alpha levels and IVOL covering the period from January 2000 to December 2015, the results point out that there is a negative (positive) IVOL-abnormal returns in portfolio with negative (positive) alpha levels. This finding supported by prospect theory in the research of Wang et al. (Citation2017) rules out explanation that the negative relation between idiosyncratic risk and stock returns is more identified among firms in which investors deal with prior losses.
To give more evidence about this conditional sign of IVOL-stock returns relation, Liu et al. (Citation2019) by using all A stocks of Shanghai and Shenzhen Stock Exchange covering the period from 1997 to 2016 show that a significantly positive relation between IVOL and expected return still exists when employing Fama-French five-factor model to compute IVOL. However, when using GARCH (1,1) model to compute IVOL, the finding shows no significant IVOL-stock returns relation. As a result, in Chinese stock market, relying on the method to calculate IVOL might lead to different sign of the relation between IVOL and expected returns. Therefore, IVOL matter is defined as phenomenon.
For findings argued recently, there still have conflicting results about relation between IVOL and stock returns. While many studies find a positive evidence between IVOL and stock returns either the firm-level or the portfolio-level, this linkage has been shown insignificant, and sometimes negative or even conditional. Whether idiosyncratic risk has an impact on the expected stock returns is still puzzling.
3. Data and methodology
3.1. Data
In our study, we use sorting method and Fama-Macbeth regression method to discover the linkage between IVOL and stock returns. Database collected from Vietnam stock market during the period from January 2007 to December 2018 covers the official estimated period from February 2008 to December 2018. We measure MOM0212, for example, in month January 2008 by computing cumulative returns from January 2007 to December 2007. In addition, IVOL in February 2008 is calculated by using MKT, SMB, HML and WML in January 2008. We also exclude firms for missing financial report data and remove financial firms due to its unique characteristics (Gu, Kang, & Xu, Citation2018).
3.1.1. Idiosyncratic volatility and abnormal returns measure
According to Ang et al. (Citation2006), we firstly employ three models such as CAPM, FF3 and CH4 to calculate daily and monthly time series value of IOVL.
For each day d, in order to calculate daily IVOL, we run time series regressions with respect to three asset pricing models as follows:
Where is raw return of stock i in day d.
is the risk-free rate of government bond with 10-year maturity.
is MKT factor; that is the daily excess return of market portfolio over risk-free rate of government bond with 10-year maturity.
is the intercept to calculate time series value of abnormal return. These abnormal return levels are employed to sort full-sample into negative and positive sub-samples.
is the market return computed based on Vietnam index in day d.
,
,
,
are the intercepts such as coefficient factors of market return premium, size premium, value premium and momentum premium, respectively.
is the residual error in day d.
is computed as daily returns of the difference between lowest portfolio and the largest portfolio sorted by size (market capitalization);
is calculated as daily returns of the highest portfolio minus lowest portfolio ranked by book-to-market ratio and
is defined as daily premium between winners and losers. For the period t, stocks are ranked based on size into small-MC and big-MC portfolios; then sorted into three groups HIGH-BM, MID-BM and LOW-BM portfolios based on book-to-market ratio in order to create total six intersection portfolios including S/H, S/M, S/L, B/H, B/M and B/L. Besides, stocks are also ranked by size and momentum to form six intersection portfolios including S/W, S/M, S/L, B/W, B/M and B/L. We calculate MKT, SMB, HML and WML as reported in Table .
Table 1. Description of variables
We continuously compute the standard deviation of daily residual to obtain monthly IOVL in each CAPM, FF3 model and CH4 model:
With reference to Fu (Citation2009), we demand the daily returns of minimum 14 trading days for a month in Vietnam stock market and exclude the stock with daily non-zero trading volume to compute IVOL. Due to the day off for Vietnamese lunar new year, there are 14 trading days, for example, in February, 2015. Besides, from 1st January (2007) to 31st December (2018), months with 14 days of non-zero trading volume account for 2.21% observations. Therefore, we require the minimum 14 trading days that have volume to remain the consistency of data series.
3.1.2. Control variables
When regressing the FF3 and CH4 models, it is vital to contain several firm’s features defined as control variables. All these variables are computed in monthly frequency as follows.
Size is estimated as natural logarithm of the market capitalization (in Vietnam Dong) at the end of the month. Book-to-market ratio (BM) is the fiscal year-end book value equity divided by calendar year-end market value of equity. MOM0212 is calculated as the cumulative return from month t-12 to month t-2. TURN is the ratio of total trading volume to the share outstanding of equity in a month and REV is the return in previous 1 month. Control variables are shown in Table .
We add these predictive variables into regression to examine whether the IVOL-stock returns relation is driven by other firm characteristics. Moreover, we also give more information about the significant coefficient in regression of IVOL-stock returns that could be held regardless of whether control variables are included.
3.2. Research methodology
3.2.1. Sorting method summary
The critical purpose of this study is to investigate the impact of IVOL (calculated from three model regression such as CAPM, FF3 and CH4) on stock returns in full-sample and two different stock samples such as negative abnormal return and positive abnormal returns sub-samples (based on negative and positive alpha levels). In the scope of this study, we employ sorting methods including bivariate and univariate portfolio-level sorting method to form portfolios in research of Jegadeesh and Titman (Citation1993) and Fama and French (Citation1993).
Firstly, at the beginning of each month according to each control variable including size, BM and MOM, by bivariate portfolio-level sorting method, we divide stocks into total 12 sub-samples to estimate the daily time series value of factors such as SMB, HML and WML, respectively.
Secondly, by univariate portfolio-level sorting method, we form stock’s portfolios based on IVOL with structured pattern for quantile of the number of stocks with reference to the research of Chaves (Citation2016); Chen, Kim, Yao, and Yu (Citation2010); Iihara, Kato, and Tokunaga (Citation2004); Kang, Liu, and Ni (Citation2002);
Lin, Ko, Feng, and Yang (Citation2016); Nartea, Kong, and Wu (Citation2017); Naughton, Truong, and Veeraraghavan (Citation2008) and Schiereck, De Bondt, and Weber (Citation1999) test the significant sign of IVOL-stock returns relation for different returns between the highest of first quantile and the lowest fifth quantile portfolios. Besides, before using robustness test by Fama-Macbeth regression, we sort data depending on alpha levels into two sub-samples (negative level and positive alpha level) to investigate further findings of IVOL-stock returns within different pattern of alpha levels. Thirdly, to examine conditional signs in relation between IVOL and stock returns for different sub-samples, we employ bivariate portfolio-level sorting method to rank full-sample into sub-samples based on alpha level and then IVOL.
3.2.2. Cross-sectional Fama-Macbeth regression method summary
Fama-Macbeth regression could be able to capture residual’s and other factors’ fluctuation in cross-sectional stock return. Fama-Macbeth regression could also give more power to control for different factor loadings and characteristics in a model while sorting method could cause dispersion with more equal than two ranking dimensions. This problem could remain within portfolio with a few stocks and a lot of noise issue. Taking this approach aims to control the impact of simultaneous firm characteristics on the cross-sectional stock returns.
Following Fama and MacBeth (Citation1973), at the beginning of month, we estimate cross-sectional regression with stock’s monthly return (dependent variable) and control variables to get the time-series value of each estimated factor exposure. The cross-sectional Fama-Macbeth model is specified as follows:
Where is the return of stock i in month t;
is idiosyncratic volatility computed as the standard deviation of residual;
is three factors in the research of Fama and French (Citation1993) and four factors in the research of Carhart (Citation1997) including
(value-weighted market return),
(small minus big),
(high minus low),
(winner minus loser); that are excess return on the market portfolio, monthly premium of market capitalization factor, monthly of book-to-market factor and monthly premium on winners minus losers, respectively;
and
are the estimated factor exposures;
is residual from least squares regression.
After that, we compute the average, variance and t-statistic value of the estimated factor exposures as follows:
The t-test aimed to compare between average of time series value of the estimated factor exposures with zero to realize significant difference.
4. Empirical results
4.1. Description statistics and correlations among variables
Descriptive statistics for alpha and IVOL and variables of firm characteristics are reported in Table .
Table 2. Statistic summary
Table contains the mean, standard deviation, maximum and minimum for all variables. Rate of return, Rm is 0.94% in monthly average. Alpha values in CAPM, FF3 model and CH4 model are 0.04%, 0.03% and 0.04%, respectively. Compared with CAPM and CH4 model, stock returns that could be not explained in FF3 model reach the lowest figure so FF3 model would be able to explain stock return more than CAPM and CH4 model. In addition, IVOL estimated from all models is approximately 0.2% per month.
Table expresses the correlations among variables such as alpha, IVOL for all models and control variables. IVOLCAPM, IVOLFF3 and IVOLCH4 have highly correlation from 0.96 to 0.99. This correlation is nearly equal, which confirms about strong relation among these IVOLs. Therefore, we separate it into independent model to investigate the IVOL-stock returns relation. All variables left have low correlation which gives more evidence to reject multi-collinearity phenomenon.
Table 3. Correlations
4.2. Sorting method
We first investigate the IVOL-raw stock returns relation by using the full-sample. IVOLs are computed from three models such as CAPM, FF3 model and CH4 model. Stocks are divided in five portfolios based on estimated IVOL. As Table reported, a zero-cost equal-weighted portfolio of highest quantile and lowest quantile (also called IVOL strategy) for IVOLCAPM, IVOLFF3 and IVOLCH4 (5_1) is −0.2771%, 0.0636% and −0.0891%, respectively while a zero-cost value-weighted portfolio of highest quantile and lowest quantile for IVOLCAPM, IVOLFF3 and IVOLCH4 (5_1) is −0.0119%, −0.0040% and 0.0062%, respectively. However, all these figures are insignificant, which reflects unclear relation between IVOL and stock returns within full-sample sorted according to IVOL.
Table 4. IVOL-stock returns relation on full-sample for both weighted methods
Table also reports the pattern of IVOL quantile. In CAPM, the raw return of total quantiles reduces from 0.8363% for the lowest IVOL to 0.5592% for the highest IVOL in equal-weighted return method. Therefore, portfolios with highest IVOLCAPM tend to have lower return compared to portfolios with lowest IVOLCAPM. This result is likely to imply a negative IVOL-stock returns relation when employing Fama-Macbeth regression in firm level. This result is consistent with research of Guo and Savickas (Citation2006). For both the equal-weighted and the value-weighted portfolio for FF3 and CH4 models, the stock returns of all quantile levels fluctuate abnormally and absolutely have no trend. Therefore, a basic trading strategy based on IVOL by going short with IVOL of fifth portfolio and long with IVOL of first portfolio could be expected not to yield a significant return. This finding is consistent with the research of Bali and Cakici (Citation2008).
To have a deep more understanding, the relation of IVOL-stock returns in alpha sub-samples (for both negative and positive alpha levels). We separate all stocks into two groups according to the sign of alpha levels. After that, within each sub-sample, we further rank these samples into five portfolios based on IVOL and compute returns by two weighted methods. Within positive alphaCAPM sample (reported in Table ), for both equal-weighted and value-weighted methods, we could find the evidence of the negative significant relation between IVOL and raw stock returns.
Table 5. IVOL-stock returns relation on positive alpha sub-samples
We conclude that with sample formed by alpha in CAPM, portfolios with highest IVOLCAPM tend to have lower return compared to portfolios with lowest IVOLCAPM. Investors could be generating profit by using opposite strategy against IVOL strategy. For more details, it could yield monthly return of 0.91% in equal-weighted and 0.31% in value-weighted method.
The same finding with alphaFF3 and alphaCH4 (only for equal-weighted method), we could find the negative IVOL-raw returns with 10% and 5% significant levels, respectively. Accordingly, the opposite IVOL strategy for alphaFF3 and alphaCH4 could create 0.97% and 1.02% of monthly equal-weighted return. The sign still remains in using value-weighted method but it turns into insignificant for all significant levels. More interestingly, a zero-cost equal-weighted portfolio of highest quantile and lowest quantile for IVOLCAPM, IVOLFF3 and IVOLCH4 (5_1) is −0.9146%, −0.9733% and −1.0242%, respectively. This is nearly the same to the trading profit for Ang et al. sample studied in 23 developed markets (approximately −0.89%). We could conclude that high (low) IVOL, low (high) raw stock returns relation could happen strongly in firm-level rather than in portfolio-level. These results disappear when investigating this IVOLFF3 and IVOLCH4-stock returns relation in negative alpha sample for both weighted methods (shown in Table ). Only case in alphaFF3 sample with value-weighted method, there are positive relation between IVOL and raw returns. It could generate monthly return of 0.0933% for investor when using IVOL strategy. We conclude that with alphaFF3 and alphaCH4 sample, positive IVOL-raw returns relation could be weak both in portfolio-level and in firm-level.
Table 6. IVOL-stock returns relation on negative alpha sub-samples
To sum up, it could not be found strongly significant signs in IVOL-stock returns relation when these signs do not show consistence in studied samples. Therefore, this linkage between IVOL and stock returns is not robust in portfolio-level and testing IVOL-stock returns in statistic regression gives more precise conclusion.
4.3. Fama-Macbeth regression results
To further analyze and test robustness of IVOL-stock returns, covering the period from January 2008 to December 2018, we employ Fama-Macbeth regression to investigate the relation between IVOL and stock returns by controlling other independent variables such as size, BM, MOM0212, REV and TURN. The dependent variable is the monthly return of each stock (in percentage form) during the next month; the month when IVOL is calculated.
The finding in Table reports that for full-sample employing CAPM to estimate IVOL, the coefficient of IVOL is significant at 5% and 10% levels and negative in three-fourth regressions (from model (2) to (4) regression). More importantly, this sign and significance remain consistence regardless of any predictive variables are contained. For instance, the coefficient of IVOL in the model (4) is −0.2531 with significant at 10% level. It could be concluded that IVOLCAPM has a negative impact on stock returns in full-sample. In other words, stocks with past high IVOLCAPM tend to have much lower returns after controlling firm characteristics. We could ignore this valuable finding if using only sorting methods to examine the overall relation between IVOLCAPM and stock returns. Our finding proves that idiosyncratic risk plays a vital role in generating stock returns for the studied full-sample when employing CAPM.
Table 7. IVOLCAPM and stock returns
We continue creating two portfolios of stocks ranked by alphaCAPM (positive and negative alpha sub-samples). We observe the significantly negative sign of IVOLCAPM-raw stock returns relation from model (5) to model (8) in positive alphaCAPM sub-samples. None of portfolios in negative
sub-samples show evidence of IVOL-stock returns relation at any significant levels. All coefficients from model (5) to model (8) are greater and more significant than ones from model (9) to model (12). It could be implied that the negative IVOLCAPM-raw returns relation in full-sample is driven by this relation in positive alpha sub-samples. These findings are consistent with Guo and Savickas (Citation2006), Ang et al. (Citation2006), Ang et al. (Citation2009) and Qu et al. (Citation2019). Using alpha levels estimated from FF3 and CH4 models to form portfolios, Tables and show that there is no significant relation between IVOL and stock returns in full-sample. However, there is still significant evidence for a negative linkage between IVOL and raw returns for positive alpha sub-samples. IVOLFF3 and IVOLCH4 still have a significant negative in positive sub-samples, for example, in three-fourth portfolios in CH4 model and 100% portfolios in FF3 model. It could also find that the absolute value of coefficient IVOL in positive sub-samples are greater than negative sub-samples. Our findings are not supported by the study of Qu et al. (Citation2019) when IVOL-stock returns relation is negative in negative alpha sub-samples and positive in positive alpha sub-samples. However, it is completely consistent with findings from Fang et al. (Citation2017) study when IVOL has no significant relation with stock returns 1-month prior for full-sample by using data of Vietnam stock market covering the period from 2007 to 2014.
Table 8. IVOLFF3 and stock returns
Table 9. IVOLCH4 and stock returns
4.4. The explanatory power of asset pricing model in stock returns and IVOL strategy returns
By employing GRS test from the work of Gibbons, Ross, and Shanken (Citation1989), we could examine the explanatory power of asset pricing models in stock returns based on IVOL quantile and IVOL strategy returns in the Vietnam stock market. Basic models such as CAPM, FF3 model and CH4 model are employed to investigate this explanatory power. We sort full-sample into sub-samples based on IVOL quantile with both equal- and value-weighted themes and then run regression of factors and stock returns to evaluate the explanatory power of each model based on the results of R2 and p value of alpha. Testing results for IVOLCAPM, IVOLFF3 and IVOLCH4 predictability is similar so we only report the results of IVOLFF3 portfolios, but these findings are available upon request.
Table shows the GRS test results of equal-weighted IVOL portfolios, and Table expresses the GRS test results of value-weighted IVOL portfolios. As reported in Table , p values in GRS test and mean value of alphas for all models are insignificant. Thus, we have a strong evidence to confirm that all asset pricing models could explain effectively for returns of equal-weighted IVOL portfolios. Besides, mean value of alpha in (5_1) portfolio is insignificant in all models, these results indicate that models also have strong explanatory power to predict IVOL strategy returns.
Table 10. The explanatory power of asset pricing model for stock returns based on IVOL-sorted portfolio with equal-weighted method and IVOL strategy return
Table 11. The explanatory power of asset pricing model for stock returns based on IVOL-sorted portfolio with value-weighted method and IVOL strategy return
In addition, FF3 model has the lowest value alpha in average compared with CH4 model and CAPM so FF3 model’s predictability is more efficient than CH4 model and CAPM. The highest ranges of average R2 for FF3 model in explaining equal-weighted IVOL portfolios are from 78.9% to 87.5% implying that FF3 model’s explanatory power is the best for estimating returns in each quantile of IVOL. Besides, the average R2 for FF3 model in explaining IVOL strategy is also the best with approximately 28%. By contrast, as reported in Table , p values in GRS test in all models for IVOL-stock returns and IVOL strategy are significant, so that neither models could explain the returns of value-weighted IVOL portfolios. However, the explanatory power to IVOL strategies have similar the results in Table . To sum up, these results are slightly different from research of Fang et al. (Citation2017) in the same context of Vietnam that FF3 and CH4 could well explain the returns for both weighted schemes but our result shows this explanatory power in equal-weighted method only.
5. Conclusion
Huang et al. (Citation2013) suggest that idiosyncratic risk is of less significance in priced stocks of emerging stock market, especially after liberalized process. Investors have more chances to collect stocks into diversified portfolios so idiosyncratic risk is likely to disappear from their portfolio. But there is an argument that idiosyncratic risk could be included in diversified selection of portfolios. Thus, idiosyncratic risk possibly plays a vital role in priced factor; however, this risk could not be tested in traditional asset pricing model. In developed markets, empirical evidences indicate that idiosyncratic risk might be priced in estimating stock returns since there is a significant relation between idiosyncratic risk and stock returns. For instance, Ang et al. (Citation2006) and Ang et al. (Citation2009) indicate a negative linkage between the lagged idiosyncratic risk and expected stock returns. This phenomenon could be named as IVOL puzzle that attracts numerous attentions around the world. Hou and Loh (Citation2016) explore some explanations causing to this puzzle, such as investor’s lottery preferences, market frictions and others related to uncertainty as well as finance distress, By contrast, it has not been clear sign of IVOL puzzle in emerging stock markets. Testing IVOL puzzle in Vietnam stock market definitely contribute more empirical evidence to current literature.
Some reasons for IVOL effect in emerging market is that investors lack opportunity to form diversified portfolio due to small market where there are not many stocks to collect from the research of Huang et al. (Citation2013). Moreover, systematic risks still hold dominated position in creating stock returns, thus idiosyncratic risk would be less power to predict stock returns compared to developed market (Diamonte et al., Citation1996). Motivated by the research of Qu et al. (Citation2019) to investigate IVOL-stock returns relation in sub-samples based on alpha levels. After running regression and using sorting method in full-sample, we separate full-sample into negative and positive sub-samples depending on alpha levels to understand more.
Our perspective is that it can be missing to explain IVOL puzzle if we only study in term of full-sample. It is noticed that there is not clear significant existence of IVOL-stock returns relation in portfolio-level by sorting method covering the period from January 2008 to December 2018. When employing regression in positive alpha sub-samples, we could find the significantly negative relation for all models (such as CAPM, FF3 model and CH4 model). This finding indicates that IVOL puzzle in Vietnam stock market especially occurs at firm-level when dividing full-sample into sub-samples according to alpha levels. Our study could show many implications in academic and practical perspectives that could be taken advantage of by investors and researchers. The evidence of IVOL effect on stock returns does not exist in the full-sample in Vietnam stock market but exists the negative significant relation in positive alpha sub-samples. These findings further add more understanding about IVOL-stock returns relation in both portfolio-level and firm-level. For emerging country case as Vietnam, it is obviously important to separate full-sample into sub-samples based on alpha levels to further examine. In addition, we employ traditional asset pricing models such as CAPM, FF3 model and CH4 model because of the reliable predictability in explaining the returns, especially with equal-weighted method. For investors, there still has an opportunity to make opposite IVOL strategy with stocks that are collected from positive alpha sub-samples to create portfolio with significant returns.
Additional information
Funding
Notes on contributors
Xuan Vinh Vo
Xuan Vinh Vo is from the Institute of Business Research, University of Economics Ho Chi Minh City, Vietnam and CFVG Ho Chi Minh City, University of Economics Ho Chi Minh City, Vietnam. He has strong research interest in Economics and Finance.
Email address: [email protected]
Van Phong Vo
Van Phong Vo is from the Institute of Business Research, University of Economics Ho Chi Minh City, Vietnam. He has strong research interest in Economics and Finance.
Email address: [email protected].
Thanh Phuc Nguyen
Thanh Phuc Nguyen is from the Institute of Business Research, University of Economics Ho Chi Minh City, Vietnam. He has strong research interest in Economics and Finance. University of Economics Ho Chi Minh City, Vietnam.
Email address: [email protected].
References
- Ang, A., Hodrick, R. J., Xing, Y., & Zhang, X. (2006). The cross‐section of volatility and expected returns. The Journal of Finance, 61(1), 259–25. doi:10.1111/j.1540-6261.2006.00836.x
- Ang, A., Hodrick, R. J., Xing, Y., & Zhang, X. (2009). High idiosyncratic volatility and low returns: International and further US evidence. Journal of Financial Economics, 91(1), 1–23. doi:10.1016/j.jfineco.2007.12.005
- Bali, T. G., & Cakici, N. (2008). Idiosyncratic volatility and the cross section of expected returns. Journal of Financial and Quantitative Analysis, 43(1), 29–58. doi:10.1017/S002210900000274X
- Brockman, P., & Yan, X. S. (2008, March 1). The time-series behavior and pricing of idiosyncratic volatility: Evidence from 1926 to 1962. Working paper, University of Missouri - Columbia.
- Carhart, M. M. (1997). On persistence in mutual fund performance. The Journal of Finance, 52(1), 57–82. doi:10.1111/j.1540-6261.1997.tb03808.x
- Chaves, D. B. (2016). Idiosyncratic momentum: US and international evidence. The Journal of Investing, 25(2), 64–76. doi:10.3905/joi.2016.25.2.064
- Chen, L. H., Jiang, G. J., Xu, D., & Yao, T. (2012, January 28). Dissecting the idiosyncratic volatility anomaly. Working paper, University of Iowa - Henry B. Tippie College of Business. DOI:10.1094/PDIS-11-11-0999-PDN
- Chen, X., Kim, K. A., Yao, T., & Yu, T. (2010). On the predictability of Chinese stock returns. Pacific-Basin Finance Journal, 18(4), 403–425. doi:10.1016/j.pacfin.2010.04.003
- Diamonte, R. L., Liew, J. M., & Stevens, R. L. (1996). Political risk in emerging and developed markets. Financial Analysts Journal, 52(3), 71–76. doi:10.2469/faj.v52.n3.1998
- Drew, M. E., Marsden, A., & Veeraraghavan, M. (2007). Does idiosyncratic volatility matter? New Zealand evidence. Review of Pacific Basin Financial Markets and Policies, 10(3), 289–308. doi:10.1142/S0219091507001070
- Fama, E. F., & French, K. R. (1992). The cross‐section of expected stock returns. The Journal of Finance, 47(2), 427–465. doi:10.1111/j.1540-6261.1992.tb04398.x
- Fama, E. F., & French, K. R. (1993). Common risk factors in the returns on stocks and bonds. Journal of Financial Economics, 33(1), 3–56. doi:10.1016/0304-405X(93)90023-5
- Fama, E. F., & MacBeth, J. D. (1973). Risk, return, and equilibrium: Empirical tests. Journal of Political Economy, 81(3), 607–636. doi:10.1086/260061
- Fang, K., Wu, J., & Nguyen, C. (2017). The risk-return trade-off in a liberalized emerging stock market: Evidence from Vietnam. Emerging Markets Finance and Trade, 53(4), 746–763. doi:10.1080/1540496X.2015.1103129
- Fu, F. (2009). Idiosyncratic risk and the cross-section of expected stock returns. Journal of Financial Economics, 91(1), 24–37. doi:10.1016/j.jfineco.2008.02.003
- Gibbons, M. R., Ross, S. A., & Shanken, J. (1989). A test of the efficiency of a given portfolio. Econometrica: Journal of the Econometric Society, (1), 1121–1152. doi:10.2307/1913625
- Gu, M., Kang, W., & Xu, B. (2018). Limits of arbitrage and idiosyncratic volatility: Evidence from China stock market. Journal of Banking & Finance, 86(1), 240–258. doi:10.1016/j.jbankfin.2015.08.016
- Guo, H., & Savickas, R. (2006). Idiosyncratic volatility, stock market volatility, and expected stock returns. Journal of Business & Economic Statistics, 24(1), 43–56. doi:10.1198/073500105000000180
- He, X. D., & Zhou, X. Y. (2014). Myopic loss aversion, reference point, and money illusion. Quantitative Finance, 14(9), 1541–1554. doi:10.1080/14697688.2014.917805
- Hou, K., & Loh, R. K. (2016). Have we solved the idiosyncratic volatility puzzle? Journal of Financial Economics, 121(1), 167–194. doi:10.1016/j.jfineco.2016.02.013
- Huang, B., Wald, J., & Martell, R. (2013). Financial market liberalization and the pricing of idiosyncratic risk. Emerging Markets Review, 17(1), 44–59. doi:10.1016/j.ememar.2013.08.005
- Iihara, Y., Kato, H. K., & Tokunaga, T. (2004). The winner–loser effect in Japanese stock returns. Japan and the World Economy, 16(4), 471–485. doi:10.1016/j.japwor.2003.06.001
- Jegadeesh, N., & Titman, S. (1993). Returns to buying winners and selling losers: Implications for stock market efficiency. The Journal of Finance, 48(1), 65–91. doi:10.1111/j.1540-6261.1993.tb04702.x
- Kang, J., Liu, M.-H., & Ni, S. X. (2002). Contrarian and momentum strategies in the China stock market: 1993–2000. Pacific-Basin Finance Journal, 10(3), 243–265. doi:10.1016/S0927-538X(02)00046-X
- Lin, C., Ko, K.-C., Feng, Z.-X., & Yang, N.-T. (2016). Market dynamics and momentum in the Taiwan stock market. Pacific-Basin Finance Journal, 38(1), 59–75. doi:10.1016/j.pacfin.2016.03.009
- Lintner, J. (1965). Security prices, risk, and maximal gains from diversification. The Journal of Finance, 20(4), 587–615.
- Liu, S., Kong, A., Gu, R., & Guo, W. (2019). Does idiosyncratic volatility matter?—Evidence from Chinese stock market. Physica A: Statistical Mechanics and Its Applications, 516(1), 393–401. doi:10.1016/j.physa.2018.09.184
- Malkiel, B. G., & Xu, Y. (2002, November). Idiosyncratic risk and security returns. Working paper, University of Texas at Dallas.
- Merton, R. C. (1987). A simple model of capital market equilibrium with incomplete information. The Journal of Finance, 42(3), 483–510. doi:10.1111/j.1540-6261.1987.tb04565.x
- Morck, R., Yeung, B., & Yu, W. (2000). The information content of stock markets: Why do emerging markets have synchronous stock price movements? Journal of Financial Economics, 58(1–2), 215–260. doi:10.1016/S0304-405X(00)00071-4
- Mossin, J. (1966). Equilibrium in a capital asset market. Econometrica: Journal of the Econometric Society, 1, 768–783. doi:10.2307/1910098
- Nartea, G. V., Kong, D., & Wu, J. (2017). Do extreme returns matter in emerging markets? Evidence from the Chinese stock market. Journal of Banking & Finance, 76(1), 189–197. doi:10.1016/j.jbankfin.2016.12.008
- Nartea, G. V., Ward, B. D., & Yao, L. J. (2011). Idiosyncratic volatility and cross‐sectional stock returns in Southeast Asian stock markets. Accounting & Finance, 51(4), 1031–1054. doi:10.1111/j.1467-629X.2010.00384.x
- Naughton, T., Truong, C., & Veeraraghavan, M. (2008). Momentum strategies and stock returns: Chinese evidence. Pacific-Basin Finance Journal, 16(4), 476–492. doi:10.1016/j.pacfin.2007.10.001
- Perotti, E. C., & Van Oijen, P. (2001). Privatization, political risk and stock market development in emerging economies. Journal of International Money and Finance, 20(1), 43–69. doi:10.1016/S0261-5606(00)00032-2
- Qu, Z., Liu, X., & He, S. (2019). Abnormal returns and idiosyncratic volatility puzzle: Evidence from the Chinese stock market. Emerging Markets Finance and Trade, 55(5), 1184–1198. doi:10.1080/1540496X.2018.1468249
- Schiereck, D., De Bondt, W., & Weber, M. (1999). Contrarian and momentum strategies in Germany. Financial Analysts Journal, 55(6), 104–116. doi:10.2469/faj.v55.n6.2317
- Stambaugh, R. F., Yu, J., & Yuan, Y. (2015). Arbitrage asymmetry and the idiosyncratic volatility puzzle. The Journal of Finance, 70(5), 1903–1948. doi:10.1111/jofi.12286
- Treynor, J. L. (1961). Market value, time, and risk. Time, and Risk (August, 8, 1961.
- Vo, X. V, & Bui, H. T. (2016). Asset growth and the cross section of stock returns-evidence from vietnam. Afro-asian Journal of Finance and Accounting, 6(4), 289-304.
- Vo (2018), M&As in the Process of Banking Consolidation – Preliminary Evidence from Vietnam. Asian Journal of Law and Economics, 9(2), doi:10.1515/ajle-2017-0032
- Wang, H., Yan, J., & Yu, J. (2017). Reference-dependent preferences and the risk–return trade-off. Journal of Financial Economics, 123(2), 395–414. doi:10.1016/j.jfineco.2016.09.010