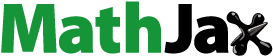
Abstract
This paper aims at contributing to the international portfolio investment decisions among the emerging BRICS countries where individual and institutional investors seek diversification benefits and to help in advocating policy changes and implementation as a response to the changing dynamics in these countries pre- and post-BRICS formation. Therefore, the context of this paper is aimed towards examining the short term causalities and long term integration among the BRICS stock market pre- and post-BRICS formation. The research applies the Augmented Dicker-Fuller (ADF) and Philips-Perron tests (PP) tests to analyze stationarity among the selected variables. The pre- and post-BRICS formation long-term linear relationship is investigated using Johansen and Juselius cointegration test while the Granger Causality is applied to assess the direction of the causality between the stock market indices. The study also extends the investigation by employing the impulse response function and variance decomposition to evaluate the reaction of each of the BRICS market to a shock from other BRICS stock markets. Weekly stock market indices of BRICS countries were used covering the period from January 2003 to December 2018. One key finding is that the degree of financial integration among the BRICS stock markets has moderately strengthened in the post-BRICS formation period compared to the pre-BRICS formation period. Another significant finding is that the Chinese stock market are mostly independent from other BRICS markets in the two aforementioned sub-periods, implying diversification benefits for the international investors both in the short and the long run. Further, the results also reveal a unidirectional causal relationship from the Russian stock market to its BRICS counterparts in both periods. Finally, the overall results show an increased responsiveness of stock markets in BRICS countries to shocks in each other after the formation of the bloc as compared to pre- formation period.
PUBLIC INTEREST STATEMENT
The research in this paper attempts to understand the contemporary issues and trends in financial markets in the wake of the escalation of financial and economic amalgamations such as the formation of the BRIC bloc. We believe that the interconnectedness of financial markets of countries in this bloc will strongly appeal to the global investors, researchers, policy makers, and financial markets’ participants. The findings of the study will assist readers to recognize the importance and dynamics of financial integration among emerging markets, and help them realize any potential diversification benefits, if at all present. The study is also expected to generate more interest among researchers to explore the impact of trade relations and regional coordination on financial integration and portfolio diversification. The main finding of this research is indicate that the degree of financial integration among BRICS markets has moderately strengthened post-BRICS formation. Moreover, the results imply the potential of portfolio diversification for global investors both in the short and the long run.
1. Introduction
BRICS is an acronym referring to a bloc of emerging national economies: Brazil, Russia, India, China and South Africa. The BRIC bloc was established in June 2009 with four founding member nations; South Africa joined in 2010. Today, the five BRICS countries together account for almost a quarter of the Earth’s landmass, more than 40 percent of the world’s population, and 46 percent of the world’s labor force. BRICS countries are credited for nearly 30 percent of global gross domestic product and almost 50 percent of the global economic growth (Rasoulinezhadr & Jabalameli, Citation2018). Additionally, the combined GDP of BRICS countries is projected to exceed 128 USD trillion in 2050 as compared to a projected 66 USD trillion for the G7 countries (Hammoudeh et al., Citation2013). BRICS nations now play a key role in the world’s economy as producers of goods and services and potential consumer markets for most developed countries with a significant improvement in their share of the world trade over the last two decades from 3.6 percent to over 15 percent. BRICS exports value has expanded from 494 USD billion in 2001 to 2902 USD billion in 2016, and BRICS imports increased from 417 USD billion in 2001 to 2339 USD billion in 2016 (Rasoulinezhadr & Jabalameli, Citation2018). Because of their high levels of economic growth and high returns on investment, the BRICS countries have proved to be attractive destinations to foreign investors: foreign direct investment (FDI) inflows to BRICS countries have increased from 81 billion USD in 2000 to over 221 billion USD in 2012. Furthermore, since financialisation in BRICS countries has gained considerable momentum, their financial markets have emerged as attractive prospective avenues for international financial investors (Adu et al., Citation2015).
International portfolio diversification is an investment strategy used to reduce portfolio risk to any particular financial market and reduce the volatility of returns (Vo, Citation2017, Citation2018a, Citation2018b, Vo & Ellis, Citation2018; Vo et al., Citation2017; Vo & Tran, Citation2020). Foreign investors are looking to diversify their portfolio in emerging markets equities also provide significant benefits for firms in these markets (Vo, Citation2018c, Citation2019; Vo & Chu, Citation2019). Investors became more aware of the benefits of investing internationally due of the fact that securities tend to be less correlated across countries than within a country. However, the effectiveness of diversifying investments across different international markets depends on how integrated the financial markets are. If two international financial markets are highly integrated, then the benefits of diversifying investments across the two markets are very limited. Modern portfolio theory suggests that the benefits of international diversification depend on the degree to which the markets move together. If the markets are perfectly correlated, then there will be no benefit to portfolio diversification. Therefore, markets that are less integrated or segmented will likely provide opportunities to investors to achieve the benefits of portfolio diversification.
To aid efficient international investment, it is important to examine the level of financial integration between different international financial markets and the bias behavior of investors (Batten & Vo, Citation2010; Daly & Vo, Citation2013; Vo, Citation2009, Vo & Daly Citation2005a, Citation2005b; Vo & Daly, Citation2008). This is important for investors, but it also an important area of research for policy makers, bankers and regulators. Policy makers want to make sure that international portfolio diversification leads to efficient portfolio management. However, as markets become more integrated, there are important regulatory issues, for example, relating to the need to manage cross border financial contagion in a crisis. Therefore, the examination of the integration between various financial markets using improved econometric technics is an important area of research that this paper contributes.
Having a clear understanding of the financial integration among BRICS stock markets is necessary for investors, investment funds and policy makers. This is in part because stock markets in BRICS countries might have the potential to become a safe destination for portfolio diversification. As such, this paper examines the shift in the nature and strength of financial integration among BRICS markets. To this end, the paper seeks to answer three important research questions: i) Have the short and long-term financial linkage between the BRICS countries increased (become more extensive) in the post-formation period?; ii) When comparing pre-formation and post-formation sub-periods, has each BRICS stock market become more responsive to shocks in other BRICS stock markets?; and iii) Do portfolio diversification benefits among BRICS stock markets still exist after the formation of the BRICS bloc?
In exploring the answers to these questions, the paper employs the Johansen-Juselius cointegration test for cointegration along with the relevant tests of weak exogeneity, exclusion, and normalization. It also applies the Granger Causality test within a Vector Error Correction Model (VECM), and Innovation Accounting Analysis for quantitative causality techniques involving the Variance Decomposition and Impulse Response Function in order to explore causal relationships.
The remainder of this study proceeds as follows. Section 2 reviews the recent empirical studies on international financial integration. Section 3 introduces the data set. Section 4 provides the empirical results. Finally, section 5 concludes the paper.
2. Review of recent empirical studies
Numerous empirical studies have examined financial integration between developed economies, the financial integration among Asian stock markets and between Asian and developed markets, the financial integration between stock markets in Latin American Countries, and financial integration among stock markets in the Middle East and North Africa (MENA) region.Footnote1 Now there is an emerging body of research utilizing cointegration and causality techniques to measure the financial linkages and integration among BRICS stock markets. However, because the BRICS bloc only came into existence in 2009, there are relatively few empirical studies of the impact of the formation of the bloc, and thus little consensus about the nature and degree of financial integration within the BRICS bloc and between the bloc members and developed markets.
The first empirical studies of what became the BRICS bloc were Chittedi (Citation2009), Bahr and Nikolova (Citation2009), An and Brown (Citation2010), and Chittedi (Citation2009) used the Johansen-Juselius cointegration method with the Granger causality tests to find a long-term relationship between the US, UK and Japanese stock markets for the period 1998 to 2009. He also found the Indian stock market to be the most active of the BRIC and more closely linked to developed markets compared to the Brazilian, Russian and Chinese markets. Bahr and Nikolova (Citation2009) similarly found the Indian stock market to be strongly integrated at regional and international levels when compared to the other BRIC countries. An and Brown (Citation2010), however, examining the period 1995 to 2009, found that only China exhibited a strong long-term equilibrium with the US, while India along with Brazil, Russia, and non-US developed were found to be less integrated with the US. Fahami (Citation2011) and Aloui et al. (Citation2011) focused their attention on the impact of the global financial crisis (GFC). Fahami (Citation2011), examining the international financial integration of BRIC stock markets, before, during and after the GFC, used the Johansen-Juselius cointegration and Granger causality tests. He found that all BRIC stock markets were cointegrated in the three periods, however, an increasing number of causal relations occurred during the crisis period. Aloui et al. (Citation2011) also examined the financial interdependences between BRIC countries and the US over the period 2004 to 2009 in order to examine the impact of the GFC. The results show strong evidence of time-varying dependency between BRIC and US markets. Sheu and Liao (Citation2011), using both linear (Engle-Granger) and non-linear (Enders-Siklos) cointegration methods along with the Granger causality test, also investigated the integration among stock markets in Brazil, China, India, Russia and US and found not just short-term but long-term relationships between the BRIC markets. Further, Gambhir and Bhandari (Citation2011), using Johansen-Juselius cointegration and Granger causality tests of BRIC stock markets between 2004 and 2010, found the markets to be cointegrated, with no market having a stronger influence than any other.
Despite the differences between these studies, their overall findings implied that most of the BRIC markets offered considerable portfolio diversification opportunities for international financial investors, especially in non-crisis periods. However, because these studies covered only the pre-bloc period, they were unable to examine the possible implications of greater financial integration due to the formation of the bloc.
The incorporation of data from the post-formation period of the BRICS by more recent studies has cast doubt on the above findings. Gupta (Citation2011), for example, examined the correlation between the BRICS stock markets between 2008 and 2011 using the Granger causality test and found short-term relationships between the BRIC countries. Ahmad et al. (Citation2013), using the DCC-GARCH model, examined the contagion effect of a Eurozone crisis on stock markets in Brazil, Russia, India, Indonesia, China, South Africa and South Korea over the period 2009 and 2012, finding strong links between Brazil, India, Russia, China and South Africa and the Eurozone crisis. Zhang et al. (Citation2013), using the same method to measure the effect of the GFC on the correlation among BRICS and U.S. and European stock markets, found that a permanent change in the long-term correlations between the emerging BRICS and the developed markets with Brazil and Russia’s stock markets exhibiting stronger correlations with developed countries than that of India and China.
Zhong et al. (Citation2014) used the nonparametric cointegration test of Bierens (Citation1997) to investigate whether there are long-term benefits from international equity diversification between BRICS countries and the US over the period 1997 to 2012. Their findings revealed that stock markets of BRICS exhibit pairwise cointegration with the US market. Similarly, Nashier (Citation2015) examined integration among BRICS, US and the UK over the period 2004 to 2013 and found the all markets were integrated in both the short and the long-term. By contrast, when Dasgupta (Citation2013) examined the dynamic linkage among stock markets in BRIC countries and the US (using the Johansen-Juselius cointegration method), he found that all stock markets in these countries were segmented in the long-term—except for the Indian and Brazilian markets, which showed long-term integration.
More recently, Ouattara (Citation2017) examined the short-term linkages and the long-term cointegration level among the BRICS stock markets over the period from 2000 to 2015 using quarterly data. This study used the Augmented Dicker-Fuller (ADF) and Philips-Perron (PP) tests to analyze stationarity among the selected variables. The research applied the correlation test to investigate the degree of freedom existing among BRICS stock markets. The research used Johansen-Juselius cointegration test to investigate the existence of long term cointegration, while the Pairwise Granger Causality and the Wald tests were applied to gauge the direction of the causality between the stock market. A key findings was that the Chinese stock market was found to be mostly independent from other BRICS stock markets and the BRICS stock markets are not cointegrated in the long-term, making them a favorable destination for long-term international investors who seek diversification benefits in these other markets. In the same vein, Prakash et al. (Citation2017) examined the degree of financial integration among BRICS equity markets using monthly data over the period 2005 to 2014. They use the Johansen-Juselius cointegration test and Granger causality test. The results suggest that there is an ascending but weak long-term financial cointegration between BRICS equity markets. The results of the Granger causality test showed no causality among market pairs China–Brazil, Russia–Brazil, South Africa–Brazil, Russia—China, and South Africa–India.
In summary, the empirical findings on the question of integration/segmentation of the BRICS bloc are mixed, if not somewhat contradictory. We suggest the lack of empirical consensus could be due to two factors. First, the earliest studies could only examine the pre-formation period and therefore, unlike later studies, did not include the post-formation period data. Second, generally speaking, the later empirical studies which examined longer time-series did not seek to distinguish the pre- and post-formation periods. As such, little work has been done on the impact of BRICS bloc formation on the interconnectedness of stock markets. Yet it is prima facie plausible that the formation of the BRICS group has had a significant effect on both domestic and international financial markets, which in turn could open the door to a high level of regional financial integration. We propose that some of the empirical ambiguity in the literature about BRICS bloc integration/segmentation can be removed by delineating the pre-formation and post-formation bloc periods, thereby potentially revealing emergent changes in integration/segmentation between the BRICS due to the formation of the bloc in 2009. Our study thus intends to shed the light on the potential changes of stock market integration among BRICS countries arising out of the post-BRICS formation period as compared to the pre- formation era.
3. Data
This study employs weekly prices of major BRICS Indices, choosing one index from each country for a period beginning January 1st, 2003 to December 31st, 2018. The advantages of using weekly data are well documented in the finance literature. Weekly data has a significant advantage over the daily and monthly data since, on the one hand, it avoids the autocorrelation and noise related to daily observations (Wang et al., Citation2003), and on the other hand, it enables more robust testing for short-lived interactions than monthly observations. The choice of the indices was made on the basis of the availability of data on the Thomson Reuters Eikon database. The data for the following indices have been selected: Sao Paulo SE Bovespa Index (Brazil), MOEX Russia Index (Russia), NIFTY 50 Index (India), Shanghai SE Composite Index (China), and Johannesburg Stock Exchange All Share Index (South Africa). Crucial to this study, the data is divided into two sub-periods: pre-BRICS formation (January 2003 to December 2009) and post-BRICS formation (January 2010 to December 2018).Footnote2 (The BRICS formation point has been chosen according to the evolution of the BRICS acronym in 2010 when South Africa was included in the group.)
4. Empirical analysis and results
The methodology employed in this paper is comprised of four main parts. First, we apply the conventional augmented Dickey-Fuller (ADF) test (Dickey & Fuller, Citation1979) and the Phillips-Perron (PP) test (Phillips & Perron, Citation1988) to test the unit root hypothesis—i.e. the stationarity of variables under consideration. Second, we test for the existence of long-term co-movement among BRICS stock markets using the Johansen-Juselius cointegration technique. Third, we test for short and long-term causal relationships between BRICS stock markets by applying Granger Causality test (by VECM). Finally, we utilize the Innovation Accounting Analysis of Variance Decomposition and Impulse Response Function to quantify the impact (effect) of each of the BRICS markets on each other.
4.1. Augmented Dickey Fuller (ADF) and Phillip-Perron (PP) Tests for Stationarity
The results of the Augmented Dickey Fuller (ADF) and Phillip-Perron (PP) tests indicate that our variables are stationary in first level, integrated of order one I(1), satisfying the necessary condition for Cointegration.Footnote3
4.2. Cointegration Test: The Johansen-Juselius approach
Granger’s (Citation1981) original conception of cointegration testing sought to show that time-series variables become cointegrated when they are bound in one or more long-term linear relationships, in spite of any short-term deviation from the long-term path (Johansen & Juselius, Citation1990). Cointegration testing was further developed and refined by Engle and Yoo (Citation1987), Engle and Granger (Citation1987), and Stock and Watson (Citation1988), Johansen (Citation1988, Citation1991). In this paper, we apply the Johansen-Juselius maximum likelihood approach to test for cointegration among BRICS stock markets in pre- and post-BRICS formation periods.
Johansen and Juselius (Citation1990) reproduced the VAR (K) model as follows:
Where; ),
and
is an
vector of nonstationary time series variables which are
(1);
is an intercept vector;
and L is the lag operator;
is the identity vector and
is n
n long-term matrix; and
represents all stationary linear combinations among
variables. The Johansen-Juselius approach aims at finding the number of characteristic roots of
, which is equivalent to testing whether the eigenvalues of
are significantly different from zero (Kasa, Citation1992).Footnote4
Johansen and Juselius (Citation1990) proposed two test statistics to discover the rank of matrix , that is the number of cointegrating vectors. The first test is called the trace statistic. The aim of this test is to determine whether the smallest eigenvalues are significantly different from zero (Almohamad et al., Citation2018):Footnote5
where are the estimated values of the characteristic roots, attained from matrix Π. T is the number of observations of each variable. According to the trace test, the null hypothesis is
, which is tested against the alternative hypothesis
.
The second test statistic is the maximum eigenvalues test. This test is based on the largest estimated eigenvalues (+ 1):
where the null hypothesis of this test , is tested against the alternative hypothesis
.
Table demonstrates the results of the Johansen-Juselius test in the pre- and post-BRICS formation periods. In the pre-BRICS formation period, the results of the trace statistic test illustrate that one cointegrating vector (long-term relationship) exists among BRICS stock markets in the pre-BRICS formation period. However, the results of the maximum eigenvalue test indicate that no cointegration exist among the aforementioned stock markets in the pre-BRICS formation period. We adopt the outcomes of the trace statistic test since it is more powerful than the maximum eigenvalue test because the former takes into consideration all (n-r) of the smallest eigenvalues (Kasa, Citation1992).
Table 1. Johansen-Juselius cointegration test results for pre- and post-BRICS Formation sub-periods
The same conflict appears to exist between the trace statistic test and maximum eigenvalue test results in the post-BRICS formation period: the former indicates the existence of one cointegration relationship among BRICS stock markets in post-BRICS formation, whereas the latter exhibits no such cointegration. Again, we draw our conclusion based on the results of trace statistic test indicating the presence of one cointegration vector.
4.2.1. Normalizing the cointegration vector: Exclusion and weak exogeneity tests
Based on the results in Table which indicate the existence of one cointegration vector in both the pre- and post-BRICS formation periods, we can rewrite equation (1) as follows:Footnote6
where, represent the contribution of stock market indices in each of the BRICS countries to the cointegration vector;
represent the speed of adjustments of the aforementioned stock markets to the cointegration vector that follows any short-term deviation from the long-term cointegration relationship.
The presence of a cointegration relationship does not in itself mean that all markets enter the common trend, nor does it definitively mean that the benefits to portfolio diversification have been eroded (Almohamad et al., Citation2018). Hence, further testing must be conducted in order to discover the absence of any corresponding market in the long-term linear relationship. A valuable feature of the Johansen-Juselius approach to cointegration is that it allows testing for restricted forms of cointegration vectors by applying Exclusion and Weak Exogeneity tests.
The application of the exclusion test is necessary for determining the variables that are bound together in a long-term linear relationship (cointegration) by examining the following hypothesis; for
= 1 (one cointegration vector), for each variable (j = 1, …,5).
Table displays the results of the exclusion test for the pre- and post-BRICS formation periods. In the pre-BRICS formation period, we reject the null hypotheses of at 1% confidence level only for the Chinese, Russian and South African stock markets. This indicates that in the pre-BRICS formation period, financial integration existed among the Chinese, Russian and South African stock markets, whereas the Brazilian and Indian stock markets did not contribute to the long-term relationship among BRICS stock markets. On the other hand, the results of post-BRICS formation period indicate that the null hypothesis of exclusion is rejected for all BRICS stock markets (at different levels of confidence) except for China. Hence, the overall outcomes of the exclusion test indicate that the financial integration among most of the BRICS stock markets has strengthened after the formation of the BRICS bloc since more variables contribute to the cointegration vector in the post- as compared to the pre-BRICS formation period examined.
Table 2. Exclusion test results for pre- and post-BRICS Formation
Prior to displaying the long-term linear equations among cointegrated BRICS stock markets in both periods, we need to determine the dependent/independent variables in the cointegration equation. To this end, we perform the weak exogeneity test that examines the null hypothesis of = 0 by discovering the variables which have zero speed of adjustment to the cointegration vector. Variables which have zero speed of adjustment are identified as exogenous variables that never deviate from the long-term equilibrium relationship, whereas the variables that have non-zero speed of adjustment are identified as endogenous variables (Almohamad et al., Citation2018).
Table shows the results of the weak exogeneity test for the pre- and post-BRICS formation periods. It should be noted that the weak exogeneity test has only been applied to the stock markets which contribute to the long-term integration during the pre- and post-formation periods—i.e. the outcomes of the weak exogeneity tests for BRICS stock markets excluded from the cointegration system have not been reported since they are not part of the long-term cointegration equation. The results in Table indicate that in the pre-BRICS formation period, the null hypothesis of weak exogeneity can be rejected only for the South African stock market, indicating that it is expected to deviate from the long-term equilibrium relationship in the short-term. However, the South African stock market adjusts to changes in exogenous variables (viz. stock markets in China and Russia) in order to retain the long-term equilibrium. For the post-BRICS formation period, Table demonstrates that the null hypothesis of weak exogeneity is rejected for the Brazilian and South African stock markets, hence both are endogenous to the system, which indicates that the burden of adjusting the short-term deviations to long-term equilibrium falls on these two markets.
Table 3. Weak Exogeneity test results for pre- and post-BRICS Formation
Based on the outcomes of the exclusion and weak exogeneity tests, we can normalize the cointegrating vectors in the pre- and post-BRICS formation periods. As mentioned above, the main motivation behind the normalization is to depict the nature of the long-term relationship shared among the cointegrated stock markets (Almohamad, Citation2016). The cointegration equation is normalized around the variable that meets the following conditions: (i) is significant in the system (contributes to cointegration relationship), (ii) is endogenous to the system (has non zero speed of adjustment), and (iii) exhibits the largest value of test statistics for weak exogeneity (Almohamad, Citation2016).
The pre-BRICS formation cointegration vector can be normalized around the South African stock market since it is significant to the system (included in long-term relationship), and is the only endogenous variable:
The cointegration EquationEquation (5)(5)
(5) in the pre-BRICS formation suggests that a 1% increase in the stock market index of China leads to 0.83% increase in the South African stock market index, whereas, a 1% increase in the stock market index of Russia exhibits a greater impact and leads to 3.62% increase in the South African stock market index. Following the same procedure, the cointegration equation for post-BRICS formation is normalized around stock market of South Africa as follows:
EquationEquation (6)(6)
(6) states that for a 1% increase in Brazilian, Indian and Russian stock market indices, there is a 0.76%, 0.04% and 0.24% increase respectively in the South Africa stock market index. As per EquationEquations (5)
(5)
(5) and (Equation6
(6)
(6) ), the South African stock market appears to be influenced by other BRICS partners in both the pre- and post-BRICS formation periods. These results are plausible considering that first, the South African economy is smaller than its BRICS counterparts, and second, because South Africa plays an important role in facilitating trade access for BRICS countries to the African continent since South Africa is the trading hub of the South African Development Community (SADC).Footnote7 It should also be noted that in EquationEquation (6)
(6)
(6) China does not contribute to the long-term cointegration equation in the post-BRICS formation period. This might be explained by the fact that the Chinese economy relies heavily on finished product exports, mostly to developed economies; China’s top ten trading partners during 2018 (with the exception of India) are developed economies, and account for 55% of total Chinese exports (World’s Top Exports, Citation2019).
4.3. Short and long-term causality tests: The Granger causality approach
The existence of cointegration relationships among BRICS stock markets in the pre- and post-BRICS formation periods suggests short and long-term channels of causality among these markets. We thus apply the Granger (Citation1969, Citation1988) causality test within the vector error correction mechanism (VECM) in order to highlight the short and long-term causal relationships among BRICS stock markets in the pre- and post-BRICS formation periods. The VECM model for pre- and post-BRICS formation periods is represented as follows:
where is (
),
is (
),
is (
), and
= [
]. The short-term causal relationships among BRICS stock markets are depicted through the column matrix
which indicates the short-term effect of row matrix
on changes in
. The long-term causal effects are measured through the error correction-term
.
indicates the speed of the short-term disequilibrium correction needed to return to long-term equilibrium.
Table reports the short and long-term causal relations found among the BRICS stock markets in the pre-BRICS formation period. The t statistics of the error correction term is only significant at 5% for the South African stock market, which indicates a long-term causal effect of the Chinese and Russian stock markets on their South African counterpart. Furthermore, the burden of the short-term adjustment to the long-term equilibrium falls on the South African stock market. These results are in line with the findings of the cointegration equation in the pre-BRICS formation period—see EquationEquation (5)(5)
(5) —where the South African stock market is the dependent variable. The results of short-term causal relations are represented by the F statistics, which indicate there are no bidirectional causalities between the BRICS stock markets in the pre-BRICS formation period. The results in Table also indicate that the Russian stock market plays an important role in the pre-BRICS formation period, as it influences all other BRICS markets. This might be explained by the fact that Russia is the largest producer of energy products among the BRICS economies, and a major oil supplier to its BRICS counterparts: according to World’s Top Exports’s (Citation2019), Russia is the largest oil exporter to China with Russian oil making up 15.8% of China’s total oil imports; it is also a major oil exporter to India. Furthermore, the F-statistics in Table illustrate the existence of unidirectional causality from the Russian to the Brazilian stock market. This might be attributed to the similarities in their economic structure, both highly dependent on commodity prices compared to other countries (Aloui et al., Citation2011). The results in Table also show that the Chinese stock market influences markets in Brazil, India and South Africa. Some other unidirectional causal relations are also found from the South African stock market to markets in Brazil and India. This result is somewhat unexpected since the stock market in South Africa is considered as the least active as compared to other BRICS markets.
Table 4. Ganger Causality Results Based on Vector-Error Correction Model (VECM) for pre- BRICS Formation
Table demonstrates the causal relationships among the stock markets in the post-BRICS formation period. The error correction terms are estimated for all BRICS stock markets except for China since it has no contribution to the long-term equilibrium. The t statistics indicate the existence of various long-term causal relationships among the remaining stock markets: for instance, from India, Russia and South Africa to Brazil; from Brazil, Russia and South Africa to India; from Brazil, India, and South Africa to Russia; and from Brazil, India, and Russia to South Africa. Nevertheless, the t statistics is insignificant for South Africa. The results of the F statistics show that the Russian stock market continues to play a significant role in the post-BRICS formation era since it causes all other BRICS stock markets, as it did in the pre-BRICS formation. The results also indicate for the existence of a bi-directional causality among the Brazilian and the Indian stock markets. Finally, the Indian stock market is found to have a short-term causal effect on the stock markets in Brazil and China.
Table 5. Ganger Causality Results Based on Vector-Error Correction Model (VECM) for post-BRICS Formation
4.4. Innovation accounting analysis
The application of the Granger Causality test within the VECM above might be considered a qualitative measure of causal relationships—i.e. the test investigates the existence and direction of short-term effects on selected variables, but not the size of the effects (Almohamad et al., Citation2018). In this section, we conduct a dynamic analysis of causal relationships (viz. an innovation accounting analysis) that involves the Variance Decomposition and Impulse Response Function in order to quantify and thereby measure the exact degree of short-term causal impacts between the stock markets in the pre- and post-BRICS formation periods.
4.4.1. Variance Decomposition (VD)
The decomposition of the forecast error variance of BRICS stock markets provides an insight into the relative contribution of one variable in creating deviations in its own return and other markets’ returns (Janakiramanan & Lamba, Citation1998). Based on the outcomes of the Johansen-Juselius cointegration test, where one common cointegration vector has been found in both pre- and post-BRICS formation periods, the VD analysis is applied via the error correction-term (ECT).
Table shows the results of the VD test for the pre-BRICS formation period. The results reveal that the stock market in Russia appears to be the most exogenous of the BRICS markets, as it almost completely explains its own error variance. This result is in line with the short-term causality outcomes in Table , where the Russian stock market was found to be Granger-causing all other BRICS markets. As mentioned above, this may be attributed to the economic similarities between Russia and Brazil, as well as to the volume of exports from Russia to China and India. On the other hand, Table indicates that the stock market in South Africa seems to be the most endogenous market, as the highest percentage of its error variance is explained by other markets, and it only explains on average 39% of its own error variance. This outcome is consistent with the cointegration testing results for pre-BRICS formation—see EquationEquation (5)(5)
(5) —where the South African stock market was the dependent variable. Finally, Indian and South African markets are highly impacted by the Brazilian stock market for 10 weeks periods ahead.
Table 6. Decomposition of forecast error variances for all stock markets in pre-BRICS Formation sub-period
Table demonstrates results of the VD test for the post-BRICS formation period. The results do not indicate any significant change from the pre-BRICS formation period—the stock market in South Africa remains the most endogenous variable and its error variance is largely explained by stock market movements in Brazil. Again, this may be due to strong trade relation among Brazil and Africa, pertinently Brazil was ranked as the third largest trading partner of Africa, primarily for South Africa and Nigeria in 2010 (SAIIA, Citation2013). Results in Table also indicate that the stock market in China seems to be the most exogenous variable in the post-BRICS formation period, which is consistent with the outcomes of the cointegration test in the post-BRICS formation period, where the stock market in China does not share in the long-term co-movement among BRICS stock markets. Moreover, Indian and South African markets continue to be highly impacted by the Brazilian stock market, although less impact as compared to the pre-BRICS formation period.
Table 7. Decomposition of forecast error variances for all stock markets in post-BRICS Formation sub-period
4.4.2. Impulse Response Function (IRF)
The aim of the impulse response function is to provide insight into the patterns and time path of reactions of BRICS stock markets due to shocks (“impulses”) in these markets. The IRF analysis also illustrates the duration of the effect of a shock originating in a BRICS stock market on each of the other stock markets. The generalized impulse response function developed by Koop et al. (Citation1996) and Pesaran and Shin (Citation1998) is used here because it is invariant to different orderings of variables in the system (Enders, Citation2010). In our analysis, we consider 1, 5 and 10 week horizons to allow responding markets to adjust back to equilibrium.
Table shows the results of the impulse response analysis during the pre-BRICS formation period. We notice that, in general, the stock market in South Africa appears to be the most endogenous variable because it exhibits the highest response to shocks arising in other BRICS stock markets. This supports the findings of the cointegration and weak exogeneity tests in pre-BRICS formation period. On the other hand, the Russian stock market seems to be more exogenous than other BRICS markets since it exhibits the highest response to its own shock, whereas its responses to shocks in its BRICS counterparts appear to be negligible. Table also illustrates that none of the impacts of the shocks in BRICS stock markets dies out completely by week 10, which suggests that none of the BRICS stock markets are able to quickly return to the long term equilibrium after being affected by a shock originating in another BRICS stock market.
Table 8. Generalized Impulse Response by country to one standard deviation innovation in each stock market (pre-BRICS Formation)
Table shows the responsiveness of BRICS stock markets to shocks in each other in the post-BRICS formation period. We notice that although the shocks in the BRICS stock markets have a decreasing effect from Week 1 onwards, they do not die out completely by week 10. Table also reveals that the stock market in China is the most exogenous market as it is least responsive to shocks in its BRICS counterparts. This may be attributed to the sensitivity and responsiveness of the Chinese stock market to international, rather than regional factors such as the U.S-China trade conflict that was magnified when the U.S government imposed higher tax rates on commodities imported from China. On the other hand, the stock market in India is found to be most active as compared to other BRICS stock markets. Finally, the overall results of the impulse response analysis provide a clue from increased responsiveness of stock markets in BRICS countries to shocks in each other after the formation of the bloc as compared to pre- formation period. These outcomes are consistent with the findings of the Johansen-Juselius cointegration and exclusion tests in the post-BRICS formation period where more variables contribute to the long-term equilibrium relationship.
Table 9. Generalized Impulse Response by country to one standard deviation innovation in each stock market (post-BRICS Formation)
5. Conclusions
The results of this study demonstrate that the degree of financial integration among the BRICS stock markets has moderately strengthened in the post-BRICS formation period compared to the pre-BRICS formation period. All BRICS stock markets, except China, now contribute to the cointegration relationship compared to only three markets in pre-BRICS formation period (China, Russia and South Africa).
The results of Granger causality testing indicate there is a unidirectional causal relationship from the Russian stock market to its BRICS counterparts in both periods. This might be due to the important role of the Russian energy exports to its BRICS counterparts, especially, China and India. Further, the results of Variance Decomposition and Impulse Response analyses demonstrate that the Chinese stock market appears to be the most exogenous market—i.e. it is less affected by shocks in other BRICS stock markets, probably due to its stronger international trade linkages.
The results of this paper have important implications for portfolio investors and policy makers. The presence of increased linkages among BRICS stock market indices that the opportunity for portfolio diversification among BRICS stock markets has decreased but has not been entirely eliminated. Since the Chinese stock market appears to be more influenced by global rather than regional economic trends and financial conditions, it continues to exhibit a large degree of independence with only weak linkages to its BRICS counterparts. As such, BRICS can still be considered as a destination for global portfolio diversification. However, if as seems likely, the other BRICS economies eventually become more integrated into the increasing influential Chinese economy with respect to trade, foreign direct investment flows, and migration, it is possible that this avenue of risk avoidance for international financial investors will correspondingly diminish. It remains to be seen whether this future will eventuate.
The research in this paper has considered the level of integration between BRICS markets to help identify where the most benefits from portfolio diversification can be achieved. While this is one factor that needs to be considered when making investment decisions, another key factor is to consider the macro-economic factors and other residual variables that will influence the performance of different stock markets. The techniques used in this paper can be an important tool in future research exploring the impact of the various residual variables on the integration of markets.
Further, by building on the analysis in this paper, including the techniques applied, another important area of future research would be to apply this to study issues of volatility linkages and volatility spillovers between different financial markets. These are important new areas of empirical research that can be explored further.
Additional information
Funding
Notes on contributors
Xuan Vinh Vo
Somar Al-Mohamad research focuses on Financial Integration, Portfolio Diversification, Cryptocurrencies.
Audil Rashid research focuses on Financial Inclusion, Alternative Finance, Financial Innovation, Cryptocurrencies.
Walid Bakry research focuses on Financial Markets Efficiency, Stock Market Volatility, Financial Integration, Portfolio Diversification and Islamic Finance.
Ammar Jreisat research focuses on Bank Efficiency, Financial Markets Efficiency, Portfolio Diversification.
Xuan Vinh Vo research focuses on the areas of macroeconomics, banking and finance.
Research in this paper evaluates and analyzes the degree of financial integration among stock markets in one of the world’s main economic blocs. Having a clear understanding of the financial integration among stock markets is necessary for investors, investment funds and policy makers. Portfolio diversification leads to efficient portfolio management, thus the knowledge about financial integration aids efficient international investment decision. Furthermore, financial integration among markets could lead to escalation in both of pace and influence of financial crises beyond their main epicenters.
Notes
1. For studies of developed economies see Taylor and Tonks (Citation1989), Chan et al. (Citation1992), Kasa (Citation1992), Arshanapalli and Doukas (Citation1993), Allen and Macdonald (Citation1995), Masih and Masih (Citation1997), Wang et al. (Citation2003), Hunter (Citation2006), Pukthuanthong and Roll (Citation2009), Assidenou (Citation2011), Nikkinen et al. (Citation2012), Padhan and Sujit (Citation2013), Lehkonen (Citation201), and Deltuvaite (Citation2015). For studies of Asian economies see Chan et al. (Citation1992), Yang et al. (Citation2003), Erie and Aldrin (Citation2008), Abo Majid et al. (Citation2009), and Seth and Sharma (Citation2015). For studies of Latin American economies see Choudhry (Citation1997), Garrett and Spyrou (Citation1999), and Diamandis (Citation2009). For studies of the MENA economies see Darrat et al. (Citation2000), Assaf (Citation2003), Neaime (Citation2005), Bley and Chen (Citation2006), Legoarde-Segot and Lucey (Citation2007), Alkulaib et al. (Citation2009), Genc et al. (Citation2010), Chaudhry and Boldin (Citation2012), Neaime (Citation2012), Goucha and Hamdi (Citation2014), Bakry and Almohamad (Citation2018), Almohamad et al. (Citation2018), and Al-Mohamad et al. (Citation2018).
2. The descriptive statistics are available from the authors on request.
3. The results of unit root tests are available from authors on request.
4. The rank of matrix Π denominated by r shows the number of cointegration vectors. There are three possible cases (Almohamad, Citation2016):
a. Matrix Π has a zero rank, r = 0, which indicates that there is no long-term stationary linear relationship among yt variables.
b. Matrix Π has a full rank, r = n (invertible matrix), which indicates that the variables are stationary in levels I(0).
c. Matrix Π has a rank of 0 < r < n, which implies that there are r cointegrating vectors among yt variables and matrix Π can be expressed as Π = αδ′ where α and δ′ are of dimension r × n. δ represents the matrix of cointegrating vectors, while α denotes the matrix of weights with which each cointegrating vector goes through each of the Δyt equations.
5. Prior to applying the Johansen-Juselius cointegration test, we identified the appropriate lag length based on Akaike Information Criteria (AIC).
6. The following country abbreviations are used: Br = Brazilian stock market, Ch = Chinese stock market, In = Indian stock market, Ru = Russian stock market, SA = South African stock market.
7. SADC is an economic bloc including 16 African countries (https://www.sadc.int/about-sadc/)
References
- Abo Majid, M. S., Kassim, S. H.(2009). Impact of the 2007 US financial crisis on the emerging equity markets. International Journal of Emerging Markets, 4(4), 341–19. https://doi.org/10.1108/17468800910991241
- Adu, G., Alagidede, P., & Karimu, A. (2015). Stock return distribution in the BRICS. Review of Development Finance, 5(2), 98–109. https://doi.org/10.1016/j.rdf.2015.09.002
- Ahmad, W., Sehgal, S., & Bhanumurthy, R. N. (2013). Eurozone crisis and BRIICHS stock markets: Contagion market interdependence. Economic Modelling, 33, 209–225. https://doi.org/10.1016/j.econmod.2013.04.009
- Alkulaib, Y. A., Najand, M., & Mashayekh, A. (2009). Dynamic linkages among equity markets in the Middle East and North African countries. Journal of Multinational Financial Management, 19(1), 43–53. https://doi.org/10.1016/j.mulfin.2008.03.002
- Allen, D. E., & Macdonald, G. (1995). The long-run gains from international equity diversification: Australian evidence from cointegration tests. Applied Financial Economics, 5(1), 33–42. https://doi.org/10.1080/758527669
- Almohamad, S. (2016). Financial Integration in the MENA Region [PhD. Dissertation], School of Business and Law, Western Sydney University.
- Al-Mohamad, S., Elkanj, N., & Gangopadhyay, P. (2018). Time-varying integration of MENA stock markets. International Journal of Development and Conflict, 8(2), 85–114. https://researchdirect.westernsydney.edu.au/islandora/object/uws:52183/
- Almohamad, S., Mishra, V. A., & Yu, X. (2018). MENA stock markets integration: Pre and post Global Financial Crisis. Australian Economic Papers, 57(2), 107–141. https://doi.org/10.1111/1467-8454.12106
- Aloui, R., Ben Aissa, M. S., & Nguyen, D. K. (2011). Global financial crisis, extreme interdependences, and contagion effects: The role of economic structure? Journal of Banking and Finance, 35(1), 130–141. https://doi.org/10.1016/j.jbankfin.2010.07.021
- An, L., & Brown, D. (2010). Equity Market Integration between the US and BRIC Countries: Evidence from Unit Root and Cointegration test. Research Journal of International Studies, 16, 15–24. https://api.semanticscholar.org/CorpusID:59416024
- Arshanapalli, B., & Doukas, J. (1993). International stock market linkages: Evidence from pre- and post-October 1987 period. Journal of Banking and Finance, 17(1), 193–208. https://doi.org/10.1016/0378-4266(93)90088-U
- Assaf, A. (2003). Transmission of stock price movements: The case of GCC stock markets. Review of Middle East Economics and Finance, 1(2), 171–189. https://doi.org/10.1080/1475368032000139305
- Assidenou, K. E. (2011). Cointegration of major stock market indices during the 2008 global financial distress. International Journal of Economics and Finance, 3(2), 212–222. https://doi.org/10.5539/ijef.v3n2p212
- Bahr, R., & Nikolova, B. (2009). Oil prices and equity returns in the BRICS Countries. World Economy, 32(7), 1036–1054. https://doi.org/10.1111/j.1467-9701.2009.01194.x
- Bakry, W., & Almohamad, S. (2018). Further evidence on Middle East and North Africa financial markets integration. International Journal of Economics and Business Research, 15(1), 1–22. https://doi.org/10.1504/IJEBR.2018.088513
- Batten, J. A., & Vo, X. V. (2010). The determinates of equity portfolio holdings. Applied Financial Economics, 20(14), 1125–1132. https://doi.org/10.1080/09603101003761879
- Bierens, H. J. (1997). Nonparametric cointegration analysis. Journal of Econometrics, 77(2), 379–404. https://doi.org/10.1016/S0304-4076(96)01820-9
- Bley, J., & Chen, K. H. (2006). Gulf Cooperation Council (GCC) stock markets: The dawn of a new era. Global Finance Journal, 17(1), 75–91. https://doi.org/10.1016/j.gfj.2006.06.009
- Chan, K. C., Gup, B. E., & Pan, M. (1992). An empirical analysis of stock prices in major Asian markets and the United States. The Financial Review, 27(2), 289–307. https://doi.org/10.1111/j.1540-6288.1992.tb01319.x
- Chaudhry, M., & Boldin, R. J. (2012). GCC equity market indices integration. Applied Financial Economics, 22(6), 471–478. https://doi.org/10.1080/09603107.2011.619490
- Chittedi, K. R. (2009). Global stock markets development and integration: With special reference to BRIC countries. MPRA working paper, No. 18602.
- Choudhry, T. (1997). Stochastic trends in stock prices: Evidence from Latin American markets. Journal of Macroeconomics, 19(2), 285–304. https://doi.org/10.1016/S0164-0704(97)00016-5
- Daly, K., & Vo, X. V. (2013). The determinants of home bias puzzle in equity portfolio investment in Australia. International Review of Financial Analysis, 27, 34–42. https://doi.org/10.1016/j.irfa.2012.05.005
- Darrat, A. F., Elkhal, K., & Hakim, S. R. (2000). On the integration of emerging stock markets in the Middle East. Journal of Economic Development, 25(2), 119–129. https://api.semanticscholar.org/CorpusID:59452436
- Dasgupta, R. (2013). BRIC and US integration and dynamic linkages: An empirical study for international diversification strategy. Interdisciplinary Journal of Contemporary Research in Business, 5, 536–563. https://api.semanticscholar.org/CorpusID:73660612
- Deltuvaite, V. (2015). An empirical investigation of the Baltic stock markets global integration. Procedia-Social and Behavioral Sciences, 213, 430–435. https://doi.org/10.1016/j.sbspro.2015.11.562
- Diamandis, P. F. (2009). International stock market linkages: Evidence from Latin America. Global Finance Journal, 20(1), 13–30. https://doi.org/10.1016/j.gfj.2009.03.005
- Dickey, D. A., & Fuller, W. A. (1979). Distribution of the estimator for autoregressive time series with a unit root. Journal of American Statistical Association, 74(366), 427–431. https://www.jstor.org/stable/2286348
- Enders, W. (2010). Applied Econometric Time Series. John Wiley& Sons, Inc.
- Engle, R. F., & Granger, C. W. (1987). Co-integration and error correction: Representation, estimation, and testing. Econometrica, 55(2), 251–276. https://doi.org/10.2307/1913236
- Engle, R. F., & Yoo, B. S. (1987). Forecasting and testing in co-integrated system. Journal of Econometrics, 35(1), 143–159. https://doi.org/10.1016/0304-4076(87)90085-6
- Erie, F., & Aldrin, H. (2008). Co-integration and causality among Jakarta stock exchange, Singapore stock exchange, and Kuala Lumpur stock exchange. MPRA Working Paper No. 9637.
- Fahami, A. N. (2011). The structure of linkage and causal relationships between BRIC and developed equity markets. International conference on Information and Finance 21, 72–77.
- Gambhir, G., & Bhandari, J. (2011). BRIC Stock markets: An econometric analysis. Asia-pacific Business Review, VII(1), 102–110. https://doi.org/10.1177/097324701100700107
- Garrett, I., & Spyrou, S. (1999). Common stochastic trends in emerging equity markets. The Manchester School, 67(6), 649–660. https://doi.org/10.1111/1467-9957.00173
- Genc, I. H., Jubain, A., & Al-Mutairi, A. (2010). Economic versus financial integration or decoupling between the US and the GCC. Applied Financial Economics, 20(20), 1577–1583. https://doi.org/10.1080/09603107.2010.508713
- Goucha N. B. S., & Hamdi, I. (2014). Financial Integration before and after the crisis of 2008: Evidence from GCC and MENA Stock market indices. 5th Asia-Pacific Business Research Conference.
- Granger, C. W. J. (1969). Investigating causal relations by econometric models and cross- spectral methods. Econometrica, 37(3), 424–438. https://doi.org/10.2307/1912791
- Granger, C. W. J. (1981). Some properties of time series data and their use in econometric model specification. Journal of Econometrics, 16(1), 121–130. https://doi.org/10.1016/0304-4076(81)90079-8
- Granger, C. W. J. (1988). Some recent developments in a concept of causality. Journal of Econometrics, 39(1–2), 199–211. https://doi.org/10.1016/0304-4076(88)90045-0
- Gupta, S. (2011). Study of BRIC countries in the financial turmoil. International Affairs and Global Strategy, 1, 1–15.
- Hammoudeh, S., Sari, R., Uzunkaya, M., & Liu, T. (2013). The dynamics of BRICS’ country risk ratings and domestic stock markets, U.S. stock market and oil price. Mathematics and Computers in Simulation, 94, 277–294. https://doi.org/10.1016/j.matcom.2012.01.002
- Hunter, D. M. (2006). The evolution of stock market integration in the post-liberalization- A look at Latin America. Journal of International Money and Finance, 25(5), 795–826. https://doi.org/10.1016/j.jimonfin.2006.06.001
- Janakiramanan, S., & Lamba, A. S. (1998). An empirical examination of linkages between Pacific-Basin stock markets. Journal of International Financial Markets, Institutions and Money, 8(2), 155–173. https://doi.org/10.1016/S1042-4431(98)00029-8
- Johansen, S. (1988). Statistical Analysis of cointegration vector. Journal of Economic Dynamics and Control, 12(2), 231–254. https://doi.org/10.1016/0165-1889(88)90041-3
- Johansen, S. (1991). Estimation and hypothesis testing of cointegration vector in Gaussian vector autoregressive models. Econometrica, 59(6), 1551–1580. https://doi.org/10.2307/2938278
- Johansen, S., & Juselius, K. (1990). Maximum likelihood estimation and inference on cointegration- with application to the demand for money. Oxford Bulletin of Economics and Statistics, 52(2), 169–210. https://doi.org/10.1111/j.1468-0084.1990.mp52002003.x
- Kasa, K. (1992). Common stochastic trends in international stock markets. Journal of Monetary Economics, 29(1), 95–124. https://doi.org/10.1016/0304-3932(92)90025-W
- Koop, G., Pesaran, M. H., & Potter, S. M. (1996). Impulse response analysis in nonlinear multivariate models. Journal of Econometrics, 74(1), 119–147. https://doi.org/10.1016/0304-4076(95)01753-4
- Legoarde-Segot, T., & Lucey, B. M. (2007). Capital market integration in the Middle East and North Africa. Emerging Markets Finance and Trade, 43(3), 34–57. https://doi.org/10.2753/REE1540-496X430303
- Lehkonen, H. (2015). Stock market integration and the global financial crisis. Review of Finance, 19(5), 2039–2094. http://ezproxy.uws.edu.au/login?url=https://search-proquest-com/ezproxy.uws.edu.au/docview/1711583615?accountid=36155
- Masih, A. M. M., & Masih, R. (1997). Dynamic linkages and the propagation mechanism driving major international stock markets: An analysis of pre- and post- crash eras. The Quarterly Review of Economics and Finance, 37(4), 859–885. https://doi.org/10.1016/S1062-9769(97)90008-9
- Nashier, T. (2015). Financial Integration between BRICS and developed stock markets. International Journal of Business and Management Invention, 4(1), 65–71. https://api.sematicscholar.org/CorpusID:11237318
- Neaime, S. (2005). Financial market integration and macroeconomic volatility in MENA region: An empirical investigation. Review of Middle East Economics and Finance, 3(3), 231–253. https://doi.org/10.1080/14753680500407258
- Neaime, S. (2012). The global financial crisis, financial linkages and correlations in returns and volatilities in emerging MENA stock markets. Emerging Markets Review, 13(3), 268–282. https://doi.org/10.1016/j.ememar.2012.01.006
- Nikkinen, J., Piljak, V., & Aijo, J. (2012). Baltic stock markets and financial crisis of 2008–2009. Research in International Business and Finance, 26(3), 398–409. https://doi.org/10.1016/j.ribaf.2012.03.003
- Ouattara, S. B. (2017). Re-examining stock market integration among BRICS countries. Eurasian Journal of Economics and Finance, 5(3), 109–132. https://doi.org/10.15604/ejef.2017.05.03.009
- Padhan, P. C., & Sujit, K. S. (2013). Pre & post-recession stock markets integration: Some empirical evidence. International Journal of Business and Management, 8(8), 147–161. https://doi.org/10.5539/ijbm.v8n8p147
- Pesaran, H. H., & Shin, Y. (1998). Generalised impulse response analysis in linear multivariate models. Economics Letters, 58(1), 17–29. https://doi.org/10.1016/S0165-1765(97)00214-0
- Phillips, P. C. B., & Perron, P. (1988). Testing for unit root in time series regression. Biometrika, 75(2), 335–346. https://doi.org/10.1093/biomet/75.2.335
- Prakash, V. J., Nauriyal, K. D., & Kaur, S. (2017). Assessing financial integration of BRICS equity markets: An empirical evidence. Emerging Economy Studies, 3(2), 20–35. https://doi.org/10.1177/2394901517730734
- Pukthuanthong, K., & Roll, R. (2009). Global market integration: An alternative measure and its application. Journal of Financial Economics, 94(2), 214–232. https://doi.org/10.1016/j.jfineco.2008.12.004
- Rasoulinezhadr, E., & Jabalameli, F. (2018). Do BRICS countries have similar trade integration patterns? Journal of Economic Integration, 33(1), 1011–1045. https://doi.org/10.11130/jei.2018.33.1.1011
- SAIIA. (2013). BRICS FDI: A preliminary view. South Africa Institute of International Affairs. Retrieved August 1, 2019, from https://saiia.org.za/research/brics-fdi-a-preliminary-view/on
- Seth, N., & Sharma, A. K. (2015). International stock market efficiency and integration. Journal of Advances in Management Research, 12(2), 88–106. https://doi.org/10.1108/JAMR-07-2011-0010
- Sheu, H.-J., & Liao, C.-H. (2011). Dynamics of stock market integration between the US and the BRIC. African Journal of Business Management, 5(9), 3674–3689. https://api.semanticscholar.org/CorpusID:56465219
- Stock, J. H., & Watson, M. W. (1988). Testing for common trends. Journal of the American Statistical Association, 83(404), 1097–1107. https://doi.org/10.1080/01621459.1988.10478707
- Taylor, M. P., & Tonks, I. (1989). The internationalisation of stock markets and the abolition of U.K. exchange controls. The Review of Economics and Statistics, 71(2), 332–336. https://doi.org/10.2307/1926980
- Vo, X. V. (2009). International financial integration in Asian bond markets. Research in International Business and Finance, 23(1), 90–106. https://doi.org/10.1016/j.ribaf.2008.07.001
- Vo, X. V. (2017). Trading of foreign investors and stock returns in an emerging market-Evidence from Vietnam. International Review of Financial Analysis, 52, 88–93. https://doi.org/10.1016/j.irfa.2017.05.007
- Vo, X. V. (2018a). Trade integration and international financial integration: Evidence from Asia. The Singapore Economic Review, 1–12. https://doi.org/10.1142/S0217590818500248
- Vo, X. V. (2018b). Determinants of capital flows to emerging economies-Evidence from Vietnam. Finance Research Letters, 27, 23–27. https://doi.org/10.1016/j.frl.2018.02.031
- Vo, X. V. (2018c). Foreign investors and stock price crash risk: Evidence from Vietnam. International Review of Finance. https://doi.org/10.1111/irfi.12248
- Vo, X. V. (2019). Do foreign investors promote stock price efficiency in emerging markets? International Review of Finance, 19(1), 223–235. https://doi.org/10.1111/irfi.12164
- Vo, X. V., & Chu, T. K. H. (2019). Do foreign shareholders improve corporate earnings quality in emerging markets? Evidence from Vietnam. Cogent Economics & Finance, 7(1), 1698940. https://doi.org/10.1080/23322039.2019.1698940
- Vo, X. V., & Daly, K. (2008). Volatility amongst firms in the Dow Jones EurostoXX50 index. Applied Financial Economics, 18(7), 569–582. https://doi.org/10.1080/09603100601057888
- Vo, X. V., & Daly, K. J. (2005a). European equity markets integration—implications for US investors. Research in International Business and Finance, 19(1), 155–170. https://doi.org/10.1016/j.ribaf.2004.10.007
- Vo, X. V., & Daly, K. J. (2005b). International financial integration: An empirical investigation into Asian equity markets pre-and post-1997 Asian Financial Crisis. Contemporary Studies in Economic and Financial Analysis, 86, 75–100.
- Vo, X. V., & Ellis, C. (2018). International financial integration: Stock return linkages and volatility transmission between Vietnam and advanced countries. Emerging Markets Review, 36, 19–27. https://doi.org/10.1016/j.ememar.2018.03.007
- Vo, X. V., Nguyen, D. P., Ho, V. T., & Nguyen, T. T. (2017). Where do the advanced countries invest? An investigation of capital flows from advanced countries to emerging economies. Journal of International Financial Markets, Institutions and Money, 51, 142–154. https://doi.org/10.1016/j.intfin.2017.09.004
- Vo, X. V., & Tran, T. T. A. (2020). Modelling volatility spillovers from the US equity market to ASEAN stock markets. Pacific-Basin Finance Journal, 59, 101246. https://doi.org/10.1016/j.pacfin.2019.101246
- Wang, Z., Yang, J., & Bessler, D. A. (2003). Financial crisis and African stock market integration. Applied Economics Letters, 10(9), 527–533. https://doi.org/10.1080/1350485032000100198
- World’s Top Exports. (2019, August 1). www.worldstopexports.com/chinas-top-import-partners/
- Yang, J., Kolari, J. W., & Min, I. (2003). Stock market integration and financial crisis: The case of Asia. Applied Financial Economics, 13(7), 477–486. https://doi.org/10.1080/09603100210161965
- Zhang, B., Li, X., & Yu, H. (2013). Has recent financial crisis changed permanently the correlations between BRICS and developed stock markets? North American Journal of Economics and Finance, 26, 725–738. https://doi.org/10.1016/j.najef.2013.05.003
- Zhong, M., Chang, T., & Tzeng, H. (2014). International equity diversification between the United States and BRICS countries. Romanian Journal of Economic Forecasting, 17(1), 123–137. https://api.semanticscholar.org/CorpusID:150657604