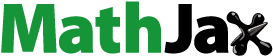
Abstract
Volatility is one of the most important factors of investment decisions. Unexpected information forces the investor to trade abnormally in the market which in turn affects the volatility of the market. But this kind of trading behavior has a different impact on the different market segments. This study investigates the effect of unexpected DII and FPI flows on the volatility of large-cap, mid-cap and, small-cap stocks in Indian markets. Using ARMA (1, 1) and TGARCH (1, 1) model, we estimate the impact of unexpected FPI and DII flows on volatility. The main result of the study shows that unexpected flow of FPIs has a positive impact on market volatility but this impact is reduced by unexpected flow of DIIs. Further, results show that unexpected selling of FPIs increase volatility more than unexpected purchase. Impact of unexpected flow of DIIs flow is more dominating in small-cap stocks. Results from this study are useful for policymakers and regulator.
Keywords:
PUBLIC INTEREST STATEMENT
There are two broad sources of investments in Indian stock market: Foreign Portfolio Investments (FPIs) and Domestic Institutional Investments (DIIs). DIIs, unlike FPIs, are considered a stable source of capital because they are domestic in nature. Unexpected information forces abnormal trade, but this abnormality is non-uniform in different market segments. This study investigates the effect of unexpected DIIs and FPIs flows on the volatility (fluctuation) of large-cap, mid-cap and, small-cap stocks in Indian markets. Results show that unexpected flow of FPIs has a positive impact on volatility but this impact is reduced by unexpected flow of DIIs in large cap and midcap stocks. In small-cap stocks, unexpected flow of DIIs increases volatility.
This study recommends that government should maintain a balance between FPIs and DIIs. This study is the first attempt to explore the relationship between market volatility of three different segments and investment flows of both DIIs and FPIs.
1. Introduction
A disciplined financial market is the key engine of the economic development of any country. After liberalizing economic policies and globalization of the asset market, the world is looking towards emerging markets for a better return on investments. This expectation for higher returns increases the flow of capital in emerging markets not only from the foreign investors but also from the domestic investors.Footnote1 Capital inflows into the equity market increase the stock price, lowers the cost of equity capital, increases knowledge flow, improves market efficiency, strengthens corporate governance and improves the market design of the security market. However, foreign investors are ill-informed about the emerging market and this lack of sound information may generate herding and positive feedback trading (Edelen & Warner, Citation2001; Watson & Wickramanayake, Citation2012). These kinds of behavior can push security prices away from their fair value and exacerbate volatility. On the other hand, domestic investors provide a stable source of capital to the security market. But, if the relative strength of the domestic investors is weaker in comparison to foreign investors, the foreign players start dominating in the equity market and drive the equity price away from their equilibrium price leading to volatility.
Volatility is defined as the degree of price variation during a particular period hence considered a proxy of risk. Since the expected return from an investment is determined by the risk associated with the same, volatility is also affected by the expected returns. Investors interpret an increase in stock market volatility as an increase in the risk of equity investment and consequently they shift their funds to less risky assets. It puts a downward pressure on the stock prices making room for higher expected future returns to compensate for the excess risk. The volatility is one of the crucial inputs in all the investment decisions including portfolio construction, pricing of assets and their derivatives, designing hedging strategies, determining capital adequacy and margin requirements besides other tools of risk management.
The flow of information is the main determinant of volatility; more the amount of unexpected information (news) reaching the market, more volatility it causes in the asset prices. Volatility is also affected by the price discovery mechanism and speed of adjustment of security prices to the news. Gradual adjustment in the prices causes less volatility than the instantaneous adjustment. On the other hand, when the security prices are subject to over-reaction, prices are more volatile than in the case of instantaneous adjustment. Prices are more volatile in a market dominated by noise traders and investors with heterogeneous beliefs.
In the past decade, the Indian stock market has become an attractive place to park their capital for foreign investors in search of high risk-adjusted returns. Foreign Portfolio Investment (FPI) flows are continuously growing to a remarkable extent in the India stock market. The quantum of FPIs depends on the returns and sentiments of the stock market. The FPI inflows contribute to an increase in the stock market indices and their exit brings down the market indices and as such creates huge fluctuations in the stock markets of the host country, resulting in volatility. On the other hand, the quantum of Domestic Institutional Investment (DII) is in its nascent stage in India in comparison to that of developed nations. Savings and investments are important factors behind the stock market development of any economy. DIIs like banks, insurance companies and, mutual funds play a key role to mobilize funds from the household sector to channelize them into capital markets. These funds unlike foreign investments are considered a stable source of capital in the stock markets.
This study has been undertaken to analyze the influence of FPIs and DIIs investment on stock market volatility. It is believed that increasing participation of institutional investment increases the volatility of the domestic market in emerging economies. There are two possible channels of volatility-effect. First, the herding behavior of foreign portfolio investors may cause sudden wide-mismatch in demand and supply equilibrium dragging the prices far away from their fundamentals. Second, increasing participation of domestic investors increases the linkages between domestic markets and foreign investor to prevent excessive volatility. The objective of this study is to investigate the effect of DII and FPI flows on the volatility of Indian stock markets. We estimate the volatility of large-cap, mid-cap and small-cap index using the GARCH model and use unexpected DII flow and unexpected FPI flows as additional regressors in the volatility equation. The coefficients of these repressors capture the impact of DII and FPI flow on stock market volatility.
Our study contributes to several aspects of literature: First, earlier studies focus on the effect of FPIs in market volatility while this study is a first attempt to enlighten the relationship between the market volatility and investment flow of both the DIIs and FPIs. Second, this study models the volatility of three different segments of the market namely large-cap, mid-cap and small-cap index. This is important because after demonetization and increased financial literacy, savings have been channelized into stock markets in the form of mutual fund investments which are across large, mid and, small-cap funds. Further, analysis of volatility is very important from the viewpoint of the policymakers for a country like India where international investment in securities is increasingly assuming importance as a source of external finance. Also, the influence of FPIs and DIIs investment on stock market volatility is important from the perspective of policymaker for monitoring of systemic risk for India in particular and EM basket of countries in general. The results of this study have importance for policymakers, regulators, investors, and academicians.
The rest of the paper is structured as follows. A brief review of previous studies is given in section 2. Section 3 describes the data and methodology used for the study. Section 4 presents and discusses the empirical results while Section 5 provides conclusions and policy suggestions.
2. Review of literature
The volatility of stock market has attracted a lot of attention from academic researchers since the past few decades. Stock market liberalization opened the doors of emerging capital markets for foreign investors. As a result, the pursuit of high returns on investments encourages the FPIs and DIIs to invest in emerging stock markets. A vast literature is devoted to explain the relationship between stock price movement and investment flows.Footnote2 This increase in investment by institutional investors generates a sharp increase in returns through the size of the trade (Gabaix et al., Citation2006). Bessembinder and Seguin (Citation1993) reported that heterogeneous trading patterns of different investors may affect volatility because various investor groups can have different motivations. Researchers are divided in view that which types of investors create more volatility. Some researchers argue that individual investors exacerbate stock return volatility (Barber & Odean, Citation2000; Barber et al., Citation2009; Daigler & Wiley, Citation1999; Foucault et al., Citation2011) while others oppose this argument (Avramov et al., Citation2006). For institutional investors, De Long et al. (Citation1990) presented evidence that institutional investors increase stock return volatility.
Since the pay-off of all foreign portfolio investors depends on a similar set of variables; they are governed by similar motives in their investment and disinvestment decisions. This leads us to believe that the FPIs may exhibit “herding behavior”, i.e. they may invest in an economy in a lot when there are better expectations and withdraw in bulk when prospects are weak. Herding behavior of foreign investors may put heavy demand and supply pressure on the stock and forex-markets of developing countries where financial markets lack depth. This may make the markets highly volatile and sometimes may turn mild financial distress into a full-fledged financial crisis. The similar phenomenon was observed in the East-Asian markets during the Asian of 1998 crisis and it was understood to be responsible for its deepening (Chakrabarti & Roll, Citation2002; Choe et al., Citation1999; W Kim & Wei, Citation2002; Krugman, Citation1998; Stiglitz, Citation1998).
Given the huge volume of investment-fund at their disposal, FPIs can move the market. They could play a role of market-makers and book their profits, i.e. they can buy financial assets when the prices are declining and sell when the prices of the assets are increasing (Bae et al., Citation2004; Dhingra et al., Citation2016; Gordon & Gupta, Citation2003). However, the empirical evidence on this issue is mixed. Levine and Zervos (Citation1995) find the evidence supporting the liberalization has increased the volatility in emerging markets; while, many other studies have found that there is no systematic effect of liberalization on stock market volatility (Geert & Harvey Campbell, Citation1998; De Santis & Imrohoroglu, Citation1997; E H Kim & Singal, Citation2000). Studies have shown empirical evidence where foreign investment induces greater volatility in markets compared to domestic investors (Jo, Citation2002) and stocks mainly traded by these investors experience higher volatility than those in which these investors do not have much interest (Bae et al., Citation2004). On the contrary, evidence that international investments do not have a significant impact in increased volatility of stock returns (Geert & Harvey Campbell, Citation1998) are also there and these render the concern for the volatility of such flows largely unwarranted (Errunza, Citation2001). What emerges from this evidence is that the role of volatility cannot be ignored and its impacts on portfolio investments differ widely among countries. Hence, the analysis of the volatility of such flows is very important from the viewpoint of the policymakers for a country like India where international investment in securities is increasingly assuming importance as a source of external finance.
In the contemporary Indian scenario, there are a few studies addressing the issue of interlinkages between stock prices and investment flows. Almost all the studies conclude that the equity returns have a significant and positive impact on the FPI-flow but FPI-flow is not causing the future movements of the market (Agarwal, Citation1997; Badhani, Citation2006; Batra, Citation2003; Chakrabarti, Citation2001; Panda, Citation2005; Rao & Sensharma, Citation2005; Trivedi & Nair, Citation2003). Mazumdar (Citation2004) documents that FPIs flow has enhanced liquidity in the Indian stock market but not much evidence is present to support the hypothesis that FPI flow has generated volatility in the asset returns. Ahmed et al. (Citation2005) also, confirm that there has been a very little destabilizing effect of FPI flow on individual equity returns.
Existing literature focuses on the volatility of the stock market caused by FPIs flow (Batra, Citation2003; Dhingra et al., Citation2016; Geert & Harvey Campbell, Citation1998; Rao & Sensharma, Citation2005; De Santis & Imrohoroglu, Citation1997; Trivedi & Nair, Citation2003). Virtually, no study has been carried out which compares the effect of DIIs and FPIs flow on stock market volatility. Further, this study documents the volatility of three different segment namely large-cap, mid-cap and, small-cap indices of the stock market while earlier studies only focus on market-wide volatility of the stock market (Bae et al., Citation2004; Errunza, Citation2001).
3. Data and methodology
3.1. Data
This study covers a period from March 2009 to March 2018 and daily FPI and DII-flow data have been used for the study. Data regarding the other variables are also collected for similar frequencies. The S&P CNX Nifty has been used as a proxy of stock-prices in India. Secondary data is used in this study collected from different sources. The daily data regarding FPI and DII-flow (gross purchase, gross sales, and net investment flow) is collected from the Security and Exchange Board of India (SEBI). Daily closing data of Nifty 50,Footnote3 Nifty Midcap 50, Nifty Small-cap50 and India VIX is also collected from SEBI.
3.1.1. Descriptive results
It is observed that return from indexes is moving along with FPIs while market indexes moving in the opposite direction of DIIs. This can be confirmed by correlation structure presented in Table . Descriptive results in Table show that the mean inflow of FPIs is approximately two and half times of DIIs which indicate that FPIs can drive the market by volume. High kurtosis of FPI inflow indicates that the probability of an extreme event is higher which is of particular interest to policymakers dealing with asset pricing bubble leading to systemic risk. Standard deviation is also high for FPIs which shows the high volatile nature of these large flows.Footnote4
Table 1. Descriptive statistics of variables
Table 2. Correlation structure of variables
3.2. Methodology
3.2.1. Test of stationarity
The basic assumption behind any econometric model is stationary of data. To check the stationarity we check the presence of unit root in the series. Presence of unit root represents the non-stationarity of data. Augmented Dickey–Fuller (ADF) test is used to check the stationarity of data. Table shows the result of the ADF test which confirms that all the series are stationary at level form.
3.2.2. Expected and unexpected component of Net DII/Net FPI flow
The market is believed to react to unexpected changes in relevant exogenous (economic or non-economic) variables. Therefore, the net DII-flow and Net FPI-flow are decomposed into expected and unexpected components using ARMA (p, q) model. A closer inspection of the patterns of autocorrelations and partial autocorrelations of the series suggests that series can possibly be modeled as ARMA (1, 1)Footnote5 process. Therefore, we choose ARMA (1, 1) model to separate the expected and unexpected components of DII-flow and FPI-flow.
The ARMA (1,1) model can be specified to get the unexpected components of DII-flow as follows:
where coefficient β1 is the AR (1) coefficient and γ1 is the MA (1) coefficient.
Hence, the unexpected DII is represented by the residual term.
The ARMA (1,1) model again used to get the unexpected components of FPI-flow as follows:
Where coefficient β2 is the AR (1) coefficient and γ2 is the MA (1) coefficient.
Hence, the unexpected FPI is represented by the residual term.
Before using the unexpected DII and FPI-flow as a variable to explain the volatility of market returns, the residuals obtained are standardized using time-varying standard deviation. Therefore, the standardized unexpected DII and FPI flow are obtained as follows:
where z is standardised residual and σ is the time varying standard deviation of DII and FPI flow.
3.2.3. Modelling the volatility of market returns
where ϑ ̴N(0,1)
To capture the volatility of market returns and the impact of unexpected shocks of DII-flow and FPI-flow on volatility, we use GARCH model. It is a well-documented stylized fact that the volatility of asset returns reacts asymmetrically to return shocks. A negative shock in return produces higher volatility in comparison to a positive shock of the same magnitude. Many variants of GARCH model have been suggested in the literature to capture this asymmetric reaction of volatility. In this study, we use a Threshold GARCH (TGARCH)Footnote6 model as suggested by Glosten et al. (Citation1993) which is popularly known as GJR model to capture this phenomenon. First, we model the conditional mean of the return as AR (1) process.
Rt is the return at time t, c is constant, ρ is the coefficient of AR(1) process and Ω is the coefficient of Volatility index (VIX).
where EquationEquation (6)(6)
(6) is a mean equation and EquationEquation (7)
(7)
(7) is the variance equation. In mean equation, we add India volatility index (VIX) as a control factor which act as a catch all proxy for all other exogenous shocks. α and β are coefficients of TGARCH model. α Shows the impact of a positive news on the volatility while δ is the incremental impact of a negative news. The t-statistics are based on Bollerslev-Wooldridge robust standard errors. The estimation is conducted for all Nifty 50, Nifty midcap 50 and Nifty small-cap 50.We further include squared standardized unexpected DII flow, unexpected FPI-flow, unexpected FPI Purchase, unexpected DII Purchase, unexpected FPI Sales, unexpected DII Sales, Purchase RatioFootnote7 and, Sales RatioFootnote8 in the conditional variance equation of return series as the additional regressors to capture the effect of FPIs and DIIs on volatility.Footnote9
4. Results and discussion
The results of the AR (1) model are presented in Table , for Nifty50, Nifty midcap50 and Nifty small-cap50, respectively. The estimated AR (1) parameter, is statistically significant at a very low level in Nifty 50 and Nifty midcap50 and one unit change in past return explains 6% and 9% variation in returns in Nifty 50 and Nifty midcap 50, respectively. These observations are consistent with the efficient market hypothesis. While in Nifty small-cap50 parameter of AR (1) is significant and one unit change in past return explains around 16% variation in return. These results indicate that, in India small cap section past returns have more predicting powers in small-cap market than large-cap or midcap and small cap market in India is more inefficient in India.
Table 3. Stationary test
Table 4. Estimations of AR(1) model for Nifty 50, Nifty midcap50 and Nifty small-cap50
In the variance equation, the coefficient of asymmetry (Table ) is quite high and statistically significant. In fact, the impact of positive return shock on the volatility, captured by coefficient α, is statistically insignificant in Nifty50 and Nifty midcap50 while significant in Nifty small-cap50. A positive return shock of 1 unit increases the volatility by 0.05 units in Nifty50, 0.08 in Nifty midcap50 and 0.109 in Nifty small-cap50. On the other hand, a negative shock of the same magnitude increases the volatility by 0.28 units (
) in Nifty50, 0.37 in Nifty midcap50 and 0.43 in Nifty small-cap50.
Table 5. Estimation of TGARCH(1,1) model for Nifty 50, Nifty midcap50 and Nifty small-cap50
4.1. Impact of net FPI and Net DII flow on volatility
The results of the estimation are presented in Tables –. Table presents the estimate of different regressors on the volatility of Nifty 50. Results show that coefficient of unexpected FPI-flow is statistically significant in Nifty50 and its coefficient is positive which indicate one unit change in unexpected FPI flow increase the volatility of market 0.28 unit.1 unit Unexpected DII flow individually decreases the volatility by 0.12 units. The Combined effect of FPI and DII flow increases the volatility by 0.098 units (0.237–0.139) which is less than the individual effect of FPI (0.28). This result shows that DII mitigate the risk of unexpected FPI flow and stabilize the market. Further investigation is done by separating unexpected FPI and DII flow into unexpected purchases and sales components. Results show that unexpected sales shock by FPI destabilise market more than unexpected purchase shock. One unit change in FPIs’ unexpected sale increase volatility by 0.35 units while same amount of unexpected purchase increases volatility by 0.07 units. Unexpected DII purchase increases volatility by 0.10 units. Purchase ratio has insignificant effect of volatility but sales ratio increases the volatility by 0.32 units. It means that equal amount of sales by FPIs and DIIs increases volatility by 0.32 units. These results indicate that FPIs sales bring more volatility in Nifty 50 than FPIs purchase. On the other hand, it is observed that DIIs bring volatility down and try to stabilise the market if FPIs overreact (particularly in case of selling).
Table 6. Estimation of FPIs and DIIs on nifty 50 using TGARCH (1,1) for Nifty 50
Table 7. Estimation of FPIs and DIIs on nifty midcap 50 using TGARCH (1,1) for Nifty midcap50
Table 8. Estimation of FPIs and DIIs on nifty small-cap 50 using TGARCH (1,1) for Nifty small-cap50
Table captures the impact of FPIs and DIIs trading activity on volatility of Nifty midcap 50. In midcap index, coefficient of unexpected FPIs and DIIs flow is positive and significant. This shows that positive change in FPIs and DIIs flow increase the volatility. But their combined impact is less this may be due to their opposite behaviour. Midcap firms are growing firms and their perceived risk is higher than large-cap which is relatively more financially stable firms. This may be a possible reason that these stocks react more to unexpected flow of investment. However, unexpected sales by FPIs create more havoc in midcap50 than large-cap 50. Unlike Nifty50, these stocks also overreact to purchase shocks.
Table captures the impact of FPIs and DIIs trading activity on volatility of Nifty small-cap50. Results show that unexpected DIIs draw the returns far from mean more than unexpected FPIs. Coefficient of unexpected FPIs flow is 0.199 while the coefficient of unexpected DIIs flow is 0.537. This may be due to the high prospect of growth in these stocks. Private information for these stocks may also be a reason that DIIs invest unexpectedly more in these stocks. Higher sales of FPIs also increase volatility in small-cap 50. FPIs invest more in large-cap firm, high selling by FPIs create an opportunity for DIIs in large-cap firm. This may be a reason that DIIs dilute their position from small-cap firms which increase volatility in these stocks. This argument can be explained by the panel 3 and panel 5 of Table that shows that the coefficient of unexpected FPIs selling is positive and significant while the coefficient of FPIs purchase is insignificant. Further, the selling ration is positive and significant that confirms that higher FPIs selling make small-cap stocks more vulnerable.
5. Conclusion
Since last few decades, researchers, regulators, policymakers and, investors are concerned about the stock price behavior. Researchers have diverse opinions on stock market volatility and investment flow. This study provides a comprehensive understanding of stock market volatility and trading activity of institutional investors.
The results suggest that the unexpected FPI-flow increases stock market volatility but its coefficient is small in Nifty small-cap 50. It is found that the impact of unexpected FPI shock decreases in presence of DIIs in large-cap and midcap stocks. In small-cap stocks, the unexpected flow of DIIs increases volatility. The results suggest that selling shock affects markets more than purchase shock. Small-cap stocks are found to be more vulnerable than large-cap and midcap stocks. Not only trading activity of FPIs, but trading activity of DIIs also pushes these stocks far from their equilibrium mean price.
The study offers various points that help policymakers to strengthen the Indian stock market. First, in current economic scenario, India has adopted the policy to promote the FPIs in the Indian capital markets, as FPIs increase capital flow in the country without increasing the foreign debt of the country. But an increase in FPIs brings vulnerability to stock prices as the selling pressure of FPIs pulls the stock prices down. On the other hand, DIIs provide a stable source of finance so the government needs to strengthen domestic investment and participation. This is to be done by changing the investment, saving outlook and tax policy of the country. Second, small-cap firms, mainly those firms that are in their early stages of development, need more financial stability and efficiency. The government needs to formulate effective policies for the sustainable financial development of these firms as these firms are a major source of employment and growth of a country. Any financial shock in these firms creates a scarcity of employment. Policies should focus on maintaining a balance between FPIs and DIIs. Domestic investment institutions, such as mutual funds, insurance funds, and pension funds should be promoted while encouraging the FPI flow. The input of this study will also be of interest to investors and portfolio managers to understand the linkages of FPI and DII investment and stock market volatility to further make effective volatility strategies for their investment returns. This study can be extended in various directions; first high-frequency data gives more insight into market movement. Although high-frequency data on market prices is available yet the high-frequency data on FPIs and DIIs trading is not available. Second, it will be useful to investigate the effects of FPIs and DIIs flow on not just the aggregate level but at the stock-specific level.
Additional information
Funding
Notes on contributors
Bhaskar Chhimwal
Bhaskar Chhimwal is a young scholar in Shailesh j. Mehta school of Management (SJMSOM), Indian Institute of Management Bombay (IIT Bombay), India. His research interest is financial economics, Investment strategies and portfolio management.
Varadraj Bapat
Varadraj Bapat is Associate Professor in Accounting and Finance in Shailesh j. Mehta school of Management (SJMSOM), Indian Institute of Management Bombay (IIT Bombay), India. He has obtained professional qualifications in Chartered Accountancy (with All India Rank), Cost Accountancy, and Information System Audit before obtaining PhD from IIT, Bombay. He has a consulting and teaching experience over 25 years. He has authored many research papers in national and international index journals and has authored many books published by reputed publishers. His areas of research include financial accounting, Managerial accounting, Financial Inclusion, Corporate Governance, Family Business analysis, Portfolio Management, Indian business and economic Model. He is member of Board of Governors for IIT Guwahati and NIT Manipur.
Notes
1. Henry (Citation2000) reported that stock market liberalizations increase private investment booms in developing countries, which leads to an increase in profitability and reduce the cost of capital in these countries. Foreign portfolio investment increased from USD 1638 Million in 1993–1994 to USD 251,545 Million in December 2017 whereas mutual fund investment increased from INR 62,076 Cr in 1993–1994 to INR 15,395,928 Cr in December 2017. Security and exchange board of India (SEBI): Handbook of statistics.
2. Based on this research the following hypotheses are very pronounced—the base-broadening hypothesis, the price-pressure hypothesis and the positive feedback hypothesis (Apte & Badrinath, Citation2003; Clerk & Berko, Citation1997). The base-broadening hypothesis suggests that the expansion of investor-base leads to the increased level of diversification which reduces the systematic risk, required risk premium cost of capital and, increase expected return (Errunza & Losq, Citation1985, Citation1989; Merton, Citation1987). The entry of foreign portfolio investors in emerging markets also reduces the perceived liquidity risk in the market (Allen & Gale, Citation1991; Hargis, Citation1995; Pagano, Citation1989). Thus base-broadening hypothesis suggests a long-term cointegrating relationship between the flow of foreign portfolio investment and aggregate level of stock-prices with causality running from investment flow to stock prices. Warther (Citation1995) in his price-pressure hypothesis suggests that temporary illiquidity increase in the share price and predicts that this change in share price is subsequently reversed. According to positive feedback trading investors take information signals from the recent past price patterns and form their extrapolative expectation about the future prices therefore, the investment-flow lags behind the returns (Brennan & Cao, Citation1997). Warther (Citation1995), Edelen and Warner (Citation2001), and Watson and Wickramanayake (Citation2012) study and find a positive relationship between aggregate fund flows and market returns.
3. Nifty 50 is the index of large-cap firms so Nifty 50 is used as synonymous of Nifty largecap50.
4. To tackle the high variability, we took natural logarithm of the entire variable. Hence the study uses natural logarithm of all variable for modelling.
5. The lags have been selected after evaluating other lags as it pronounced by Box Jenson methodology.
6. The LB statistic for residuals of the model for cumulative autocorrelations up to lag 10 is small but significant at 5 percent. However, the LB statistic for squared residuals is not significant which shows that the TGARCH (1, 1) is sufficient to capture the ARCH effect in the return series.
7. Purchase ratio is defined as the gross purchase by FPIs divided by gross purchase By DIIs.
8. Sales ratio is defined as the gross sales by FPIs divided by gross sales By DIIs.
9. Full equation model used for the study is described in appendix.
References
- Agarwal, R. N. (1997). Foreign portfolio investment in some developing countries: A study of determinants and macroeconomic impact. Indian Economic Review, 32(2), 217–14. https://www.jstor.org/stable/29794138?casa_token=DDqoiMcONKAAAAAA:A1UavK72ZJSzvIzbIEU0wcq6HJXPyWwIPhuqUwuiL3-UGotKFRwT4820ftlhMyF3drg1TnaNM5-ajEX5AFXGIVB9P7W8_h5SaZa8goPIhyp-eufcS-4a&seq=1#metadata_info_tab_contents
- Ahmed, K. M., Ashraf, S., & Ahmed, A. (2005). An empirical investigation of FPI’s role in the indian equity market. IFCAI Journal of Applied Finance, 11(8), 21–33. https://papers.ssrn.com/sol3/papers.cfm?abstract_id=1702855
- Allen, F., & Gale, D. (1991). Limited market participation and volatility of asset prices. American Economic Review, 84, 933–955. https://www.jstor.org/stable/2118039casa_token=pfsHqIsccMIAAAAA:LPFBRQQg3GJgLytAPU6yTy2C0uMUHerlO0fXUup7OG1int0HCO80K4aMiP90lTH1GTUkk7Kw8wCmOrnJJt4l_dwV29i1o8kEyYkkPJvDp1vOH93Po3&seq=1#metadata_info_tab_contents
- Apte, P. G., & Badrinath, H. R. (2003, December 18–19). Impact of FPI investment flows on stock prices. Seventh annual capital markets conference, Mumbai: UTI Institute of Capital Markets.
- Avramov, D., Chordia, T., & Goyal, A. (2006). The impact of trades on daily volatility. The Review of Financial Studies, 19(4), 1241–1277. https://doi.org/10.1093/rfs/hhj027
- Badhani, K. N. (2006). Dynamic relationship among stock prices, exchange rate and net FPI investment flow in India. Prajnan, 35(3), 231–248.
- Bae, K. H., Chan, K., & Ng, A. (2004). Investibility and return volatility. Journal of Financial Economics, 71(2), 239–263. https://doi.org/10.1016/S0304-405X(03)00166-1
- Barber, B. M., & Odean, T. (2000). Trading is hazardous to your wealth: The common stock investment performance of individual investors. The Journal of Finance, 55(2), 773–806. https://doi.org/10.1111/0022-1082.00226
- Barber, B. M., Odean, T., & Zhu, N. (2009). Systematic noise. Journal of Financial Markets, 12(4), 547–569. https://doi.org/10.1016/j.finmar.2009.03.003
- Batra, A. (2003, December 18–19). The dynamics of foreign portfolio inflows and equity returns in India. Seventh annual capital market conference, Mumbai: UTI Institute of Capital Markets
- Bessembinder, H., & Seguin, P. J. (1993). Price volatility, trading volume, and market depth: Evidence from futures markets. Journal of Financial and Quantitative Analysis, 28(1), 21–39. https://doi.org/10.2307/2331149
- Brennan, M., & Cao, H. (1997). International portfolio investment flow. Journal of Finance, 52(5), 1815–1880. https://doi.org/10.1111/j.1540-6261.1997.tb02744.x
- Chakrabarti, R. (2001). FPI flow to India: Nature and causes. Money and Finance, 2,(7). https://papers.ssrn.com/soL3/papers.cfm?abstract_id=649852
- Chakrabarti, R., & Roll, R. (2002). East Asia and Europe during the 1997 Asian collapse: A clinical syudy of a financial crisis. Journal of Fonancial Markets, 5(1), 1–30. https://doi.org/10.1016/S1386-4181(01)00022-2
- Choe, H., Kho, B.-C., & Stulz, R. M. (1999, October). Do foreign investors destabilize stock markets? The Korean experience in 1997. Journal of Financial Economics, 54(2), 227–264. Elsevier. https://doi.org/10.1016/S0304-405X(99)00037-9.
- Clerk, J., & Berko, E. (1997). Foreign investment fluctuation and emerging market stock returns: The Case of Mexico. (Staff Report No. 24). Federal Reserve Bank of New York.
- Daigler, R. T., & Wiley, M. K. (1999). The impact of trader type on the futures volatility‐volume relation. The Journal of Finance, 54(6), 2297–2316. https://doi.org/10.1111/0022-1082.00189
- De Long, B., Shleifer, A., Summers, L., & Waldmann, R. (1990). Noise trader risk in financial markets. Journal of Political Economy, 98(4),703–738. https://doi.org/10.1086/261703
- De Santis, G., & Imrohoroglu, S. (1997). Stock returns and volatility in emerging financial markets. Journal of International Money and Finance, 16(4), 561–579. https://doi.org/10.1016/S0261-5606(97)00020-X
- Dhingra, V. S., Gandhi, S., & Bulsara, H. P. (2016). Foreign institutional investments in India: An empirical analysis of dynamic interactions with stock market return and volatility. IIMB Management Review, 28(4), 212–224. https://doi.org/10.1016/j.iimb.2016.10.001
- Edelen, R. M., & Warner, J. B. (2001). Aggregate price effects of institutional trading: A study of mutual fund flow and market returns. Journal of Financial Economics, 59(2), 195–220. https://doi.org/10.1016/S0304-405X(00)00085-4
- Errunza, V. (2001). Foreign portfolio equity investments, financial liberalization and economic development. Special Issue of Review of International Economics 9(4), 703–726. https://doi.org/10.1111/1467-9396.00308
- Errunza, V., & Losq, E. (1985). International assets pricing under mild segmentation: Theory and test. Journal of Finance, 40(1), 105–124. https://doi.org/10.1111/j.1540-6261.1985.tb04939.x
- Errunza, V., & Losq, E. (1989). Capital flow controls international assets pricing and investors’ welfare: A multi country framework. Journal of Finance, 44(4), 1025–1037. https://doi.org/10.1111/j.1540-6261.1989.tb02636.x
- Foucault, T., Sraer, D., & Thesmar, D. J. (2011). Individual investors and volatility. The Journal of Finance, 66(4), 1369–1406. https://doi.org/10.1111/j.1540-6261.2011.01668.x
- Gabaix, X., Gopikrishnan, P., Plerou, V., & Stanley, H. E. (2006). Institutional investors and stock market volatility. The Quarterly Journal of Economics, 121(2), 461–504. https://doi.org/10.1162/qjec.2006.121.2.461
- Geert, B., & Harvey Campbell, R. (1998). Capital flows and the behaviour of emerging market equity returns. NBER Chapters. In Capital flows and the emerging economies: Theory, evidence, and controversies (pp. 159–194). National Bureau of Economic Research, Inc.
- Glosten, L. R., Jagannathan, R., & Runkle, D. E. (1993). On the relation between the expected value and the volatility of the nominal excess return on stocks. The Journal of Finance, 48(5), 1779–1801. https://doi.org/10.1111/j.1540-6261.1993.tb05128.x
- Gordon, J., & Gupta, P. (2003, January). Portfolio flows into India: Do domestic fundamentals matter? (IMF Working Paper 03/20). International Monetary Fund.
- Hargis, K. (1995). The internationalization of emerging equity markets: Domestic market development or retardation (Working Paper). University of Illinois.
- Henry, P. B. (2000). Stock market liberalization, economic reform, and emerging market equity prices. The Journal of Finance, 55(2), 529–564. https://doi.org/10.1111/0022-1082.00219
- Jo, G. J. (2002). Foreign equity investment in Korea. Association of Korean Economic Studies.
- Kim, E. H., & Singal, V. (2000). Stock market openings: Experience of emerging economies. Journal of Business, 73(1), 25–66. https://doi.org/10.1086/209631
- Kim, W., & Wei, S. (2002). Foreign portfolio investment before and during a crisis. Journal of International Economics, 56(1), 77–96. https://doi.org/10.1016/S0022-1996(01)00109-X
- Krugman, P. (1998, September 7). Saving Asia: It’s time of get radical. Fortune.
- Levine, R., & Zervos, S. J. (1995). Capital control liberalization and stock market performance [Unpublished]. World Bank.
- Mazumdar, T. (2004). FPI inflow to India: Their effect on stock market liquidity. IFCAI Journal of Applied Finance, 10(7), 5–20. https://www.iupindia.in/704/IJAF_FII_Inflows_to_%20India_Stock_Market_Liquidity_Volatility_5.html
- Merton, R. C. (1987). A simple model of capital market equilibrium with incomplete information. Journal of Finance, 42(3), 483–510. https://doi.org/10.1111/j.1540-6261.1987.tb04565.x
- Pagano, M. (1989). Endogenous market thinness and stock price volatility. Review of Economic Studies, 56(2), 269–287. https://doi.org/10.2307/2297461
- Panda, C. (2005). An emperical analysis of the impact of FPIs on investment in the Indian stock market. IFCAI Journal of Applied Finance, 11(1), 53–61.
- Rao, D., & Sensharma, R. (2005, March 17–18). FPI inflow, stock returns and monetary policy in India: Exploring the interlinkages [ Paper Presentation]. Conference on accounting and finance, Indian Institute of Management.
- Stiglitz, J. (1998, March 25). Boats, planes and capital flows. Financial Times.
- Trivedi, P., & Nair, A. (2003, January 30–February 1). Determinants of FPI investment inflow to India [ Paper Presentation]. 5th annual conference on monday & finance in The Indian Economy, Indra Gandhi Institute of Development Research (IGIDR).
- Warther, V. A. (1995). Aggregete mutual fund flow and security return. Journal of Financial Economics, 39(2–3), 209–235. https://doi.org/10.1016/0304-405X(95)00827-2
- Watson, J., & Wickramanayake, J. (2012). The relationship between aggregate managed fund flows and share market returns in Australia. Journal of International Financial Markets, Institutions and Money, 22(3), 451–472. https://doi.org/10.1016/j.intfin.2012.02.001
Appendix
To estimate Tables – we modify EquationEquation (7)(7)
(7) as given below for Nifty 50, Nifty midcap50 and, Nifty small-cap50 respectively.
Where, R(FPIt) is the unexpected FPIs flow at time t, R(DIIt) is the unexpected DIIs flow at time t, R(FPIP,t) is the expected FPI purchase at time t, R(DIIP,t) is the unexpected DIIs purchase at time t, R(FPIs,t) is the unexpected FPIs sell at time t, R(DIIS,t) is the unexpected DIIs sell at time t and, PRt is the purchase ratio and SRt is the sell ratio.