Abstract
Electronic banking services have not been widely used by most bank customers in Ethiopia; most bank customers continue to conduct most of their banking transactions using traditional methods. Therefore, the main objective of this study is to identify factors that affect customers’ usage of electronic banking services. The type of research applied in this study is explanatory in nature. A research model was developed by integrating the constructs of Technology Acceptance Model (TAM) and Theory of Planned Behavior (TPB). A total of 420 actual users of e-banking services were used as a sample. A well-structured questionnaire was used to collect the relevant information. The data analyzed using Structural Equation Modeling revealed that perceived usefulness, perceived ease of use, attitude towards e-banking, perceived behavioral control, subjective norms, behavioral intention, awareness as well as the availability of internet/network connection have a significant positive impact on users e-banking usage practice. However, perceived risk has a significant negative impact. These findings suggest that banks in Ethiopia should create awareness to their customers with regard to the usage and benefits of e-banking service delivery channels.
PUBLIC INTEREST STATEMENT
The adoption of e-banking services by bank customers is in an infant stage in Ethiopia. Therefore, this study is intended to identify the factors that affect the adoption of this service delivery channel from bank customers’ perspective in Ethiopia. Thus, this study will help both banks and customers to benefit from the adoption of this technology. It will also give direction for policymakers to improve the infrastructures required for the effective adoption of e-banking services in the banking industry. Once the potential influential factors are identified and appropriate solutions are taken by banks, customers will be benefited from their adoption of the technology. Further, it helps to fill significant knowledge gaps about e-banking landscape in Ethiopia; thereby it will give insight to researchers and students about the problems and stimulate further investigation of the issue.
1. Introduction
E-banking can be simply defined to mean a process where banks create a platform for its customers to generally access information and to transact businesses electronically through an electronic device without necessarily being present at the bank (Annin et al., Citation2013). Electronic banking enhances the development of the financial system in general and the banking industry in particular, and it is considered as a strategic weapon for banks to cop up with today’s stiff competition (Al-Smadi, Citation2012). Although it provides various benefits for both banks and customers, low level of customers’ adoption of electronic banking services is noted in Ethiopia (Garedachew, Citation2010). However, electronic banking services cannot achieve expected benefits if it is not adopted or used by banking customers (Venkatesh et al., Citation2003). According to Rogers and Shoemaker (Citation1971) and in view of this study, adoption is defined as the acceptance and continued use of a product, service or idea (like e-banking for the current study). As investigated by previous technology acceptance studies, there are many factors that have been suggested and to influence individuals to accept a new technology. For example, studies conducted by Al-Smadi (Citation2012), Baraghani (Citation2008), and Yitbarek and Zeleke (Citation2013) concerning the factors that affect the adoption of e-banking among bank customers by integrating TAM and TPB revealed that perceived usefulness, perceived ease of use, subjective norms, perceived behavioral control and attitude have a positive and significant impact on customers’ behavioral intention and thereby on their actual e-banking usage or adoption behavior. Kamel and Hassen (Citation2003) stated that perceived risk is among the factors that negatively affect consumers’ intention and their e-banking service usage behavior. With regard to the different factors, most of the scholars include in their study limited variables. For example, some researchers conduct their study only by using Technology Acceptance Model (Mir et al., Citation2013; Davis, Citation1989; Akinyemi et al., Citation2013; Jahangir & Begum, Citation2008) and theory of planned behavior (Yaghoubi & Bahmani, Citation2011; Gholami et al., Citation2010;Tan et al., Citation2010) constructs separately and few studies conducted by integrating Technology Acceptance Model and Theory of Planned Behavior (Al-Smadi, Citation2012; Yaghoubi & Bahmani, Citation2010) but although information technology is changing drastically the way in which people live and particularly banks offer their products and services in most developed countries, studies conducted in Ethiopia to identify factors that affect users’ adoption of new technology (e-banking) are remaining scarce. Therefore, the main objective of this study is to identify the factors that affect the usage of electronic banking services among the banking customers (only users’) in Ethiopia. This would deepen the knowledge of the factors which influence either positively or negatively the customers’ attempt to use electronic banking services. In order to achieve this main objective a research model was developed from both theoretical and empirical literature search. Accordingly, this study proposes a comprehensive research or conceptual model that integrates the constructs derived from the Technology Acceptance Model (TAM) and Theory of Planned Behavior (TPB) together with perceived risk, availability and quality of internet/network connection and awareness. This integration is credible fill limitation of previous empirical studies and so that to increase the body of knowledge in this area and to apply structural equation modeling (SEM) method of analysis.
In order to develop a comprehensive research conceptual model that can be able to fill the previous studies limitation or gap various theoretical and empirical studies related to technology acceptance in electronic banking context were reviewed. Based on these reviews, the most appropriate theory and significant factors that influence usage of e-banking from bank customers’ viewpoint were determined and a model of the factors inducing customers’ usage of electronic banking service is developed which is as shown below in Figure . Recently, a growing body of research has focused on integrating Technology Acceptance Model (TAM) developed by Davis (Citation1989) and Theory of Planned Behavior (TPB) developed by Ajzen (Citation1991) to examine IT usage and e-service acceptance because the two models are complementary (Sanayei & Bahmani, Citation2012; Lee, Citation2008; Al-Smadi, Citation2012). Taylor and Todd (Citation1995) also argued that despite TAM and TPB have been widely used to examine information system applications, neither one of them have been found to provide consistently superior explanation or behavior prediction. In line with this Al-Smadi (Citation2012) suggested that an integrated model may provide more explanatory power than the individual use of TAM and TPB. Even the integrated model itself may not sufficient to get more predictive power because Aboelmaged and Gebba (Citation2013) on their study suggested that the integrated model of TAM and TPB constructs have low predictive power with regard to users’ adoption of new technology and hence they recommend that future researchers should include more variables beyond TAM and TPB when predicting e-banking adoption. Therefore, in this research model constructs derived from TAM and TPB models as well as other important factors resulting from the existing literature review were included. Accordingly, based on the above justification, the researcher has developed an extension of the Technology Acceptance Model and Theory of Planned Behavior integrated model by incorporating additional constructs such as awareness, perceived risk and availability and quality of internet/network connection from the extant literature review.
In the conceptual model developed, constructs are identified as either exogenous or endogenous. Then to demonstrate the relationship easily, they are depicted visually in the path diagram, where straight arrows portray the impact of one construct on another or representing the dependence relationship between the cause and the effect variable. Concerning the context of the path diagram, even though, a number of prior studies (Al-Smadi, Citation2012; Yitbarek & Zeleke, Citation2013; Yaghoubi & Bahmani, Citation2010; Olatokun & Owoeye, Citation2012; Khanifar et al., Citation2012; Liao et al., Citation2010; Moga, Citation2010; Poursaleh & Parhizgar, Citation2014; Yaghoubi & Bahmani, Citation2011) were conducted by considering behavioral intention as an endogenous variable, in this study actual usage behavior was used as the ultimate endogenous or dependent variable because Bagozzi (Citation2007) justified that intention may not be representative enough of actual use, because the time period between intention and adoption could be full of uncertainties and other factors that might influence an individual’s decision to adopt a technology, rather he stated that actual usage behavior should be used as a dependent variable and should be predicted by behavioral intention. Actual usage behavior used as a dependent variable distinguished from previous studies refers to the manifest, observable response in a given situation with respect to a given target (Ajzen & Fishbein, Citation1980). Perceived ease of use, perceived usefulness, subjective norms, perceived behavioral control, perceived risk, awareness and availability and quality of internet/network connection constructs are identified as exogenous variables because they are similar to independent variables in the regression model, while perceived usefulness, attitude and behavioral intention were used as mediating variables (both as exogenous and endogenous variable) that mediates the relationship between the dependent and independent variables. Intention is hypothesized to act as a mediator between all relationships of exogenous variables and actual usage behavior.
1.1. Description of variables and hypotheses formulation
1.1.1. Perceived usefulness (PU)
It is defined as “the degree to which a person believes that using a particular system would enhance his or her job performance (Davis, Citation1989). The importance of perceived usefulness has been widely recognized in the field of e-banking. According to the previous research studies usefulness is the subjective probability that the application of a new technology would improve the way a user could complete a given task (Singh, Citation2012). There is also broad research that presents evidence of the significant impact of perceived usefulness on user acceptance of e-banking. The positive effect of perceived usefulness on customers attitude as well as their intention to adopt e-banking services has been validated in many prior studies (Al-Smadi, Citation2012; Qureshi et al., Citation2008; Elgahwash, Citation2013; Yitbarek & Zeleke, Citation2013; Singh, Citation2012; Akinyemi et al., Citation2013) and in this study, it is hypothesized that:
H1a:Perceived usefulness has positive effect on customers’ attitude towards the usage of e-banking services.
H1b:Perceived usefulness has positive effect on customers’ intention to use e-banking services.
H1c:Perceived usefulness mediates the positive effect of perceived ease of use on attitude towards e-banking usage.
1.1.2. Perceived ease of use (PEU)
Perceived ease of use (PEU) is the degree to which a person believes that using a particular information system or information technology would be free of effort (Davis, Citation1989). Hence, an application perceived to be easier to use than another is more likely to be accepted by users. The significant impact of perceived ease of use on usage intention from the preceding research provided evidence that it is either directly or indirectly through its effect on perceived usefulness and attitude that perceived ease of use would have a positive effect on users’ perception of credibility in their interaction with the e-banking systems (Al-Smadi, Citation2012; Qureshi et al., Citation2008; Olatokun & Owoeye, Citation2012; Aderonke & Charles, Citation2010; Singh, Citation2012; Poursaleh & Parhizgar, Citation2014). Hence in this study, it is hypothesized that:
H2a:Perceived ease of use has positive effect on customers’ attitude to use e-banking services.
H2b:Perceived ease of use has positive effect on customers’ perceived usefulness of e-banking usage.
1.1.3. Subjective norm
It refers to an individual’s “perception of social pressure to perform or not to perform the behavior in question (Ajzen, Citation1988, p. 132). There is extensive empirical evidence that supports the significant effect of subjective norms on the intention to use e-banking services (Khanifar et al., Citation2012; Al-Smadi, Citation2012; Yitbarek & Zeleke, Citation2013). Therefore, in this study, it is hypothesized that:
H3:Subjective norm has positive effect on customers’ intention to use e-banking services.
1.1.4. Perceived behavioral control
Perceived behavioral control refers to an individual’s perceptions of the presence or absence of the requisite resources or opportunities necessary for performing a behavior (Ajzen, Citation1985).The significant effect of perceived behavioral control on e-banking usage was investigated by a number of scholars (Yitbarek & Zeleke, Citation2013; Khanifar et al., Citation2012; Al-Smadi, Citation2012; Yaghoubi & Bahmani, Citation2010). Hence in this study, it is hypothesized that:
H4a:Perceived behavioral control has positive effect on customers’ intention to use e-banking services.
H4b:Perceived behavioral control has positive effect on customers’ e-banking usage behavior.
1.1.5. Perceived risk
It is defined as the potential for loss in the pursuit of a desired outcome of using electronic services. It is one of the factors that affect users adoption of e-banking and it can be viewed through several dimensions such as security risk and privacy risk. Security risk is generally associated with loss of personnel data or money and errors in transactions whereas privacy risk is associated with violation of customers’ privacy such as disseminating customer information to other (Featherman & Pavlou, Citation2002). The effect of perceived risk on customers’ usage or adoption of e-banking services has been validated in many prior studies such as (Yitbarek & Zeleke, Citation2013; Lee, Citation2008; Olatokun & Owoeye, Citation2012; Abenet, Citation2010; and Nasri, Citation2011). Hence in this study, it is hypothesized that:
H5a:Perceived risk has negative effect on customers’ behavioral intention to use e-banking services.
H5b:Perceived risk has negative effect on customers’ e-banking usage behavioral.
1.1.6. Attitude (ATT)
Attitude explains a person’s favorable or unfavorable assessment regarding the usage of the service or the person positive or negative feeling about e-banking usage (Davis et al., Citation1989). An individual will hold a favorable attitude toward a given behavior if he/she believes that the performance of the behavior will lead to mostly positive outcomes. Attitude plays an important role in influencing an individual’s intention to adopt a new technology. The direct as well as the mediating effect of attitude on customers’ intention to adopt e-banking services has been validated in many prior studies such as Al-Smadi (Citation2012), Qureshi et al. (Citation2008), Olatokun and Owoeye (Citation2012), Aderonke and Charles (Citation2010), and Poursaleh and Parhizgar (Citation2014). Therefore, for the current study, it is hypothesized that:
H6a:Attitude has positive direct effect on customers’ intention to use e-banking.
H6b:Attitude mediates the positive effect of perceived usefulness on intention to use e-banking services
H6c:Attitude mediates the positive effect of perceived ease of use on intention to use e-banking services
1.1.7. Awareness
Awareness refers to the amount of information consumers have about e-banking and its benefit (Pikkarainen et al., Citation2004) or it refers to the degree to which the users are informed about the existence of the new technological innovation (Fonchamnyo, Citation2013). According to Pikkarainen et al. (Citation2004) the amount of information about e-banking (particularly internet banking) and its benefit is a determinant factor in motivating customers to use e-banking services. The effect of awareness on customers’ adoption of e-banking services has been validated in many prior studies such as (Al- Somali et al., Citation2011; Oye et al., Citation2009; Safeena & Date, Citation2010; Geetha and Malarvizih, Citationn.d.; Makosana, Citation2014; and Azouzi, Citation2009). Hence for the current study, it is hypothesized that:
H7a:Awareness has positive effect on customer’s e-banking usage behavior.
1.1.8. Availability and quality of internet and network connection
Availability and quality of internet connection is a prerequisite for the adoption of e-banking services. Several prior studies were confirmed the impact of the availability and quality of internet and network connection on e-banking usage (Amini et al., Citation2011; Pikkarainen et al., Citation2004; Al- Somali et al., Citation2011) and in this study, it is hypothesized that:
H8a:Availability and Quality of internet connection has a significant effect on customers’ e-banking usage behavior.
1.1.9. Behavioral intention
It refers to the cognitive representation of a person’s readiness to perform a given behavior, and it is considered to be the immediate antecedent of actual usage behavior (Fishbein and Ajzen, Citation1975). The direct as well as the mediating effect of intention on users actual usage behavior has been validated in many prior empirical studies conducted by using Theory of planned behavior (TPB) and Technology acceptance model (TAM) and both suggested that a person’s behavior is determined by his/her intention to perform the behavior. The best predictor of behavior is intention. There are numerous related past studies that have found a significant relationship between intention and behavior (I. Ajzen, Citation1985, Citation1991; Tan et al., Citation2012; Venkatesh et al., Citation2003). Therefore, in this study, it is hypothesized that:
H9a: Intention has positive direct effect on customers’ actual usage behavior.
H9b: Intention mediates the relationship between attitude toward the behavior and usage behavior
H9c: Intention mediates the relationship between subjective norm and usage behavior
H9d: Intention mediates the relationship between perceived behavior control and usage behavior
H9e: Intention mediates the relationship between perceived usefulness and usage behavior
H9f: Intention mediates the relationship between perceived risk and usage behavior
2. Research methodology
2.1. Population and sampling
The sampling population was defined as customers’ of Ethiopian commercial banks who are using at least one form of e-banking channels. Both private and public commercial bank customers located in Addis Ababa, Ethiopia were included as a target population. The research was carried out in Addis Ababa as a representative geographical area of the population of Ethiopia. Purposive sampling technique was used to select the target population for this study (i.e., only the customers of those banks that are providing e-banking services by using at least two forms of e-banking channels as well as those banks who started offering the service before a year were included in the sample). When this research was conducted, there are 16 commercial banks in Ethiopia. However, based on the above criteria samples were taken from seven banks such as commercial bank of Ethiopia, Dashen Bank S.C, Wogagen bank S.C, United Bank S.C, Abyssinia Bank S.C, Abay Bank S.C and Zemen Bank S.C. Of which one is government bank (commercial bank of Ethiopia) and the remaining six are private banks. Then, random sampling was used to select sample respondents. The necessary sample size was estimated based on the number of independent variables. Based on the recommendations of Joseph et al. (Citation2010), the sample size should be 15–20 observations per variable for generalization purposes. Krejcie and Morgan (Citation1970) also recommended that for a population having more than 1,000,000 target groups a sample size of 384 is acceptable. Hence, based on these justifications, and by giving allowance for errors and non-response rates, a total of 600 (40 variables*15 observation for each variable) respondents were considered as the acceptable sample size for the current study. However, among these much of questionnaires distributed only 495 were returned which gives a response rate of 82.5% but after removing those incomplete questionnaires, the actual sample size used for analysis in this study were 420 respondents (70%).
2.2. Measurement development
This explanatory or casual study used the survey method to collect the required cross-sectional primary data. A self-administered questionnaire was developed based on preliminary semi- structured interview with selected bank employees and extensive literature review to identify the factors that affect bank customers’ usage of e-banking services. All items incorporated in the research questionnaire were measured using a five-point Likert scales, ranging from strongly disagree (1) to strongly agree (5). To ensure content validity, the constructs in the research model were operationalized through items validated in prior research studies with significant modifications (Baraghani, Citation2008; Elgahwash, Citation2013; Venkatesh et al., Citation2003; Talukder et al., Citation2014; Davis, Citation1989; Al- Somali et al., Citation2011; Olatokun & Owoeye, Citation2012; Al-Smadi, Citation2012; Al- Somali et al., Citation2011; Pikkarainen et al., Citation2004; Tan et al., Citation2012). In addition, structured interview was conducted with e-banking department heads or managers of each respective banks in order to collect qualitative data that can able to substantiate the data collected using a structured questionnaire. Secondary data obtained from related-published journals, online articles, books and international conference papers were also used.
2.3. Method of data analysis
Once the data is collected, coded, entered and cleaned; it goes through both descriptive and quantitative data analysis techniques. Descriptive techniques involved the use of descriptive statistics whereas quantitative techniques involved two steps. In the first step the reliability and validity of variables in the research model were assessed using Confirmatory Factor Analysis (CFA) or measurement model. To check reliability Cronbach’s alpha and construct reliability tests were used. The rule of thumb for either reliability test (Cronbach’s alpha or construct reliability) estimate is that 0.7 or higher suggests good reliability. Reliability between 0.6 and 0.7 may be acceptable provided that other indicators of a model’s construct validity are good. The presence of high construct reliability indicates that internal consistency exists, meaning that all the measurement items are consistently representing the same latent construct (Joseph et al., Citation2010). The convergent validity of the measures were tested using factor loadings, Average variance extracted (AVE) and construct reliability and at a minimum all factor loadings and AVE values should be statistically significant and a good rule of thumb is that standardized factor loadings and AVE estimates should be 0.5 or higher (Joseph et al., Citation2010) whereas its discriminant validity was assessed by comparing the AVE values for any two constructs with the square of correlation estimate between these two constructs and then, to ensure discriminant validity, the average variance extracted estimates should be greater than the squared correlation estimate (Joseph et al., Citation2010). Then, based on the results in step one, the data were analyzed in step two by applying Structural Equation Modeling (SEM) using Maximum Likelihood Estimation. These entire tests were performed with SPSS plus AMOS version 21 and Excel is used to calculate AVE and Construct Reliability (CR). The goodness of fit assumptions of using SEM was met. In order to evaluate the fitness of the measurement and the structural model; Chi-Square and its associated degrees of freedom, RMSEA, Normed Chi-Square as well as TLI and CFI were used as GOF criteria because in line with this Tabachnick and Fidell (Citation2007) and Joseph et al. (Citation2010) suggested that in order to evaluate the acceptability of the model, the researcher should report one absolute fit index (RMSEA or SRMR), one incremental fit index (CFI or TLI), one goodness of fit index (CFI or TLI) and one badness of fit index (RMSEA or SRMR) together with Chi-Square and its associated degrees of freedom.
3. Data analysis and findings
3.1. Demographic profile of respondents
The table shows the demographic profile of respondents. Accordingly, it indicated that more than half of the respondents are males (243, 57.9%). This implies that males have better e-banking usage experience as compared to females. In line with this finding, a study conducted by Alagheband (Citation2006) to identify factors affecting the adoption of e-banking services indicated that men represent the segment with the highest usage of e-banking (Milion, Citation2013: Abrehe, Citation2015 and Muzividzi et al., Citation2013).
With regard to age category, majority of the respondents are young with the age category of 18–32 (75.7%). This suggests that majority of the e-banking users are young or in other words young customers have better e-banking usage practice as compared to old customers. Consistent with this, a study conducted by Abenet (Citation2010) concerning the determinants of e-banking (like internet banking) adoption in Ethiopia revealed that the young age group is more computer literate and finds it easy to accept and use new technologies. Poon (Citation2008) and Azouzi (2009) on their study also supports that young and computer literate respondents are using or are willing to use electronic banking. Related to educational level, majority of the respondents are first degree and above holders (328, 78.1%). This also suggests that educated peoples having a first degree and above educational level have better e-banking usage experience as compared to those who have below first degree educational qualification which suggests that educational level is a factor for e-banking adoption. In line with this, Edwin et al. (Citation2014) on their study found that consumers’ level of education and information communication technology knowledge impacts their acceptance of e-banking services (Tater et al., Citation2011 and Abrehe, 2015). In relation to marital status more than half of the respondents are single or not married (238, 56.7%). This implies that not married or single customers have better e-banking usage practice as compared to married and divorced counter parts which is in line with Izogo et al. (Citation2012) in Nigeria and Abrehe (2015) in Ethiopia.
The tables also indicate the average monthly income category of respondents and it revealed that most of them have an average monthly net income of between 4000 and 6000 (44%) Ethiopian birr. This implies that majority of the e-banking users are those who have better average monthly net income. In line with this Ismail and Osman (Citation2012) on their study investigated that e-banking usage is associated with clients’ income. High-income clients and those who are computer and internet literate are more likely to use e-banking services (Poon, 2008 and Annin et al., Citation2013).
Finally, the table shows the occupational status of respondents and it revealed that more number of respondents (185, 44%) were government employees which indicate that government employees have better e-banking usage practice as compared to others. Similarly, Alagheband (2006) and Milion (2013) also found that higher users’ of e-banking (like credit card) have been evident for government employees rather than other types of employments.
The descriptive statistics result as shown above in Table indicated that the mean values for perceived usefulness, perceived ease of use, subjective norms, attitude, perceived behavioral control and behavioral intention were above 3.0. This shows respondents’ positive response to these constructs whereas the mean value of above 3.0 for the construct perceived risk implies that users have high-risk perception about e-banking. However, there is a problem of accessing quality internet or network connection (mean = 2.428 and std.dev = 0.961) because the mean value of this variable is below average. Finally, the tables revealed that due to their perception of high risk about e-banking (mean = 3.618 and std.dev = 1.378), awareness problem (mean = 3.049 and std.dev = 1.240) and problem of accessing internet or network connection, users’ e-banking usage practice is average (mean = 3.032 and std.dev = 1.00).This implies that bank customers’ are not using e-banking service delivery channels frequently and always or in other words they are not fully utilizing the service. The standard deviations for most of the variables were less than one which indicates that the item scores for each construct were distributed around the mean score or it shows the normality distribution of the sample.
Table 1. Demographic profile of respondents
The measure of goodness of fit result of the measurement model (Figure ) is presented above in table and it indicated that except for chi-square the remaining GOF measure indices are within the range of the recommended benchmark fit index value (Joseph et al., Citation2010). In addition, the multicollinearity test result attached in the appendix part (Table A2) indicated that the maximum correlation between constructs is 0.542 which implies that there is no multicollinearity problem among the variables because Joseph et al. (Citation2010) and Tabachnick and Fidell (Citation2007) suggested that variables having a correlation coefficient of 0.9 and above are subjected to multicollinearity problem.
3.2. Assessing the validity of the measurement model
The construct validity of the measurement model is assessed and discussed in terms of its convergent and discriminant validity. The existence of convergent validity is checked by using standardized factor loadings, Average Variance Extracted (AVE) and reliability test through Cronbach’s alpha and construct reliability test (CR) (Joseph et al., Citation2010). The result of all these tests as presented below in the appendix section (Table A1) indicated that all the construct reliability and Cronbach’s alpha test results are above the minimum threshold cut of value of 0.7 and above (Joseph et al., Citation2010). Their factor loadings result is also statistically significant and above the cut of value of 0.5 or higher. The high loadings suggest that the indicators converge on a common point and the indicators are strongly related to their associated constructs. However, those items having factor loading results below the minimum cut of value of 0.5 are deleted as suggested by Joseph et al. (Citation2010) and Tabachnick and Fidell (Citation2007). With regard to the variance extracted in each construct except perceived usefulness all the remaining constructs have average variance extracted of above the minimum cut of value of 0.5 as recommended by Joseph et al. (Citation2010). However, given other convergent validity tests above the cut of value, the average variance extracted value of 0.423 for perceived usefulness has no significant impact on further analysis. Therefore, all these tests imply that the measurement model has satisfied the requirement of convergent validity.
The discriminant validity of the constructs which shows the extent to which a construct is truly distinct from other constructs is tested and presented under the appendix section (Table A3). As stated in the research methodology part, the existence of discriminant validity among the construct is evaluated by comparing the average variance extracted of two constructs with their respective squared correlation estimate and the average variance extracted value should be greater than their squared correlation result for a truly distinct construct (Joseph et al., Citation2010). Accordingly, all the average variance extracted (AVE) values of two constructs are greater than their respective squared correlation result. In other words, all the constructs in the measurement model are statistically different from each other which supports the existence of good discriminant validity power of the constructs included in the model. All this results implies that the measurement model is valid and can proceed to further structural model testing.
The simplest diagnostic test used to check normality is a visual check of normal probability plot. The normal distribution forms a straight diagonal line and to check whether the data is normal or not the plotted data values are compared with the diagonal. If a distribution is normal, the actual data distribution line closely follows the diagonal line (Tabachnick & Fidell, Citation2007; Joseph et al., Citation2010). In line with this, in this study, normal probability test and histogram was used to assess the normality of the data (attached under the appendix part) and the result indicated that there is no significant departure from normality, however, slight departure is expected in social science researches (Pallant, Citation2011).
3.3. Specifying the structural model
Once the measurement model is developed and tested for its validity, the next stage of SEM analysis involves specifying the structural model by assigning relationships from one construct to another (from exogenous latent constructs to endogenous constructs) based on the proposed theoretical model but to minimize the number of pages in this article even though the SEM process go through model re-specification process, the results of the re-specified structural model are presented and discussed below.
3.4. Assessing the validity of the structural model
The validity of the re-specified model is also assessed based on the goodness of measure indices and its corresponding structural path coefficients. Accordingly, the goodness of fit indices and the result of the structural relationship (Figure ) between the exogenous and endogenous latent constructs as discussed above are presented below.
As shown above (Table ), except for chi-square which indicates significance p-value, all the goodness of fit measure fit indices are above their respective minimum cut of value (Joseph et al., Citation2010). In principle, the chi-square statistics value as a fundamental measure of differences between the observed and estimated covariance matrices (goodness of fit measure) should be insignificant which shows the absence of significance difference between the observed and estimated covariance. On the other hand, a p-value of below 0.05 indicates that the two covariance matrices are statistically different and shows problems with model fitness (Joseph et al., Citation2010). However, Hair et al. suggested that the statistical test or resulting p-value is less meaningful as sample sizes become large (greater than 250) or the number of observed variables becomes large (greater than or equal to 30) and in this case the researcher should expect significant p-value result. Similarly, Stevens (Citation2009) also suggested that the large chi-square values may be due, at least in part, to the large sample size, rather than to any substantial misspecification of the model. Accordingly, in this study having a sample size of 420 and 40 indicators, the presence of significant p-value has no significant impact on the fitness of the model.
The results of the structural model parameter estimates as presented above indicated that all the hypothesized relationships between the exogenous and endogenous latent constructs are supported which shows the validity of the structural model as well as it provides support for the hypothesis regarding the directional linkage among the variables included in the research model because in line with this finding Joseph et al. (Citation2010) suggested that a theoretical model is considered valid to the extent that the parameter estimates are statistically significant and in the predicted direction. Except for perceived risk which has a negative significant effect on behavioral intention and actual usage behavior, all the other exogenous constructs have a positive significant effect on their respective endogenous constructs.
4. Results and discussions of findings
In order to investigate the effect of different factors included in the research model on customer’s e-banking usage behavior, the research model was tested with the application of Structural Equation Modeling (SEM). In general, as recommended by Joseph et al. (Citation2010) in SEM, a two-step approaches than a single-step approach was used. Firstly, the measurement model was evaluated using CFA to ensure that the items used to measure each of the constructs are adequate as well as to check the validity and reliability of the indicators. The second step involves the assessment of the structural model which shows the relationships between the constructs. In the following section, the structural path estimate results of the re-specified structural model are discussed below with respect to each construct incorporated in the research model.
4.1. Perceived ease of use and perceived usefulness
The effect of perceived ease of use on perceived usefulness and individual’s attitude towards e-banking service delivery channels was tested and the result indicated that perceived ease of use has a positive significant direct effect. This implies that the usefulness perception of e-banking users is highly and significantly influenced by their perception towards the easiness or user-friendly of the technology. Further, it implies that other things being equal, the easier a certain technology (i.e., e-banking) can be learned or used, the more useful it will be perceived by users’. This result is supported by the Technology Acceptance Model (TAM) developed by Davis (Citation1989) because this theory of technology acceptance stated that perceived ease of use and perceived usefulness directly and positively affect the attitudes towards the acceptance of a given information system. In addition to its impact on attitude and behavioral intention, perceived ease of use has also a positive effect on perceived usefulness. Similarly, Baraghani (Citation2008) on his empirical study found that perceived usefulness is predicted by perceived ease of use and his finding imply that consumers use e-banking for the benefits and also due to its easiness to use it. However, contrary to this finding, Yitbarek and Zeleke (Citation2013) found that perceived usefulness is not predicted by perceived ease of use.
The effect of perceived ease of use on user’s attitude towards e-banking is also significant and it implies that the higher perceived ease of utilizing a particular e-banking service delivery channels makes it more likely that the individual will have a positive feeling toward using it. In line with the finding of this study, Yaghoubi and Bahmani (Citation2010) on their empirical study and Davis (Citation1989) on his technology acceptance theory indicated that perceived ease of use does not have a direct impact on intention to use, although it affects the attitude and perceived usefulness, which in turn leads to greater acceptance of online banking. This implies that difficulty in using online systems is becoming less of a concern as they are increasingly user-friendly.
The construct perceived usefulness has a direct positive and significant effect on user’s attitude towards e-banking and on their behavioral intention to use e-banking services which is in line with the technology acceptance model developed by Davis (Citation1989). This implies that customer’s attitude towards e-banking services as well as their behavioral intention to use e-banking services are highly and directly influenced by their perception towards the usefulness of the technology. Again the implication is that the more the technology is useful (perceived as time-saving, convenient and help to manage banking transactions effectively); the better becomes their attitude and behavioral intention to adopt the technology (e-banking). As indicated by its higher beta coefficient in the table above, the effect of perceived usefulness on users attitude towards e-banking is more than that of perceived ease of use. Therefore, it implies that perceived usefulness appears to be the more important direct predictor of users’ attitude towards e-banking usage. In line with this, Taylor and Todd (Citation1995) in their study found that perceived usefulness has a significant direct influence on behavioral intention. Similarly, Al-Smadi (Citation2012) found that perceived usefulness and perceived ease of use has a positive and significant impact on customers’ attitude toward electronic banking services. People shape their attitude positive or negative when they think a particular technology is beneficial and require less effort to deal (Khurshid et al., Citation2014).
4.2. Attitude towards E-banking
Users behavioral intention to use e-banking services is directly, significantly and positively influenced by their attitude towards e-banking which is in line with the theory of planned behavior developed by Ajzen (Citation1988) and technology acceptance model proposed by Davis (Citation1989). This finding indicates that the better the customer’s attitude (developing positive feeling) towards e-banking, the more becomes their behavioral intention to use the service. In consistent with this finding, Al-Smadi (Citation2012), Baraghani (Citation2008), Yitbarek and Zeleke (Citation2013), and Poursaleh and Parhizgar (Citation2014) on their empirical study investigated that attitude has a positive and significant influence on customers’ intention to use electronic banking services. Contrary to this, Taylor and Todd (Citation1995) in their study found that attitude does not have a significant influence on behavioral intention.
4.3. Perceived behavioral control and subjective norms
The influence of perceived behavioral control on user’s behavioral intention to adopt e-banking as well as on their actual usage behavior was tested and the result reveals that both behavioral intention and actual usage behavior are directly, positively and significantly influenced by users perceived behavioral control. This result is strongly supported by the Theory of Planned Behavior proposed by Ajzen (Citation1988). This empirical finding implies that the more users have the required knowledge, skill and other resources such as mobile phone and computer, the better becomes their behavioral intention as well as their actual e-banking usage practice and vice versa. This is due to the belief of most users’ that having the skills, resources and knowledge is an important requirement to operate electronic banking services (Al-Smadi, Citation2012). Similarly, the effect of subjective norms or social influence on user’s behavioral intention to adopt e-banking services was tested and the result showed that behavioral intention is directly, positively and significantly influenced by subjective norms (Ajzen, Citation1988). It implies that many users are adopted e-banking due to the existence of social pressure from their friends, families, colleagues and other service user community or it implies that most bank customers associate certain degree of risk with e-banking services as a result their utilization of e-banking services is more influenced by the words of friends and relatives than by the words of bank employees or advertisements since they believe that the former will give them more accurate and trust worthy feedback than the latter (Agarwal, Rastogi and Mehrotra, Citation2009). In consistent with this study finding, a number of researchers also found a similar result. For example, a study conducted by Yaghoubi and Bahmani (Citation2011) to evaluate the causality of TPB factors on influencing customers’ adoption of e-banking services using structural equation modeling shows that attitude, perceived behavioral control and subjective norm have significant positive effects on behavioral intention to use e-banking.
4.4. Awareness
As indicated in Table , awareness has a direct positive and significant effect on the user’s actual e-banking usage behavior. The implication is that the more the customers become aware about e-banking service delivery channels (having enough information about its benefit and how to use it); the better becomes their actual usage behavior. In line with this, a study conducted by Padachi et al. (Citation2007) to identify factors affecting the adoption of e-banking found that there is a statistically significant relationship between awareness and the usage of internet banking. Qureshi et al. (Citation2008), Safeena and Date (Citation2010) and Makosana (Citation2014) on their study also suggested that the amount of information provided to the customers by different means like advertisement through print and electronic media about e-banking is useful in customer acceptance of the technology. In line with this Pikkarainen et al. (Citation2004) also identified that the amount of information users have on online banking is the most influential factor explaining the use of online banking services. This finding refers to the fact that while consumers get more information about e-banking, the more informative they become about the benefits it offers.
Table 2. Descriptive statistics of variables included in the research model
Table 3. Goodness of fit measure results of the CFA measurement model
Table 4. Goodness of fit measure results of the structural model
Table 5. Summary of direct effect results of the structural model
Table A2. Correlation matrix among the latent constructs
Table A3. Correlation squared and average variance extracted matrix (Discriminant validity) of the constructs included in the research model
4.5. Availability of quality internet or network connection
The availability or accessibility of quality internet or network connection has direct positive and significant influence on users’ e-banking actual usage behavior. The implication is that users’ actual e-banking usage practice is highly and significantly influenced by the existence of quality internet or network connection. That means the more the accessibility of internet or network connection, the better becomes their usage behavior and vice versa or in other words, it implies that the existence of fast, accessible and consistent network/internet connection facilitates the rate of e-banking adoption among the banking community. In line with this, Folorunso et al. (Citation2010) on their study identified that poor internet facility and network as well as the high cost of internet has a significant direct and indirect impact on customers’ usage of card banking. Similarly, a study conducted by Auta (Citation2010) to identify factors affecting e-banking adoption among bank customers also found that a shortage of telecommunication facilities are among the major factors that influence consumers adoption of e-banking.
4.6. Perceived risk
The impact of perceived risk on users behavioral intention towards e-banking as well as on their actual usage behavior was hypothesized and tested in the structural research model and the findings showed that perceived risk has a direct negative and significant influence on users behavioral intention to adopt e-banking as well as on their actual usage behavior. These findings which is supported by the Theory of Perceived Risk developed by Featherman and Pavlou (Citation2002) indicated that users’ behavioral intention and their actual usage practice is significantly influenced by their e-banking risk perception which implies that the more customers perceive e-banking as risky, the less becomes their behavioral intention to use as well as their actual usage behavior or it imply that using e-banking as a means of conducting banking transactions is not safe and secured as a result users’ prefer to go to the bank branch to do banking transactions. Further, this finding implies that e-banking users’ are not confident in electronic banking services. Customers are not willing to take any risk, and as a result they want to keep away from risks. This is because electronic banking services are in inherently risky environment due to the absence of personal contact, physical product evaluation, warranties, or contracts and the customers usually have difficulties in asking for compensation when transaction error occurs (Al-Smadi, 201 2 and Pavithran et al., Citation2014).
4.7. Behavioral intention
Finally, the influence of behavioral intention to use e-banking on users’ actual usage behavior was tested and the finding reveals that users’ actual usage behavior is directly and positively influenced by their behavioral intention which is in line with Theory of Reasoned Action (TRA) Proposed by Fishbein & Ajzen (1975), The Theory of Planned Behavior (TPB) proposed by I. Ajzen (Citation1988), the Decomposed Theory of Planned Behavior (DTPB) proposed by Taylor and Todd (Citation1995), Technology Acceptance Model (TAM) proposed by Davis (Citation1989) and Extended Technology Acceptance Model (ETAM) developed by Venkatesh and Davis (Citation2000). All these technology acceptance theories as well as the findings in this study implies that the better users have positive behavioral intention to continue and increase its usage than branch-based traditional banking, the more become their actual usage practice and vice versa. In line with this finding, Talukder et al. (Citation2014) on their study investigated that behavioral intention is a direct predictor of usage behavior. Similarly, in addition to behavioral intention, the direct effect of awareness, availability of quality internet/network connection, perceived behavioral control and perceived risk on users’ actual e-banking behavior was tested and as discussed above one by one the result indicated that both these variables have a significant direct effect on consumers actual usage behavior. The effect of availability of internet/network connection on consumers’ actual usage behavior is more than other variables. Therefore, this implies that availability of internet/network connection is the more important predictor of consumers’ actual e-banking usage behavior in Ethiopia. However, due to the individuals’ low level of e-banking usage practice, the relationship between behavioral intention and actual usage practice is less than the relationship between attitude and behavioral intention. This implies that even though the current actual usage behavior of the individuals’ is low due to the above-mentioned factors, their intention to use e-banking services more effectively in the future is high.
5. Conclusion and recommendation
Based on the results discussed above it is possible to conclude that perceived behavioral control, behavioral intention, subjective norms, attitude towards use, perceived usefulness, perceived ease of use, availability of internet/network connection and awareness have a significant positive impact on customers usage of e-banking service delivery channels, however, perceived risk has a negative significant impact. Hence, as a researcher it is suggested that the currently available basic infrastructure (ICT) for e-banking diffusion is not sufficient to full fill the requirements of e-banking technology in Ethiopia. Therefore, efforts to improve this basic infrastructure by the government should be strengthened both in terms of coverage and quality. Further, all banks should aggressively create continuous awareness to the society with regard to the usage and benefits (usefulness) of e-banking services by using different media. Finally, in order to reduce customers’ frustration concerning the security of e-banking channels (especially internet and mobile banking), banks in Ethiopia need to develop risk-reducing strategies.
6. Limitations and directions for future study
Even though this research is unique with regard to the application of structural equation modeling as well as the usage of the integration of two well-known technology acceptance models (theory of planned behavior and technology acceptance model) and three additional important factors from the view point of Ethiopian banking customers, the major limitations include: Exclusion of the voice of non-users (those who are not using e-banking), as the electronic banking service delivery channels covered in this research, are so broad and not specific to each e-banking channel there is a possibility that the different factors considered influencing e-banking usage behavior as well as the challenges identified may be for a certain part of e-banking channels and not the whole of it. For instance, a user may perceive that internet banking is more risky than ATM. In addition, the results of this study might have been influenced by the early introduction of ATMs in Ethiopian banking sector as compared to other e-banking channels such as mobile and internet banking. Thus, future researchers should consider these limitations as an opportunity and should, therefore, include the views of non-users as well as conducting comparative studies focusing on differences in adoption behavior between the different forms of e-banking service delivery channels (such as ATM, internet banking and mobile banking).
Additional information
Funding
Notes on contributors
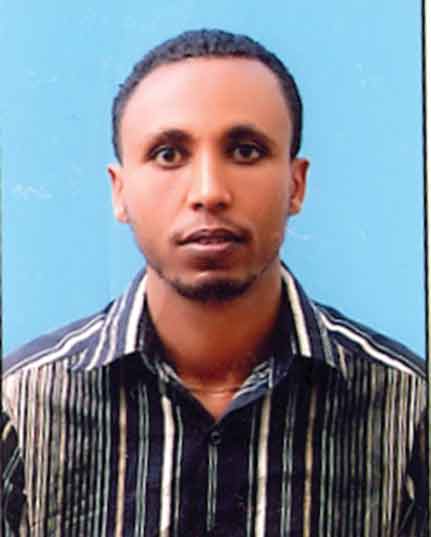
Beza Muche Teka
This is to inform you that the manuscript entitled as Factors Affecting Bank Customers Usage of Electronic Banking in Ethiopia: Application of Structural Equation Modeling (SEM) is my own research work. Currently I am working as an assistant professor under the department of accounting and finance, Debremarkos University, Ethiopia. I have more than 13 years teaching and research experience. I have six (6) published research outputs in different international journals. The adoption of e-banking services by bank customers is in an infant stage in Ethiopia. Therefore, the identification of influential factors will help both banks and customers to benefit from the adoption of this technology. It will also give direction for policy makers to improve the infrastructures required for the effective adoption of e-banking services in the banking industry. Further, it helps to fill significant knowledge gaps about e-banking landscape in Ethiopia; thereby it will give insight to researchers and students about the problems and stimulate further investigation of the issue.
References
- Abenet, Y. (2010). Key factors that determine adoption of internet banking in Ethiopia. Retrieved from http://etd.aau.edu.et/dspace/bitstream/123456789/31501
- Aboelmaged, M. G., & Gebba, T. R. (2013). Mobile banking adoption: An examination of technology acceptance model and theory of planned behavior. International Journal of Business Research and Development, 2(1), 35‐50. https://doi.org/10.24102/ijbrd.v2i1.263
- Abrehe, F. (2015). ATM adoption of customers in commercial bank of Ethiopia (CBE), Mekelle. Journal of Business Management & Social Sciences Research (JBM&SSR), 4(5), 365–27.
- Aderonke, A., & Charles, K. (2010). An empirical investigation of the level of users’ acceptance of e-banking in Nigeria. Journal of Internet Banking and Commerce, 15(1), 1–13. http://www.arraydev.com/commerce/jibc/
- Agarwal, R., Rastogi, S., and Mehrotra, A. (2009). Customers’ perspectives regarding e-banking in an emerging economy. Journal of Retailing and Consumer Services, 16(5), 340–351.
- Ajzen, I. (1985). From intentions to actions: A theory of planned behavior. In J. Kuhl & J. Beckmann (Eds.), Action control: From cognition to behavior (pp. 11–39). Springer-Verlag.
- Ajzen, I. (1988). Attitudes, personality and behavior. Dorsey Press.
- Ajzen, I. (1991). The theory of planned behavior. Organizational Behavior and Human Decision Processes, 50(2), 179–211. https://doi.org/10.1016/0749-5978(91)90020-T
- Ajzen, I., & Fishbein, M. (1980). Understanding attitudes and predicting social behavior. Prentice-Hall.
- Alagheband, P. (2006). Adoption of electronic banking services by Iranian customers. Retrieved from http://epubl.ltu.se/1653-0187/2006/49/LTU-PB-EX-0649-SE.pdf
- Akinyemi, I. O., Asani, E. O., & Adigun, A. A. (2013). An Investigation of users’ acceptance and satisfaction of e-banking system as a panacea towards a cashless economy in Nigeria. Journal of Emerging Trends in Computing and Information Sciences, 4(12), 954–963. http://eprints.covenantuniversity.edu.ng/2704/#.Xvb3YigzbIU
- Al- Somali, A., Gholami, R., & Clegg, B. (2011). Internet banking acceptance in the context of developing countries: An extension of the technology acceptance model. In Operations & information management group, aston business school, Birmingham B47ET, UK (pp. 1–16).
- Al-Smadi, O. (2012). Factors affecting adoption of electronic banking: An analysis of the perspectives of banks’ customers. International Journal of Business and Social Science, 3(17), 294–309. https://www.researchgate.net/publication/285005684
- Amini, M. T., Ahmadinejad, M., & Azizi, M. J. (2011). Adoption of internet banking by Iranian customer: An empirical investigation. The International Journal of Management Science and Information Technology (IJMSIT), 1(1), 47–64. https://www.econstor.eu/bitstream/10419/97860/1/785287213.pdf
- Annin, K., Adjepong, O. M., & Senya, S. S. (2013). Applying logistic regression to e-banking usage in Kumasi Metropolis, Ghana. International Journal of Marketing Studies, 6(2), 153–162. http://www.ccsenet.org/journal/index.php/ijms/article/view/31822
- Auta, M. E. (2010). E-banking in developing economy: Empirical evidence from Nigeria. Journal of Applied Quantitative Methods, 5(2), 212–222. http://www.jaqm.ro/issues/volume-5,issue-2/pdfs/auta.pdf
- Azouzi, D. (2009). The adoption of electronic banking in Tunisia: An exploratory study. Journal of Internet Banking and Commerce, 14(3), 1–11.
- Bagozzi, R. P. (2007). The legacy of the technology acceptance model and a proposal for a paradigm shift. Journal of the Association for Information Systems, 8(4), 244–254. https://doi.org/10.17705/1jais.00122
- Baraghani, N. S. (2008). Factors influencing the adoption of internet banking. Master’s thesis. Lulea University of technology, division of industrial marketing and e-commerce Master’s thesis, 2008:099-ISSN 1653-0187-ISREN: LTU-PB-EX—08/099--SE. http://tech.uh.edu/conklin/IS7033Web/7033/Week14/Internetbanking.pdf
- Davis, F. D. (1989). Perceived usefulness, perceived ease of use, and user acceptance of information technology. MIS Quarterly, 13(3), 319–340. https://doi.org/10.2307/249008
- Davis, F. D., Bagozzi, R. P., & Warshaw, P. R. (1989). User acceptance of computer technology: A comparison of two theoretical models. Management Science, 35(8), 982–1003. https://doi.org/10.1287/mnsc.35.8.982
- Edwin, M. A., Ailemen, I. O., Okpara, A., & Mike, O. A. (2014). Impediments to e-banking services marketing in developing economies – A case study of Nigerian banks. European Journal of Business and Social Sciences, 3(3), 228–248. https://www.academia.edu/7676221/
- Elgahwash, O. S. (2013). The role of communication and information technologies in enhancing customer relationships in the Libyan banking industry. University of Wollongong thesis collection, Australia, 96–108.
- Featherman, M. S., & Pavlou, A. P. (2002). Predicting e-services adoption: A perceived risk facets perspective. Eighth America Conference Information System, 1034–1046. http://sighci.org/amcis02/CR/Featherman.pdf
- Fishbein, M. and Ajzen, I. (1975). Belief, attitude, intention and behavior: An introduction to theory and research. Reading, MA: Addison-Wesley.
- Folorunso, O., Ateji, A., & Awe, O. (2010). An exploratory study of the critical factors affecting the acceptability of automated teller machine (ATM) in Nigeria. Annals Computer Science Series, 8(1), 151–162. https://unaab.edu.ng/
- Fonchamnyo, D. C. (2013). customers’ perception of e-banking adoption in Cameroon: An empirical assessment of an extended TAM. International Journal of Economics and Finance, 5(1), 166–176.
- Garedachew, W. (2010). Electronic banking in Ethiopia- practices, opportunities and challenges. Journal of Internet Banking and Commerce, 15(2), 1–8. http://www.arraydev.com/commerce/jibc/
- Geetha, K.T., & Malarvizhi, V. (n.d.). Acceptance of e-banking among customers (an empirical investigation in India). Journal of Management and Science, 2(1), 1–9.
- Gholami, R., Ogun, A., Koh, E., & Lim, J. (2010). Factors affecting e-payment adoption in Nigeria. Journal of Electronic Commerce in Organizations, 8(4), 51–67. https://doi.org/10.4018/jeco.2010100104
- Ismail, M. A., and Osman, M. A. (2012). Factors influencing the adoption of e-banking in Sudan: Perceptions of retail banking clients. Journal of Internet Banking and Commerce, 17(3), 1–16. Retrieved from http://www.arraydev.com/commerce/jibc/
- Izogo, E. E., Nnaemeka, O.C., Onuoha, O.A., and Ezema, K. S. (2012). Impact of demographic variables on consumers’ adoption of e-banking in Nigeria: An empirical investigation. European Journal of Business and Management, 4(17), 27–39.
- Jahangir, N., & Begum, N. (2008). The role of perceived usefulness, perceived ease of use, security and privacy, and customer attitude to engender customer adaptation in the context of electronic banking. African Journal of Business Management, 2(1), 32–40. http://www.academicjournals.org/AJBM
- Joseph, F., Hair, J., Black, W., Babin, C., & Anderson, P. E. (2010). Multivariate Data Analysis (7th ed.). Pearson Prentice Hall.
- Kamel, S., & Hassen, A. (2003). Assessing the introduction of electronic banking in Egypt using the technology acceptance model. Edited by Mehdi Kosrow-Pour. Annals of Cases on Information Technology, 5, 1–26. Retrieved from HYPERLINK “http://www.igi-global.com/chapter/assessing” www.igi-global.com/chapter/assessing -introduction-electronic-banking -Egypt/44530?camid=4v1
- Khanifar, H., Niya, J. M., Jandaghi, G. R., Molavi, Z., & Emami, M. (2012). Factors influencing the intendancy of e-banking: An integration of TAM & TPB with e-service quality. Elixir International Journal of Finance and Management, 46, 8072–8079. www.elixirpublishers.com
- Khurshid, A., Rizwan, M., & Tasneem, E. (2014). Factors contributing towards adoption of e-banking in Pakistan. International Journal of Accounting and Financial Reporting, 1(1), 437–455. https://doi.org/10.5296/ijafr.v4i2.6584
- Krejcie, R., & Morgan, D. (1970). Determining sample size for research activities. Educational and Psychological Measurement, 30(3), 607–610. https://doi.org/10.1177/001316447003000308
- Lee, M. C. (2008). Factors influencing the adoption of internet banking: An integration of TAM and TPB with perceived risk and perceived benefit. Electronic Commerce Research and Applications, 8(3), 1–12. https://www.academia.edu/15023883/
- Liao, C., Lin, N. H., & Liu, P. Y. (2010). Predicting the use of pirated software: A contingency model integrating perceived risk with the theory of planned behavior. Journal of Business Ethics, 91(2), 237–252. https://doi.org/10.1007/s10551-009-0081-5
- Makosana, M. (2014). Customers’ adoption of electronic banking: An investigation on the commercial banking industry in Zimbabwe. International Journal of Economics, Commerce and Management, United Kingdom, 2(7), 1–14. https://mpra.ub.uni-muenchen.de/57351/1/MPRA_paper_57351.pdf
- Mir, R. A., Ara, R., & Dar, A. A. (2013). Customer attitude and factors influencing users acceptance of e-banking in J&K. International Journal of Business and Management Invention, 2(7), 68–78. https://www.ijbmi.org/papers/Vol(2)7/Version-2/H0272068078.pdf
- Milion, A. (2013). The impact of electronic banking on customers’ satisfaction in Ethiopian banking industry (the case of customers of Dashen and wogagen banks in Gondar city). A thesis submitted to faculty of business and economics (FBE) in fulfillment of the requirement for the degree in master of business administration (MBA), 33–57. Retrieved from http://papers.ssrn.com/sol3/papers.cfm?abstract_id=2354281
- Moga, M. L. (2010). The adoption of e-banking: An application of theories and models for technologies acceptance. Journal of Development, Energy, Environment, Economics, 255–259. https://pdfs.semanticscholar.org/6ae1/6343b6c77a3d0fd8b32b4d48f38151cb9cfe.pdf
- Muzividzi, D., Mbizi, R., and Mukwazhe, T. (2013). An analysis of factors that influence internet banking adoption among intellectuals: case of Chinhoyi University of technology, Zimbabwe. Interdisciplinary journal of contemporary research in business, 4(11), 350–369.
- Nasri, W. (2011). Factors influencing the adoption of internet banking in Tunisia. International Journal of Business and Management, 6(8), 143–160.
- Olatokun, W., & Owoeye, O. J. (2012). Influence of individual, organizational and system factors on attitude of online banking users. Proceedings of informing science & IT education conference (InSITE), Africa Regional Centre for Information Science (ARCIS) University of Ibadan, Nigeria, 389–403.
- Oluoch, R. A. (2013). Factors affecting adoption of mobile banking technology in Kenya: A case of bank customers within Nakuru municipality. Asian Journal of Business and Management Sciences, 2(11), 1–13. https://www.semanticscholar.org/paper/
- Oye, N. D., Shakil, M. A., & Iahad, N. A. (2009). E-Banking: A case study of askari commercial bank Pakistan. International Journal of Engineering Research and Applications (IJERA), 1(3), 1152–1167. https://pdfs.semanticscholar.org/06ba/6424f1a9fbada5f360683e4a249cdd728ca3.pdf
- Padachi, K., Rojid, S., & Seetanah, B. (2007). Analyzing the factors that influence the adoption of internet banking in Mauritius. Proceedings of the 2007 Computer Science and IT Education Conference, 560–574. https://www.researchgate.net/publication/228983840
- Pallant, J. (2011). SPSS survival manual a step by step guide to data analysis using SPSS (4th ed.). Allen and Unwin.
- Pavithran, R., Vraprasad, G., Sridharan, R., & Unnithan, A. (2014). An empirical investigation of mobile banking adoption in developing countries. International Journal of Enterprise Information System, 10(1), 76–93. https://doi.org/10.4018/ijeis.2014010105
- Pikkarainen, T., Pikkarainen, K., Karjaluoto, H., & Pahnila, S. (2004). Consumer acceptance of online banking: An extension of the technology acceptance model. Journal of Internet Research, 14(3), 224–235. https://doi.org/10.1108/10662240410542652
- Poursaleh, P., & Parhizgar, M. M. (2014). Factors affecting the intention to use internet banking services. Journal of Social Issues & Humanities, 2(3).
- Poon, W. C. (2008). Users’ adoption of e-banking services: The Malaysian perspective. Journal of Business & Industrial Marketing, 23(1), 59–69.
- Qureshi, T. M., Zafar, M. K., & Khan, M. B. (2008). Customer acceptance of online banking in developing economies. Journal of Internet Banking and Commerce, 13(1), 1–9. http://www.arraydev.com/commerce/jibc/
- Rogers, M. E., & Shoemaker, F. (1971). Communications in innovation. Free press.
- Safeena, R., & Date, H. (2010). Customer perspectives on e-business value: Case study on internet banking. Journal of Internet Banking and Commerce, 15(1), 1–13. http://www.arraydev.com/commerce/jibc/
- Sanayei, A., & Bahmani, E. (2012). Integrating TAM and TPB with perceived risk to measure customers’ acceptance of internet banking. International Journal of Information Science and Management, 10(1), 25–37. https://www.researchgate.net/publication/288270138
- Singh, S. (2012). An empirical investigation of the determinants of users’ acceptance of e-banking in Singapore (a technology acceptance model). International Journal of Management and Business, 2(1), 69–84. http://ijmbr.srbiau.ac.ir/article_64.html
- Stevens, J. P. (2009). Applied multivariate statistics for the Social Sciences (5th ed.). University of Cincinnati: Routledge Taylor and Francis Group.
- Tabachnick, B., & Fidell, L. (2007). Using multivariate statistics (5th ed.). HarperCollins.
- Talukder, M., Quazi, A., & Sathye, M. (2014). Mobile phone banking usage behavior: An Australian perspective. Australasian Accounting, Business and Finance Journal, 8(4), 81–104. https://doi.org/10.14453/aabfj.v8i4.6.
- Tan, B. P., Potamites, R. P., & Chi, W. L. (2012). Applying the TAM to understand the factors affecting use of online banking in the Pescadores. ARPN Journal of Science and Technology, 2(11), 1022–1028.
- Tan, W. G., Chong, C. K., Ooi, K. B., & Chong, Y. L. (2010). The adoption of online banking in Malaysia. An empirical analysis, 169-193. International Journal of Business and Management Science, 3(2), 169–193. http://www.safaworld.org/ijbms/abs/abs3.2/IJBMS0055%20Garry%20169-193%20v3n2%202010SAFA.pdf
- Tater, B., Tanwar, M., and Murari, K. (2011). Customer adoption of banking technology in private banks of India. International Journal of Banking and Finance, 8(3), 72–88.
- Taylor, S., & Todd, P. (1995). Understanding information technology usage: A test of competing models. Information Systems Research, 6(2), 144–176. https://doi.org/10.1287/isre.6.2.144
- Venkatesh, V., & Davis, F. D. (2000). A theoretical extension of the technology acceptance model: Four longitudinal field studies. Management Science, 45(2), 186–204.
- Venkatesh, V., Morris, M. G., Davis, G. B., & Davis, F. D. (2003). User acceptance of information technology: Toward a unified view. MIS Quarterly, 27(3), 425–478. https://doi.org/10.2307/30036540
- Yaghoubi, N. M., & Bahmani, E. (2010). Factors affecting the adoption of online banking an integration of technology acceptance model and theory of planned behavior. International Journal of Business and Management, 5(9), 159–165. https://doi.org/10.5539/ijbm.v5n9p159
- Yaghoubi, N. M., & Bahmani, E. (2011). Behavioral approach to policy making of the internet banking industry: The evaluation of factors influenced on the customers’ adoption of internet banking services. African Journal of Business Management, 5(16), 6785–6792. http://www.academicjournals.org/AJBM
- Yitbarek, T., & Zeleke, S. (2013). Analysis of factors influencing customers’ intention to the adoption of e-banking service channels in Bahir Dar city, Ethiopia: An integration of TAM, TPB and PR. European Scientific Journal, 9(13), 402–417. https://www.researchgate.net/publication/236979221
Appendix
Table A1. Confirmatory factor analysis result of the measurement model
Assessment of Normality
(1) Histogram
(2) Normal probability plot (p-p plot)