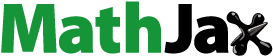
Abstract
In the literature, existing meta-regressions on efficiency have focused on specific sectors in a country or multiple country and on specific economic activity. None of the available efficiency meta-regressions covers multiple sectors of an economy. We contribute to the literature by investigating the technical efficiency differentiation within a multi-sectoral environment. Using data from 152 publications yielding 223 observations from diverse sources and applying meta-regression analysis, we investigated the heterogeneity in mean technical efficiency (MTE), assessed the temporal and spatial drivers of estimated technical efficiency for Ghana. We found heterogeneity in the estimated MTE. The selected cauchit functional form of the fractional regression model showed sectoral and spatial variables drive heterogeneity in MTE. There was a seeming technical efficiency regression with average MTE of 0.676 that requires greater effort in the management of production than has been the case previously in order to close the output gap.
PUBLIC INTEREST STATEMENT
In the literature, none of the available efficiency meta-regressions covers multiple sectors of an economy. This article contributes to the literature by investigating the levels of technical efficiency (TE) of various units and groups of units of economic activities over the years, examine their differences and identify the factors that explain these differences for Ghana. Using data from 152 publications yielding 223 observations, the average of the overall TE was 68% suggesting that the country operated at less than 70% of her full potential over the period 2001 to 2019. We found differences in mean TE across industries studied, geographical locations and nature of the data used. Managers of business units across the country need to attain a higher percentage of the potential output with special attention to the coastal regions of the country.
1. Introduction
According to Farrell (Citation1957), technical efficiency (TE) is the “success in producing maximum output from a given set of inputs” (259). Based on the output perspective, TE can also be perceived as the difference betweenthe optimal output frontier (maximum attainable theoretically or from peers) and that observed for a firm (unit of analysis). To measure TE, two popular approaches have emerged; stochastic frontier analysis (SFA) (Aigner et al., Citation1977; Meeusen & Van Den Broeck, Citation1977) and data envelopment analysis (DEA) (Banker et al., Citation1984; Charnes et al., Citation1978; Fare et al., Citation1983). Whilst the former uses econometric functional estimations, the latter applies linear programming.Footnote1 The understanding of how, or indeed whether, economic agents are efficient is becoming an intriguing field of research in economics because of the fact that efficiency of economic activities fosters economic growth, productive use of resources and profitability (Aiello & Bonanno, Citation2016).
To the best of our knowledge, the first technical efficiency study on Ghana was reported by Gokcekus et al. (Citation2001) in the Journal of Economic Development. Using SFA and 242 cross-sectional observations on wood products, they reported technical efficiency of 0.618. A decade later, in 2011 alone, eight studies were published in several journals with varying characteristics (study descriptors) such as mean technical efficiency (MTE), sample size, geographical area covered, method of estimation and data structure, among others. The methodological tools and the early works have led to a surge in studies that seek to understand the efficiency of economic units in diverse sectors in Ghana. From the foregoing, we raise the following research questions: Is there heterogeneity in mean technical efficiency in Ghana? Which sectors do technical efficiency studies cover in Ghana? Are there temporal differences in the estimated MTEs? Does geographical space play any role in the variation in estimated MTEs? What other factors drive technical efficiency levels in Ghana?
In the literature, existing meta-regressions analysis (MRA) on efficiency has focused on specific sectors in various countries, for example, in agriculture (Djokoto et al., Citation2016 on Ghana; Ogundari & Brummer, Citation2011 on Nigeria; Hina & Bushra, Citation2016 on Pakistan; Geffersa et al., Citation2019; Solomon & Mamo, Citation2019 on Ethiopia), hospitals (Kiadaliri et al., Citation2013 on Iran) and banking (Irsova & Havranek, Citation2010 for the USA). Those that cover multiple countries were also sector specific, agriculture (Africa—Ogundari, Citation2014; developing countries—Thiam et al., Citation2001; World—Bravo-Ureta et al., Citation2007; Djokoto, Citation2015; Mareth et al., Citation2016; Moreira López & Bravo-Ureta, Citation2009; Nandy et al., Citation2019), microfinance (World—Fall et al., Citation2018), hospitals (World—Nguyen & Coelli, Citation2009), education (World—Papadimitriou, Citation2013) and transportation (World—Brons et al., Citation2005; Odeck & Bråthen, Citation2012). None of the available efficiency meta-regressions cover multiple sectors of an economy. We contribute to the literature by investigating the technical efficiency differentiation across sectors of an economy, Ghana.
The next section presents a review of literature. The methodology is captured in section 3. Section 4 contains the results and discussions with associated recommendations. Concluding remarks constitutes the last section.
2. Literature review
2.1. MRA in a nutshell
Glass (Citation1976, p. 3) describes meta-regression analysis (MRA) as “analysis of analysis”. This becomes necessary when studies on a phenomenon abound (Sterne, Citation2009). This methodology provides the opportunity to analyse results from many individual studies with the aim of integrating the findings. As an alternative to causal, narrative discussions and non-quantitative literature review, MRA involves seeking out the large individual studies, collating and isolating the appropriate measures of interest based on the objective of the study. In addition to the goal of combining evidence, MRA enables exploration of the variability in the phenomenon under study and the drivers thereof (Hess & Von Cramon-Taubadel, Citation2008; Nelson & Kennedy, Citation2009). In the case of TE MRA, various MTEs are extracted from the studies reviewed and the corresponding study characteristics identified (Stanley, Citation2005, Citation2008; Stanley & Jarrell, Citation1989). The resulting dataset is fit to a model. Whilst each study may constitute a single observation, multiple MTEs from a study are included as separate observations in the MRA model (Djokoto, Citation2015; Espey et al., Citation1997). The estimation procedures used in the literature have included OLS, Tobit, logistic, truncated regression, transformed truncated regression and fractional regression modelling (Bravo-Ureta et al., Citation2007; Djokoto & Gidiglo, Citation2016; Nandy et al., 2018; Ogundari & Brummer, Citation2011). MRA was originally applied to other disciplines, such as medicine and psychology (Egger et al., Citation1997; CitationHedges & Olkin, 1985; Rosenthal, Citation1984; Thiam et al., Citation2001). In recent times, however, there has been a surge in MRA applications in many fields in economics. Poot (Citation2012) counts 626 papers that applied MRA in economics between 1980 and 2010, with an exponential growth in the 2000s with Thiam et al. (Citation2001) as the first MRA on efficiency. A shortcoming of MRA is that it brings together studies that are very different from one another (Glass, Citation1976; Glass et al., Citation1981). This “apples and oranges” problem can be addressed by refining the identification of the problem to be studied (Aiello & Bonanno, Citation2016).
2.2. Empirical review
The MRAs reviewed here cover 5 out of the 21 sections of the main industries according to the United Nation’s International Standard Industrial Classification (ISIC). These are agriculture, forestry and fishing; transportation and storage; financial and insurance activities; education; and human health and social work activities. The less than 25% coverage points to the need for studies in the other industries in order to create data for MRAs to be conducted in the remaining 16 industries. Out of the 22 MRAs reviewed, 8 relate to specific countries (Ethiopia-2, Ghana-2, Iran-1, Nigeria-1, Pakistan-1, USA-1), regions (Africa-1, developing countries-1) and the world (12). The number of studies comprising the MRAs varied between 22 (Alatawi et al., Citation2019) and 442 (Ogundari, Citation2014) and yielded observations ranging from 25 (Alatawi et al., Citation2019) to 8325 (Papadimitriou, Citation2013). The means were respectively 79 and 590. The high number of observations far exceeding the number of studies found arose from multiple observations from the same study, in some cases (Djokoto, Citation2015; Espey et al., Citation1997). The primary studies in the MRA covered different years, which period ranged from a low of 6 years (Djokoto et al., Citation2016) to a high of 43 (Hina & Bushra, Citation2016) with a mean of 19 years.
Reported average MTE (AMTE) from existing MRAs ranged from 0.600 to 0.863 with an average of 0.732 and standard deviation of 0.075 which implies a high cluster around the mean. The mean of 0.732 suggests, without increasing input usage, the output can be increased by about 27%. All MRAs except Djokoto and Gidiglo (Citation2016) cover one industry. Djokoto and Gidiglo (Citation2016) cover agricultural production and agricultural processing in Ghana hence closer to the current MRA than all others regarding industry. The relevant characteristics that explain MTE in this and other MRAs are reviewed next.
Crop production was found to be more efficient than animal production (Djokoto et al., Citation2016; Nandy et al., Citation2019). On the contrary, Djokoto (Citation2015) found a statistically significant lower efficiency of organic crops as opposed to animal production globally. Yet, Djokoto and Gidiglo (Citation2016), Hina and Bushra (Citation2016), and Ogundari (Citation2014) found no difference between perennial crop and other crop production. There appears to be no difference in the TE of agribusiness activities across geographical regions in Ghana (Djokoto & Gidiglo, Citation2016). Ogundari and Brummer (Citation2011) however reported lower TE in agriculture in southern Nigeria as opposed to the north.
As technology improves overtime time, it is expected that TE will increase over time as well. Whilst Aiello and Bonanno (Citation2016), Djokoto and Gidiglo (Citation2016), Fall et al. (Citation2018), and Ogundari and Brummer (Citation2011) confirmed this, Iliyasu et al. (Citation2014) and Odeck and Bråthen (Citation2012) found the contrary. However, Brons et al. (Citation2005), Djokoto (Citation2015), and Djokoto et al. (Citation2016), Nandy et al. (2018), Solomon and Mamo (Citation2019) and Thiam et al. (Citation2001) found no temporal changes in MTE.
Turning to the control variables; methodological properties, MTE from panel data sets were found to be higher than those from cross-sectional data analysis (Aiello & Bonanno, Citation2016; Bravo-Ureta et al., Citation2007; Brons et al., Citation2005; Fall et al., Citation2018; Nguyen & Coelli, Citation2009; Thiam et al., Citation2001). Brons et al. (Citation2005), Djokoto and Gidiglo (Citation2016) and Hina and Bushra (Citation2016) also found higher TE values for time series data sets than for cross-sectional data sets. The exception, however, is Djokoto (Citation2015) who found statistically significantly lower TE for time series data than for cross-sectional data for organic agriculture across the world. Hina and Bushra (Citation2016) and Moreira López and Bravo-Ureta (Citation2009) reported higher TE from cross-sectional data than for panel data. Interestingly, Djokoto and Gidiglo (Citation2016), Irsova and Havranek (Citation2010), and Mareth et al. (Citation2016) found no significant difference between the two data structures regarding TE. Greene (Citation1993) noted that panel data models are likely to yield more accurate efficiency estimates than other data structures given that there are repeated observations on each unit.
Deterministic models tend to overstate TE estimates (Kumbhakar & Lovell, Citation2000). This is because some of the random errors in the model are accounted for as inefficiency. With improvements in DEA efficiency measurements over time, the difference is expected to narrow (Cook & Seiford, Citation2009; Emrouznejad et al., Citation2008; Kao, Citation2014; Koronakos, Citation2019; Mariz et al., Citation2018). Consequently, TE estimates from SFA models have been found to be lower than those from DEA models (Bravo-Ureta et al., Citation2007; Iliyasu et al., Citation2014; Irsova & Havranek, Citation2010; Odeck & Bråthen, Citation2012). Whilst Alatawi et al. (Citation2019), Brons et al. (Citation2005), Djokoto (Citation2015), and Ogundari (Citation2014) found the contrary, others could not distinguish TE based on SFA and DEA (Djokoto & Gidiglo, Citation2016; Djokoto et al., Citation2016; Fall et al., Citation2018; Kiadaliri et al., Citation2013; Mareth et al., Citation2016; Papadimitriou, Citation2013; Thiam et al., Citation2001).
Generally, high sample size produces more efficient estimates (Greene, Citation2004). Although this does not necessarily lead to lower TE, Aiello and Bonanno (Citation2016), Brons et al. (Citation2005), Fall et al. (Citation2018), Kiadaliri et al. (Citation2013), Nguyen and Coelli (Citation2009), Ogundari and Brummer (Citation2011), and Solomon and Mamo (Citation2019) found lower TE with high levels of sample size. Whilst none of the existing TE MRAs reported the reverse, Djokoto (Citation2015), Djokoto et al. (Citation2016), and Iliyasu et al. (Citation2014), Nandy et al. (2018), Odeck and Bråthen (Citation2012) and Thiam et al. (Citation2001) found no statistically significant relationship between TE and sample size.
Dissemination is an important part of the research process, and productivity and TE studies are no exceptions. Consequently, there are some common ways of dissemination of research such as thesis, working papers, conference papers and journals. Whilst Djokoto (Citation2015) and Solomon and Mamo (Citation2019) concluded that there are no differences in MTE regarding dissemination outlet, Aiello and Bonanno (Citation2016), Djokoto and Gidiglo (Citation2016), Djokoto et al. (Citation2016), Geffersa et al. (Citation2019), and Ogundari (Citation2014) found variation across these dissemination media. Specifically, Djokoto et al. (Citation2016), Geffersa et al. (Citation2019), and Ogundari (Citation2014) found higher TE from journals as opposed to other dissemination media. Aiello and Bonanno (Citation2016) and Djokoto and Gidiglo (Citation2016) reported the contrary.
From the foregoing, the influence of study characteristics on the variations in MTE is not uniform across all studies. This may be attributable to the different sectors studied, spatial coverage, period of study as well as modelling of the MRA. Thus, whilst the findings of the current MRA could be consistent with the previous studies in some respects, there is bound to be a departure in some other respects.
3. Methodology
To improve transparency and to raise the quality of MRA, the meta-analysis of economics research-network (MAER-Net) has created reporting guidelines (Stanley et al., Citation2013). The data and methods section follows these guidelines.
3.1. Literature search, compilation and coding
Databases and publishers’ websites such as EBSCOhost, Scopus, AgEcon search, Google scholar, Wileyonlinelibrary, EmeraldInsight among others were searched for literature. The keywords included “Efficiency” and “Ghana”, “Data Envelopment Analysis” and “Ghana”, “DEA” and “Ghana”, “Stochastic Frontier Analysis” and “Ghana”, “SFA” and “Ghana”, “Frontier Analysis” and “Ghana”, “inefficiency” and “Ghana”, “Stochastic frontier” and “Ghana”. The search which ended at 10:31GMT on 22 May 2019 yielded 2112 publications.
To be included in the survey, TE should have been measured by SFA or DEA and its associated procedures. Further, the studies should have reported on the sector that the data relates to and the geographical coverage. Application of this inclusion criteria and elimination of duplicates resulted in 152 publications and 223 observations (See Supplementary file). Except the MTEs, sample sizes and year of publication, all other variables were coded 0 and 1 (dummy variables) as appropriate, as detailed below. The coding was accomplished by one author and checked by the other authors.
Dummy variables are useful in capturing factors that determine the study-to-study variation in the MTE (Nelson & Kennedy, Citation2009). For example, Thiam et al. (Citation2001), used 10 out of total of 13 covariates; Bravo-Ureta et al. (Citation2007), 12 out of 13; Ogundari and Brummer (Citation2011), 10 out of 14; Ogundari (Citation2014), 14 out of 17. Therefore, the multiplicity of dummy variables in the technical efficiency MRA specified above and described below is not out of place. The statistical significance or otherwise of dummies may have research and policy implications.
3.2. Modelling
The general model to respond to the last three research questions is
We defined sectors in Ghana based on the 3-digit level of activities of the International Standard Industrial Classification (ISIC) of All Economic Activities Revision 4 (United Nations, Citation2008) to capture the various economic activities. NONPERR (011) refers to non-perennial crops, assigned 1 and 0 otherwise. PERR (012) is 1, capturing perennial crops and 0 otherwise. CROPS capture a combination of both perennials and non-perennials and equal 1 and 0 otherwise. ANIM (014) refers to animal production and assigned 1 and 0 otherwise. The reference is mixed farming (015). Marine and freshwater fishing (FISH, 031) = 1 and 0 else. The reference is aquaculture (032). Regarding section C, manufacturing of the ISIC, Food manufacturing is represented as FOODMANU (101–107) and is 1 and 0 otherwise. FOODMANU captured several 3-digit activities because the primary studies did not specify the specific food manufacturing such as meat, fish, vegetables, etc. Textile manufacturing is TEXTMANU (131 and 139, 141–143) defined as 1 and 0 otherwise. WOOD (161–162) represents wood products defined as 1 and 0 otherwise. PHARMANU (210) represents the manufacture of pharmaceuticals, medicinal chemical and botanical products and captured as 1 and 0 else. The reference is other manufacturing not elsewhere classified (n.e.c.) (329). Regarding section H of the ISIC, transportation and storage, other land transport (TRANSPT, 492) is 1 and 0 otherwise. Turning to division K, financial and insurance activities; monetary intermediation (banking, credit union, microfinance) recognised as INTERMED (641) is 1 and 0 otherwise. The reference is insurance services (651). PUBADM (841) referring to administration of the State and the economic and social policy of the community is 1 and 0 else. EDUCPRIM (851) refers to pre-primary and primary education and is assigned 1 and 0 else. Educational support activities including libraries are EDUCSUP (855) = 1 and 0 otherwise. The reference is other education (854). HOSP (861) described by hospital activities is 1 and 0 otherwise. The reference is medical and dental practice activities (excluding hospitals, including health centres) (862).
For space (spatial), areas covering the coastal regions are COASTL = 1 and 0 otherwise. The MID = 1 refers to Ashanti, Brong-Ahafo and Eastern Regions and 0 otherwise. NORTH refers to Northern, Upper East and Upper West Regions. These together were assigned 1 and 0 otherwise. The primary studies were reported prior to the creation of new administrative Regions; hence, the old Regions were adhered to in this work. Studies that used data across any two of the three divisions or all Regions are the reference.
There were some gaps in the year of data used in the primary studies as would have been observed in the supplementary file. Whilst some studies did not report the period or year the data used referred to, others reported groups of periods, whilst others reported production period spanning 2 years. A linear correlation coefficient was estimated between the year of publication and some of the specific years of data reported. The coefficient of 0.666 was statistically significant at 1%. The year of publication (DYEAR) was then used as a proxy for a year of data.
Dissemination of research output includes journal, conference, working paper and thesis. JOURNAL = 1 if the paper was disseminated in a journal and 0 otherwise. CONF = 1 refers to conference papers and 0 otherwise. WP = 1 refers to working papers and 0 otherwise. The reference is THESIS.
Methodological characteristics of the primary studies: DEA = 1 for studies that used data envelopment analysis and 0 otherwise (SFA). XSECTION = 1 captures cross-section data structure and 0 otherwise. TSERIES = 1 represents time series data structure and 0 otherwise. The reference is panel data. SSIZE is sample size of the primary study.
3.3. Issues with MRA
Some issues are associated with MRA; publication selection bias, possible heteroscedasticity and non-independence of multiple observations from a study. To detect possible publication bias, funnel plot can be used. This is a simple scatter diagram of the variables of interest, such as effect size (MTE in this case), versus a measure of precision (Chaimani et al., Citation2014). Owing to subjectivity in the interpretation of the funnel plot, a more objective approach is to estimate EquationEquation 2(2)
(2) :
where is the overall effect size and
is the measure of asymmetry of the funnel plot and a strength of publication bias and
is the error term (see Stanley, Citation2005; Stanley & Doucouliagos, Citation2012; Egger et al., Citation1997; Rose & Stanley, Citation2005). Although the popular measures of precision include estimated standard error, standard deviation and variances (Fall et al., Citation2018; Ogundari et al., Citation2012), some studies do not report these (Ogundari & Brummer, Citation2011; Ogundari, Citation2014). Therefore, the sample size and its variants such as the square root and its inverse which are often reported in primary studies can be used (Fall et al., Citation2018; Ogundari et al., Citation2012). The underlying assumption behind EquationEquation 2
(2)
(2) is that
≠ 0 implies publication bias and
≠ 0 denotes a genuine empirical effect of the MTE estimates across the chosen studies. In the absence of publication bias, reported MTE is expected to vary randomly around the true MTE estimate, while the presence of a genuine empirical effect suggests that the estimate has been corrected for publication bias/selection across the primary studies. A solution to publication bias is to include studies from other sources such as conference papers, working papers and thesis as well including other relevant covariates in the MRA model (Aiello & Bonanno, Citation2016; Appiah-Adu & Djokoto, Citation2015).
With MRA, the metadata set incorporates studies that reported more than one MTE estimates from the same study (Aiello & Bonanno, Citation2016; Espey et al., Citation1997). Consequently, the independence of the values of the outcome variables across the observations cannot be guaranteed which can lead to biased standard errors (Aiello & Bonanno, Citation2016; Thiam et al., Citation2001). Espey et al. (Citation1997) however noted that multiple observations not exceeding five can be accommodated. No study contributed more than five observations to our metadata set.
Finally, as primary studies might have used different data sets, different sets of variables and different sample sizes, errors of the resulting MRA are less likely to be constant (homoscedastic) (Moreira López & Bravo-Ureta, Citation2009). An approach to assess possible heteroscedasticity with fractional regression modelling is to estimate a heteroscedastic fractional model, say, probit, as below (Alvarez & Brehm, Citation1997; Freeman et al., Citation2015; Harvey, Citation1976):
where z is a vector of covariate(s) that explains the variance of the error term, are parameters of the variance function. The detailed specification is
SSIZE is used as a covariate for the variance because it is a proxy for the standard error, that is often used to weight the error term to a non-varying state in the case of weighted regression. A test for heteroscedasticity involves a simple likelihood ratio test (Davidson & MacKinnon, Citation1984; Engle, Citation1984). The test compares an unrestricted model (the heteroscedastic probit, LH) to a restricted model (ordinary probit, Lo). The null hypothesis is that the error variances are homoscedastic (that is, ), indicating that an ordinary probit will suffice. The alternative hypothesis is that
). This test is implemented using an LR test.
The result of the probit model is applicable to other functional forms of the fractional regression model (FRM).
3.4. Estimation procedure
Ordinary least squares (OLS) has been used in estimating MRAs (Brons et al., Citation2005; Iliyasu et al., Citation2014; Kiadaliri et al., Citation2013; Nguyen & Coelli, Citation2009; Papadimitriou, Citation2013). Bravo-Ureta et al. (Citation2007), Fall et al. (Citation2018), Mareth et al. (Citation2016), Moreira López and Bravo-Ureta (Citation2009), and Thiam et al. (Citation2001) justified the use of two limit Tobit procedure because of the censoring of efficiency scores between 0 and 1. The use of Tobit assumes an unobserved latent variable of interest, y*, such that -∞ < y* < +∞. What is observed rather is y, defined as y = 0 if y* ≤ 0, y = y*if 0 < y* <1 and y = 1 if y* ≥1. There is, therefore, a linear relationship between the y* and the covariates, as in EquationEquation 6(6)
(6) .
where x is a vector of covariates whilst are parameters. The linearity condition of the OLS is unlikely to be satisfied regarding the estimation of the effects of covariates on efficiency measures as in EquationEquation 6
(6)
(6) (Ramalho et al., Citation2010). Given the bounded nature of the inefficiency scores, the marginal effect of unit changes in xj on efficiency score is given by EquationEquation 7
(7)
(7) ,
which is constant over the range of y and is incompatible with the non-linear properties of efficiency scores. Papke and Wooldridge (Citation1996) rightly proposed FRM that overcomes the problems with linear and Tobit procedures outlined above since McDonald (Citation2009) showed that the data generation process (DGP) of efficiency scores is not censored but fractional. The model is useful for handling efficiency scores as dependent variables, observable within the unit interval. Geffersa et al. (Citation2019), Ogundari (Citation2014), and Solomon and Mamo (Citation2019) used the FRM with a priori functional form. However, Djokoto (Citation2015), Djokoto and Gidiglo (Citation2016), and Djokoto et al. (Citation2016) empirically selected the appropriate one. The FRM is specified as in EquationEquation 8(8)
(8) .
where G(.) is some nonlinear function satisfying 0 ≤ G(.) ≤ 1. The G (.) could be specified as any cumulative distribution function such as logit and probit (Papke & Wooldridge, Citation1996) and as log-log, cloglog and cauchit (Ramalho et al., Citation2010). Logit,
Log-log,
Complementary log-log (cloglog),
Cauchit
Partial effect for all specifications is
Unlike EquationEquation 7(7)
(7) , EquationEquation 14
(14)
(14) varies with g (xθ) satisfying the non-linear properties of the technical efficiency measure. Equations 9–13 are estimable by quasi maximum likelihood estimation (QML) (Papke & Wooldridge, Citation1996). However, Ramalho et al. (Citation2010) proposed the use of nonlinear least squares or maximum likelihood estimation. The former is less efficient than QML estimation whilst the latter requires specification of the conditional distribution of y and x. The beta distribution is commonly used (Ramalho et al., Citation2010). The various fractional regression models were estimated using package and code provided by Ramalho (Citation2013, Citation2014). The availability of a family of nonlinear functions and the requirement for the exploration of various specifications necessitated the estimation and testing of all five functional forms (Ramalho et al., Citation2010; Papke & Wooldridge, Citation1996; Stanley et al., Citation2013).
3.5. Selection of functional form
Unlike Ogundari (Citation2014), Geffersa et al. (Citation2019), and Solomon and Mamo (Citation2019) who chose a functional form a priori, we follow Djokoto (Citation2015), Djokoto and Gidiglo (Citation2016), and Djokoto et al. (Citation2016) in selecting the appropriate functional form using a battery of tests; RESET test (Ramsey, Citation1969), generalised goodness-of-functional form test (GGOFF) (Ramalho et al., Citation2014) and non-nested P test (Davidson & MacKinnon, Citation1981). The RESET test is also applicable to any type of index models and not to only linear models (Cameron & Trivedi, Citation2013, page 52; Pagan & Vella, Citation1989; Ramalho et al., Citation2010, Citation2011). The RESET test examined the presence of misspecification in the model. Unlike the usual hypothesis tests, the RESET test notes that the model is free of misspecification if the null hypothesis cannot be rejected. The generalised goodness-of-functional form test (GGOFF tests), test for how well the data fits the specified functional form. The non-nested P test provided an opportunity for one-on-one comparison of the functional forms using the selected functional form (s) from the first two stages as alternative hypotheses, since there exists the possibility that more than one functional form could be selected by the RESET and GGOFF tests. The use of the battery of tests (empirical approach) is necessary to arrive at a reliable functional form as there is no theoretical basis for the selection (Ramalho et al., Citation2010, Citation2011; Greene, Citation2004; Ramalho, Citation2014).
4. Results and discussions
4.1. Summary statistics and heterogeneity in MTE
As noted earlier, and observable from Table , our metadata is composed of 223 records (below the mean of 590 of existing MRAs) obtained from 152 publications (above the mean of 79 for previous MRAs). The higher number of publications relative to the metadata observation points to fewer observations per study contributed to the metadata. This was partly influenced by the need to remain within a maximum of five observations per publication recommended by Espey et al. (Citation1997) to avoid the non-independence of observations from the same study that may bias standard errors. The MTE ranged from 0.073 to 0.990 (wider than those of previous MRAs, 0.600–0.863) with an average of 0.676 (lower than that of existing MRAs, 0.732). The standardised spread around the mean is 0.188, higher than that of previous MRAs (0.075). This means the true population MTE would lie within a wider band or confidence interval than for previous studies. This average MTE of 0.676 implies that economic units covered by the primary studies together have lost 32% of output due to inefficiency. Thus, there is an output growth potential of as much as 32% of output. This is achievable without the use of additional inputs. This output gap compares with that of agribusiness in Ghana of 38% (Djokoto & Gidiglo, Citation2016). Clearly, our findings regarding a number of publications, sample size and average MTE departs from previous MRAs markedly. Although the difference between our MRA and others is in the multi-sector coverage of technical efficiency studies on a national scale, the similarity of the measures to that of agribusiness suggests it is the case that economic units in Ghana are less technically efficient. The sources of the inefficiency deserve to be identified for redress.
Table 1. Summary statistics and heterogeneity in MTE estimates
Sample size of primary studies (SSIZE) ranged from a low of 3 (in the case of DEA estimation) to a high of 2507 and averaging at 211. Within the crop category of agriculture, most of the crops studied were non-perennials (NONPERR) (120 observations) with average MTE of 0.672, close to the overall average MTE of 0.676. The least is OTHERCROPS, two observations, referring to samples of studies that combined both perennial and non-perennial crops. Their average MTE of 0.500 is quite removed from the overall average MTE. Within agriculture in general, ANIM recorded the highest average MTE of 0.808. Aquaculture recorded 0.771 higher than fishery (0.532). Within the manufacturing group, MANNEC (general manufacturing) recorded the lowest of 0.310 with a high of 0.665 by TEXTMANU. Average MTE for the services group is generally high with a minimum of 0.623 (PUBADM) and a maximum of 0.869 for EDUCPRIM. It is worth noting that INTERMED (banking, credit union) provided the highest observations (28) to the services group with an average MTE of 0.718.
The NORTH contributed most observations regarding geographical space, 69 more than the sum of COASTL and MID. The 69 observations also represent 31% of the total metadata size of 223. A further examination of the publications shows that authors are either students or lecturers in the University of Development Studies (UDS), Ghana. The average MTE for COASTL, MID and NORTH is respectively 0.677, 0.734 and 0.660. This works to an average of 0.691. Interestingly, this is like the average MTE of studies with national coverage, 0.688. The metadata is dominated by studies from SFA (165) as opposed to that of DEA (58). The former showing a narrower spread (range-0.891, standard deviation-0.176) than the latter (range 0.917, standard deviation-0.220). The resulting average MTE is 0.669 and 0.690, respectively, conforming to the theoretical position that DEA estimates of technical efficiency overstate the technical efficiency estimate (Kumbhakar & Lovell, Citation2000).
The observations in the metadata arose from the use of three data structures; cross-sectional, time series and panel data. Most observations (195, 87.4%) were contributed from cross-sectional data structure. Time series datasets contributed the least (2, 0.9%). Time series and panel data, respectively, recorded higher average MTE, 0.805 and 0.709, than cross-sectional data, 0.670. Unlike cross-sectional data, the other data structures tend to have repeated observations over the objects thereby reinforcing measures; thus, the technical efficiency measures tend to be more accurate (Greene, Citation1993). Sixty-nine percent (154) of the metadata was extracted from journals. The remaining 31% is shared among thesis (17%), conference (7.2%) and working papers (6.7%). A typical publication transition is from thesis/working papers—conference papers—journals. The numbers suggest more papers have attained the final destination than those in transition to the final destination. Regarding their MTEs, these are within the 60% range; 0.615 (working papers) to 0.688 (thesis).
In response to the first hypothesis; Is there heterogeneity in mean technical efficiency in Ghana?, we emphatically state that, yes, there is heterogeneity in the MTE, not only among the various study characteristics but within the categories of the characteristics as well. This further justifies the need to seek factors that explain the variability in the observed MTEs; the last three of our research questions.
4.2. Heteroscedasticity test
The likelihood ratio test for heteroscedasticity is reported in Table . The likelihood ratio of 0.01508 is less than the critical value of 2.706 at 10% level. Since the former is less than the latter, the null hypothesis that there is no heteroscedasticity could not be rejected. Therefore, there is no heteroscedasticity, that is, there may well be homoscedastic error terms. Thus, there is no need to estimate a weighted regression as in the case of Aiello and Bonanno (Citation2016) and Ogundari (Citation2014). As the use of the probit was as an example, we then estimated five functional forms of the unweighted fractional model in order to select the most appropriate one.
Table 2. Likelihood ratio test for heteroscedasticity using probit model
4.3. Selection of functional form
The null hypothesis that the parameters of power terms in the test equation are statistically insignificant could not be rejected. Thus, based on the RESET test, none of the functional forms is misspecified (Table ). Turning to the GGOFF test, the null hypothesis that the data fits the functional forms estimated could not be rejected. From the above, all the functional forms are eligible for selection for discussion. Although the RESET and GGOFF tests do not indicate the superiority of a functional form over another when none of the functional forms is misspecified, we need to select one for discussion. And this choice should not be arbitrary. We elected to rank each of the test statistics for the RESET and GGOFF in order of the relative strength of failure to reject the null hypothesis. The ranks are presented in brackets. The sum of the ranks shows log-log and cauchit functional forms possess the highest ranks. We then compared these two using the one-on-one P test. The test statistic for log-log functional form, as the null hypothesis against cauchit functional form, as the alternative hypothesis has the P statistic of 0.622 whilst for the reverse, the P statistic is 0.177. From these two statistics, the relative strength of failure to reject the null hypothesis is stronger for cauchit functional form, as the null hypothesis than for log-log, as the null hypothesis. Since cauchit functional form is preferred to log-log functional form, the former is selected for discussion. The empirical selection of cauchit functional form in this MRA is a departure from previous MRAs. Logit functional form was empirically selected by Ramalho et al. (Citation2010) and Djokoto (Citation2015) whilst Djokoto and Gidiglo (Citation2016) and Djokoto et al. (Citation2016) empirically selected log-log.
Table 3. Hypothesis tests for selection of functional form of the unweighted fractional regression model
4.4. Discussion of selected model
The first column of the coefficients reports the results of the Egger’s test, EquationEquation 2(2)
(2) . The constant is statistically significant (Table ). This implies the combined effect size of MTE in Ghana from the metadata is 0.703. This is within the confidence interval of the AMTE of 0.676 ±0.032. On the other hand, the AMTE of 0.676 lies within the confidence interval of the effect size of 0.70315 ±0.12800 for the cauchit functional form. The statistically significant coefficient of SSIZE shows that there is publication bias in the literature on technical efficiency in Ghana. In the complete model, however, the parameter of SSIZE is statistically insignificant. This confirms the elimination of the publication bias in the final model. This is attributable to the inclusion of other covariates including studies from thesis, working papers and conference papers (Aiello & Bonanno, Citation2016; Appiah-Adu and Djokoto, Citation2015).
Table 4. Egger’s test and selected unweighted cauchit fractional regression model
The parameters of the crop sub-sector are negatively signed. However, only that of CROPS is statistically significant. The marginal effects of CROPS appear to be a crystallisation of the lower MTEs than the reference. This statistically significant negative sign of CROPS parameter implies that studies that combined perennial and non-perennial crops reported lower MTE than those of mixed farming. This, together with the negative signs of the parameters of all the crop sub-sectors points to a generally high output gap in the crop sub-sector. It does appear that not only that polices pursued towards improving the efficiency of crop production have not yielded the desired results vis-à-vis technical efficiency, it also means that urgent actions are required to fill the output gap. This is important because the NONPERR includes rice (contributing 34 observations to the metadata of 120 non-perennials), maize, vegetables, legumes that are used to produce staple food in Ghana. Further, to reduce the rice import bill of 287 USD m (“FAOSTAT,” Citation2016) annually, increased technical efficiency in rice production cannot be overlooked. The positive and statistically insignificant marginal effect of ANIM shows that animal production is indifferent from mixed farming regarding technical efficiency. Animal production does appear to be more efficient than crop production in general. Whilst this finding agrees with Djokoto (Citation2015) regarding organic crop production globally, the reverse is the case for agriculture in Ghana (Djokoto et al., Citation2016). Djokoto and Gidiglo (Citation2016), Hina and Bushra (Citation2016), and Ogundari (Citation2014) found no difference between perennial crop and other crop production.
The negative and statistically significant marginal effect of FISH suggests that the MTE of fisheries is lower than that of the reference, aquaculture. This is unsurprising as aquaculture is managed within a specified space; provision of feed, aeration of the water and general husbandry. In the case of fishing, however, the nourishing of the fish is left to mother nature, within a virtually limitless ocean and marine water. The expanse of the water places constraints on what quantities are captured. Also, overexploitation of stocks, environmental degradation, and use of unapproved fishing gears and methods and low water levels in the case of inland waters have negatively impacted fish production. Fish production has been on the decline since 2010, at the rate of 910 t per year, that is, 1.1% of the average annual inland capture fishery production. Further, for the past 20 years, catches from the reservoirs in northern Ghana have been dwindling (Abban et al., Citation2002; Abobi et al., Citation2019; Amevenku & Quarcoopome, Citation2006; Ghana Business News, Citation2019). Enforcement of close seasons, use of appropriate-sized nets, avoidance of the pair-trawling and use of lights and dynamites would help in the regeneration of fish stock and thereby increase catches which should lead to increased output given resources deployed for fishing.
Within the division of manufacturing, only the marginal effect of pharmaceutical manufacturing is statistically distinguishable from zero. The negative sign implies that MTE of pharmaceuticals is lower than that of the reference, unclassified manufacturing. Given the importance of health to national development and the import bill of pharmaceuticals, the need to improve efficiency in pharmaceutical production cannot be overstated.
Within the services sector, only the parameters of education variables are statistically distinguished from zero. Together with the positive signs, the results imply primary education (EDUCPRIM) and support services such as library services (EDUCSUP) are higher in technical efficiency than other education. Clearly, the MTE of library services is higher than that of the reference, other education (sports and recreation education, cultural education etc.). Whilst the technical efficiency of primary education and library services needs to be sustained, efforts must be made to pursue policies and programmes to raise the efficiency of other education.
The statistically insignificant marginal effect of HOSP implies technical efficiency of hospitals and the reference, health centres, do not differ significantly. Hospitals tend to offer a wider range of medical services and are more resourced (financial, human and material) than health centres. It appears however that these wider service offerings have not contributed to increased efficiency.
Two out of the three spatial variables have statistically significant positive marginal effects. This implies the reference, MTEs covering Ghana (and COASTL), is lower than those covering areas defined as MID (middle) and north sections of the country. This is rather surprising as the generally better development and urban environs in the coastal regions have not led to higher efficiency in productive activities. This situation deserves further study to unearth the specific causes for redress. This finding is contrary to the earlier conclusions of Djokoto et al. (Citation2016) and Djokoto and Gidiglo (Citation2016) for agriculture and agribusiness in Ghana.
Turning to the temporal perspective, the marginal effect of DYEAR is negative and statistically indistinguishable from zero. Although the size of the parameter may be statistically insignificant, the negative sign suggests that MTE may be declining over time. This is unexpected as with time improved technology, know-how and experience should lead to increased output relative to the potential. This technical efficiency regression requires urgent steps by managers of economic activities in the industries studied, to improve technology and know-how and managerial prowess in order to reverse technical efficiency regression. It is worth noting that, whilst the inverse relation agrees with those of Iliyasu et al. (Citation2014), Odeck and Bråthen (Citation2012), Ogundari (Citation2014), and Solomon and Mamo (Citation2019), it is at variance with those of Aiello and Bonanno (Citation2016), Djokoto and Gidiglo (Citation2016), Fall et al. (Citation2018), and Ogundari and Brummer (Citation2011).
The statistical insignificance of the marginal effect of DEA shows that the MTE of DEA and SFA do not differ significantly. Although DEA is known to overstate MTE (Bravo-Ureta et al., Citation2007; Iliyasu et al., Citation2014; Irsova & Havranek, Citation2010; Kumbhakar & Lovell, Citation2000; Odeck & Bråthen, Citation2012), the finding of statistically insignificant marginal effect does not confirm this. The improvements in DEA estimation over the years may have accounted for this (Cook & Seiford, Citation2009; Kao, Citation2014; Koronakos, Citation2019; Mariz et al., Citation2018). This finding, however, concurs with Djokoto and Gidiglo (Citation2016), Djokoto et al. (Citation2016), Fall et al. (Citation2018), Kiadaliri et al. (Citation2013), Mareth et al. (Citation2016), Papadimitriou (Citation2013), and Thiam et al. (Citation2001).
The statistically significant and positive marginal effects of TSERIES imply that this exceeds panel data in MTE. Since MTE from cross-section data (XSECTION) is indifferent from those of panel data, it may well be the case that MTE from time-series data studies exceeds those of both panel and cross-section data. That of cross-sectional data is reinforced by the negative sign of the parameter. Indeed, from the univariate analysis in Table , AMTE of time series data exceeded those of cross-sectional and panel data by at least 0.1. About 87% of the metadata arose from cross-sectional data analysis whilst about 12% was contributed by panel data studies, suggesting a tendency of not using time series data in efficiency estimation in Ghana. That notwithstanding, it must be borne in mind that time-series data could overestimate MTE. This finding is in line with those of Hina and Bushra (Citation2016) and Moreira López and Bravo-Ureta (Citation2009). Other MRAs had however reported higher MTEs from cross-sectional analysis than for panel data analysis (Aiello & Bonanno, Citation2016; Bravo-Ureta et al., Citation2007; Brons et al., Citation2005; Fall et al., Citation2018; Nguyen & Coelli, Citation2009; Thiam et al., Citation2001). Whilst the findings suggest the level of MTE obtained may be influenced by choice of data structure, other factors such as cost and availability among others should be weighed together with MTE in order arrive at the most appropriate choice of data structure.
The marginal effects of all three variables of dissemination of research works are statistically indistinguishable from zero. This also implies MTE found in studies disseminated in journals, conference papers and working papers are indifferent from those of the control, thesis. The results could mean that irrespective of the stage of the dissemination media, the MTE generated do not differ. The result could also mean that the review and assessment process of manuscripts and publications on technical efficiency on Ghana do not result in changes in the measures or estimates of technical efficiency. For students, theses are often the starting point of research publications. For non-student academics, working papers and conference papers are the starting point. In all cases, journals are mostly the terminal media for the dissemination of research output. Whilst Djokoto (Citation2015) and Solomon and Mamo (Citation2019) agree with this finding, others disagree (Aiello & Bonanno, Citation2016; Alatawi et al., Citation2019; Djokoto & Gidiglo, Citation2016; Djokoto et al., Citation2016; Geffersa et al., Citation2019; Ogundari, Citation2014). The finding does not in any way discount the need to finally publish research reports as journal articles.
5. Concluding remarks
This MRA set out to explore the heterogeneity in MTE and study characteristics that explain the observed heterogeneity. We departed from all previous MRAs in studying a whole economy, Ghana. We found heterogeneity in MTE within and across all industries studied. We identified the industries at the 3-digit level using the UN SIC Rev, 4 of 2008. At least each industry division significantly explained the variability in MTE. There was spatial variability in MTE as well. There was no temporal differentiation in MTE. MTEs in journals, working papers, conference papers and theses could not be distinguished from one another. MTE was differentiated courtesy time series data. Generally, there is a need to increase MTE across industries and space.
Since this is the first comprehensive metadata on efficiency at the national level, when updated, could be applied to other forms of review as well as citation analyses. The data could form a basis for regional and global metadata on technical efficiency that could assist in unearthing important information in the discipline of efficiency and productivity.
Supplemental Material
Download MS Word (86 KB)Disclosure statement
We have no conflict of interest.
Supplementary material
Supplemental data for this article can be accessed here.
Additional information
Funding
Notes on contributors
Justice G. Djokoto
Ghana has witnessed many studies on efficiency and productivity across many economic activities, by diverse authors, from different institutional affiliations. The collaborations have also spanned countries and continents. Acursory examination of some of the papers showed varied data structures and methods of analysis since 2001. It is thus appropriate to review these studies. That will establish the gaps to provide new directions among others. Consequently, since 2019, the team has been working on the National Efficiency and Productivity Project. The current paper is the second. The first was a bibliometric review. A third is a citation analysis that examined the drivers of citations of efficiency and productivity studies in Ghana.
Team members:
Justice G. Djokoto, Ferguson K. Gidiglo, Francis Y. Srofenyoh, Kofi Aaron a-O. Agyei-Henaku, Akua a. Afrane Arthur and Charlotte Badu-Prah, all of Agribusiness Management Department, Central University, Accra, Ghana.
Notes
1. Fried et al. (Citation2008) have provided an overview of further methodological developments on these.
References
- Abban, E. K., Kwarfo-Apegyah, K., & Amedorme, K. 2002. Fish monitoring in relation to onchocerciasis control programme in Ghana. CSIR–Water Research Institute. Annual report.
- Abobi, S. M., Mildenberger, T. K., Kolding, J., & Wolff, M. (2019). Assessing the exploitation status of main fisheries resources in Ghana’s reservoirs based on reconstructed catches and a length-based bootstrapping stock assessment method. Lake and Reservoir Management, 35(4), 415-434.
- Aiello, F., & Bonanno, G. (2016). Efficiency in banking: A meta-regression analysis. International Review of Applied Economics, 30(1), 112–19. https://doi.org/10.1080/02692171.2015.1070131
- Aigner, D., Lovell, C. A. K., & Schmidt, P. (1977). Formulation and estimation of stochastic production function models. Journal of Econometrics, 6(1), 21–37. https://doi.org/10.1016/0304-4076(77)90052-5
- Alatawi, A., Ahmed, S., Niessen, L., & Khan, J. (2019). Systematic review and meta-analysis of public hospital efficiency studies in Gulf region and selected countries in similar settings. Cost Effectiveness and Resource Allocation, 17(1), 1–12. https://doi.org/10.1186/s12962-019-0185-4
- Alvarez, R. M., & Brehm, J. (1997). Are Americans ambivalent towards racial policies. American Journal of Political Science, 41(2), 345–374. https://doi.org/10.2307/2111768
- Amevenku, F., & Quarcoopome, T. (2006). Fish and fisheries of Bontanga and Libga reservoirs in northern Ghana, West Africa. West African Journal of Applied Ecology, 10, 9–19.
- Appiah-Adu, K., & Djokoto, J. G. (2015). Publication bias and the market orientation-performance nexus literature. African Journal of Management Research, 23(1), 73–96. https://www.ajol.info/index.php/ajmr/article/viewFile/122975/112515
- Banker, R. D., Charnes, A., & Cooper, W. W. (1984). Some models for estimating technical and scale inefficiencies in data envelopment analysis. Management Science, 30(9), 1078–1092. https://doi.org/10.1287/mnsc.30.9.1078
- Bravo-Ureta, B. E., Solís, D., López, V. H. M., Maripani, J. F., Thiam, A., & Rivas, T. (2007). Technical efficiency in farming: A meta-regression analysis. Journal of Productivity Analysis, 27(1), 57–72. https://doi.org/10.1007/s11123-006-0025-3
- Brons, M., Nijkamp, P., Pels, E., & Rietveld, P. (2005). Efficiency of urban public transit: A meta-analysis. Transportation, 32(1), 1–21. https://doi.org/10.1007/s11116-004-0939-4
- Cameron, A. C., & Trivedi, P. K. (2013). Regression analysis of count data (Econometric society monographs). Cambridge University Press.
- Chaimani, A., Mavridis, D., & Salanti, G. (2014). A hands-on practical tutorial on performing meta-analysis with Stata. Evidence Based Mental Health, 17(4), 111–116. https://doi.org/10.1136/eb-2014-101967
- Charnes, A., Cooper, W. W., & Rhodes, E. (1978). Measuring the efficiency of decision-making units. European Journal of Operational Research, 2(6), 429–444. https://doi.org/10.1016/0377-2217(78)90138-8
- Cook, W. D., & Seiford, L. M. (2009). Data envelopment analysis (DEA) - thirty years on. European Journal of Operational Research, 192(1), 1–17. https://doi.org/10.1016/j.ejor.2008.01.032
- Davidson, R., & MacKinnon, J. (1984). Convenient specification tests for logit and probit models. Journal of Econometrics, 25(3), 241–262. https://doi.org/10.1016/0304-4076(84)90001-0
- Davidson, R., & MacKinnon, J. G. (1981). Several tests for model specification in the presence of alternative hypotheses. Econometrica: Journal of the Econometric Society, 49(3), 781–793. https://doi.org/10.2307/1911522
- Djokoto, J. G. (2015). Technical efficiency of organic agriculture: A quantitative review. Studies in Agricultural Economics, 117(2), 67–71. https://doi.org/10.7896/j.1512
- Djokoto, J. G., & Gidiglo, K. F. (2016). Technical efficiency in agribusiness: A meta‐analysis on Ghana. Agribusiness, 32(3), 397–415. https://doi.org/10.1002/agr.21457
- Djokoto, J. G., Srofenyo, F. Y., & Afrane-Arthur, A. A. (2016). Technical inefficiency effects in agriculture—a meta-regression. Journal of Agricultural Science, 8(2), 109–121. https://doi.org/10.5539/jas.v8n2p109
- Egger, M., Davey, S. G., Schneider, M., & Minder, C. (1997). Bias in meta-analysis detected by a simple, graphical test. British Medical Journal, 315(7109), 629–634. https://doi.org/10.1136/bmj.315.7109.629
- Emrouznejad, A., Parker, B. R., & Tavares, G. (2008). Evaluation of research in efficiency and productivity: A survey and analysis of the first 30 years of scholarly literature in DEA. Socio-economic Planning Sciences, 42(3), 151–157. https://doi.org/10.1016/j.seps.2007.07.002
- Engle, R. F. (1984). Wald, likelihood ratio and Lagrange multiplier tests in econometrics. In Z. Griliches & M. D. Intriligator (Eds.), Handbook of econometrics (Vol. 11, pp. 775-826). North-Holland.
- Espey, M., Espey, J., & Shaw, W. D. (1997). Price elasticity of residential demand for water: A meta-analysis. Water Resources Research, 33(6), 1369–1374. https://doi.org/10.1029/97WR00571
- Fall, F., Akim, A. M., & Wassongma, H. (2018). DEA and SFA research on the efficiency of microfinance institutions: A meta-analysis. World Development, 107, 176–188. https://doi.org/10.1016/j.worlddev.2018.02.032
- FAOSTAT (Food and Agriculture Organisation). (2016). http://www.fao.org/faostat/en/#data/TP
- Fare, R., Grosskopf, S., Lovell, C. A. K., & Fare, R. (1983). The structure of technical efficiency. The Scandinavian Journal of Economics, 85(2), 181–190. https://doi.org/10.2307/3439477
- Farrell, M. J. (1957). The measurement of productive efficiency. Journal of the Royal Statistical Society. Series a (General), 120(3), 253–290. https://doi.org/10.2307/2343100
- Freeman, E., Keele, L., Park, D., Salzman, J., & Weickert, B. (2015). The plateau problem in the heteroskedastic probit model. arXiv Preprint arXiv, 1508.03262v1. https://arxiv.org/pdf/1508.03262.pdf
- Fried, H. O., Lovell, C. A. K., & Schmidt, S. S. (2008). Efficiency and productivity. In H. Fried, C. A. K. Lovell, & S. Schmidt (Eds.), The measurement OF productive efficiency and productivity change (pp. 3–91). Oxford University Press.
- Geffersa, A. G., Agbola, F. W., & Mahmood, A. (2019). Technical efficiency in crop production across agro-ecological zones in Ethiopia: A meta-analysis of frontier studies’. Outlook on Agriculture, 48(1), 5–15. https://doi.org/10.1177/0030727019830416
- Ghana Business News. (2019), Foreign vessels are the problem, not us – Elmina fisher folks. Retrieved August 19, 2019, from https://www.ghanabusinessnews.com/2019/08/19/foreign-vessels-are-the-problem-not-us-elmina-fisher-folks/Creative Imaginations Publicity
- Glass, G. V. (1976). Primary, secondary, and meta-analysis of research. Educational Researcher, 5(10), 3–8. https://doi.org/10.3102/0013189X005010003
- Glass, G. V., McGaw, B., & Smith, M. L. (1981). Meta-analysis in social research. Sage Publications.
- Gokcekus, O., Anyane-Ntow, K., & Richmond, T. R. (2001). Human capital and efficiency: The role of education and experience in micro-enterprises of Ghana’s wood-products industry. Journal of Economic Development, 26(1), 103–114. http://www.jed.or.kr/full-text/26-1/gokcekus.PDF
- Greene, W. H. (1993). The econometric approach to efficiency analysis. In H. O. Fried, C. A. K. Lovell, & S. S. Schmidt (Eds.), The measurement of productive efficiency: Techniques and applications (pp. 68–119). Oxford University Press.
- Greene, W. H. (2004). Econometric analysis. Prentice Hall.
- Harvey, A. (1976). Estimating regression models with multiplicative heteroscedasticity. Econometrica, 44(3), 461–465. https://doi.org/10.2307/1913974
- Hedges, L. V., & Olkin, I. (1985). Statistical methods for meta-analysis. Academic Press.
- Hess, S., & Von Cramon-Taubadel, S. (2008). a meta-analysis of general and partial equilibrium simulations of trade liberalisation under the Doha development agenda. The World Economy, 31(6), 804–840. https://doi.org/10.1111/j.1467-9701.2008.01103.x
- Hina, F., & Bushra, Y. (2016). Efficiency and productivity analysis of Pakistan’s farm sector: A meta-analysis. Pakistan Journal of Agricultural Research, 29(3), 312–322. https://drive.google.com/file/d/0B6lqTE3dYfqTdkwtU1FxeklZVVE/view
- Iliyasu, A., Mohamed, Z. A., Ismail, M. M., & Abdullah, A. M. (2014). A meta-analysis of technical efficiency in aquaculture. Journal of Applied Aquaculture, 26(4), 329–339. https://doi.org/10.1080/10454438.2014.959829
- Irsova, Z., & Havranek, T. (2010). Measuring bank efficiency: A meta-regression analysis. Prague Economic Papers, 19(4), 307–328. https://doi.org/10.18267/j.pep.379
- Kao, C. (2014). Network data envelopment analysis: A review. European Journal of Operational Research, 239(1), 1–16. https://doi.org/10.1016/j.ejor.2014.02.039
- Kiadaliri, A. A., Jafari, M., & Gerdtham, U.-G. (2013). Frontier-based techniques in measuring hospital efficiency in Iran: A systematic review and meta-regression analysis. BMC Health Services Research, 13(1), 312. https://doi.org/10.1186/1472-6963-13-312
- Koronakos, G. (2019). a taxonomy and review of the network data envelopment analysis literature. In G. Tsihrintzis, M. Virvou, E. Sakkopoulos, & L. Jain (Eds.), Machine learning paradigms (Vol. 1, pp. 255–311). Springer.
- Kumbhakar, S. C., & Lovell, C. (2000). Stochastic frontier analysis. Cambridge University Press.
- Mareth, T., Thomé, A. M. T., Cyrino Oliveira, F. L., & Scavarda, L. F. (2016). Systematic review and meta-regression analysis of technical efficiency in dairy farms. International Journal of Productivity and Performance Management, 65(3), 279–301. https://doi.org/10.1108/IJPPM-02-2015-0027
- Mariz, F. B., Almeida, M. R., & Aloise, D. (2018). A review of dynamic data envelopment analysis: State of the art and applications. International Transactions in Operational Research, 25(2), 469–505. https://doi.org/10.1111/itor.12468
- McDonald, J. (2009). Using least squares and tobit in second stage DEA efficiency analyses. European Journal of Operational Research, 197(2), 792–798. https://doi.org/10.1016/j.ejor.2008.07.039
- Meeusen, W., & Van Den Broeck, J. (1977). Efficiency estimation from Cobb-Douglas production functions with composed error. International Economic Review, 18(2), 435–444. https://doi.org/10.2307/2525757
- Moreira López, V. H., & Bravo-Ureta, B. E. (2009). A study of dairy farm technical efficiency using meta-regression: An international perspective. Chilean Journal of Agricultural Research, 69(2), 214–223. https://doi.org/10.4067/S0718-58392009000200011
- Nandy, A., Singh, P. K., & Singh, a. K. (2019). Systematic review and meta-regression analysis of technical efficiency of agricultural production systems. Global Business Review, 1-26 0972150918811719.journals.sagepub.com/home/gbr
- Nelson, J. P., & Kennedy, P. E. (2009). The use (and abuse) of meta-analysis in environmental and natural resource economics: An assessment. Environmental and Resource Economics, 42(3), 345–377. https://doi.org/10.1007/s10640-008-9253-5
- Nguyen, K. H., & Coelli, T. (2009). Quantifying the effects of modelling choices on hospital efficiency measures: A meta-regression analysis, (No. WP072009). School of Economics, University of Queensland.
- Odeck, J., & Bråthen, S. (2012). A meta-analysis of DEA and SFA studies of the technical efficiency of seaports: A comparison of fixed and random-effects regression models. Transportation Research Part A: Policy and Practice, 46(10), 1574–1585. https://doi.org/10.1016/j.tra.2012.08.006
- Ogundar, K., & Brummer, B. (2011). Technical efficiency of Nigerian agriculture: A meta-regression analysis. Outlook on Agriculture, 40(2), 171–180. https://doi.org/10.5367/oa.2011.0038
- Ogundari, K. (2014). The paradigm of agricultural efficiency and its implication on food security in Africa: What does meta-analysis reveal? World Development, 64, 690–702. https://doi.org/10.1016/j.worlddev.2014.07.005
- Ogundari, K., Amos, T. T., & Okoruwa, V. O. (2012). A review of Nigerian agricultural efficiency literature, 1999–2011: What does one learn from frontier studies? African Development Review, 24(1), 93–106. https://doi.org/10.1111/j.1467-8268.2011.00307.x
- Pagan, A., & Vella, F. (1989). Diagnostic tests for models based on individual data: A survey. Journal of Applied Econometrics, 4(S1), 29–59. https://doi.org/10.1002/jae.3950040504
- Papadimitriou, M. (2013). Technical efficiency in higher education. A meta-regression analysis (Master’s dissertation, University of Macedonia, Thessaloniki, Greece). University of Macedonia. http://www.semanticscholar.org/paper.Technical-efficiency-in-higher-education.a meta-regression analysis
- Papke, L. E., & Wooldridge, J. M. (1996). Econometric methods for fractional response variables with an application to 401 (k) plan participation rates. Journal of Applied Econometrics, 11(6), 619–632. https://doi.org/10.1002/(SICI)1099-1255(199611)11:6<619::AID-JAE418>3.0.CO;2-1
- Poot, J. (2012). Learning from the flood of findings: Meta-analysis in economics (pdf). National Institute of Demographic and Economic Analysis. The University of Waikato. https://www.nzae.org.nz/wp-content/uploads/2012/09/NZAE-2012-Poot-meta-analysis.pdf
- Ramalho, E. A., Ramalho, J. J., & Murteira, J. M. (2011). Alternative estimating and testing empirical strategies for fractional regression models. Journal of Economic Surveys, 25(1), 19–68. https://doi.org/10.1111/j.1467-6419.2009.00602.x
- Ramalho, E. A., Ramalho, J. J., & Murteira, J. M. (2014). A generalised goodness‐of‐functional form test for binary and fractional regression models. The Manchester School, 82(4), 488–507. https://doi.org/10.1111/manc.12032
- Ramalho, E. A., Ramalho, J. J. S., & Henriques, P. D. (2010). Fractional regression models for second stage DEA efficiency analyses. Journal of Productivity Analysis, 34(3), 239–255. https://doi.org/10.1007/s11123-010-0184-0
- Ramalho, J. J. S. (2013). FRM: Stata module to estimate and test fractional regression models, statistical software components 457542. Boston College Department of Economics.
- Ramalho, J. J. S. (2014). FRM: Regression analysis of fractional responses, R package. Comprehensive R Archive Network.
- Ramsey, J. B. (1969). Tests for specification errors in classical linear least-squares regression analysis. Journal of the Royal Statistical Society. Series B Methodological, 31(2), 350–371. https://www.jstor.org/stable/2984219
- Rose, a. K., & Stanley, T. D. (2005). A meta‐analysis of the effect of common currencies on international trade. Journal of Economic Surveys, 19(3), 347–365. https://doi.org/10.1111/j.0950-0804.2005.00251.x
- Rosenthal, R. (1984). Meta-analytic procedures for social research. Sage Publications.
- Solomon, T., & Mamo, T. (2019). A synthesis of Ethiopian agricultural technical efficiency: A meta-analysis. African Journal of Agricultural Research, 14(9), 559–570. https://doi.org/10.5897/AJAR2017.12729
- Stanley, T. D. (2005). Beyond publication bias. Journal of Economic Surveys, 19(3), 309–345. https://doi.org/10.1111/j.0950-0804.2005.00250.x
- Stanley, T. D. (2008). Meta-regression methods for detecting and estimating empirical effect in the presence of publication selection. Oxford Bulletin of Economics and Statistics, 70(1), 103–127. https://doi.org/10.1111/j.1468-0084.2007.00487.x
- Stanley, T. D., & Doucouliagos, H. (2012). Meta-regression analysis in economics and business. Routledge.
- Stanley, T. D., Doucouliagos, H., Giles, M., Heckmeyer, J., Johnston, R., Laroche, P., Nelson, J. P., Paldam, M., Poot, J., Pugh, G., Rosenberger, R. S., & Rost, K. (2013). Meta-analysis of economics research reporting guidelines. Journal of Economic Surveys, 27(2), 390–394. https://doi.org/10.1111/joes.12008
- Stanley, T. D., & Jarrell, S. B. (1989). Meta‐regression analysis: A quantitative method of literature surveys. Journal of Economic Surveys, 3(2), 161–170. https://doi.org/10.1111/j.1467-6419.1989.tb00064.x
- Sterne, J. C. (2009). Meta-analysis in stata: An updated collection from the stata journal, Jonathan Sterne 9781597180498. CRC PRESS.
- Thiam, A., Bravo‐Ureta, B. E., & Rivas, T. E. (2001). Technical efficiency in developing country agriculture: A meta‐analysis. Agricultural Economics, 25(2‐3), 235–243. https://doi.org/10.1111/j.1574-0862.2001.tb00204.x
- United Nations. (2008). International standard industrial classification of all economic activities revision 4. Statistical Papers, Series M No. 4/Rev.4