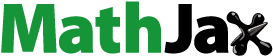
Abstract
The upsurge in social media websites has in no doubt triggered a huge source of data for mining interesting expressions on a variety of subjects. These expressions on social media websites empower firms and individuals to discover varied interpretations regarding the opinions expressed. In Sub-Saharan Africa, financial institutions are making the needed technological investments required to remain competitive in today’s challenging global business environment. Twitter as one of the digital communication tools has in recent times been integrated into the marketing communication tools of banks to augment the free flow of information. In this light, the purpose of the present study is to perform a sentiment analysis on a large dataset of tweets associated with the Ecobank Group, a prominent pan-African bank in sub-Saharan Africa using four different sentiment lexicons to determine the best lexicon based on its performance. Our results show that Valence Aware Dictionary and sEntiment Reasoner (VADER) outperforms all the other three lexicons based on accuracy and computational efficiency. Additionally, we generated a word cloud to visually examine the terms in the positive and negative sentiment categories based on VADER. Our approach demonstrates that in today’s world of empowered customers, firms need to focus on customer engagement to enhance customer experience via social media channels (e.g., Twitter) since the meaning of competitive advantage has shifted from purely competing over price and product to building loyalty and trust. In theory, the study contributes to broadening the scope of online banking given the interplay of consumer sentiments via the social media channel. Limitations and future research directions are discussed at the end of the paper.
Public Interest Statement
In financial economics, information seeking is very useful for day-to-day activities with regards to pre and post-financial transactions, particularly, among banking institutions and other financial outlets in general. Hence, social media channels continue to be the major driving force for the exchange of information amongst individuals, corporate organizations, and even non-business institutions. Undoubtedly, e-businesses are making the needed investment to harness the potentials of integrating social medial communication tools for sustainable business activity. In Sub-Saharan Africa, financial institutions are making the needed technological investments required to remain competitive in today’s challenging global business environment. Twitter as one of the digital communication tools has in recent times been integrated into the marketing communication tools of banks to augment the free flow of information. This study therefore investigates and makes deductions from the opinions expressed by customers of a sub-Saharan African bank on Twitter, precisely the Ecobank group. The authors henceforth, remind readers that the study implication for banks and other stakeholders are discussed in the paper.
1. Introduction
In financial economics, information seeking is very useful for day-to-day activities with regards to pre and post-financial transactions particularly among banking institutions and other financial outlets in general. At the same time, this acquired information from the advent of innovation (FinTech) helps particularly these banks to effectively and efficiently distribute resources in markets in which decisions are made under uncertainty. Owing to this, the emergence of electronic business is one of the most profound changes that has revolutionized the process of buying, selling, and exchanging products and services over the Internet (Kennedy, Citation2012; Wright et al., Citation2019). In light of this, online banking transactions have grown at an exponential rate due to the high intensity of competition among banks and non-banking institutions. This phenomenon in today’s technological era has attracted a growing interest by scholars and industry players concerning the effectiveness and efficiency characterized by service delivery in both production and service-based organizations (Botchway et al., Citation2019; Ernst & Young, Citation2011; Mogaji et al., Citation2016). More so, internet banking has attracted several distinguished stakeholders including bankers, customers, regulators amongst others to adopt it in their day-to-day activities.
Interestingly, the presence of digital society has become a crucial driver for business organizations to thrive (Jibril, Kwarteng, Chovancova, Pilik et al., Citation2019; Tafesse & Wien, Citation2017, Citation2018). Data mining in the virtual space complement with its accessible devices has triggered some level of competition among business operators, thus deepening the understanding of a perfectly competitive market as a result of breaching the information gap in the business society. However, the development of marketing communication channel, particularly, social media channels (like Facebook, Instagram, Twitter, LinkedIn among others) has made it possible to view, listen and to access volume of unstructured data such as customer suggestions, customer complaints and customer feedback (Raicu & Turkes, Citation2016; Sailunaz & Alhajj, Citation2019). Other prominent business areas that have witnessed heavy use of social media applications include brand awareness creation (Eisenmann, Citation2006), identification of new product ideas and niche markets (Van Sinderen & Almeida, Citation2011) and establishing contacts with employees, business partners and competitors (Ahmad et al., Citation2006; Gillin & Schwartzman, Citation2010). Table lists the benefits of this new collaborative web (Web 2.0) under three distinct groups as shown in the work of (Bughin et al., Citation2009) using the McKinsey Quarterly survey. It is worthy to note from Table that 68%, 43%, and 22% of respondents stated that using social media tools in Web 2.0 resulted in effective marketing increase internally, an increase in customer satisfaction and an increase in the number of successful innovations respectively. Again, 54% of internal respondents as well as 49% external respondents experienced a reduction in communication cost due to social media usage.
Table 1. Business benefits from Web 2.0
Additionally, the texts from these social media channels, the positive, negative, and neutral expressions noticed are somewhat described as individual perceived strengths and weaknesses of the said product/service respectively. While the usually assigned product/service in question ratings on these platforms represent the overall valuation ranging from product/service type to product/service satisfaction (Bott, Citation2014). Nonetheless, this phenomenon has drawn attention to contemporary researchers and other industry players within the spheres of online-related activities like banks, telecoms, and other service-related organizations.
However, despite the availability of other forms of business/marketing communication, the text remains one of the most common ways of communication in a social network today. Having this in mind, the rationale behind this work described in this paper is to detect and analyse sentiment and emotion expressed by people from the text in their twitter posts and use them for generating recommendations. In order words, the important research gab/focus at this point is how to deduce or convert this chunk of customers’ opinions (sentiments) from a particular social media channel (say, Twitter) of a Sub-Saharan African bank (Ecobank Group) into a collective managerial decision-making process. Similarly, how these opinions are meaningful and useful with regards to a product or service improvement as well as knowledge management in the medium to long-term sustainability. More so, considering the rapid increase in the quantity of customer data has encouraged the necessity and interest in analysing these texts for managerial decision making regarding product/service performance and redevelopment (Cambria et al., Citation2013; Sailunaz & Alhajj, Citation2019). Again, the growing use of these Apps (social media channels) by several individuals and firms have created a relationship between them. For example, a bank, typically a commercial organization connected to these platforms aims at drawing closer to its customers for easier exchange or dissemination of information across each other. Also, the operator bank of this App has the opportunity to market its products on offer to potential and existing customers. This interaction over the internet is deemed important since the marketing trends in recent times consider the customer as a core element of product design and development.
Against this backdrop, the objective of the study is to track and deduce customers’ opinions with regards to the Ecobank Group’s products and services with the aid of sentiment analytic approach Africa using four different sentiment lexicons. To determine the best lexicon based on its performance, the lexicons used are the Valence Aware Dictionary and sEntiment Reasoner (VADER), AFINN, SentiWordNet, and TextBlob. Meanwhile, it is worth noting that online social networks are in no doubt emerged as a contemporary social platform that provides a ground for people to share their views and perspectives on a variety of issues and subjects with their acquaintances, family, relatives, among others. In this vein, individuals can share their thoughts, mental state, moments, stand on specific social, national, international issues through text, photos, audio, and video messages and posts.
To ensure the growth and survival of financial firms in this technological era regarding competitive advantage, sentiments associated with textual data of customers must be explored and analysed. This will assist in deducing some contextual meaning of consumer’s opinions, frustrations, and satisfaction which consequently culminates to have a positive impact on the business(bank). Overall, the study adds up to broaden the scope of internet marketing (banking) given the interplay of consumer sentiments via social media channels. Similarly, consumers can use sentiment analysis to search for a product or service before making a purchase. On the other hand, marketers/bankers can use this to research public opinion of their company and products, in terms of customer satisfaction and vice-versa. More so, Organizations can also use this to gather critical feedback about problems in newly released products. With respect to financial economics, for that matter, monetary activities, this study further deepens the existing literature by offering some empirical analysis from the developing world (Africa) in terms of the application of FinTech within the mainstream of the banking sector. However, this study is without limitation. First, it considers only a single platform (twitter app) of the aforementioned bank without recourse to other equally important social medial channels. Last but not the least, the sentiment lexicons (algorithm) used may not necessarily be the best/robust procedures to ascertain the overall objective of the study since other approaches such as the Fuzzy rule-based method and topic modeling could also be used in the current study.
The remainder of this paper is organized as follows; Section two shows the background of financial technology, sentiment analysis, and overview of Ecobank Ghana’s limited bank and the social media presence. Section three explains the research methodology implored to mine customer’s sentiments followed by the results and discussion in section four. Finally, section 5 presents conclusions and future work.
2. Literature review
2.1. Financial technology and customer expectation
The concept of financial technology (FinTech) is a contemporary means of finance by providing an innovative product that would augment the existing practices and facilitates consumer’s experiences in financial transactions effectively and efficiently (Abbasi & Weigand, Citation2017). Financial technology, often shortened to FinTech, is the technology and innovation that aims to compete with traditional financial methods in the delivery of financial services. Arguably, it is an emerging industry that uses technology to improve activities in finance. FinTech is utilized to help companies, business owners, and consumers better manage their financial operations, processes, and lives by utilizing specialized software and algorithms that are used on computers and, increasingly, smartphones.
This development (FinTech) among other things, assists several stakeholders in the financial industry. For instance, small-business owners feel the support of FinTech by providing them with relevance services from financial service providers, such as seed capital, loans, financial advice, digital financial solutions, online services, and many more(Farina et al., Citation2014). It is quite interesting to note that Fintech refers to a distinct area of financial innovation where mostly the center of interest is a transformative technology. A typical example of FinTech is a P2P lending platform called “Zopa”, which gives people access to loans directly from connected devices, and many other services deemed beneficial to the user (Davidov et al., Citation2010).
Customer expectation, on the other hand, refers to the perceived value or benefits that a customer seeks when purchasing a good or service. In other words, it consists of the feelings, needs, and ideas that customers have towards certain products or services. Similarly, customers’ experience is based on what they want from the products or services they are paying for. Studies have shown that most successful organizations always aim at meeting or exceeding customer expectations through high-quality products and services (Das et al., Citation2019; Kang & Park, Citation2014). These expectations influence decision-making towards a product or service selection from medium-to-long-term plans. For example, customers expect famous brands to be of high quality than new and generic brands. Therefore, for banks to meet such expectations from their customers, it is important to engage them in an interactive way using a social media channel (online communication tool).
Considering this, customer satisfaction in business terms is regarded as one of the key performance indicators within businesses. In today’s competitive marketplace where businesses compete for customers, the management of customer satisfaction is very essential in all spheres. Hence, one of the important sources of customer feedback is product reviews. Therefore, sentiment analysis of customer reviews has been a very interesting topic in the two last decades. Though early works were mainly focused on identifying the positivity and negativity of reviews (Moghaddam, Citation2015; Raicu & Turkes, Citation2016), later research tries to extract more detailed information by estimating the sentiment score of each product aspect/feature. In this work, we go detail on sentiment analysis by extracting and deducing actionable information from customer feedback on a twitter platform of the Ecobank Group, one of the leading commercial banks in the sub-Sahara African region. To conclude, the term “customer satisfaction” is a measure of how products and/or services supplied by a company meet or surpass customer expectations in a given period. Therefore, managing and monitoring customer satisfaction is essential for businesses in the current competitive marketplace. Technology for that matter FinTech has made it easier for firms to obtain feedback from their customers to manage their satisfaction levels. On this note, customers usually share their experience with a product/service provided by a company in review sites, community blogs, and forums. These are some of the important sources of customer feedback for managerial decision making in the long-run period.
2.2. Sentiment analysis on twitter sites
Sentiment analysis refers to the use of natural language processing, text analysis, computational linguistics, and biometrics to systematically identify, extract, quantify, and study affective states and subjective information. It has been handled as a Natural Language Processing (NLP) task at many levels of data interpretation. In the beginning stage, it was considered as a document level classification task, thereafter at the sentence level and more recently at the phrase level(Ahmad et al., Citation2006; Cambria et al., Citation2013; Wertalik, Citation2017). Moreover, the microblog data, like Twitter on which users post real-time reactions to and opinions about everything poses newer and different challenges. An ample of studies’ results on sentiment analysis of Twitter data by(Algur & Patil, Citation2017; Botchway et al., Citation2019; Kouloumpis et al., Citation2011) were tailored towards service organizations in their quest to examine positive, negative and neutral opinions on product and service satisfaction. Given this, we argue in this paper that, understanding contextual emotions/feelings or opinions of people is vital with regards to product and service reviews so far as the study of financial marketing is concerned. Therefore, a financial firm like Ecobank Ghana in the context of this present study; gauging interest in their products and services by analyzing public opinion is key to their survival in today’s competitive business environment.
Again, social media websites, especially, Twitter and Facebook continue to be major communication tools (Jibril, Kwarteng, Chovancova, Vykydalova et al., Citation2019; Moussa et al., Citation2018) where users could share their ideas on several subjects under discussion. Sentiment analysis helps to determine the opinions or emotions expressed via these platforms(Ahmad et al., Citation2006; Davidov et al., Citation2010; Nabareseh et al., Citation2018). Generally, in broader scrutiny of this approach, a numeric sentiment score is assigned to each tweet in the dataset. Using a classification strategy, a tweet with a numeric sentiment score closer to zero shows a neutral sentiment whereas a tweet with a sentiment score greater than zero corresponds to positive sentiment. Again, a score of less than zero corresponds to negative sentiment (Hutto & Gilbert, Citation2014). With this assertion, customer relationship management (CRM) has become a fundamental tool for-profit and nonprofit business organizations to engage profitably to ensure customer satisfaction for business growth and development (Chen et al., Citation2012). Therefore, for businesses to obtain relevant information for relationship management, text mining has become imperative to many businesses especially digital marketing in the 21st century. This, however, has compared many financial institutions to consider their operations in responding to sophisticated customers (Linoff & Berry, Citation2011), and this consequently help in determining the factors which are pertinent to the customer’s selection process.
Nonetheless, in the works of (Irresberger et al., Citation2015; Rodano et al., Citation2016), they argued that big data analysis in contemporary marketing research is not only related to highly digitalized performing business but also take into consideration start-ups and micro-to small-to-medium firms that seek to face challenges of market development. By extension, business intelligence and analytics have emerged as one of the important areas and have drawn attention to scholars and practitioners in problem-solving in response to modern business trends. According to (Chen et al., Citation2012) text mining of customer’s opinion in the online platforms is viewed as a core component of Business Intelligence Research (BIR) which seeks to unravel hidden data which has an impact on business processes and evaluation. We, therefore, rely on the grounds of the literature review as a gauge to situate our study in a developing country setting so as evaluate the presence of integrated marketing communication (IMC) tool in one of the leading commercial banks as mentioned above.
Some articles have attempted to apply sentiment analyses to extract opinions expressed on social media referring to various disciplines in some countries in sub-Saharan Africa. In Ghana, Amaquandoh and Brown (Citation2008) performed a content analysis of visitors to tour sites in Cape Coast. In another article Nabareseh et al. (Citation2018) focused on leveraging fine-grained sentiment analysis for competitivity in the telecommunication sector in Ghana. To the best of our knowledge no article that focuses on analyzing social media sentiments of customers in the financial sector in sub-Saharan Africa using a pan-African bank as a case study.
2.3. Overview of Sub-Sahara African bank: Ecobank group
Ecobank Transnational Incorporated (“ETI”) is the parent company of the Ecobank Group, a leading independent Pan-African banking group. The Ecobank Group employs over 15,000 people and serves about 20 million customers in the consumer, commercial, and corporate banking sectors across 33 African countries. The Group has a banking license in France and representative offices in Addis Ababa, Ethiopia; Johannesburg, South Africa; Beijing, China; London, the UK, and Dubai, the United Arab Emirates. The Group offers a full suite of banking products, services, and solutions including bank and deposit accounts, loans, cash management, advisory, trade, securities, wealth, and asset management. ETI is listed on the Nigerian Stock Exchanges in Lagos, the Ghana Stock Exchange in Accra, and the Bourse Régionale des Valeurs Mobilières in Abidjan (please, visit: www.ecobank.com)
In Ghana, Ecobank Ghana is a commercial bank. It is one of the commercial banks licensed by the Bank of Ghana, the national banking regulator headquartered in Accra (national capital). It has about 1,617 employees (see https://ecobank.com/personal-banking). Ghana’s financial sector has gone through a lot of restructuring for decades, all geared towards the improvement of the sector to stand the test of global financial competition. Ghana’s financial sector is made up of both banks and non-banking institutions, whilst this study focuses on only the banking industry (Ecobank Ghana limited). With regards to the bank’s distribution channel, the bank strategically locates its retail outlets in the fastest growing towns and cities in Ghana to leverage its footprint to gain competitive advantage. It delivers its products and services through these principal channels: 67 Branches Network, 230 ATMs, Telephone Banking services via the Ecobank 24/7 Contact Centre, Remote banking on Ecobank’s Internet Banking platform, Mobile Banking and other E-Banking platforms (available at https://ecobank.com/gh/personal-banking/countries).
2.4. Research questions
Undeniably, there have been many studies by researchers that have shown considerable interest in user-generated content on social media including (Afful-Dadzie, et al., Citation2014, Aggarwal & Subbian Citation2014 and Akehurst, Citation2009). This paper employed opinion mining to do sentiment analysis using unstructured textual information on Twitter sites that has tweets containing the keyword “ecobank”. The following research questions guided the study:
Which lexicon produces the best output that describes the opinions of bank clients on twitter?
What insights can be gained from the expressions garnered from the Ecobank group twitter app?
Since Fintech has come to stay, what lessons can the bank learn from social media to improve their service delivery?
3. Methodology
Figure shows the entire workflow for the study which includes data source, method, and analysis. For this purpose, we collected tweets that mentioned the keyword “ecobank” on Twitter for a specified time interval. Like several other social media research, our data collection step precedes the implementation of our method and analysis phase. Data pre-processing helps to filter out and remove noise in our dataset. After data cleaning, sentiment analysis is performed on the collection of tweets using four different lexicons namely: Valence Aware Dictionary and sEntiment Reasnor—VADER (Hutto & Gilbert, Citation2014), AFINN (Nielson, Citation2011), SentiWordNet (Baccianella, Esuli, & Sebastiani, Citation2010) and Text Blob (Loria, Citation2018). The results of the sentiment analysis using each lexicon are compared against a human-labelled sample drawn from the dataset and then trained using the naive Bayes classifier to determine the best lexicon based on the accuracy score. We subsequently discuss the potential reasons for the results obtained. Finally, we construct word clouds using the tweets from all the two sentiment classes namely: positive and negative to understand the pain points expressed by customers and look for opportunities to connect with them.
3.1. Data collection and pre-processing
Among the Web 2.0 tools, Twitter, originally launched in 2006 has evolved as a major revolution in the field of social media. It has become the most preferred social media channel with 330 million monthly active users according to Statista, Number of monthly active twitter users worldwide from 1st quarter Citation2010to 1st quarter (2019). Twitter is freely available and allows registered users to post short messages with a character set limit of 280 to other registered users and vice versa (Pak & Paroubek, Citation2010). Its global connectivity, diverse user-base, and active user participation make it the qualitative and quantitative source from which sentiment rich data can be extracted and analysed. Consequently, we preferred using Twitter as our social media platform for conducting this study.
The dataset used for the study consists of 7,730 English tweets collected between 1 January 2015 and 31 December 2019 using an application called Twint (Poldi, Francesco, 2019). The tweets were crawled using the search keyword “ecobank”. Listing 3.1 provides the code details:
import twint
def getTweets(keyword)
# Utility function for scraping tweets
c = twint.Config() #setup TWINT config
c.Until = “2019–12-31”
c.Since = “2015–01-01”
c.Search = keyword
c.Store_csv = True
c.Output = “tweets.csv”
c.Hide_output = True
c.Count = True
c.Lang = “en”
twint.run.Search(c)
return
Listing 3.1 Snippet of code used to scrape tweets on Twitter
Some of the variables found in each tweet include message (the text contained in the tweet) tweet id (the unique code assigned to the tweet), date (the day and time of posted tweet), source (utility or device used to post the tweet) and likes (number of favourites per tweet) and language (the tweet language used). Some users normally post information that depicts sentiment using abbreviations, emoticons, URLs, slangs, or hashtags. A sample of tweets from the dataset depicting discussions on a range of topics including the bank’s corporate social responsibility, training programme on financial literacy and inclusion, support for financial technology innovation, commitment towards women empowerment and customers experiences is shown in Figure . We used Python with the NLTK library in our experiments (Bird et al., Citation2009) for data pre-processing tasks. In this phase, we cleaned the text by eliminating punctuations, stop words, URLs, slangs, @ symbol used to mention usernames, and converted all text to lowercase. Finally, terms with low frequency were omitted and meaningless words filtered out accordingly.
import re
def cleanTweet(tweet):
# Utility function for cleaning each tweet in the dataset
return ‘ ‘.join(re.sub(“(@[A-Za-z0-9]+)|([^0-9A-Za-z\t])|(\w+:\/\/\S+)”, “ “, tweet).split())
Listing 3.2 Code snippet for cleaning tweets
3.2. Lexicons for sentiment analysis
In this study, a lexicon-based approach was used to analyse the sentiment of each tweet. A sentiment lexicon is defined as a list of lexical features that are generally labeled based on their semantic orientation (Moussa et al., Citation2018). The semantic orientation could be positive or negative. Although the lexicon approach is an unsupervised method, it is considered a major sentiment analysis technique where the text in the dataset is categorized into a set of predefined sentiment classes. These sentiment scores are computed based on the lexicon.
VADER is a lexicon and rule-based sentiment analysis tool that is specifically attuned to sentiments expressed via social media. It is fast and computationally efficient without compromising accuracy. Training data is not required and works very well on social media text. Additionally, it does not suffer extremely from a speed-performance tradeoff. Given a document, VADER examines its lexical features to determine an initial sentiment score before applying five different rules based on grammatical conventions and syntax to amend that score. These rules handle capitalization and exclamation marks as sentiment amplifiers. Again, they also handle negations and contrastive conjunctions very well. VADER produces positive, negative, neutral, and compound scores for each tweet in the dataset. The positive, negative, and neutral scores are ratios for proportions of text that lie in these categories. The compound score is a metric that sums up all the lexicon ratings which have been normalized between −1(most extreme negative) and +1(most extreme positive) (Hutto & Gilbert, Citation2014).
SentiWordNet is a lexical resource for opinion mining and an extension of WordNet in which 147,306 synonym sets (synsets) are annotated with three numerical scores relating to positivity, negativity, objectivity. They describe how positive, negative, and objective the terms in the synset are. Each of the sentiment scores ranges from 0.0 to 1.0 and sums up to 1.0 for each synset.
Because the text in microblogs is different compared to text in reviews and blogs, some researchers built appropriate resources for microblogs. The AFINN lexicon created by Finn (Arup Nielsen in 2011) is one example. It contains a list of English terms manually rated for valence with an integer ranging between −5 (negative) and +5 (positive)by Finn Arup Neilsen in 2011. This lexicon is better equipped to handle tweets expressed using internet slangs and obscene words.
TextBlob (Loria, S. 2018) is a library for natural language processing that works very well with python. It assigns both polarity and subjectivity scores to each text in the dataset. The sentiment property returns a named tuple of the form sentiment (polarity, subjectivity). The polarity score is a float which ranges within the interval [−1,1]. The subjectivity is a value within the range [0.0, 1.0] where 0.0 and 1.0 are considered very objective very subjective respectively.
In this paper, we investigate four different sentiment lexicons in isolation with each other namely: VADER (Hutto & Gilbert, Citation2014), SentiWordNet (Barccianella. Ensuli, & Sebastiani, 2010) and AFINN (Nielson, 2011).
3.3. Word clouds
A word cloud (also known as text cloud or tag cloud) is a tool for visually summarising a large amount of text. In this study, we generated a word cloud to visualize the large volume of tweets for each sentiment category based on the VADER scores after removing stop words. The word clouds are created using the word cloud library available in Python programming language, based on the frequencies of the words used in the dataset to determine the size of the words. Thus, the bigger and bolder a word appears, the more frequent it is mentioned within a given context and the more important it is.
4. Results and discussion
In this section, we present and discuss the results obtained as answers to our research questions.
4.1. Analysing tweet sentiment based on lexicons
For this study, we performed sentiment analysis of 7,730 English tweets collected between 1 January 2015 and 31 December 2019 using all the four lexicons in isolation from each other. We generated an overall sentiment score for each tweet using each lexicon separately. The sentiment scores were classified into three groups namely: positive, negative, and neutral. Out of 7,730 tweets VADER classified 4,317 as positive while the remaining 1,233 and 2,180 were classified negative and neutral respectively. Similarly, the AFINN lexicon categorized 4,038 tweets as positive while the negative and neutral classes had 1,567 and 2,125 tweets each. Table depicts the classification of tweets based on all four lexicons. Again, Figure shows a graph of all the lexicons described in Table .
Table 2. Classification of tweets generated by each lexicon
It is also worthy to note that the positive sentiment category dominated three out of the four lexicons used in the study. These three lexicons are VADER, AFINN, and TextBlob. Interestingly, the SentiWordNet lexicon had the highest number of tweets with negative sentiments as well as the least number of tweets in the positive sentiment category. Again, the TextBlob lexicon had the highest number of tweets classified as neutral followed by VADER, AFINN, and SentiWordNet.
In response to research question 1, we manually label a sample of 773 tweets constituting 10% of the tweets in the dataset using two independent annotators. Out of 773 tweets that were hand-labeled, 525 was classified positive with 46 and 202 categorized as negative and neutral respectively. The inter-annotator agreement (Cohen’s Kappa) reached 0.75 which indicates a reasonable agreement level (Pustejovsky & Stubbs, Citation2013). We train the labeled tweets of each lexicon using the naive bayes classifier to obtain the respective classification scores. The classification accuracy (AC) score is defined in Equationequation (1)(1)
(1) based on the number of true positives (TP), the number of true negatives (TN), the number of false positives (FP) and the number of false negatives (FN) follows:
TP, TN, FP, and FN refer respectively to the number of true positive instances, the number of true negative instances, the number of false positive instances and the number of false negative instances. The accuracy values obtained for each lexicon when compared to the hand labeled datasets (positive and negative classes only) is shown in Table .
As can be seen from the results in Table , computing sentiments scores of tweets using the VADER lexicon outperforms all the other lexicons used in this study. This indeed confirms what is stated in the literature (Hutto & Gilbert, Citation2014) since it is accustomed to sentiments expressed on social media. Figure shows a distribution of sentiment scores using the VADER lexicon. We now proceed with our analysis using the results obtained via the VADER lexicon as our reference (see Figure ).
Table 3. AC scores for each lexicon
4.2. Analysing word clouds
To gain further insight into the opinions expressed in the tweets, we examine the word clouds generated for two sentiment categories based on the sentiment scores obtained via the VADER lexicon. Figure and Figure show word clouds for the positive and negative sentiment categories respectively.
Figure presents the word frequencies of English tweets classified as positive. Some words such as “please”, “hello”, “account”, “bank”, “number”, “nigeria”, were counted as frequent terms amongst several others with the word “please” being the most prominent. By observing this word cloud, there is a feeling that the bank pays very serious attention to the way it communicates and aids its customers. Sampling the tweets containing these terms can help us find out exactly what is being said in them. These tweets involved discussions regarding banking transactions, customer relations, and the country with the high customer segment. Since these discussions emanate from tweets that fall within the positive sentiment category, we believe that the bank is getting things right with such matters.
We now consider Figure which also contains text from 1,233 tweets expressing negative sentiments visualized as a word cloud after removing stop words. Overall, words such as “nigeria”, “money”, “account”, “eco”, “people”, “atm”, and “customer” were counted as frequent terms amongst several others. Interestingly, the term “nigeria” was the most dominant in this text cloud. Hence, we can deduce that the tweets contained discussions regarding frustrations (pain-points see Figure ) customers face concerning the usage of the e-banking solutions provided by the bank. These revelations, when taken seriously, can provide valuable insights to the bank to make meaningful changes to boost their service delivery. Again, it will be quite interesting to investigate tweets containing terms such as “fraud” “scam”, “mobile” and “atm” to find out the message they convey. This result in our view answers research question 2 adequately.
Our work corroborates recent studies by researchers (Grégoire et al., Citation2015) which shows that organisations such as financial service firms are increasingly interested in looking for better ways of improving the service experience of customers. As such, the proactive engagement by financial organizations with customers via social media (e.g., Twitter) with the view to respond timely to their specific concerns, inquiries, and queries can go a long way to improve the quality of service experience enjoyed by clients. According to the Ernst & Young (Citation2017) global banking survey, customer experience combined with traditional elements such as price, product, the scale of branch network with a renewed emphasis on simplicity and convenience of interactions across several communication channels holds the key to the prosperity of today’s financial companies. Ibrahim and Wang (Citation2019) in their work used a lexicon-based approach to analyse online customer tweets before using time series to analyse the trends exhibited by the volume and sentiments of tweets. The results of our study demonstrate that in today’s world of empowered customers, financial houses need to focus on customer engagement to enhance customer experience via social media channels (e.g., Twitter) since the meaning of competitive advantage has shifted from purely competing over price and product to building loyalty and trust.
5. Research contributions and conclusion
In financial economics, information seeking is very useful for day-to-day activities with regards to pre and post-financial transactions particularly among banking institutions and other financial outlets in general. At the same time, this acquired information from the advent FinTech help particularly these banks to effectively and efficiently distribute resources in markets in which decisions are made under uncertainty(Joung et al., Citation2019). Therefore, financial decisions by banks must often consider future events, whether those be related to new products/services, individual stocks, portfolios, or the market as a whole so far as information seeking and knowledge management are concerned.
The study provides important contributions for researchers and practitioners in online marketing (banking) and social media literature. Theoretically, our work offers a better understanding of customers’ opinions towards online banking and provides insight into what they are thinking about by analysing their views/opinions expressed on Twitter. Adopting a data-driven approach for the study produces robust and generalizable outputs compared to conventional marketing approaches such as customer surveys, focus groups, and interviews. Similarly, this study further augments the existing literature by offering some empirical evidence from a developing country where internet penetration is relatively low despite the effort made towards technological advancement across the globe. Notwithstanding, this study would beef up the related works with regards to some theories within the confines of information system and technology adoption since the application of ICT in the business operation is concerned. The study also encourages researchers from other fields of studies (ie. Psychology, Economics, Business, Computer sciences, etc.) to ensure a sound collaboration of multi-discipline study/approach since the technology and innovation has come to stay.
From a broader managerial perspective, the study findings can make firms responsive to customer needs, think strategically, and focus on areas of service provision that are vital to business growth. It is imperative to note that the competitiveness of services-based organizations, particularly banks, in which our study focused on, clamour for potential customers these days. Given this, the present study offered a marketing and financial engineering strategy to curtail or reply to the intercepted tweets in a more friendly manner to ensure effective and efficient customer relationship management service in the banking activities. Again, since communication is a two-way concept, the channel (Twitter) which served as a virtual platform would be easier and faster to convey and exchange relevant information with less time consumed. To ensure business growth and survival, this study further suggests that practitioners of digital marketing, as well as digital finance, should do more in terms of technological investment to meet this era of a technological outburst. Interestingly consumers can use sentiment analysis to search for a product or service before making a purchase decision. Whereas, marketers can use this to research public opinion of their company and products, or to analyse customer satisfaction. Similarly, organisations can also use this to gather critical feedback about problems in newly released products.
This study aimed at gaining insight into the opinions expressed in tweets associated with a financial institution operating in sub-Saharan Africa via sentiment analysis. We used Twitter data because it is noted in the literature as the popular choice for text analysis tasks since it has a limited number of characters (thus, 280) is allowed and it also includes a global use of it to express opinions on different issues among people of all ages, races, cultures, genders among others. To achieve this, we downloaded English tweets from twitter using an application called Twint (Poldi, Francesco, 2019). After data pre-processing, we investigated four sentiment lexicons namely VADER, AFINN, SentiWordNet, and TextBlob separately from each other. The overall discussion was positive when VADER, AFINN, and TextBlob lexicons were applied to the dataset suggesting that positive tweets govern the dataset concerning these three lexicons. In the case of the SentiWordNet lexicon, the discussion was negative. Our results show the VADER lexicon produces the best performance in terms of accuracy and computational efficiency. Its unique suitability for social media text contributed greatly to its high performance. The tweets in the dataset used in the study contain customer opinions ranging from outrage to satisfaction and happiness which duly reflects in the sentiment categories obtained. The sentiment categories based on the VADER lexicon were positive, negative, and neutral. Generating word clouds based on these sentiment categories enabled us to gain further insights into the discussions contained within the tweets. The discussions in the positive sentiment word cloud bothered on banking transactions, customer relations, and the country with the high customer segment. Again, the revelations from the negative sentiment word cloud identify areas upsetting customers where management can make meaningful changes to enhance service delivery and understand customers’ behaviour. In summary, using consumer sentiment data from social media channels might show the greater marginal significance for measuring consumer expectation/confidence and thereby forecasting their transaction behaviour, since it captures true information about consumer purchasing/transaction behaviour. Nevertheless, care needs to be taken with social media contents as these fluctuate in their positive and negative outcomes to their topics over time. The same individual may give positive sentiment at one point of time and perhaps in six months provide negative sentiment (Shayaa et al., Citation2018).
However, this study is not without limitation. First, it considers only a single platform (twitter app) of the aforementioned bank without recourse to other equally important social medial channels. Second, the sentiment lexicons (algorithm) used may not necessarily be the best/robust procedures to ascertain the overall objective of the study since other approaches such as the Fuzzy rule-based method and topic modeling could also be used in the current study. Unfortunately, ignoring non-English tweets was a major limitation to this study since their inclusion could potentially enrich the work by providing interesting insights that could be exploited. In the future, it will be valuable to include other methods such as topic modeling and time series to improve the analysis of social media data.
Acknowledgements
This work was supported by the Internal Grant Agency of Tomas Bata University under the Projects no. IGA/CebiaTech/2020/001, IGA/FaME/2019/008 and IGA/FaME/2020/002. The work was further supported by the resources of A.I. Lab at the Faculty of Applied Informatics, Tomas Bata University in Zlin (ailab.fai.utb.cz). We are also thankful to the academic advice offered by Prof. Boris Popesko (Vice-dean for Research and Business Liaison) and Dr. Bedrich Zimola, all at the Faculty of Management and Economics of the above-mentioned University.
Additional information
Funding
Notes on contributors
Raphael Kwaku Botchway
Raphael Kwaku Botchway is a Ph.D. candidate with research interest in Soft Computing, Social Media and Text Analytics at the Faculty of Applied Informatics, Tomas Bata University in Zlin, Czech Republic. His research results have been presented at numerous reputable international conferences.
Abdul Bashiru Jibril
Abdul Bashiru Jibril is a Doctoral Researcher at the Department of Management and Marketing at TBU in Zlin. He is particularly interested in deploying data mining techniques in extracting intelligence to improve business decision making. The results of his research have been published in peer-reviewed scientific journals and presented at numerous international conferences.
Zuzana Komínková Oplatková
Zuzana Komínková Oplatková is an Associate Professor at the Faculty of Applied Informatics, Tomas Bata University in Zlin, Czech Republic. Her research results and publications (see ailab.fai.utb.cz/our-team)
Miloslava Chovancová
Miloslava Chovancová is an Associate Professor at the Department of Management and Marketing at TBU in Zlin. Her research spans Consumer behaviour and Brand management, and SME’s development. She has published in peer-reviewed scientific journals and presented at numerous international conferences.
References
- Abbasi, T., & Weigand, H. (2017). The impact of digital financial services on firm’s performance: A literature review. ArXiv Preprint, ArXiv, 1705.10294. https://arxiv.org/abs/1705.10294
- Afful-Dadzie, E., Nabareseh, S., Komínková, O. Z., & Klímek, P. (2014). Enterprise Competitive Analysis and Consumer Sentiments on Social Media. In Proceedings of 3rd International Conference on Data Management Technologies and Applications (pp. 22-32). SCITEPRESS-Science and Technology Publications, Lda. doi:10.5220/0004991300220032
- Aggarwal, C., & Subbian, K. (2014). Evolutionary network analysis: A survey. ACM Computing Surveys (CSUR), 47(1),1-19. doi:https://doi.org/10.1145/2601412
- Ahmad, K., Gillam, L., & Cheng, D. (2006). Sentiments on a grid: Analysis of streaming news and views. LREC, 2524–2527. http://www.lrec-conf.org/proceedings/lrec2006/pdf/394_pdf.pdf
- Akehurst, G. (2009). User generated content: the use of blogs for tourism organisations and tourism consumers. Service business, 3(1), 51. doi:https://doi.org/10.1007/s11628-008-0054-2
- Algur, S. P., & Patil, R. H. (2017). Sentiment analysis by identifying the speaker’s polarity in Twitter data. 2017 international conference on electrical, electronics, communication, computer, and optimization techniques (ICEECCOT), 1–5. Mysuru, India: IEEE.
- Amaquandoh, E., & Brown, D. O. (2008). A content analysis of sentiments expressed by visitors to cultural heritage sites at the elmina and cape coast former slave castles in Ghana. Consortium Journal of Hospitality & Tourism, 12, 2. http://eds.b.ebscohost.com/abstract?site=eds&scope=site&jrnl=15350568&asa=Y&AN=31185216&h=R2u5pmEUTG2JsSBc3N%2fOKBdFmECTxJdJSpHwmbOQz6bUnvUn%2fIWJ%2bRIu6QdFXKpEo1Fx%2bmivnCBJWCGOJpY2cQ%3d%3d&crl=c&resultLocal=ErrCrlNoResults&resultNs=Ehost&crlhashurl=login.aspx%3fdirect%3dtrue%26profile%3dehost%26scope%3dsite%26authtype%3dcrawler%26jrnl%3d15350568%26asa%3dY%26AN%3d31185216
- Baccianella, S., Esuli, A., & Sebastiani, F. (2010). Sentiwordnet 3.0: An enhanced lexical resource for sentiment analysis and opinion mining. Lrec, 10, 2200-2204. http://lrec-conf.org/proceedings/lrec2010/pdf/769_Paper.pdf
- Bird, S., Klein, E., & Loper, E. (2009). Natural language processing with Python: analyzing text with the natural language toolkit. O'Reilly Media, Inc. http://117.3.71.125:8080/dspace/bitstream/DHKTDN/6460/1/Natural%20Language%20Processing%20with%20Python.4149.pdf
- Botchway, R. K., Jibril, A. B., Kwarteng, M. A., Chovancova, M., & Oplatková, Z. K. (2019). A review of social media posts from UniCredit bank in Europe: A sentiment analysis approach. Proceedings of the 3rd international conference on business and information Management, 74–79. Paris, France: ACM.
- Bott, R. (2014). Marketing na Internet. Igarss, 2014. https://doi.org/10.1007/s13398-014-0173-7.2
- Bughin, J., Chui, M., & Miller, A. (2009). How companies are benefiting from Web 2.0. McKinsey Quarterly, 9(4), 84–85. https://www.mckinsey.com/business-functions/mckinsey-digital/our-insights/how-companies-are-benefiting-from-web-20-mckinsey-global-survey-results
- Cambria, E., Schuller, B., Xia, Y., & Havasi, C. (2013). New avenues in opinion mining and sentiment analysis. IEEE Intelligent Systems, 28(2), 15–21. https://doi.org/10.1109/MIS.2013.30
- Chen, H., Chiang, R. H. L., & Storey, V. C. (2012). Business intelligence and analytics: From big data to big impact. MIS Quarterly, 36(4), 4. https://doi.org/10.2307/41703503
- Das, G., Agarwal, J., Malhotra, N. K., & Varshneya, G. (2019). Does brand experience translate into brand commitment?: A mediated-moderation model of brand passion and perceived brand ethicality. Journal of Business Research, 95, 479–490. https://doi.org/10.1016/j.jbusres.2018.05.026
- Davidov, D., Tsur, O., & Rappoport, A. (2010). Enhanced sentiment learning using twitter hashtags and smileys. Proceedings of the 23rd international conference on computational linguistics: Posters, 241–249. Association for Computational Linguistics. Stroudsburg, PA.
- Eisenmann, T. R. (2006). Internet companies’ growth strategies: Determinants of investment intensity and long‐term performance. Strategic Management Journal, 27(12), 1183–1204. https://doi.org/10.1002/smj.567
- Ernst & Young. (2011). The digitisation of everything. http://www.ey.com/Publication/vwLUAssets/The_digitisation_of_everything_-_How_organisations_must_adapt_to_changing_consumer_behaviour/$FILE/EY_Digitisation_of_everything.pdf
- Ernst & Young. (2017). Customer experience: Innovate like a fintech. https://www.ey.com/Publication/vwLUAssets/ey-gcbs-customerexperience/$FILE/ey-gcbs-customer-experience.pdf
- Farina, V., Gabbi, G., & Previati, D. (2014). Good news, bad news: A proposal to measure banks’ reputation using Twitter. In T. Lindblom (Ed.), Governance, regulation and bank stability (pp. 242–259). Springer.
- Gillin, P., & Schwartzman, E. (2010). Social marketing to the business customer: Listen to your B2B market, generate major account leads, and build client relationships. John Wiley & Sons.
- Grégoire, Y., Salle, A., & Tripp, T. M. (2015). Managing social media crises with your customers: The good, the bad, and the ugly. Business Horizons, 58(2), 173–182. https://doi.org/10.1016/j.bushor.2014.11.001
- Hutto, C. J., & Gilbert, E. (2014). Vader: A parsimonious rule-based model for sentiment analysis of social media text. Eighth international AAAI conference on weblogs and social media. Ann Arbor, Michigan.
- Ibrahim, N. F., & Wang, X. (2019). Decoding the sentiment dynamics of online retailing customers: Time series analysis of social media. Computers in Human Behavior, 96, 32–45. https://doi.org/10.1016/j.chb.2019.02.004
- Irresberger, F., Mühlnickel, J., & Weiß, G. N. F. (2015). Explaining bank stock performance with crisis sentiment. Journal of Banking & Finance, 59, 311–329. https://doi.org/10.1016/j.jbankfin.2015.06.001
- Jibril, A. B., Kwarteng, M. A., Chovancova, M., & Pilik, M. (2019). The impact of social media on consumer-brand loyalty: A mediating role of online based-brand community. Cogent Business & Management, 6(1), 1673640. https://doi.org/10.1080/23311975.2019.1673640
- Jibril, A. B., Kwarteng, M. A., Chovancova, M., & Vykydalova, N. (2019). The role of the social media brand community on consumers’ purchasing attitude. ECSM 2019 6th European conference on social media, 135. Academic Conferences and publishing limited. University of Brighton, UK.
- Joung, J., Jung, K., Ko, S., & Kim, K. (2019). Customer complaints analysis using text mining and outcome-driven innovation method for market-oriented product development. Sustainability, 11(1), 40. https://doi.org/10.3390/su11010040
- Kang, D., & Park, Y. (2014). based measurement of customer satisfaction in mobile service: Sentiment analysis and VIKOR approach. Expert Systems with Applications, 41(4), 1041–1050. https://doi.org/10.1016/j.eswa.2013.07.101
- Kennedy, H. (2012). Perspectives on sentiment analysis. Journal of Broadcasting & Electronic Media, 56(4), 435–450. https://doi.org/10.1080/08838151.2012.732141
- Kouloumpis, E., Wilson, T., & Moore, J. (2011). Twitter sentiment analysis: The good the bad and the omg! Fifth international AAAI conference on weblogs and social media. Catalonia, Spain.
- Linoff, G. S., & Berry, M. J. A. (2011). Data mining techniques: For marketing, sales, and customer relationship management. John Wiley & Sons.
- Loria, S. (2018). textblob Documentation. Release 0.15, 2. https://buildmedia.readthedocs.org/media/pdf/textblob/latest/textblob.pdf
- Mogaji, E., Farinloye, T., & Aririguzoh, S. (2016). Factors shaping attitudes towards UK bank brands: An exploratory analysis of social media data. Cogent Business & Management, 3(1), 1223389. https://doi.org/10.1080/23311975.2016.1223389
- Moghaddam, S. (2015). Beyond sentiment analysis: Mining defects and improvements from customer feedback. European conference on information retrieval, 400–410. Vienna, Austria: Springer.
- Moussa, M. E., Mohamed, E. H., & Haggag, M. H. (2018). A generic lexicon-based framework for sentiment analysis. International Journal of Computers and Applications, 42(5), 1–11. https://doi.org/10.1080/1206212X.2018.1483813
- Nabareseh, S., Afful-Dadzie, E., & Klimek, P. (2018). Leveraging fine-grained sentiment analysis for competitivity. Journal of Information & Knowledge Management, 17(2), 1850018. https://doi.org/10.1142/S0219649218500181
- Nielson, K. B. (2011). Self-study with language learning software in the workplace: What happens?. Language Learning & Technology, 15(3),110-129. http://llt.msu.edu/issues/october2011/nielson.pdf
- Pak, A., & Paroubek, P. (2010, May). Twitter as a corpus for sentiment analysis and opinion mining. LREc, 10(2010), 1320–1326. https://lexitron.nectec.or.th/public/LREC-2010_Malta/pdf/385_Paper.pdf
- Pustejovsky, J., & Stubbs, A. (2013). Natural Language Annotation for Machine Learning. OReilly Media.. Inc.
- Raicu, I., & Turkes, M. C. (2016). An opinion mining and sentiment analysis approach for evaluating customer satisfaction in a digital banking environment. Annales Universitatis Apulensis Series Oeconomica, 2(18), 1–16. http://www.journals.uab.ro/index.php/oeconomica/article/view/321/312
- Rodano, G., Serrano-Velarde, N., & Tarantino, E. (2016). Bankruptcy law and bank financing. Journal of Financial Economics, 120(2), 363–382. https://doi.org/10.1016/j.jfineco.2016.01.016
- Sailunaz, K., & Alhajj, R. (2019). Emotion and sentiment analysis from Twitter text. Journal of Computational Science, 36, 101003. https://doi.org/10.1016/j.jocs.2019.05.009
- Shayaa, S., Ainin, S., Jaafar, N. I., Zakaria, S. B., Phoong, S. W., Yeong, W. C., Zahid Piprani, A., Zahid Piprani, A., & Al-Garadi, A. (2018). Linking consumer confidence index and social media sentiment analysis. Cogent Business & Management, 5(1), 1509424. https://doi.org/10.1080/23311975.2018.1509424
- Statista, Number of monthly active twitter users worldwide from 1st quarter 2010 to 1st quarter. 2019. (in millions). [Online]. https://www.statista.com/statistics/282087/number-of-monthly-active-twitter-users
- Tafesse, W., & Wien, A. (2017). A framework for categorizing social media posts. Cogent Business & Management, 4(1), 1284390. https://doi.org/10.1080/23311975.2017.1284390
- Tafesse, W., & Wien, A. (2018). Implementing social media marketing strategically: An empirical assessment. Journal of Marketing Management, 24(1-2), 1–18.
- van Sinderen, M., & Almeida, J. P. A. (2011). Empowering enterprises through next-generation enterprise computing. Taylor & Francis.
- Wertalik, D. (2017). Social media and building a connected college. Cogent Business & Management, 4(1), 1320836. https://doi.org/10.1080/23311975.2017.1320836
- Wright, L. T., Robin, R., Stone, M., & Aravopoulou, D. E. (2019). Adoption of big data technology for innovation in B2B marketing. Journal of Business-to-Business Marketing, 26(3–4), 281–293. https://doi.org/10.1080/1051712X.2019.1611082