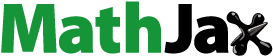
Abstract
Is the poor aggregate employment growth of the manufacturing sector in Sub-Saharan African countries the outcome of inadequate job creation or rival forces of job creation and job destruction acting in opposite direction? Using a unique dataset from the Kenyan manufacturing sector, we examine this question using ordinary least squares method and feasible generalized least squares technique. We find simultaneous high rates of job creation and job destruction. In most cases, job creation in the manufacturing sector was not adequate to offset the rapid destruction of jobs. Second, we find that the presence of simultaneously high rates of job creation and job destruction cut across firm size and age. Third, we find that young firms account for almost all of the net job creation rates implying that the firm’s age is important in shaping its contribution to net job creation rates. Our findings have practical implications. First, job creation policies alone without policies that mitigate the destruction of jobs created might not be successful. For job creation to be successful in the manufacturing sector, effort should be made towards developing strategies that mitigate the destruction of jobs created. This is because job creation and destruction rates simultaneously follow each other. Second, to expand employment growth, effort should be dedicated to supporting young firms (6–10 years), through possibly, tax relief, providing technology of production or any possible government subsidy that can help these firms grow and survive in the turbulent business environment.
PUBLIC INTEREST STATEMENT
Job creation has become a matter of urgency for many Sub-Saharan African countries that are grappling with unemployment. In this paper, we examine whether the poor aggregate employment growth of the manufacturing sector in Sub-Saharan African countries is the outcome of inadequate job creation or rival forces of job creation and job destruction acting in opposite direction. Across firm size and age, we find evidence of simultaneously high rates of job creation and job creation acting in opposite direction. Job creation rates in each firm size and age category are closely matched by almost similar rates of job destruction across firms of different sizes and ages. Moreover, these findings reveal that young firms account for almost all of the net job creation rates implying that the firm’s age is important in shaping its contribution to job creation. Policies targeting job creation in the manufacturing sector should be directed at young firms if more jobs are to be created.
1. Introduction
Is the poor aggregate employment growth of the Kenyan manufacturing sector the outcome of inadequate job creation or the consequence of rival forces of job creation and job destruction acting in opposite direction? Why is unemployment still an obstacle to the bulk of Sub-Saharan African countries? Can limited job creation account for the slow employment growth in sub-Saharan African countries or there are other factors driving unemployment in these countries? A number of Sub-Saharan African economies have received considerable criticism for the slow growth of employment during the past decades. When viewed from Gross domestic product (GDP) perspective, there is evidence that the region has been recording impressive levels of economic growth that surpasses 5% per annum for the past two decades (Rodrik, Citation2018). However, this impressive record of economic growth is not equally matched by high rates of job creation to sufficiently absorb the labor force. Consequently, unemployment still remains widespread, hindering the realization of sufficient economic progress of the population (ILO, Citation2014).
Economic theory, as postulated by Kaldo (Citation1960) emphasizes the importance of the manufacturing sector as an engine of growth. According to Kaldo (Citation1960), the expansion of the manufacturing sector creates backward and forward linkages that are beneficial to other sectors of the economy. The growth of the manufacturing sector should facilitate the absorption of increasing quantities of goods and services produced by other sectors of the economy. Moreover, the expansion of the manufacturing sector generates increased demand of other kinds of services, such as insurance, banking and accounting, among others. Additionally, as demand for goods and services from other sectors increases, there will be induced growth in these sectors which results in the increase in employment opportunities. In Kaldo’s view, the manufacturing sector possesses great potential to stimulating the expansion of non-manufacturing sector and growing the economies of the world. Thus, he summarized the sector’s potential in some empirical regularities and three laws, as follows: (i) The manufacturing sector is the engine of economic growth, (ii) the manufacturing sector’s productivity is positively correlated with the growth of the same sector, (iii) the productivity of the other sectors of the economy is positively correlated with the growth of the manufacturing sector.
Therefore, the thesis of Kaldo’s work is that for economies to realize positive employment growth, the manufacturing sector (taken to be the major engine of growth) must make a significant contribution to GDP growth, and generate enough jobs to absorb the skilled and unemployed population in countries facing pervasive unemployment rates. Given that the manufacturing sector is considered crucial to generating economic growth, developing this sector remains crucial to most Sub-Saharan African countries. This sector has failed to be the principal source of valuable employment to the workforce in Sub-Saharan African region despite significant investments and trade reforms aimed at bolstering its performance (Rodrik, Citation2018).
Using a unique dataset, this paper gives an unparalleled account of job flows for Kenya and possibly for the region, which is needed to explain total employment growth for a Sub-Saharan African economy. The data allow us to track changes in employment over a period of time (8 years). The choice of the Kenyan manufacturing sector is majorly driven by the availability of data and the fact that the country’s manufacturing sector is significantly large. Moreover, Sub-Saharan African economies share some common characteristics making the Kenyan case useful from a regional context. This paper is inspired by one important question; is the poor aggregate employment growth of the Kenyan manufacturing sector the outcome of inadequate job creation or the consequence of rival forces of job creation and job destruction acting in opposing directions? To address this question, we use firm-level panel data that covers Eight years, from 1992 to 1999. We structure the analysis around three objectives: (i) To assess the pattern of job creation and job destruction in the Kenyan manufacturing sector during the period under investigation, (ii) To examine the rates of job creation and job destruction in the country’s manufacturing sector, (iii) To evaluate how firm characteristics (size and age) shape job reallocation and employment growth, as these have been shown to be important characteristics (S. Esaku, Citation2020a, Citation2020b).
Accordingly, using macroeconomic outlook, some studies have tried to account for the poor contribution of the manufacturing sector to job creation by attributing it to the low levels of investment to expand the productive capacity of the sector and competing priorities that require more attention (Collier & Gunning, Citation1999). However, some microeconomics analysis is required to evaluate the pattern of job flows in the region and provide a better overview of firm-level variations that shape job creation and destruction. Bigsten and Gebreeyesus (Citation2007) argue that Sub-Saharan African manufacturing firms display production behavior similar to that in economies that are developed but they are not generating enough jobs to absorb the increasing labor force. With manufacturing behavior similar to those in developed economies, Sub-Saharan African firms are expected to generate jobs at rates similar to those in the developed economies. However, this is not the case as Sub-Saharan African firms perform dismally in terms of job creation. Consequently, there is a common view that the region is experiencing early deindustrialization as countries are not adequately investing in the manufacturing sector as was the case in the 1980s and 1990s. Therefore, there is now growing interest to examine firm-level evidence that explains gross job flows, and the fact that there are simultaneous job creation and destruction. For example, Davis and Haltiwanger (Citation1992) study job flows in the US manufacturing sector and find high rates of annual gross job creation and destruction that average 9.2% and 11.3% respectively. Their finding gives support to one important stylized fact about the presence of concurrently pervasive rates of job creation and destruction even within narrowly defined industries of the economy.
Further, it is documented that high rates of job creation and destruction generate pervasive rates of job reallocation that show variation in employment opportunities in most businesses. Accordingly, Davis and Haltiwanger (Citation1992) find that job creation and destruction rates are persistent across years and plants implying that most of the yearly job creation and destruction cannot be addressed by interim measures like short-term layoffs and recall policies because firm-level employment changes usually persist for some considerable periods, by which small and very young firms experience high adjustment rates. The nature of job destruction in the developed economies is considered more volatile than job creation, implying that these economies experience counter-cyclical reshuffling of jobs across firms, an indicator of the level of labor market adjustment and efficient reallocation of much needed productive resources. Consequently, some studies have linked gross job flows to firm characteristics to explain the corresponding contributions of births, growth, decline and deaths of firms.
Globally, there is some effort towards the examination of gross job flows in the manufacturing sector, focusing on differences in establishment-level employment dynamics (Chege & Wang, Citation2020; Davis & Haltiwanger, Citation1992; Yazdanfar & Ohman, Citation2018), the role of small firms in employment growth for the UK (Hijzen et al., Citation2010). In the African continent, studies have analyzed how firm size and age shape the growth of employment (see Bigsten & Gebreeyesus, Citation2007). However, there is a limited attempt to study job flows in the Sub-Saharan African region, except studies by Shiferaw and Bedi (Citation2013) with a focus on the Ethiopian manufacturing sector; Kerr et al. (Citation2014) for South Africa, Klapper and Richmond (Citation2011), for Cote d’Ivoire. However, these studies have not examined the Kenyan manufacturing sector, which this paper will do.
This paper contributes to the literature on employment dynamics by providing a detailed analysis of job flows in the Sub-Saharan African context using firm-level data from Kenya. We recognize that the lowering of trade barriers has caused significant changes in the Kenyan economy (S. Esaku, Citation2020c) that are crucial in the growth of employment. Although this paper is similar to the study by Ayyagari et al. (Citation2014), Shiferaw and Bedi (Citation2013), and Kerr et al. (Citation2014), it is different in some respects. The analysis by Ayyagari et al. (Citation2014) focuses on unveiling the firms who create jobs in developing countries and they use cross-country analysis. Similarly, Shiferaw and Bedi (Citation2013) examine the dynamics of job flows, but focus on Ethiopian manufacturing, which is a different context from the focus of this paper. Similarly, Kerr et al. (Citation2014) focus on the South African labor market. However, this paper analyzes job flows in the Kenyan manufacturing sector with an emphasis on job creation and destruction during the period 1992–1999. As shown by Esaku and Krugell (Citation2020), the manufacturing sector plays an important role in Sub-Saharan Africa especially by facilitating technology transfers which leads to productivity improvements.
This paper is organized as follows. Section 2 reviews the related literature, while section 3 gives the description of the data. Section 4 presents the methodology, while Section 5 provides the results of the empirical work and Section 6 concludes.
2. Review of related literature
Recent empirical work has documented how firms differ in their employment levels. This observed firm heterogeneity is the main feature of gross job flows in the world economy and has stimulated research in the employment dynamics. Even within narrowly defined industries, it is well documented that firms producing similar goods face different rates of job creation and job destruction, which in most cases, occurs simultaneously (Davis & Haltiwanger, Citation1992). Recently, Shiferaw and Bedi (Citation2013) find that job creation rates in the Ethiopian manufacturing sector during heightened and slow economic activity averaged 18% and 10%, respectively. Moreover, job reallocation rates across firms reflect a pro-cyclical pattern, contrary to findings in the developed economies. This may indicate that firms in the Ethiopian manufacturing sector do not consider the business cycle when creating jobs. Additionally, their findings reveal that it is the majorly firm entry and exit that drive job creation and destruction, as newly created firms join the market while those that face negative economic shocks exit.
Similarly, Kerr et al. (Citation2014), using the South African data from 2005 to 2011 show that, on average, rates of job creation and destruction in the South African economy averaged 9.9% and 10%, respectively, per year. These authors show how nearly 20% of employment is as a result of job reallocation, which is similar to patterns observed in the US economy, although lower than what is observed in other developed countries like the UK. For-example, Hijzen et al. (Citation2010) study of the UK manufacturing sector finds high rates of job reallocation, which averaged 28%. Additionally, their study finds that small firms contribute a large proportion of jobs created and destroyed (65%) and (45%), respectively; with job destruction rates higher in industries that face accelerated growth in import intensity.
Recently, empirical work by Klapper and Richmond (Citation2011) presents evidence from Cote d’Ivoire indicating that rates of job creation and destruction in the period 1976–1997 reached 14.7% and 15.3%, respectively. Overall, the study finds that about 8% of the jobs created are due to firm entry, while 8.3% of job destruction is due to the shrinking nature of incumbent firms. This evidence points to the fact that firm entry and exit play a significant contribution to job creation and destruction in most of the manufacturing industries. This evidence is suggestive of the crucial role business start-ups play in growing employment. In another study by Haltiwanger et al. (Citation2013), the contribution of business start-ups and young businesses in stimulating employment growth is highly emphasized. Additionally, Cho et al. (Citation2017) find a similar trend in Korea, where much of the employment growth is driven by firm entry of very small firms. To this end, empirical evidence shows a positive rank correlation of rates of job reallocation across industries which implies that some industries may be having job reallocation rates which are above average (Haltiwanger et al., Citation2008). Accordingly, Baldwin et al. (Citation1998) find that patterns of job flow in the manufacturing sectors of the US and Canada display similar trends; suggesting that job reallocation masks common industry level features like cost structures, technology of production and demand factors that may be driving job reallocation across different economies. Most importantly, it is documented that much of the job reallocation happens within industries rather than across industries with job creation and destruction rates being persistent, tending towards optimal firm size rather than transitory reshuffling of jobs (Davis & Haltiwanger, Citation1992).
Correspondingly, coupled with the persistent nature of jobs created and destroyed per annum, the literature on heterogeneity in employment documents the cyclical (it intensifies during economic boom or periods of high employment growth and wanes during recession) nature of job reallocation across employers in Sub-African economies (Shiferaw & Bedi, Citation2013) conflicting patterns of job reallocation observed in developed economies. For example, Davis and Haltiwanger (Citation1992) find evidence of counter-cyclical nature of job reallocation in the US manufacturing sector reflecting time variation across the sizes of specific firm-level employment activity which are not related to sectoral differences in the average employment response to total fluctuations. Correspondingly, these patterns in time differences of job reallocation intensity vary in firm size, ownership status and age. Consequently, Baldwin et al. (Citation1998) provide evidence showing that, overall, the manufacturing sector employment growth in the US and Canada is followed by a decline in rates of job creation in the absence of notable increases in jobs created. Moreover, the contraction in total employment growth is commonly associated with a rapid rise in job destruction with only a marginal drop in job creation. This shows that job destruction is a key driver of counter-cyclical movement of job reallocation (Shiferaw & Bedi, Citation2013).
On the role of firm characteristics like size and age, a number of studies have provided conflicting evidence on this issue. For example, following the influential work by Birch (Citation1981), some studies have supported Birch’s work. The thesis of Birch’s contribution is that small firms drive employment growth. Neumark et al. (Citation2011) provide evidence to support Birch’s influential work. In their study of US economy during 1992–2004, they find evidence that small firms indeed account for most of the job creation. Similarly, Heyman et al. (Citation2018) examine employment dynamics in the Swedish business sector and find that a large number of net job creation occurred in small firms. This is consistent with earlier findings by Birch (Birch, Citation1981) and findings by Chege and Wang (Citation2020), and for the case of foreign firms, Binetti and Coniglio (Citation2019). Conversely, the evidence that small firms drive employment growth is highly contested, mainly on methodological grounds. For example, Davis et al. (Citation1996) suggest that using the initial size of the firm employment, as was the case with Birch (Citation1981) leads to biased estimates in favor of small firms because of regression to the mean fallacy, whereby small firms emerging from a negative shock will be seen to grow faster than large firms. Consequently, Haltiwanger et al. (Citation2013) suggest that it is firm age rather than size that is important; claiming that, once firm age is controlled for, there is no systematic inverse connection between firm size and employment growth.
On the African scene, Rijkers et al. (Citation2014) study job creation in Tunisia and find a positive relationship between firm size and net job creation, in line with previous studies by Neumark et al. (Citation2011). Additionally, Ayyagari et al. (Citation2014) using data from a number of developing economies analyze the role of small firms in generating jobs and provide evidence to support the view that small firms contribute disproportionately to net employment growth. However, Kerr et al. (Citation2014) provide evidence inconsistent with the popular view. They argue that it is the large firms who create more jobs and not the small firms. Overall, the literature on employment dynamics in the African has just started to evolve and more needs to be done to explore these dynamics in the context of African economies.
3. The data
This paper uses a strongly balanced panel data from the Kenyan manufacturing sector for the period 1992–1999. The data were collected under the Regional Program on Enterprise Development (RPED) arranged by the World Bank, in collaboration with the Center for the study of African Economies (CSAE)Footnote1 at Oxford University. The dataset was downloaded from the CSAE website. It is a unique dataset and was used in the following studies (Granér and Isakkson, Citation2009; S. Esaku, Citation2019, Citation2020c). This dataset is unique in having measures of job flows that span across all firm sizes, most importantly; it captures the micro enterprises (employing between 0 and 9 workers). Moreover, the Kenya manufacturing sector offers a unique illustration of a progressive sector that grows at about 3.5% per annum (The World Bank, Citation2017). The dataset has key information, which relates to firm or enterprise information (firm Id, year of the survey, ownership status, value-added, capital-labor ration, material and other input costs, among others); and information on employees in each firm. To give a clear understanding of the dataset, we present the distribution of firms and their total employment in Table .
Table 1. The distribution of firms in the sample from 1992 to 1999
3.1. The characteristics and number of firms
Table presents the distribution of firms in the sample according to their size class and employment status, from 1992 to 1999. We can see that large firms (employing 100 or more workers) provide the bulk of employment. On average, these firms employ about 382 workers, followed by the medium firms (employing between 50 and 99 workers) who on average employ about 65 people. However, small firms (employing between 10 and 49 workers) and micro firms (employ from 0 to 9 workers) employ fewer workers; on average, these firms employ 25 and 4 workers, respectively.
3.2. The distribution of employment and firms according to firm age
In this section, we present the distribution of firms and employment according to firm size and age in Table . In panel (a), we report the percentage of firms according to size classification and age, while panel (b) gives the percentage of employment contribution by different firm sizes. We observe that micro firms form the bulk of firms in the entire sample (31.8%) but account for a relatively small share of employment (1.3%). Relative to micro firms, large firms are a small fraction but provide the bulk of employment. These firms are only 22.6% but account for 82% of all employment in the sample. The medium-size firms are fewer (15.3%) and provide 9.5% of employment, while small firms (30.3%), nearly reaching the number of micro firms, account for 7.2% of the employment. When analyzing the employment according to firm size and age categories, we note that old firms still provide the greatest proportion of employment. The results show that these firms contribute 58.8% of employment, followed by mature firms (age between 11 and 20 years), who account for 8.8% of employment, while very young firms (ages 0–5 years) and young firms (ages 6 − 10 years) account for 2.4% and 2.0% of employment, respectively.
Table 2. The distribution of firms according to employment and age
Turning to the distribution of firms according to their ages, we find that old firms form the bulk of the distribution. These firms dominate the sample because they are 44%, followed by mature firms, who form 32.3% of the sample, while young and very young firms are only 14.2% and 9.4 %, respectively. Overall, we also observe that old firms tend to be more uniformly distributed across the size categories than the very young firms.
4. The methodology
4.1. Introduction
In this section, we present the approach we follow in estimating the employment growth in the Kenyan manufacturing sector. Our methodology follows a standard approach in estimating rates of job creation, destruction and reallocation. We conduct the analysis in the following ways; first, we determine the employment growth rate and show how this rate is important as the measure of employment changes over time. We present the standard method of determining employment growth in Section 4.2. As shown by Haltiwanger et al. (Citation2013), the employment growth rate measure that we present in Section 4.2 is regarded as a standard measure in the estimation of employment changes. This measure has the useful properties of accounting for entry and exit rates among firms. The second step in the analysis is to estimate a set of equations that help us estimate gross job reallocation, job creation, job destruction and employment rate. We present this step in Section 4.3.
4.2. Determining employment growth
This section presents the various concepts and gives a summary of the framework that we use, which is in line with the existing literature, to evaluate employment changes. Extant literature on employment dynamics uses different classifications of firm size (see, Cho et al., Citation2017; Haltiwanger et al., Citation2013; Heyman et al., Citation2018; Klapper & Richmond, Citation2011; Lawless, Citation2014; Rijkers et al., Citation2014), making it difficult to adhere to one particular classification. Consequently, we follow Klapper and Richmond (Citation2011), with some modification, and classify firms into four size classes; (i) Micro firms – being firms that employ from 0 to 9 workers, (ii) Small firms – employing from 10 to 49 workers, (iii) Medium-size firms – employ from 50 to 99 workers, and (iv) Large firms-employ 100 or more workers. Additionally, we categorize firms according to firm age as follows: (i) Very young firms; being those from age 0–5 years, (ii) Young firms – aged from 6 to 10 years, (iii) Mature firms are those with ages ranging from 11 to 20 years, (iv) Old firms – aged 21 or more years.
To organize our analysis, we follow Davis and Haltiwanger (Citation1992) and Davis et al. (Citation1996) who define a measure of firm-level employment changes from the measures of job creation and job destruction. Following their procedure, job creation at period t is simply the aggregate of employment gains over all firms that expand (by creating more jobs or starting up new business) between periods t-1 and t. Similarly, job destruction at period t is defined as all employment losses summed up over all firms that contract (by layoffs of employees or shut down completely) between periods t-1 and t. Denote total employment of firm i in the current period t by and total employment in the previous period, t-1, as
. The difference between the two periods is the change in total employment and can be given as:
We can then determine gross job creation,, job destruction
, net job creation (NJRR), gross job reallocation rates (GJRR) and employment growth in period t. To accomplish this, we follow the analytical framework of Davis and Haltiwanger (Citation1992) which was further detailed in Davis et al. (Citation1996). We express job creation as:
And job destruction as:
Similarly, we express our measure of net job creation rate as:
And gross job reallocation rate as:
Additionally, we can also derive the rate of excess job reallocation, denoted by XJRRit, which is simply the difference between the gross job reallocation rates (GJRRit) and the net rates of job reallocation (NJRRit). Therefore, XJRRit measures the number of job adjustments in excess or above the number that is required to hold employment growth. Thus, the rate of excess job reallocation is given by:
Consequently, we express our measure of employment growth, denoted by, gi t, between period t-1 and t as:
where gi t is the employment growth, is the number of workers employed by the firm, i is the firm index. EquationEq. (7)
(7)
(7) denotes that the rate of employment growth is derived from a change in employment divided by average firm size between two periods; t-1 and t instead of using the initial employment size of the firm as was the practice in previous studies. This method is generally accepted in the literature on employment dynamics (see Davis et al., Citation1996). We observe that this approach yields a symmetric distribution of employment growth rates centered about zero and bounded by −2 and 2, to take care of entry and exit of firms. Relative to using this measure, employment growth rates derived using the initial year range between zero and infinity, and have been criticized for failing to adjust for entry and exit of firms.
4.3. Econometric framework of analyzing job flows
In this section, we present the econometric framework for estimating job creation and destruction, job reallocation and net employment growth. We extend our analysis to the estimation of an econometric model to evaluate the relevance of patterns in job flows. Consequently, we estimate the number of econometric models in which we take (gross job reallocation, job creation, job destruction and employment growth as the dependent variables) and regress each on a number of regressors. The generalized econometric model that we estimate is of the form:
where denotes the gross job reallocation rate in firm i at time t,
represents firm-level regressors lagged one period, while
denotes a composite error term with time (
) and industry (
) fixed effects and an error term (
). We include size dummies denoting the different size classes (small, medium and large). To avoid the dummy variable trap, we take micro firms as the reference group. The firm-level covariates that we include in term
include capital intensity, labor productivity, wage rate, age of the firm and ownership status. All these covariates are lagged one period to further control for any endogeneity. We also evaluate gross job creation and destruction by estimating a variant of Equationeq. (8
(8)
(8) ), with gross job creation and destruction as the dependent variable. Our basic models for estimating job creation and job destruction are of the following structure:
Similarly, we estimate growth in employment using the following equation:
The terms in the above expressions are defined as before.
Extant literature shows that employment growth rates vary with the observed firm characteristics like firm size, age, productivity, capital intensity, wage rate and ownership status. Specifically, Lawless (Citation2014) has shown that both the firm’s size and age are important components of the employment creation process. According to the author, employment growth and job creation rates exhibited systematic variation across firm size groups. Consequently, we include the size dummies and the firm’s age as explanatory variables to explain employment growth. Furthermore, research in international economics shows that firms that are large self-select into export markets employ more workers, pay higher wages and are capital intensive than firms with low productivity (see Bernard and Jensen, Citation2004; S. Esaku, Citation2019). As shown by Shiferaw and Bedi (Citation2013), controlling for the above observable firm characteristics is important in isolating the sources of variation in gross job reallocation rates (GJRR) and employment growth rates. Accordingly, we adopt the method of Shiferaw and Bedi (Citation2013), and include these observable firm characteristics as explanatory variables in our regression equations, (8), (9), (10) and (11). We also provide diagnostic tests for our results.
We estimate EquationEquations (8)(8)
(8) , (Equation9
(9)
(9) ), (Equation10
(10)
(10) ) and (Equation11
(11)
(11) ) in a regression framework, using ordinary least squares (OLS). Further, we also use a feasible generalized least squares (FGLS) method which generates efficient estimates in the existence of heteroscedastic and auto-correlated errors (Shiferaw & Bedi, Citation2013). As shown in the extant literature on employment growth, the use of FGLS estimator allows for variation in autocorrelation coefficient across firms and possesses good statistical properties (Shiferaw & Bedi, Citation2013). As a robustness check, we provide diagnostic tests for our estimation in Section 5.8.
To this end, we analyze job creation and destruction in the context of Sub-Saharan African, using Kenya as a case. We present our findings in Section 5 and conclude our discussion in Section 6.
5. Results
5.1. Annual rates of job creation, job destruction and job reallocation
In this section, we present the results of the empirical work on job creation, job destruction and job reallocation rates. Table shows the results of the analysis. Columns 1–6, denote year, annual rates of job creation, job destruction and job reallocation for periods 1992–1999. We can observe that there is positive net employment growth whenever rates of job creation are more than job destruction rates; which is reversed whenever rates of job destruction are greater than rates of job creation. The overall annual job creation rates fell from 23.46% in 1992/1993 to 8.89% in 1998/1999, while rates of job destruction fell from 22.96 to 17.28 during the same period. This shows that job creation rates fell by 14.57% (from 23.46% to 8.89%), faster than the decline in job destruction rates which only fell by 5.68% (from 22.96% to 17.28%). Consequently, job creation rates stood at 8.09%, averaging 10.4% while job destruction was at 9.66% which then averaged 12.42% between 1992 and 1999. Our findings seem to suggest that, for this period under consideration, job creation in the Kenyan manufacturing sector was not adequate to offset the rapid destruction of jobs, as can be observed from the marked drop in job creation rates, lower than the rates of job destruction. We note that these average figures from our calculations seem to mirror simultaneously pervasive rates of job creation and destruction in narrowly defined industries, in line with what was documented by previous studies (Davis & Haltiwanger, Citation1992). These results may suggest the existence of disturbances driving the restructuring of employment in the manufacturing sector that causes simultaneous movement in rates of job creation and destruction. Although outside the scope of this paper, we may suggest that these disturbances might reflect firm-level differences in the labor demand, since labor is one of the key inputs that facilitate the production process as shown by Davis and Haltiwanger (Citation1992).
Table 3. Annual rates of job creation, job destruction and job reallocation: Percent
In line with previous empirical studies, aggregating the job creation and job destruction rates gives us job reallocation rates. Our results indicate that gross job reallocation rates stand at 45.68% in 1992/1993, which subsequently dropped to 28.68% in 1998/1999; and averaged 22.65% over that period. These high rates of job reallocation seem to signal the reshuffling of job opportunities across firms, as this could be one key factor causing employees to change jobs or transition between employment and joblessness. Therefore, the average net employment growth declined by 1.75% as a result of high rates of job destruction, averaging about 2.24% during the period. We note that, although the manufacturing sector created jobs at an average of 10.4% per annum, this rate of job creation was not enough to counter rapid rates of job destruction that averaged 12.42% per annum. This means that the lack of enough jobs in the manufacturing sector in Kenya might be driven by inadequate job creation to counter rapid job destruction hence the increasing joblessness in the manufacturing sector. Throughout the sample period, we observe net employment growth only between the years 1994 and1995. The rest of the years recorded a decline in net employment, which averages 2.24%.
5.2. The contribution of firm size to job creation, job destruction and job reallocation
Table reports rates of job creation, destruction and reallocation by firm size using the average firm size as our measure of employment changes. For the period of analysis, we find that large firms accounted for most of the job creation (28.22%), accounting for 3.13% of net job creation. We also note that much of the job reallocations take place in large firms compared to other firm size. This implies that large firms are the primary source of most of the jobs that were created by the manufacturing sector. We also observe that micro firms accounted for the lowest rates of job creation (15.89%). Our findings suggest that it is the large firms who create the most jobs compared with small or micro firms. This result is in line with the observations noted in Kerr et al. (Citation2014). These authors conclude that large firms create jobs more than small- and medium-size firms. Turning to job destruction, our empirical work finds that these rates are highest in small firms (29.13%), followed by medium firms (26.04%) and large firms, with 25.09%; with micro firms destroying fewer jobs, 19.56%. This finding is in line with previous studies that show that although small firms create the most jobs, they also account for rapid job destruction compared to other firm sizes (Hijzen et al., Citation2010).
Table 4. Rates of job creation, job destruction and job reallocation by firm size: Percent
5.3. The contribution of firm age to job creation, job destruction and job reallocation
Table reports the rates of job creation, destruction and reallocation by firm age. In column 2, we observe that mature and young firms accounted for most of the job creation, 9.73%, and 8.54%, respectively. In column 3, we note that much of the destruction of jobs occurs in old firms (11.68%), followed by young firms (9.21%). Consequently, we note that much of the reallocation of jobs occurs in mature and old firms, (20.46%) and (20.01%), respectively. Our findings are in line with the work of Klapper and Richmond (Citation2011) who emphasize the important contribution of new firms (young) to job creation. These results show that new firms are indeed important in employment generation, a fact that is also confirmed by the seminal work of Lawless (Citation2014). These estimates indicate that the reshuffling of workers is higher among mature and older firms compared to other firm-size categories.
Table 5. Rates of job creation, job destruction and job reallocation by firm age: Percent
5.4. The contribution of firm size and age to job creation, job destruction and job reallocation
In this section, we report the results of the analysis on job creation, job destruction and job reallocation rates with regard to firm age and size. Our findings are presented in Table . We observe that rates of job creation are higher in firms that are young (6–10 years) and mature (11–20 years). When we consider young firms, we note that much of the net job creation occurred in small firms that are young, accounting for 11.48% of net job creation; while large firms in the same age group accounted for net job creation of 10%. In the mature age group, net job creation occurred in large firms, accounting for 11.58% of net job creation. The old firms contributed the least to net job creation. Throughout the sample period, these firms contributed negatively to net job creation. These results mirror the findings and conclusions reached by some studies that examined employment growth. For example, Cho et al. (Citation2017) find evidence that small and young firms account for much of the job creation rates in South Korea. Similarly, Ayyagari et al. (Citation2014) find similar results for developing countries. In their study, Ayyagari et al. (Citation2014) find that small firms accounted for a large share of jobs created. Our findings seem to agree with the above previous studies.
Table 6. Rates of job creation, job destruction and job reallocation by firm age and size: Percent
To conclude, our results reveal interesting findings; firstly, the rates of job creation in each age category are closely matched by almost similar rates of job destruction, save for old firms. Secondly, the rates of job creation and job destruction are more evenly distributed, and follow a similar pattern over the sample period, in line with the findings of Lawless (Citation2014). Consequently, the net job creation among young firms is remarkably higher while the rest of the firms recorded significant declines in net job creation, consistent with the findings of Lawless (Citation2014) for Irish firms. To note the most extreme example, the net job creation rate among older firms declined by 16.26% in small firms. Moreover, almost all of the net job reallocation rates are generated by the young firms (6–10 years) implying that the firm’s age shapes its contribution to aggregate job creation and job destruction rates. Consistent with previous studies, our findings reveal a significant correlation between the firm’s age and its size. Moreover, we emphasize that the job flows reported in this paper depict firm-level changes in employment growth that are pervasively high and persistent in all firms.
5.5. The contribution of firm size and age to gross job reallocation
In this section, we report estimates of the econometric model in Equationeq. (8)(8)
(8) that estimates the gross job reallocation rates among different firm classes. We report the findings in Table . We observe in model (1) that firm-specific effects account for about 55.4% of the explained variation in gross job reallocation in the manufacturing sector. When we remove time fixed effects, model (2), we observe that firm-specific effects do not change much as the explained variation in job reallocation is now at 55.1%. One remarkable finding to note is that job reallocation rises with the proportion of large firms and firms that are capital and labor-intensive. In line with the descriptive results presented earlier (Table ), large firms display job reallocation rates that are higher than the other firms. Further, we also observe from models (1) and (2) that firm age confirms that job reallocation rates fall with the firm’s age. However, the coefficients are not statistically different from zero. To consider the possibility of autocorrelation and heteroscedasticity in the analysis, we also use feasible generalized least squares technique. We report the results in columns 5 and 6, that is, models (3) and (4). We note that the findings are qualitatively and quantitatively similar, implying that OLS estimation did not induce any problems associated with the error term. Our findings are consistent with the results of Shiferaw and Bedi (Citation2013) who also find that 57% of variation in job reallocation was accounted for by industry-specific effects. We conclusively show that firm-specific determinants might be responsible for driving most of the inter-firm or cross-industry variations in job reallocation.
Table 7. The contribution of firm size and age to gross job reallocation
5.6. The contribution of firm size to employment growth
In this section, we report the results of the econometric estimation of Equationeq. (11(11)
(11) ), where we estimate the growth in employment. In column 1, model (a), we include time dummies in the regression, but remove them in model (b). We follow the same approach in models (c) and (d). In column 2, we can observe that firm-specific effects are important in employment growth. These effects account for 60.58% of the explained variation in employment growth in the sector. We also note that these effects are driven by large and medium firms. A closer examination of column 3 shows that large firms are the primary source of employment growth, as they account for 35.62% of growth in employment. They are closely followed by medium-sized firms that account for 34.1% of employment growth. Another important point to note is the importance of the firm’s age in shaping employment growth. As expected, firm age and employment growth have an inverse relationship implying that as the firm grows old, it loses its capability to generate employment. This finding is consistent with that in Haltiwanger et al. (Citation2013) and Lawless (Citation2014) who show that younger firms are more dynamic than older firms in growing employment. We argue that the more emphasized contribution of small firms to employment growth may not generally hold. It might hold for economies with enabling business environments and opportunities for firm growth. However, in developing and other emerging countries, the contribution of large firms to employment growth is still remarkable as was recently shown by Kerr et al. (Citation2014). Columns (4) and (5) report coefficient when FGLS technique is used. We note that our results are qualitatively and quantitatively similar to OLS estimates.
5.7. The contribution of firm size to job creation (JC)
In this section, we present the results of the estimation of EquationEq. (9)(9)
(9) in Table . We are interested in examining how firm-specific effects shape job creation in the manufacturing sector. Column 2 of Table indicates that firm-specific effects contribute about 25.6% of the total variation in job creation. We observe that job creation increases with the proportion of small firms than with other firm sizes. However, in Table we noted that it is not firm size per se that generates job creation but rather firm age, consistent with the findings in Lawless (Citation2014). It is probable that the contribution of small firms might be from the young firms as earlier on noted in Table . The main insight we highlight in Table is that firm-specific determinants contribute the bulk of inter-firm differences in job creation, consistent with findings of Cho et al. (Citation2017) and Shiferaw and Bedi (Citation2013). These determinants might be differences in the skills-set, technology and other firm-level variables that influence job creation. Our analysis falls short of examining these determinants due to data limitations.
Table 8. The contribution of firm size to employment growth
Table 9. The contribution of firm size to Job creation
5.8. The contribution of firm size to job destruction (JD)
In this section, we present the results of the estimation of EquationEq. (10)(10)
(10) in Table . In column 2, we can see that the magnitude of firm-specific effects is now higher (77.6%), compared to the job creation. We note that medium and large firms account for most of the variation in firm-specific effects, with medium firms contributing 29.48% and large firms 28.71%. We highlight that these firms are also responsible for most of the variations in job destruction rates. Similarly, we note that job destruction decreases with the fraction of firms that are capital and labor-intensive. This implies that job destruction falls with capital and labor intensity in a non-linear manner. The main message of Table seems to suggest that firms with high potential of creating more jobs also tend to destroy more jobs. We note that there is simultaneous job creation and job destruction pattern in our sample, implying that even when the manufacturing sector is able to create jobs in rates that are disproportionately higher these jobs are off-set by equally high rates of job destruction. Our findings seem to agree with the work of Shiferaw and Bedi (Citation2013) who find the sluggish employment growth in Ethiopian manufacturing is due to simultaneous job creation and job destruction processes acting in opposite direction. Our results generally seem to mirror the same conclusions reached by the work of Shiferaw and Bedi (Citation2013).
Table 10. The contribution of firm size to job destruction
5.9. Diagnostic tests for empirical results
In this section, we present the diagnostic tests for our findings to ensure the validity of our estimation methods. We observe that the correlation matrix reported in Tables and 1 shows that our variables have significant coefficients, significant at 5% level. We note that the variance influence factor confirms the validity and robustness of our findings. Further, the conditional index shows that the estimation results are stable over the study period, as shown in Tables and 1.
Table 11. Correlation matrix for the main variables
Table 12. Correlation matrix for the main variables
Table 13. Collinearity diagnostics
Table 14. Additional collinearity diagnostics
6. Conclusion
In this paper, we presented a detailed examination of the nature of gross job flows, with a special focus on gross job creation and gross job destruction, taking the Kenyan manufacturing sector as a case. The empirical analysis reports key findings. First, we report the estimates of job creation, job destruction and job reallocation rates in the Kenyan manufacturing sector between 1992 and 1999; noting that there is an increase in employment level whenever job creation rates exceed job destruction rates and vice versa. Our empirical work shows remarkable findings: first, we find that job creation in the manufacturing sector is simultaneously followed by job destruction of the jobs created. In most cases, job creation in the manufacturing sector was not adequate to offset the rapid destruction of jobs, as can be observed from the marked drop in job creation rates, lower than the rates of job destruction. These rates of job destruction are pervasively high and reflect the shuffling of jobs in the manufacturing sector.
Second, we find that the presence of simultaneously pervasive rates of job creation and job destruction cuts across firm size and age. We note that, for the whole sample, large firms account for high rates of job reallocation, job creation and job destruction. We however find that job creation rates in each firm and age category are closely matched by almost similar rates of job destruction, and that job creation and job destruction rates are more evenly distributed, and follow a similar pattern across the sample period. Third, we find that young firms account for almost all of the net job creation rates implying that the firm’s age is important in shaping its contribution to net job creation. In agreement with previous studies, our findings reveal that there is a significant correlation between the firm’s age and its size in shaping net job creation.
Our findings have practical implications. First, job creation policies alone without policies that mitigate the destruction of jobs created might not be successful. For job creation to be successful in the manufacturing sector, effort should be made in creating strategies that mitigate the destruction of jobs created. This is because job creation and job destruction rates simultaneously follow each other. Second, to expand employment growth, effort should be dedicated to supporting young firms (6–10 years), through possibly, tax relief, technology of production or any possible government subsidy that can help these firms grow and survive in the turbulent business environment. Finally, one limitation of our study is that the data we used are a bit old. It would be important to study the above relationships using recent firm-level data that are longitudinal in nature. Additionally, future research could be directed at developing and testing theories that drive job creation, job destruction and reallocation in the Sub-Saharan African context.
Additional information
Funding
Notes on contributors
Stephen Esaku
Stephen Esaku is adjunct faculty at Kyambogo University (Soroti Campus) in Uganda, where he lectures courses in Microeconomics. His research interests are in the areas of international economics (firms in international trade, regional integration, foreign direct investment), development economics (focusing on economic growth and development, poverty dynamics, corruption and gender studies) and empirical industrial economics (learning, pricing and market strategies). Stephen has published papers on firm-level investment and Exporting; Export markets and productivity; Trade liberalization and productivity growth in Sub-Saharan Africa, among others.
Notes
1. We thank the Center for the study of African Economies for making the data available for download for free to researchers. We are grateful to you for this assistance without which, it would not have been possible to conduct this study.
References
- Ayyagari, M., Demirguc-Kunt, A., & Maksimovic, V. (2014). Who creates jobs in developing countries? Small Business Economics, 43(1), 75–22. https://doi.org/10.1007/s11187-014-9549-5
- Baldwin, T. D., Dunne, T., & Haltiwanger, J., & J. H.. (1998). A comparison of job creation and job destruction in Canada and the United States. The Review of Economics and Statistics, 80(3), 347–356. https://doi.org/10.1162/003465398557528
- Bernard, A. B., & Jensen, J. B. (2004). Why Some Firms Export. The Review of Economics and Statistics, 86(2), 561-569. https://doi.org/10.1162/003465304323031111
- Bigsten, A., & Gebreeyesus, M. (2007). The small, the young, and the productive: Determinants of manufacturing firm growth in Ethiopia. Economic Development and Cultural Change, 55(4), 813–840. https://doi.org/10.1086/516767
- Binetti, M., & Coniglio, N. D. (2019). Foreign firms in core or peripheral areas? The spatial dimension of job creation and wages in Sub-Saharan Africa. Papers in Regional Science Journal, 98(6), 2233–2250. https://doi.org/10.1111/pirs.12466
- Birch, D. L. (1981). Who creates jobs? The Public Interest, 65(Fall), 12. https://doi.org/10.1016/S0165-1765(02)00043-5
- Chege, S. M., & Wang, D. P. (2020). Information technology innovation and its impact on job creation by SMEs in developing countries: An analysis of the literature review. Technology Analysis and Strategic Management Journal, 32(3), 256–271. https://doi.org/10.1080/09537325.2019.1651263
- Cho, J., Chun, H., Kim, H., & Lee, Y. (2017). Job creation and destruction: New evidence on the role of small versus young firms in Korea. The Japanese Economic Review, 68(2), 173–187. https://doi.org/10.1111/jere.12133
- Collier, P., & Gunning, J. A. N. W. (1999). Explaining African economic performance. Journal of Economic Literature, XXXVII(1), 64–111. https://doi.org/10.1257/jel.37.1.64
- Davis, S. J., & Haltiwanger, J. (1992). Gross job creation, gross job destruction, and employment reallocation. The Quarterly Journal of Economics, 107(3), 819–863. https://doi.org/10.2307/2118365
- Davis, S. J., Haltiwanger, J., & Schuh, S. (1996). Job creation and job destruction. Cambridge: USAMIT Press.
- Esaku, S. (2019). ‘Export markets and firm productivity in Sub-Saharan Africa. Journal of African Business, 1–20. https://doi.org/10.1080/15228916.2019.1695190
- Esaku, S. (2020a). ‘Does firm size affect learning-by-exporting? Empirical evidence from Sub-Saharan Africa’. Cogent Economics and Finance, 8(1), 1754150. https://doi.org/10.1080/23322039.2020.1754150
- Esaku, S. (2020b, April). ‘Investments, export entry and export intensity in small manufacturing firms’. Journal of Industrial and Business Economics, 2020, 1–23. https://doi.org/10.1007/s40812-020-00156-9
- Esaku, S. (2020c). ‘Trade liberalization and productivity growth: A firm-level analysis from Kenya’. Review of Economic Analysis, Forthcoming, 12(2), 1-21. https://openjournals.uwaterloo.ca/index.php/rofea/article/view/1791/2086
- Esaku, S., & Krugell, W. (2020). ‘Firm-level investment and exporting: New empirical evidence from Ghana and Tanzania’. International Economic Journal, 34(1), 125–143. https://doi.org/10.1080/10168737.2019.1663440
- Granér, M., & Isaksson, A. (2009). ‘Firm efficiency and the destination of exports: Evidence from Kenyan plant-level data”. The Developing Economies, 47(3), 279–306. https://doi.org/10.1111/j.1746-1049.2009.00087.x
- Haltiwanger, J., Jarmin, R. S., & Miranda, J. (2013). Who creates jobs? Small versus large versus young. The Review of Economics and Statistics, XCV(95(2)), 47–361. https://doi.org/10.1162/REST_a_00288
- Haltiwanger, J., Scarpetta, S., & Schweiger, H. (2008) ‘Assessing job flows across countries: The role of industry, firm size and regulations’, NBER Working Paper Series, (2450). https://doi.org/10.1017/CBO9781107415324.004.
- Heyman, F., Norbäck, P. J., & Persson, L. (2018). ‘Who creates jobs and who creates productivity? Small versus large versus young versus old. Economics Letters, 164, 50–57. Elsevier B.V.. https://doi.org/10.1016/j.econlet.2017.12.034
- Hijzen, A., Upward, R., & Wright, P. W. (2010). Job creation, job destruction and the role of small firms: Firm-level evidence for the UK. Oxford Bulletin of Economics and Statistics, 72(5), 621–647. https://doi.org/10.1111/j.1468-0084.2010.00584.x
- ILO. (2014). Global employment trends 2014: Risk of a jobless recovery? Geneva: International Labor Organization. http://www.ilo.org/wcmsp5/groups/public/—dgreports/—dcomm/—publ/documents/publication/wcms_233953.pdf
- Kaldo, N. (1960). Causes of growth and stagnation in the world economy. Cambridge University Press.
- Kerr, A., Wittenberg, M., & Arrow, J. (2014). Job creation and destruction in South Africa. South African Journal of Economics, 82(1), 1–18. https://doi.org/10.1111/saje.12031
- Klapper, L., & Richmond, C. (2011). ‘Patterns of business creation, survival and growth: Evidence from Africa. Labour Economics, 18(SUPPL. 1), S32–S44. Elsevier B.V.. https://doi.org/10.1016/j.labeco.2011.09.004
- Lawless, M. (2014). ‘Age or size? Contribution to job creation. Small Business Economics, 42(4), 815–830. https://doi.org/10.1007/s11187-013-9513-9
- Neumark, D., Wall, B., & Zhang, J. (2011). New evidence for the United States from the Review of Economics and Statistics, 93(February), 16–29. https://doi.org/10.1162/rest_a_00060
- Rijkers, B., Arouri,H., Freund, C., & Nucifora,A. (2014). ‘Which firms create the most jobs in developing countries? Evidence from Tunisia’, Labour Economics. Elsevier B.V., 31, 102–840. https://doi.org/10.1016/j.labeco.2014.10.003
- Rodrik, D. (2018). An African growth miracle? Journal of African Economies, 27(1), 10–27. https://doi.org/10.1093/jae/ejw027
- Shiferaw, A., & Bedi, A. S. (2013). The dynamics of job creation and job destruction in an African economy: Evidence from Ethiopia. Journal of African Economies, 22(5), 651–692. https://doi.org/10.1093/jae/ejt006
- The World Bank. (2017). World development indicators’. Washington, D.C.
- Yazdanfar, D., & Ohman, P. (2018). ‘Growth and job creation at the firm level: Swedish SME data’. Management Research Review, 41(3), 345–358. https://doi.org/10.1108/MRR-03-2017-0093
Appendix A
Table A1. Description of the key variables.