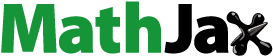
Abstract
The relationship between crime and unemployment has a long history in social science and remains a central point of debate for politicians today. In this paper, the objective is to establish whether youth unemployment has a causal effect on murder cases in KwaZulu-Natal; a province in South Africa facing high levels of both and the contribution is methodological. In particular, this paper pioneers the application of a control function procedure in testing and controlling idiosyncratic endogeneity within a count data framework in a bid to isolate the exogenous effect of youth unemployment on murder crimes. Using local municipality-level panel data observed between 2006 and 2017 and holding constant standard control variables, youth unemployment is found to be exogenous to omitted time-varying correlates suggesting that classical count data models with entity-fixed effects suffice. Also confirmed is that the control function approach reports results that are similar to the classical Poisson estimator while the Negative Binomial alternative tends to underestimate the effect of youth unemployment on crime by about 10%. Consistent with the majority of studies in literature, the analysis finds a positive and sizeable effect of youth unemployment on murder offences. A percentage point increase in youth unemployment increases the odds of murder occurrence by 1.6–1.8 times. This suggests that South Africa’s labour market could be linked to murder crimes in KwaZulu-Natal and that a social policy aimed at creating jobs for young people can be an alternative way of combating murder crimes in the province.
PUBLIC INTEREST STATEMENT
Violent crimes are endemic in South Africa as in most parts of the world. Worrisomely, it is reported that 58 people are murdered each day, at a rate of 35.8 murders per 100,000 people. Against this background, the South African Police Service (SAPS) cites three main causes namely criminal behaviour where murders happen as a result of other crimes and criminal activity; group behaviour, where gangs murder each other, taxi violence erupts, or mobs seek out vigilante justice; and social behaviour, where social ills (alcohol and drug abuse), dysfunctional relationships, unemployment and other problems lead to deaths. Evidence presented in this paper finds youth unemployment as another relevant cause of murder crimes in KwaZulu-Natal province. This means that job creation for young people can complement efforts to curb murder crimes in KwaZulu-Natal province of South Africa.
1. Introduction
Does a deteriorating labour market breed crime? Does having a legitimate job reduce one’s willingness to offend? These generic questions are common across the developing world and it is standard for practitioners to first consult theory for guidance. In the main, theory presents three conflicting views. The first view raised by criminologists is that unemployment correlates negatively with crime. Their argument is that joblessness means less crime victims and fewer goods to steal (Felson & Cohen, Citation1980; Cantor & Land, Citation1985, Citation2001,; Land et al., Citation1995). In other words, the number of people whom criminals can steal from declines as unemployment increases so crime is viewed as a negative function of unemployment. They also argue that unemployment is an extra security factor as unemployed individuals spend more time indoors safely and looking after their property.
The second explanation proposed by Merton’s (Citation1938) strain theory is that failure to achieve material success (commonly represented by the lack of jobs) can frustrate those ranking low in the social structure due to economic deprivation which consequently and potentially breeds retaliatory crime. Put differently, the failure of individuals to secure a legitimate job can breed colossal anger especially when the marginalized group find themselves positioned in the middle of successful individuals. This frustration in turn potentially serves as a justification for the economically marginalized individuals to commit criminal offences.
Contrary to the above two propositions, economists starting from Becker (Citation1968) and Ehrlich (Citation1975) advance a completely different notion which is that individuals make rational decisions based on a cost and benefit analysis of committing crime. Their argument rests on the idea that individuals choose to commit crime whenever the perceived benefits of committing crime outweigh the costs. In addition to that and more importantly, economists stress that unemployment implies the lack of a legitimate job on the labour market and not having a legitimate job lowers the opportunity cost of committing crime which consequently motivates individuals to engage in criminal activities. Therefore, unlike sociologists, economists believe that unemployment correlates positively with crime.
At the empirical quantitative level, it is now over five decades of empirical research since the seminal work of Becker (Citation1968) but the question of how unemployment correlates with criminal activities remains unresolved. One strand of literature finds the association positive and strong (Costantini et al., Citation2018; Ha & Andresen, Citation2017; Nordin & Almén, Citation2017; Tshabalala, Citation2014; Zulkifli et al., Citation2016) while the other strand (Öster & Agell, Citation2007; Lin, Citation2008; Rege et al., Citation2009; Blomquist & Westerlund, Citation2014; Bhorat et al., Citation2017) finds the association either negative, modest or miniscule. Apart from the inconclusive theory, what else could possibly explain this stark empirical dissonance?
A leading suspect in linear panel data applications is endogeneity emanating from three potential sources namely 1) simultaneity as one’s criminal record has a bearing on employment prospects, 2) unobserved time-invariant heterogeneity (herein termed heterogeneity endogeneity) and 3) idiosyncratic endogeneity stemming from omitted time-varying factors. A standard practice of dealing with simultaneity in the crime specification is to use lags of the unemployment rate which allows one to establish how contemporaneous changes in unemployment influences crime in subsequent periods. Unobserved heterogeneity on the other hand is commonly and easily dealt with by inclusion of N-1 dummies where N is the total number of cross sections in the panel. Lastly, Instrumental Variable regressions (IVrs) and the Two Stage Least Squares (2SLS) are common approaches of solving the correlation between the unemployment rate and the idiosyncratic term. Crime data are however best modelled with count data techniques which are non-linear models and until recently, addressing idiosyncratic endogeneity in count data methods has not been straightforward.
Within the crime and unemployment literature, this paper pioneers the application of a Control Function (CF) approach recently proposed by Lin and Wooldridge (Citation2019) which addresses idiosyncratic endogeneity within a count data framework. This CF approach is a modified version of that introduced and discussed extensively in Papke and Wooldridge (Citation2008) and it simultaneously circumvents both heterogeneity and idiosyncratic endogeneity. We compare results from the CF approach with those from the classical Poisson and Negative Binomial regressions for robustness purposes. This allows us to assess the importance of controlling potential idiosyncratic endogeneity which is likely to be inherent given the challenge of controlling all time-varying factors that possibly influence crime.
The empirical application in this paper relies on a novel local municipality-level panel dataset provided by Quantec which reports socio-economic data on South Africa’s local municipalities. Unlike most countries, South Africa presents a unique fertile ground for examining the relationship between unemployment and crime as it is characterised by high levels of both. Despite this characteristic, however, only Demombynes and Özler (Citation2002), Verrinder (Citation2013), Tshabalala (Citation2014), and Jonck et al. (Citation2015) and more recently Bhorat et al. (Citation2017) have focused exclusively on South Africa within the ample evidence available (Burger and Woolard, Citation2005; Öster & Agell, Citation2007; Lin, Citation2008; Rege et al., Citation2009, Blomquist and Westerlund, Citation2014; Zulkifli et al., Citation2016; Nordin & Almén, Citation2017; Ha & Andresen, Citation2017; Costantini et al., Citation2018). Different from these studies, the present analysis is limited to youth unemployment in the context of KwaZulu-Natal which is one of South Africa’s high crime provinces.
Focusing on youth unemployment is important for two reasons. Firstly, it is often higher than adult unemployment since young people possess little to no working experience leaving them more vulnerable to economic hardships than adults. Bhorat et al. (Citation2017), for example, argue that unemployment has affected all age groups in South Africa, but youths have been affected the most. From an overall unemployment rate of 27% in 2016, youth unemployment was recorded at 55% while adult unemployment was less than 30% (Statistics South Africa, Citation2016). The second reason is that the marginal propensity to commit crime is thought to be high among youths than adults (see Lochner, Citation2004). For South Africa in media and political circles, there is a growing belief that young people without jobs are largely responsible for violent crimes. It is important however for economists to step back and ask the question, is this claim backed by hard evidence?
In a bid to answer the above question, the remainder of the paper is organised as follows: section 2 presents the theoretical framework followed by data description and the empirical strategy in section 3. Results and are then presented and discussed in the penultimate section of the paper before concluding remarks.
2. Theoretical framework
The empirical analysis of this study is grounded in the theoretical framework proposed by Becker (Citation1968). The model assumes, for simplicity, that criminals make a choice between economic crimes and legitimate jobs which rules out the possibility of combining both activities. An individual seeking to maximise utility would commit a crime if the expected utility derived from committing an offense exceeds the utility that the individual would attain from the next best alternative form of employment (opportunity cost of time spent on criminal activities).
where represents the expected utility derived from criminal activities and
is the expected utility derived from a legitimate job. A criminal in this model, therefore, differs from a non-criminal not because of psychological factors but rather due to the perceived benefits and costs of committing crime.
Importantly, one’s expected utility from committing offenses is a function of the expected costs and benefits involved as well as the degree of risk aversion. If an individual chooses to commit crime, there is a probability of being caught such that the probability of not being caught can be expressed as
.
is therefore a weighted probability of these two possibilities.
where is the monetary benefit of committing crime, which is essentially equivalent to the market value of the stolen goods. Parameter
is the cost of being caught which typically comprises fines, paying for a bail out, being in jail, damage of future employment prospects, damage to one’s social reputation and so on.
in this model reflects the effort and psychic cost of committing crime. Carefully planned and well-orchestrated murder for example, take much work, effort, and time while petty offense such as stock theft may be committed only when an opportunity arises.
According to the model, one’s expected utility increases with the probability of being caught , the cost or penalty of being caught,
. Put differently in other words, the expected utility of committing crime decreases when the probability of being caught increases (i.e. when there is an improvement in policing for example) and when the cost of being caught increases (i.e. the cost of bail, the number of years behind bars, the cost of fines etc.).
While criminal benefits or the monetary gain of committing crime are fixed for any criminal activity, the corresponding cost is not. In particular, the opportunity cost of committing crime is high for an employed individual than it is for an unemployed individual. An employed lecturer for example, loses a lot from planning to murder someone for a price as his or her limited time must be spent either on planning criminal activities or lecturing. Similarly, the cost of effort differs as an unemployed individual has more time to spend on criminal activities than an employed individual.
The model also considers repeat offenders. Given the offenders’ utility choices, it may be optimal to re-offend. Legitimate income opportunities of individual who gets convicted may become fewer relative to illegitimate opportunities owing to the tarnished criminal record coupled with the impact of long imprisonment. As is commonly understood, once an individual is labelled a criminal, further imprisonment poses little harm to a reputation that is already tarnished.
An individual’s expected utility from a legal job, , on the other hand is less risky than criminal activities but not always certain. It is assumed that individuals do not know whether they will be employed or not at the beginning of the period. In addition, there are two possibilities if the individual chooses to participate in a legal activity and
is a weighted probability of the two possibilities as follows.
In this case, denotes the wage from a legal job. Given the high possibility that most criminals are likely less productive and unskilled,
is expected to be lower than the average wage in the legitimate labour market. Parameter
represents the probability of an individual being unemployed,
signals unemployment benefits and
captures the effort put into work. Furthermore, the minimum wage in this model is deemed to be higher than the unemployment benefits
. Despite the cost of effort arising from legitimate work
,
.
An increase in the probability of being unemployed reduces the expected utility derived from legitimate jobs. An individual will therefore engage in criminal activities if,
As this study will rely on municipality level data, we need to derive a specification of aggregate crime supply for a municipality. An individual will choose to participate in a crime during the time under consideration if Equationequation (7)(7)
(7) is satisfied. From this equation and condition, a behavioural function linking the engagement in a criminal activity, O, with its determinants can be formulated.
The sign beneath each right hand-side variable reflects the expected effect on crime. The total supply of crime here is essentially an aggregation of individuals’ participation in criminal activities. As the right hand-side variables will likely have distinctive effects across individuals, the intuition here is that a standard effect holds, and this effect is expected to hold for most of the individuals so that the overall effect reflects in a change in aggregate supply of crime. For example, a higher probability of being caught proxied by improved policing suggests the condition of choosing to participate in a criminal activity will hold only for less individuals which decreases the aggregate supply of crime. A higher unemployment rate reduces the expected utility derived from legitimate jobs hence the model predicts a positive relationship between the rate of unemployment and crime.
3. Data and empirical strategy
The analysis exploited a novel local municipality-level panel dataset provided by QuantecFootnote1 which reports socio-economic data on South Africa’s 226 local municipalities. It focuses on a specific area, KwaZulu-Natal (KZN, hereafter); a province where violent crimes are highly prevalent. The province comprises 50 local municipalities which are all included in the analysis. In terms of variable measurement, one of the challenges confronting every empirical study working on crime is the fact that crime data are often measured with error due to underreporting which makes the observed data prone to an underestimation of the true levels of crime. Some crime victims deliberately choose not to report crimes due to the lack of trust in police or due to the fear of victimization. Technically therefore, the accuracy of crime data largely depends on the willingness of communities to report crime cases, the effectiveness of police and the willingness of police themselves to record all reported cases. Given this inherent challenge, the analysis was restricted to murder cases, which unlike other forms of violent crimes (burglary at residential premises, rape; robbery with aggravating circumstances, common assault and so on) are less likely to suffer from underreporting.
In terms of control variables, data on socio economic indicators are reported from 1995 to 2017 but crime data supplied to Quantec by SAPS are only available starting from 2006. As a result, the analysis was restricted to the 2006–2017 sampling period which when combined with the cross-sectional dimension of 50 local municipalities produced a panel dataset of 600 observations, i.e. T = 12 and N = 50. Narayan and Smyth (Citation2004), Blomquist and Westerlund (Citation2014) and more recently Costantini et al. (Citation2018) invoked cointegrating techniques but since here the time dimension is not large enough to warrant any meaningful long-run inference, the issue of possible cointegration was not pursued.
An empirical caveat gleaned from earlier studies is that the unemployment–crime relationship should ideally be analysed at individual level given the nature of related theory. From an observational perspective, this would require one to; for example, check the employment status of criminals in order to determine whether offenders are individuals who are out of the legitimate labour market. Alternatively from an experimental perspective, one would have to randomly assign employment status to selected individuals i.e. give jobs to some individuals as the control group while others remain unemployed as the treatment group so that statistical inference can be made on whether the latter group has a higher chance of committing offences. Although the experimental approach is appealing, it is inappropriate on ethical grounds as it is highly unacceptable to randomly declare people unemployed. The observational approach on the other hand is impeded by the fact that police rarely disclose personal information of offenders and therefore assessing the employment status of criminals is quite a formidable task.
Owing to the above challenges, the majority of studies have resorted to the second-best strategy of using cross sectional, time series and panel data approaches in which the aggregated number of criminal offences is regressed against the rate of unemployment. In this direction, this analysis preferred a panel data approach over time series and cross-sectional approaches mainly because of its ability to deal with heterogeneity across cross sectional entities.
The empirical analysis of this study is grounded in both the social disorganization theory and Becker’s (Citation1968) theoretical framework although the empirical specification is to a larger informed by the latter. In the former, economically marginalized group of the society get frustrated and commit crimes as retaliation while Becker (Citation1968) views crime as a reflection of economic decisions and rational actions of individuals outside the legitimate labour market. The use of these two theoretical frameworks is based on the consideration that murder, in most cases, largely reflects anger and social frustration on the part of the offender at best but can also be an outcome of economic decisions when the murderer is hired by a third party. The later argument therefore implies that murder can itself represent a profession which reflects rational actions of unemployed individuals thriving to survive from criminal activities. There are other instances where offenders initially intend to commit non-life taking crimes i.e. car hijacking or bank robbery which are clearly motivated by economic gains but eventually commit murder mostly when victims fail to cooperate.
The dependent variable is the number of murder cases per 100,000 inhabitants which is characterised by discrete non-negative integers. Given this nature of the dependent variable, conventional methods such as the OLS technique may be inappropriate (Rufrancos et al., Citation2013) as the assumption of residual normality is not guaranteed (Chib & Winkelmann, Citation2001; Kelly, Citation2000; Osgood, Citation2000). Visually, a look at Figure clearly shows that application of the pooled OLS is inappropriate as crime data are heavily skewed towards the right.
In addition to heavy right skewness, crime data are characterised by non-negative integer values and the OLS will be inappropriate since there is likely to be a combination of that is negative as displayed in Figure (Cameron Colin & Trivedi, Citation1998). Under these circumstances, a natural starting point is a Poisson-based regression (Hausman et al., Citation1984., Cameron Colin & Trivedi, Citation1998). Intuitively, a Poisson regression models the log of the expected count which averts the problem of negative-predicted values since negative values correspond to expected counts between 0 and 1. In its basic form, the Poisson panel regression takes the following form:
where
with subscripts and
representing the local municipality and year, respectively,
is the number of murder crimes and
is a vector of explanatory variables. The common loglinear specification is considered, and it is given by:
Here serves to absorb unobservable individual-specific effects (which represent heterogeneity endogeneity) and
captures common crime trends and time dependent shocks. The main argument here is that the unemployment rate embedded in vector
can be correlated with both unobserved heterogeneity (
) and the idiosyncratic error (
) which would lead to heterogeneity endogeneity and idiosyncratic endogeneity, respectively, both which cause inconsistency and a serious bias on
(Baltagi, Citation1995). Geographical location is an immediate example of the former as it is time-invariant, heterogeneous, and possibly correlated with unemployment. Youth unemployment might be low in coastal municipalities, for example, because the majority of young people are employed on sea ports as compared to inland and underdeveloped municipalities but the same coastal municipalities might present a perfect environment conducive for crime i.e. through facilitating the smuggling of goods by the sea.
On the other hand, the assumption of strict exogeneity on youth unemployment can be quite strong even when one controls time-invariant heterogeneity. This is because one hardly picks out all time-varying factors from the idiosyncratic error that can possibly affect both crime and the unemployment rate. Dealing with this kind of endogeneity is straightforward in linear regression methods but considerably less so in non-linear regression methods. Within a Poisson cross sectional regression framework, the Generalised Method of Moments (GMM) can be applied with appropriate instruments as discussed in and Wooldridge (Citation2010). This approach is problematic however and mulled by scepticism in panel data Poisson regression given two additional challenges that panel data brings; unobserved heterogeneity and period effects often controlled by N-1 and T-1 dummies, respectively, both which have a tendency of violating order conditions as the structural equation is likely to have more parameters than instruments.
Given the above challenge, the analysis follows a CF procedure of Papke and Wooldridge (Citation2008) recently modified by Lin and Wooldridge (2018), (the LW procedure, hereafter). The LW procedure essentially proceeds in stages. Firstly in this context, the idea is to estimate the expected number of murder crimes conditioned on an endogenous youth unemployment rate (), exogenous variables (
), municipality-specific effects i.e. unobserved heterogeneity (
) and time-varying omitted factors (
). This can be represented by the following expressions.
where,
Vector also includes
time dummies denoted by
in Equationequation (2)
(2)
(2) . The first step estimates the reduced form equation for the endogenous regressor (
) by the fixed effects (FE) approach and obtain the FE residuals. In the reduced form equation, we need valid instruments which should typically be correlated with unemployment but uncorrelated with murder crimes. Here we rely on three instruments, two of which are proposed by Öster and Agell (Citation2007) and one is our own proposal.
With respect to the other two instruments adopted from Öster and Agell (Citation2007), the first instrument is essentially an interaction of initial employment composition at the municipality-level with the national trend in industrial growth to construct measures of the change in labour demand in different municipalities. For illustrative purposes, let the aggregate growth rate in industry between time
and time
be
where denotes employment at time
in industry
. Unemployment in municipality
is then instrumented by the interaction of the national growth rates and the lagged municipality-specific composition of industrial employment to formulate the first instrument (inst1).
The second instrument (inst2) is an interaction of the economy-wide trade-weighted real exchange rate and the municipality-specific manufacturing employment. This is given by
where Manuf1995 represents the share of manufacturing employment in 1994 for each municipality in the sample and is the lagged real effective exchange rate. The third instrument (inst3) is Chinese imports which are widely reported to have had a significant influence on local jobs in South Africa in the past two decades (see Edwards & Jenkins, Citation2015). Imports have an effect of taking jobs, including those that could easily accommodate young people and it is hard to think of a case where Chinese imports, i.e. garments, shoes, toys and so on, would directly increase murder crimes in uMhlathuze, Hlabisa, Ntambanana or Mtubatuba local municipality. Data for Chinese imports (in volumes) are sourced from South Africa’s department of trade and industry (DTI) while data on manufacturing employment both at national level and local municipality are sourced from Quantec.
These three instruments are included in the first step regression along with fixed effects and other control variables that appear in the structural equation (described shortly). The intuition here is that Chinese imports and municipality share of manufacturing employment embedded in become strictly exogenous once we control for local municipality-specific effects which is plausible. FE residuals are then computed as,
where the hat denotes predicted values and the two upper dots signal time averages i.e.,
and, in the second stage, plugged in the FE Poisson regression mean specification (with bootstrapped standard errors) given by,
in which robust Wald test of will be a test for exogeneity with respect to idiosyncratic shocks. The strategy applied here is to estimate three separate regressions. The first regression variant represents a classical FE Poisson regression mean specification which accounts for heterogeneity endogeneity and disregards idiosyncratic endogeneity. The second specification is essentially the LW procedure described above which accommodates idiosyncratic endogeneity. The third specification is a FE Negative Binomial regression. Note that a customary practice in count data techniques requires checking validity of the equidispersion (i.e. mean = variance) assumption. In most cases, rejection of this assumption is taken as evidence in favour of fixed-effects Negative Binomial (NB) regression over a Poisson regression (NB) (Chin & Quddus, Citation2003; Lord, Citation2006). However, we are here interested in the conditional mean;
and in this case a Poisson regression with robust standard errors is more consistent over a NB regression even in the presence of under-or-overdispersion (Wooldridge (Citation2010). A NB regression requires that the NB distribution is correct and there is independence over time conditional on the unobserved effect and covariates; an assumption which is very strong and likely violated.
The objective was to establish an empirical link between unemployment embedded in vector and the expected number of murder crimes. South Africa defines youth unemployment broadly as encompassing the 15–34 years age group which is not comparable across countries. To ensure comparability with other previous international studies, we follow the International Labour Organization’s (ILO) definition of youth unemployment which is limited to the 15–24 years unemployed age group. Given the inconclusive theory and mixed results in previous studies the relationship between youth unemployment and crime is expected to take either sign.
Controlling for other possible drivers of crime is important in order to successfully disentangle the impact of youth unemployment. Based on previous studies (Gould et al., Citation2002; Raphael & Winter-Ebmer, Citation2001), we include gender and racial composition (Zulkifli et al., Citation2016), income per capita (Rufrancos et al., Citation2013) and a police variable (Lim et al., Citation2010). As in Lim et al. (Citation2010), the police variable in this study is expenditure on policing and it attempts to capture the probability of being caught. An increase in the probability of being caught discourages individuals from committing criminal offences hence a negative relationship between police expenditure and murder is a-priori expected.
Income per capita is included to capture the influence of economic shocks and the extent of local economic development for each municipality. According to Rufrancos et al. (Citation2013), income is a relevant predictor of crime and failure to control its effect may result in a small sample parameter bias that does not disappear asymptotically. Gender composition is measured by the share of men on total population for each municipality. Several studies in literature (Jonck et al., Citation2015; Verrinder, Citation2013 for instance) argue and confirm that men have a higher probability of committing crime than women. Therefore, an increase the share of men on total population in each municipality is expected to increase murder offences.
4. Results and discussion
Table provides variable description and data source. Subsequent to Table is Table which presents summary statistics. The highest and lowest youth unemployment rates (88.77% and 23.30%) come from Indaka and Kwa Sani local municipalities, respectively. In terms of crime, murder rates are highest in Msunduzi and lowest in Kwa Sani local municipality. For the remaining variables, about 51% of total households on average own a brick house while about 61% of total households have access to electricity. At worst, only 17% of households own a brick house (Nkandla local municipality) while about 84% of households at best own a brick house (Newcastle local municipality). With respect to electricity, most households who do not have access to electricity are from Umhlabuyalingana municipality while the majority of households with access to electricity are from uMhlathuze municipality.
Table 1. Variable Description
Table 2. Summary Statistics
The average racial composition suggests that black Africans typically account for 94% of the total population living in local municipalities. This is not surprising since the majority of black Africans are generally positioned south of the income distribution and therefore afford to stay in local municipalities. Other races north of the income distribution typically reside in metropolitans which are relatively more urban than local municipalities. The gender composition on the other hand shows that males account for about 47 per cent of the total population.
Before proceeding with regression results, Table displays further measures of central tendency on murder. Firstly, we observe positive skewness values corroborating Figure that murder offences are heavily skewed towards the right suggesting that the OLS approach may not be appropriate. Secondly, there is evidence of overdispersion as indicated by a variance which is almost 34 times larger than the mean. This descriptive statistic necessitates either the NB regression or a Poisson regression with adjusted standard errors. Both approaches were employed for robustness purposes.
Table 3. Dispersion of Murder
Before presenting regression results, Figure provides a visual inspection of crime and unemployment in terms of a scatter plot. Clearly, there is a positive association between crime and unemployment. In other words, crime and unemployment move together in the same direction. With this visual inference, we can proceed with formal regression results in which the relationship between the two is rigorously examined holding constant standard controls.
Table reports first stage regression results from the reduced form equation with fixed effects and it comprises three variants. These results do not matter significantly as they are essentially meant to exogenise youth unemployment in the second stage. We observe in these preliminary results that the first two instruments proposed by Öster and Agell (Citation2007) do not enter significantly which means they may not be appropriate instruments for youth unemployment. We, therefore, relied on model (3) in which our proposed instrument, Chinese imports enter significantly negative and sizeable. As indicated above, we do not invest time and effort interpreting each of the coefficients in Table as they were simply estimated to compute residuals which are imperative in the second stage of the LW procedure. Important to note is that the residual (u_fe) was computed from model (3) in which all the instruments enter significantly.
Table 4. First Stage FE regression results (LW Procedure)
The main results are reported in Table which again comprises three regression variants. Variant (1) denoted by FE_P are results from a classical Poisson regression with fixed effects. Variant (2) reports the fixed effects Poisson LW procedure (FE_P_LWP) and variant (3) results are estimated from a fixed effects Negative Binomial (FE_NB) technique. The second stage regression in the LW procedure was estimated with bootstrapped standard errors. Interestingly, the fixed effects residual enters insignificantly reflecting a failure to reject the null hypothesis of exogeneity with respect to the idiosyncratic term. This result supports the use of a classical Poisson regression model which only controls for unobserved heterogeneity (variant (1)).
Table 5. Youth Unemployment and Murder
Evidence confirms a positive, significant, and sizeable association between youth unemployment and murder offences in the 0.46–0.51 range. For convenience, we take the antilog of these coefficients so that the partial exogenous influence of youth unemployment on murder offences can be interpreted as incidence risk ratios (IRRs). In the preferred specification, for example, FE_P estimated with bootstrapped standard errors to circumvent the problem of overdispersion, the IRR of youth unemployment is 1.66 while that of variant (3) estimated by the NB method is 1.582. This means that a percentage point increase in youth unemployment increases the odds of murder offences by roughly 1.6 times. This is consistent with Becker’s theory in which unemployment correlates positively with crime and agrees with previous studies (i.e. Ajaegbu, Citation2012; Fella & Gallipoli, Citation2007; Fougère et al., Citation2009; Lochner, Citation2004) in which youth unemployment is found to be a positive correlate of violent crimes.
Most of the control variables enter with expected signs. Expenditure on police services, for example, enters with a significantly negative sign in variant (1) corroborating the theoretical argument that policing reduces crime by increasing the probability of getting caught. Racial composition carries a positive sign which is consistent with results reported in Worrall (Citation2008) but the coefficient is statistically insignificant across all the three regression variants. Put differently, the hypothesis that race is a significant determinant of crime is not supported by empirical data in the context of South Africa.Footnote2
Gender composition is positive but significant in one of the three variants (the fixed effects Poisson variant). The fixed effects Poisson variant suggest that murder increases with the share of men on total population. This is in line with Jonck et al. (Citation2015) and Verrinder (Citation2013) who opine and validate that men have a higher probability of engaging in criminal offences than their female counterparts. It also agrees with Denno (Citation1994) who opine that gender is an important predictor of crime.
House ownership appears to significantly reduce murder offences. This could be explained by two factors. One is that housing provides shelter and safety which can prevent vulnerability of individuals (victims) to murder. The other factor could be that house ownership reduces individual anger and the breakdown of social control. According to social disorganization theories, (see Sampson & Groves, Citation1989), lack of wellbeing leads to social frustration which consequently leads to a breakdown of social control breeding social disturbances such as public protests and crimes. Based on this reasoning, results in Table , therefore, suggest that house ownership reduces the odds of murder. Access to electricity enters however with a puzzling positive and significant association with murder crimes in the first variant. A possible explanation is that electricity can be a catalyst of crime by providing visibility during the night and facilitating communication among criminals as mobile networks generally work effectively in areas with electricity. The positive and sizeable effect of income per capita on the other hand in the first variant is not surprising given the opportunity perspective raised by criminologists in which crime increases with the supply of suitable victims.
5. Concluding remarks
Literature has shown that youth unemployment can be a catalyst for violent crimes. This paper has provided further evidence on this notion focusing in particular on murder crimes in KwaZulu-Natal province of South Africa using local municipality-level data. A contribution of this study was to thread a fine line between heterogeneity endogeneity and idiosyncratic endogeneity using a control function procedure recently proposed by Lin (Citation2009) in panel count data models. The results have indicated that youth unemployment is correlated with heterogeneity endogeneity but uncorrelated with idiosyncratic endogeneity. In other words, we do not find evidence that youth unemployment is correlated with time-varying omitted factors. Put differently, a control function approach does not perform any better than the classical Poisson regression when analysing crime and unemployment. The main finding here is that unemployment is correlated with time-invariant factors and not time-varying factors nested in the error term. A classical Poisson regression with fixed effects was then used as a benchmark estimator. The main finding of the study is that youth unemployment has been positively and significantly associated with murder crimes in KwaZulu-Natal. This is in line with the majority of previous studies and it supports the idea that a deteriorating labour market can be a recipe for disaster as far as crime prevention is concerned. A key policy implication arising from the study is that job creation for young people can be an alternative mechanism through which policymakers can reduce murder crimes in KwaZulu-Natal.
Additional information
Funding
Notes on contributors
Brian Tavonga Mazorodze
Brian Mazorodze is an award winning economist specialising in trade, development and industrial economics. He is currently a research fellow at the University of Zululand main campus and a part-time lecturer at Richards Bay campus.
Notes
1. Quantec is a consultancy providing economic and financial data, country intelligence and quantitative analytical software, based in Pretoria, South Africa.
2. Notwithstanding the statistical insignificance, the positive sign next to the race variable needs to be interpreted with caution as property rents are generally lower in high crime areas and the black majority (who are relatively poor compared to all other races) are likely to reside in these areas. An alternative model was considered without the race variable for robustness checks as race turned out to be insignificant across all the variants in Table 5. The results, reported in Table 5, do not show significant differences in terms of signs and sizes of the remaining coefficients.
References
- Ajaegbu, O. O. (2012). Rising youth unemployment and violent crime in Nigeria. American Journal of Social Issues and Humanities, 2(5), 315–17.
- Baltagi, B. H. (1995). Econometric analysis of panel data (Vol. 2). Wiley.
- Becker, G. S. (1968). Crime and punishment: An economic approach. In The economic dimensions of crime (pp. 13–68). Palgrave Macmillan.
- Bhorat, H., Thornton, A., & Van der Zee, K. (2017). Socio-Economic Determinants of Crime in South Africa: An Empirical Assessment. DPRU Working Paper no. 201704.
- Blomquist, J., & Westerlund, J. (2014). A non-stationary panel data investigation of the unemployment–crime relationship. Social Science Research, 44(2), 114–125. https://doi.org/10.1016/j.ssresearch.2013.11.007
- Burger, R., & Woolard, I. (2005). The state of the labour market in South Africa after the first decade of democracy. Journal of Vocational Education and Training, 57(4), 453–476. https://doi.org/10.1080/13636820500200297
- Cameron Colin, A., & Trivedi, P. K. (1998). Regression Analysis of Count Data, Econometric Society Monograph No. 30. Cambridge University Press.
- Cantor, D., & Land, K. C. (1985). Unemployment and crime rates in the post-World War II United States: A theoretical and empirical analysis. American Sociological Review, 50(3), 317–332. https://doi.org/10.2307/2095542
- Cantor, D., & Land, K. C. (2001). Unemployment and crime rate fluctuations: A comment on Greenberg. Journal of Quantitative Criminology, 17(4), 329–342. https://doi.org/10.1023/A:1012585603639
- Chib, S., & Winkelmann, R. (2001). Markov chain Monte Carlo analysis of correlated count data. Journal of Business & Economic Statistics, 19(4), 428–435. https://doi.org/10.1198/07350010152596673
- Chin, H. C., & Quddus, M. A. (2003). Applying the random effect negative binomial model to examine traffic accident occurrence at signalized intersections. Accident Analysis & Prevention, 35(2), 253–259. https://doi.org/10.1016/S0001-4575(02)00003-9
- Costantini, M., Meco, I., & Paradiso, A. (2018). Do inequality, unemployment and deterrence affect crime over the long run? Regional Studies, 52(4), 558–571. https://doi.org/10.1080/00343404.2017.1341626
- Demombynes, G., & Özler, B. (2002). Crime and local inequality in South Africa. The World Bank.
- Denno, D. W. (1994). Gender, crime, and the criminal law defenses. The Journal of Criminal Law and Criminology (1973-), 85(1), 80. https://doi.org/10.2307/1144115
- Edwards, L., & Jenkins, R. (2015). The impact of Chinese import penetration on the South African manufacturing sector. The Journal of Development Studies, 51(4), 447–463. https://doi.org/10.1080/00220388.2014.983912
- Ehrlich, I. (1975). On the relation between education and crime. In Education, income, and human behavior (pp. 313–338). NBER.
- Fella, G., & Gallipoli, G., 2007). Education and Crime over the Life Cycle. Working Paper series 15-07, Rimini Centre for Economic Analysis
- Felson, M., & Cohen, L. E. (1980). Human ecology and crime: A routine activity approach. Human Ecology, 8(4), 389–406. https://doi.org/10.1007/BF01561001
- Fougère, D., Kramarz, F., & Pouget, J. (2009). Youth unemployment and crime in France. Journal of the European Economic Association, 7(5), 909–938. https://doi.org/10.1162/JEEA.2009.7.5.909
- Gould, E. D., Weinberg, B. A., & Mustard, D. B. (2002). Crime rates and local labor market opportunities in the United States: 1979–1997. Review of Economics and Statistics, 84(1), 45–61. https://doi.org/10.1162/003465302317331919
- Ha, O. K., & Andresen, M. A. (2017). Unemployment and the specialization of criminal activity: A neighbourhood analysis. Journal of Criminal Justice, 48(1), 1–8. https://doi.org/10.1016/j.jcrimjus.2016.11.001
- Hausman, J. A., Hall, B. H., & Griliches, Z. (1984). Econometric models for count data with an application to the patents R&D relationship. National Bureau of Economic Research. Working Paper no. (t10017).
- Jonck, P., Goujon, A., Testa, M. R., & Kandala, J. (2015). Education and crime engagement in South Africa: A national and provincial perspective. International Journal of Educational Development, 45(8), 141–151. https://doi.org/10.1016/j.ijedudev.2015.10.002
- Kelly, M. (2000). Inequality and crime. Review of Economics and Statistics, 82(4), 530–539. https://doi.org/10.1162/003465300559028
- Land, K. C., Cantor, D., & Russell, S. T. (1995). Unemployment and crime rate fluctuations in the post-World War II United States: Statistical time-series properties and alternative models. In J. Hagan & R. D. Peterson (Eds.), Crime and Inequality (pp. 317-332). Stanford University Press.
- Lim, H., Lee, H., & Cuvelier, S. J. (2010). The impact of police levels on crime rates: A systematic analysis of methods and statistics in existing studies. Pacific Journal of Police & Criminal Justice, 8(1), 49–82.
- Lin, M. J. (2008). Does unemployment increase crime? Evidence from US data 1974–2000. Journal of Human Resources, 43(2), 413–436. https://doi.org/10.1353/jhr.2008.0022
- Lin, M. J. (2009). More police, less crime: Evidence from US state data. International Review of Law and Economics, 29(2), 73–80. https://doi.org/10.1016/j.irle.2008.12.003
- Lin, W., & Wooldridge, J. M. (2019). Testing and correcting for endogeneity in nonlinear unobserved effects models. In Panel Data Wooldridge (pp. 21-43). Academic Press.
- Lochner, L. (2004). Education, work, and crime: A human capital approach. International Economic Review, 45(3), 811–843. https://doi.org/10.1111/j.0020-6598.2004.00288.x
- Lord, D. (2006). Modeling motor vehicle crashes using Poisson-gamma models: Examining the effects of low sample mean values and small sample size on the estimation of the fixed dispersion parameter. Accident Analysis & Prevention, 38(4), 751–766. https://doi.org/10.1016/j.aap.2006.02.001
- Merton, R. K. (1938). Social structure and anomie. American Sociological Review, 3(5), 672–682. https://doi.org/10.2307/2084686
- Narayan, P. K., & Smyth, R. (2004). Crime rates, male youth unemployment and real income in Australia: Evidence from Granger causality tests. Applied Economics, 36(18), 2079–2095. https://doi.org/10.1080/0003684042000261842
- Nordin, M., & Almén, D. (2017). Long-term unemployment and violent crime. Empirical Economics, 52(1), 1–29. https://doi.org/10.1007/s00181-016-1068-6
- Osgood, D. W. (2000). Poisson-based regression analysis of aggregate crime rates. Journal of Quantitative Criminology, 16(1), 21–43. https://doi.org/10.1023/A:1007521427059
- Öster, A., & Agell, J. (2007). Crime and unemployment in turbulent times. Journal of the European Economic Association, 5(4), 752–775. https://doi.org/10.1162/JEEA.2007.5.4.752
- Papke, L. E., & Wooldridge, J. M. (2008). Panel data methods for fractional response variables with an application to test pass rates. Journal of Econometrics, 145(1–2), 121–133. https://doi.org/10.1016/j.jeconom.2008.05.009
- Raphael, S., & Winter-Ebmer, R. (2001). Identifying the effect of unemployment on crime. The Journal of Law and Economics, 44(1), 259–283. https://doi.org/10.1086/320275
- Rege, M., Skardhamar, T., Telle, K., & Votruba, M., 2009. The effect of plant closure on crime (No. 593). Discussion Papers.
- Rufrancos, H., Power, M., Pickett, K. E., & Wilkinson, R. (2013). Income Inequality and Crime: A Review and Explanation of the Time series Evidence. Sociology and Criminology-Open Access, 1(1), 1-103. https://doi.org/10.4172/2375-4435.1000103
- Sampson, R. J., & Groves, W. B. (1989). Community structure and crime: Testing social-disorganization theory. American Journal of Sociology, 94(4), 774–802. https://doi.org/10.1086/229068
- Statistics South Africa. (2016). Quarterly Labour Force Survey, Quarter 1: 2016. Statistical Release. South Africa.
- Tshabalala, N. G. (2014). Crime and Unemployment in South Africa; Revisiting an Established Causality: Evidence from the KwaZulu Natal Province. Mediterranean Journal of Social Sciences, 5(15), 519.
- Verrinder, N., 2013. Untangling the determinants of crime in South Africa (Doctoral dissertation), University of Cape Town.
- Wooldridge, J. M. (2010). Econometric analysis of cross section and panel data Vol. 1. The MIT Press. Treatment.
- Worrall, J. L. (2008). Racial composition, unemployment, and crime: Dealing with inconsistencies in panel designs. Social Science Research, 37(3), 787–800. https://doi.org/10.1016/j.ssresearch.2008.01.001
- Zulkifli, M., Ling, A. B. Y., Kasim, M. M., & Ismail, N., 2016. Modeling the number of car theft using Poisson regression. In AIP Conference Proceedings (Vol. 1782, No. 1, p. 050018), Peninsula, Malaysia. AIP Publishing.