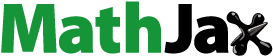
Abstract
Haricot bean is one of the most important food legumes of Ethiopia and it is considered as the main cash crop and the least expensive source of protein for the farmers. Low production and productivity, which are mainly associated with poor adoption and inefficient implementation of improved farm technologies, were among the major problems. Adoption and efficient utilization of improved farm inputs is one of the most promising ways to reduce food insecurity in study area. However, the adoption and implementation of these improved farm inputs is constrained by various factors. So, the aim of this study was to analyze the technical efficiency and impact of improved farm inputs adoption on the yield of haricot bean producers. A multi-stage sampling technique was employed to select 231 sample household heads and they were interviewed using structured interview schedule. Data analysis was done with the help of Stochastic Frontier Analysis; mainly Cobb-Douglas Production Function, logistic regression model were employed. The Stochastic Production Frontier result revealed that the allocated amount of land, labour, seed, chemical fertilizer and oxen were appeared to be positively and significantly influencing haricot bean production of both adopters and total sampled householders. The result of logistic regression model indicated that the probability of adoption of improved farm inputs affected by extension service, information access and cooperative membership positively and by market distance and crop diversification negatively. Therefore, specialization, asset formation, innovative institutional arrangement, information, extension and farmers training accompanied with more access to chemical fertilizer in the study area.
PUBLIC INTEREST STATEMENT
Technical Efficiency and Impact of Improved Farm Inputs Adoption on the Yield of Haricot bean Producers in Hadiya Zone, Ethiopia has general objective to analyze the technical efficiency, adoption of improved farm inputs and their impact on yield of haricot bean producers in Hadiya zone. Despite the fact that the current agricultural extension activities are promoting the adoption of improved haricot bean seed and chemical fertilizer utilization, the uptake of improved farm inputs by haricot bean producers have been inadequate; instead many farmers have been using local haricot bean seed varieties, and except few large-scale producers most haricot bean growers do not use chemical fertilizer at the recommended level in the study area. Overall, the information that generated from this study helps haricot bean producers, policy makers, agricultural extension workers, and other stakeholders to take evidence based decisions on efficient resource use, promotion and scaling-up of yield improving technologies.
1. Introduction
1.1. Background
Grain legume production is dominated by small-scale farmers, and it is mainly cultivated as a major consumable crop and production has been grown tremendously over the 2000–2012 period, at an annual average growth rate of 34 percent in the world (FAOSTAT, Citation2012). In 2008, the total world grain legume production was about 3.54 million tons that was grown on 7.42 million hectares.
Although pulses have many desirable characteristics in terms of high value crops, rich in nutrition and able to harness environmental benefits, in most parts of Ethiopia they are considered secondary crops. As a secondary crop category, pulses do not receive investment resources and policy attention as do the cereal crops, which are often, considered food security crops. Studies have shown that pulses on an average contribute 15% of total protein intake, account for 13% of the cultivated land and 8.5% of the total crop production in Ethiopia (Chilot et al., Citation2010). In recent years the pulse sector shows a steady increase in productivity and total volume of production. Recently the government of Ethiopia made strides to transform the subsistence smallholder agriculture to commercial-oriented one. To this effect pulses are considered as the critical pathway for transforming the subsistence agriculture into commercial-oriented agriculture (CSA, Citation2012).
Haricot beans are among the most important grain legumes produced by small-scale farmers, both for subsistence and cash. On average, haricot beans account for 16.3 percent of pulse production in Ethiopia (2005–2012), and are mainly produced in the lowlands and in the Rift Valley areas, where they are a source of income, employment and food. Virtually all bean production is carried out by about 3.1 million smallholder farmers, on small plots with minimal inputs (CSA, Citation2012).
In southern region, haricot beans are one of the major and economically important commodity crop produced by small-scale and mainly for both subsistence and commercial purposes. The average production statistics (2005–2012) obtained from CSA (2013) depicted that southern region accounted for the competent rank, almost 25% of the country’s total haricot beans production. In this region, Hadiya zone is the main haricot beans producing area at small-scale levels.
Although, many small-scale farmers have been engaged in haricot beans production for a longer period, currently large-scale (Investors) are also participating in haricot beans producing activities in Hadiya zone with 63,963 hectares of land transferred to them (North Gondar Investment Office (NGIO), Citation2014). Despite Hadiya zone has high potential in haricot beans production, average productivity of haricot beans are far below the national average per hectare (Central Statistical Authority (CSA), Citation2011). This is mainly due to poor crop management practices and limited availability and use of improved haricot beans varieties and fertilizer (CSA, Citation2012). Even though haricot beans are considered as a low nutrient feeder, organic and inorganic fertilizer application showed yield increment compared to untreated plots (Haruna, Citation2011; Umar et al., Citation2012). However, adoption and application of chemical fertilizer remains low in the study area and in Ethiopia in general. For example, in 2011, at the national level chemical fertilizer users were only 30–40 percent which primarily used for legume production while insignificant proportion is used for haricot beans farming and the physical application rate of fertilizer at household level is still below the recommended dose (Spielman et al., 201; Rashid et al., Citation2013).
The domestic economy of Ethiopia is extremely dependent on traditional agriculture and agricultural-based activities. Agriculture accounts for 38.8% of the country’s Gross Domestic Product (GDP), 85% of all exports (coffee, livestock and livestock products and oil seeds), 70% of raw materials for the industries and 85% of employment for the population in the country (MOFED, Citation2006; Ministry of Finance and Economic Development (MoFED), Citation2010). In particular, the role of agriculture in securing the food needs of the fast-growing population is considerable. Despite its contribution to the GDP and export earnings, the sector’s productivity is very low. The rationales for the low agricultural productivity in general and for haricot bean in particular in Ethiopia mainly include severe weather fluctuations, a decline in soil fertility, poor linkage of input and output markets, low technology adoption rate, production inefficiency, poor infrastructure and market access, prevalence of pests and diseases, and low capacity and in-efficient governmental and private sector institutional services (Dercon & Hill, Citation2009; FekaduGelaw, Citation2007; Katungi et al., Citation2010).
Efforts have been underway by the national agricultural research system since its establishment in 1956 and a number of improved farm inputs have been released for the farming community. In spite of these efforts, productivity gains are not as such adequate in the country. Low level of adoption of improved farm inputs is among the major reasons (Ahmed et al., Citation2013; Hailu et al., Citation2014; Spielman et al., Citation2011). In Ethiopia, over the period (2005–2012/13), area cultivated with haricot beans increased from 164 to 359 hectares, a 120 percent growth. On the other hand, the average national yield per hectare is low over the same period, with an average of 0.99 tones/hec (CSA, Citation2014). The productivity rate of haricot beans is low due to low use of chemical fertilizers, small plots for cultivation associated with population growth and land fragmentation; limited access to improved seed varieties; and poor land-management techniques (International Food Policy Research Institute (IFPRI), Citation2010).
Agricultural productivity depends on the level of improved farm inputs as well as on efficient use of resources in the production process. Among improved farm inputs that could improve productivity, chemical fertilizer and improved seed varieties are the major components. However, due to many factors, farmers are mainly using local and uncertified seed. In addition, the use of chemical fertilizer is insufficient and that only few farmers have been applying it at recommended level on their haricot bean fields while many of them are resistant to use (EPPA Ethiopian Pulses ProfileAgency, Citation2011). For this reason, despite improvements over the past decade, the productivity of agricultural system is very low and about 36% of the population are in chronic food insecurity in the study area (Zonal report, Citation2017). Similar to the other parts of the country, haricot bean is among the most important staple food crops grown in Hadiya zone. Improving the technical efficiency and technology adoption particularly for haricot bean producers would influence the food security of the private peasant households in the study area.
But researches on technical efficiency of peasant agriculture are not extensive, and the findings or conclusions of some of them are not consistent with one another. Therefore, policy implications drawn from some of the above empirical works may not allow in designing area-specific policies to be compatible with its socio-economic as well as agro-ecologic conditions and the results of some of the studies may not allow making comparative analysis of farmers’ efficiency across all areas. As improved technologies are developed and promoted, we must note that adoption is necessary, but not sufficient, to enhance yields. The technical efficiency with which these technologies are applied in the farmers‟ fields is equally if not more important (Mwangi, Citation2014).
However, many studies in relation with improved agricultural technologies are focused only on determinants of technology adoption in a single manner, but not in combination (complementary) with its determinants of efficient implementation (Ahmed et al., Citation2013; Biftu et al., Citation2016; Amare and Bekele, Citation2016). In such cases, it is difficult to have a clear understanding of the adoption of agricultural technology and its technical efficiency. Other existing studies even on haricot bean technology adoption are broad and at national level (eg. Shiferaw et.al., 2014; Jaleta, Citation2007). These, in turn, have a limitation in terms of targeting solutions towards addressing policy interventions in specific contexts. Hence, examining the impact, technical efficiency and determinants of improved farm inputs adoption on haricot bean producer’s yield and income would give information whether to continue with the existing technology by improving the efficiency of less efficient farmers or to promote an improved farm inputs adoption with improving its efficiency so as to increase haricot bean productivity in the study area.
Therefore, analyzing the factors affecting technical efficiency, improved seed and chemical fertilizer adoption in the study area was paramount importance. This study aimed at filling these research gaps. The general objective of this study is to analyze the technical efficiency, adoption of improved farm inputs and their impact on yield of haricot bean producers in Hadiya zone.
2. Empirical literature review
2.1. Efficiency analysis
Debebe et al. (Citation2015) studied on efficiency of maize production and its determinants using parametric stochastic frontier production function applying Cobb-Douglas production function and Tobit model for smallholder maize producing farmers in Jimma zone of southwestern Ethiopia. The maximum likelihood (ML) estimates of the parameter of the stochastic frontier Cobb–Douglas production function results showed that the mean technical efficiencies score was found to be 62.3%, indicating a substantial level of inefficiency in maize production. Inefficiency effects also had modelled in a second stage applying a two-limit Tobit regression model. The model revealed that technical efficiencies are positively and significantly influenced by size of household, education level, the size of livestock holding (TLU), extension service, cooperative membership and use of mobile cell-phone, while total landholding size of the household head was negatively and significantly influencing efficiencies of maize production.
The empirical performance of the parametric distance function and data envelopment analysis with application to adopters of improved cereal production technology in Eastern Ethiopia was studied by Alene and Zeller (Citation2005). Both approaches reveal that there are substantial technical inefficiencies of production among sample farmers. Technical efficiency estimates from the two methods are positively and significantly correlated. But the data envelopment analysis (DEA) approach is found to be very sensitive to outliers and choice of orientation. The parametric distance function results are very robust and revealed that adopters of improved technology have average technical efficiency of 79 percent implying that the farmers could increase food crop production on average by 21 percent if they fully exploit the potentials of improved seeds and chemical fertilizers. The authors also confirmed that the single output production frontier approach has been the standard approach to farm level efficiency analysis and most have revealed substantial inefficiencies of production in developing countries.
Mekonnen et al. (Citation2015) used a stochastic frontier production function to estimate farm household efficiency and its determinants among sesame producer farmers in south Omo zone, Southern Ethiopia. The cross–sectional data analysis from 120 randomly selected farm households of sesame producers was indicated that the Average Technical Efficiency (TE) was 67.11%. This implies that farmers can increase their sesame production on average by 32.9% if they were technically efficient. Results of the Tobit model revealed that soil fertility; non-farm income and credit access positively and significantly affected TE.
The study conducted by Bifarin et al. (Citation2010) examined technical efficiency using stochastic frontier model on cross-sectional data by fitting Cobb-Douglass production function on plantain farmers in Nigeria. The findings showed that technical efficiency indices varied widely and the estimated coefficients indicated that age and extension services are significant and positively correlated to technical efficiencies. In addition, the results of the inefficiency models revealed that farmer education and access to credit contributed significantly and positively to technical inefficiency, while extension visit and age had a significant impact, but had an inverse relationship with it. This implies that the older the farmer is, the more efficient he becomes.
Okpe et al. (Citation2012) assessed resource use efficiency and rice production in Nigeria using gross margin analysis and stochastic production function on cross-sectional data. The results indicate that yield and profit of small farmers is low compared to large farmers; the estimated coefficients using maximum likelihood show positive sign implying increase in quantities of input use would result in increase of rice output. The inefficiency model also shows that resource is not fully utilized in all farm categories. It can be noted from this finding that most farmers are inefficient in farm resource utilization which is also in line with the findings of several production efficiencies in developing countries.
Similarly, technical and scale efficiency analysis in rice production using data envelopment analysis was conducted in Nigeria by Ogisi et al. (Citation2012). They found out that most rice farmers (about 77 percent) operate with increasing returns to scale; education level, farmers experience and extension agents visit significantly influenced the efficiency level of farmers in rice production. However, farm size is found to be negatively correlated and has no effect on resource use efficiency.
Tadese et al. (Citation2014) used household survey data from Ethiopia and evaluated the impact of agricultural cooperatives on stallholder’s technical efficiency in crop production. They used propensity score matching to compare the average difference in technical efficiency between cooperative member farmers and similar non-cooperative farmers. The results show that agricultural cooperatives are effective in providing support services that significantly contribute to members‟ technical efficiency. These results are found to be insensitive to hidden bias and consistent with the idea that agricultural cooperatives enhance members‟ efficiency by easing access to productive inputs and facilitating extension linkages. According to the findings, increased participation in agricultural cooperatives should further enhance efficiency gains among smallholder farmers.
2.2. Adoption analysis
Arega et al. (Citation2010) assessed the importance of farmers’ access to resources, extension services, and availability of improved maize seed in their study on adoption of improved maize varieties measured by Tobit regression model in central highlands of Ethiopia. In addition, the study also found that farm income is a significant factor differentiating users from non-users and hence has implications for changing the existing input credit scheme, which requires farmers to settle 25–50 per cent down payments. Such requirement may be beyond the capacity of the resource poor farmers and thus represents a hindrance to adoption of improved agricultural production technology. At the same time, availability of improved seed proved to be a major constraint for adoption, a fact that calls for improvements in improved seed delivery to effectively cope with the demands of small farmers.
Yu et al. (Citation2011) examined the determinants of the adoption of fertilizer and seed technology in Ethiopia using a double-hurdle model of fertilizer use for major crops. The study reveals that access to fertilizer is related to access to extension services and that production specialization together with wealth play a major role in explaining crop area under fertilizer. Inefficiency in farm input utilization is found to be the most important factors limiting adoption of fertilizer.
Adoption study conducted by Asfaw et al. (Citation2011) examined that the farmer’s decisions to adopt agricultural technologies using cross-sectional sample data from farmers in Ethiopia. They estimated a logistic model to analyze the determinants of technology adoption conditional on overcoming access constraints. The study reveals that knowledge and perception about the attributes of improved farm inputs, household wealth (livestock and land) and availability of active labor force are major determinants for adoption of improved technologies.
Teame (Citation2011) has investigated fertilizer adoption and the intensity of fertilizer utilization using a panel data in Tigray region, Ethiopia. Tobit model has been used to examine factors that determine the probability of fertilizer adoption and the intensity of fertilizer use. The likelihood of fertilizer adoption has been explained by the head of the household’s education status, labour endowment, farm size, the number of plots that the farmer used, the distance to plots from homesteads, oxen ownership and the distance to market from residence. On the other hand, the intensity of the input use is explained by the household head’s education status, farm size, manure use, the number of plots the farmer used, the distance to plots from homesteads, and oxen ownership. The study indicated that time has its own significant impact in determining the intensity of the input use, and it has less effect on the likelihood of fertilizer adoption in the region.
Edward et al. (Citation2013) investigated the effect of factors influencing mineral fertilizer adoption among smallholder farmers in Northern Ghana. The result of logistic regression estimate indicated that income of household head, membership of farmer association, distance to agricultural office, access to input shop, income-earning household that do not participate in agricultural development project was the significant factors influencing fertilizer adoption.
3. Research methodology
3.1. Data requirement and sources
This study was used both quantitative and qualitative data to maintain balance between them because neither of the two types can be sufficed by themselves to incorporate the trends and details of the situation that they need to complement each other, to allow a complete analysis and generate reliable information (Silverman, Citation2005). When both quantitative and qualitative studies are applied together enable us to gain a sound understanding of the problems. The data include both secondary and primary data sources; both theoretical and empirical literatures, organization’s reports and, small-scale producers and experts at zonal and district level.
3.2. Sampling method and sample size
Multi-stage and a combination of both purposive and random sampling techniques were employed to draw representative sample. In the first step, out of eleven woredas in the zone, three woredas such as misrak badawacho, shashogo and meirabbadawacho were purposively selected for this study. The reason for purposive sampling was, the presence of large number of haricot bean producing household heads and its extent of production in the study area. The three woredas actually comprise of 91 rural kebeles. From these, 73 kebeles are major haricot bean producer kebeles. Since the research focusses basically on haricot bean production, haricot bean producer kebeles were the major target areas for sample selection (Zonal report, 2016/17).
In the second stage, out of 73 kebeles from three woredas, eight kebeles were selected randomly. In the third stage, the researcher stratified haricot bean producers into improved farm inputs adopters and non-adopters based on the list of households of the kebeles who produce haricot bean during 2016/17 production year, hereafter 231 sample farm household heads were selected from the total household heads of eight kebeles by using systematic random sampling (SRS) technique based on probability proportional to size (PPS).
According to Edriss (Citation2013) the bigger controversy arises when determining adequate sample size to run a regression model due to their weakness and strength of the formulae. Therefore, we need to reconcile sample size that are determined using some formulae and the minimum sample size required to run a model with a number of variables included in the model. Hence, what is suggested by most statisticians and econometricians is that to take into consideration the number of variables in the model; and determine the sample required. Thus, using:
n = 10 times the number of relevant independent variables in a given model written as:
Where n is sample size and K is the number of relevant independent variables included in the model and as long as, 10 K greater than n = 30 (the magic number in parametric statistics). To determine a representative sample size for this study, the relevant explanatory variables considered are 21 for the small-scale haricot bean producers.
Therefore, using Equationequation (1)(1)
(1) and the given values as well as the resources available for the survey, the feasible sample size (n) to be interviewed is calculated as 210 representative sample household heads were selected from the haricot bean producers.
Even though, the total sample size is calculated as 210, in order to increase accuracy of estimation and considering the large samples 10% (21 sample respondents) from the total sample were additionally selected. Therefore, the total sample respondents were 231. Then, the sample size for each producer group from each district was calculated as:
where ni is the sample size from each selected district (i = Misrak badawacho, Meirab badawacho and Shashogo) woredas where, n is total sample size of the study which is the sum of the sample size of the three woredas, and Ni is total haricot bean farm household head in respective woredas, and N is the total population or haricot bean farm households of the three woredas combined (Table ). This procedure was applied separately for each producer group. Similarly for the allocation of sample size for each kebele, the same procedure (PPS method) was applied. Then the total number of haricot bean producer households in the three woredas are 22,150 (Hadiya zone agricultural development offices, unprocessed data obtained through personal communication). The existing proportion of haricot bean producers of Misrak badawacho, Meirab badawacho and Shashogo woredas were estimated 37.7, 30.3 and 32 percent, respectively. Based on this proportion, sample respondents from participant and non-participants in improved seed; chemical fertilizer and fertilizer with improved seed for each woredas and kebelewas allocated. Finally, the participant and non-participant households were used for the analysis of all stated objectives. Numbers of sample respondents‟ distribution from each woredas are depicted as follow, Sample respondent distribution from each woredas are depicted as follow:
3.3. Data collection methods
Cross-sectional data from both primary and secondary sources were collected as well as structured and pre-tested questionnaire was applied to collect primary data. Both quantitative and qualitative information was collected through face-to-face interview with individuals, key informants and then focus groups discussions using enumerators from recruited agricultural extension staff with prior experience in survey work. Enumerators have been trained on the objectives of the survey, a detailed question-by-question review of the survey instrument, instructional sessions on interviewing techniques, role-playing exercises, and practice interviews with local farmers. Before data collection, pre-testing of the questionnaire have been carried out, and then depending on the results, there were some adjustments that would be made to the final version of the questionnaire. This interview schedule was contained socio-economic, demographic characteristics, institutional and organizational variables. In addition, personal observations and informal communication with small-scale farmers from sample kebeles was implemented in order to generate primary information. Secondary data were obtained from government offices and other relevant organizations.
3.4. Methods of data analyses
The data was analyzed using descriptive and inferential statistics and econometric methods. Descriptive and inferential statistics such as mean, percent, standard deviation, t-test, F-test, Chi-square test, likelihood ratio test and analysis of variance (ANOVA) were used to analyze the data that was collected from sample household heads. Analysis of technical efficiency, identifying determinants of adoption of improved farm inputs and analyzing impact of adoption of improved farming inputs and their determinants were carried out using econometric methods. Particularly, Stochastic Frontier Cobb-Douglas Production, Double-hurdle model analytical techniques were employed to analyze haricot bean technical efficiency, determinants of adoption of improved farm inputs and impact of agricultural technology adoption on farmer’s yield, respectively. For this analysis, STATA software version 13 was used.
3.4.1. Efficiency analysis
3.4.1.1. Specification of the stochastic frontier model
Stochastic Frontier Model was introduced by Aigner et al. (Citation1977) and Meeusen and van den Broeck (Citation1977); and for n sample farms, it can be written as:
Where Yi is haricot bean output of the household’s farm, i = (1, 2, and 3 … n) are sample household farms,
is the i_th household used by the j_th input and
is a vector of unknown parameters and
is composed of error term which can be written as:
Where is a symmetric random error which represents random variations, or random shocks in the production of the
household, outside the control of the farmer assumed independently and identically distributed as
. The error term
is a one-sided non-negative variable which measures technical inefficiency of the
household, the extent to which observed output falls short of the potential output for a given technology and input levels. The method helps to decompose deviation of the actual observed haricot bean output from the estimated frontier into random variations and inefficiency. Hence,
Where, Zi is a vector of variables that explain inefficiency of household.
Is a vector of unknown coefficients that are to be estimated in the model, and
to ensure that
(Battese and Coelli, Citation1995). The technical efficiency of production of the ith farm in the data set, given the level of inputs, is defined by the conditional expectation evaluated at the maximum likelihood estimates of the parameters in the model, where the expected maximum value of
is conditional on
. The measure of technical efficiency
must have a value between zero and one. Following Equationequations (3)
(3)
(3) and (Equation5
(5)
(5) ), technical efficiency was estimated as:
Or equivalently could be expressed as:
Where is technical efficiency, the inefficiency term
is always between 0 and 1, When
is equal to zero, then production is on the frontier
and
, therefore a farmer is technically efficient. When
is greater than zero (
> 0) the farmer is technically inefficient (
< 1), since production will be below the frontier.
Given the specifications of the stochastic frontier model expressed in Equationequations (6(6)
(6) ), the stochastic frontier output (potential output) for the ith farm is the observed output divided by the technical efficiency
:
The parametric specification of stochastic frontier production function in the Cobb-Douglas form for one output and n inputs is given by:
Where,
: is haricot bean output of
household,
: represents vector of farm inputs used
: is intercept
: is vector of production function parameters to be estimated.
Given the level of technical inefficiency derived from Equationequation (7)(7)
(7) and the above-specified
vector inefficiency explanatory variables (Table ), the coefficients of inefficiency variables was estimated simultaneously along with the coefficients of input variables. As discussed above, technical inefficiency effect
is specified as:
Where, is a vector of unknown coefficients that are to be estimated in the model.
4. Results and discussion
This chapter presents the results and discusses the core findings of the study and presents empirical analyses of impact of agricultural technology adoption with its intensity and efficiency, and it further discusses the findings of the study in comparison with earlier related research results.
4.1. Adoption analysis
In this section, we analyze factors affecting haricot bean farmers’ adoption decision of improved technologies (like chemical fertilizer and improved seeds) by taking 2016/2017 production year as a reference. To analyze the problem we employed a Logistic regression model and fourteen explanatory variables were hypothesized to influence the probability of adoption included in the analysis (Table ).
However, prior to running the final regression analysis, both the continuous and discrete explanatory variables need to be checked for the existence of multi-collinearity using Variance Inflating Factor (VIF) and the contingency coefficient (CC) methods. Accordingly, as can be seen from the results, our test result suggests that, there is no serious multi-collinearity problem in our model, since there is no strong association among the hypothesized explanatory variables. Therefore, all of the proposed potential explanatory variables were included in the final regression.
Five variables were found to be statistically significant in explaining farmer decision to improved technology. The likelihood of deciding to adopt agricultural technology for a household is affected to positively increase for those householders who have good access for extension service, information access and membership on agricultural cooperatives but negatively influenced with attitude of soil fertility and market distance.
Accesses to information positively affect the likelihood of adopting agricultural technology. This effect is captured by ownership of information supporting assets like TV, radio or mobile phone, education level of the household head and contact with extension agents. To adopt the newly introduced agricultural technology, farmers need to be aware of the available agricultural technology as adoption is sometimes hampered not only by the inherent characteristics of the technologies themselves but also by lack of awareness of the end users of the technologies. Hence, farmers’ awareness about the available technologies is an important factor for the adoption to take place. Our results confirm this preposition. Awareness of improved varieties and chemical fertilizer was statistically significant in explaining adoption. Those farmers who knew more about improved varieties and chemical fertilizer during preceding year probably have better information about the advantages of them and are likely to adopt and allocate more land during the current year. The result of study may actually show that information was the major limiting factor determining the farmer’s ability to get hold of improved seeds and chemical fertilizer. This positive effect of farmer technology awareness variable is consistent with studies for pigeonpea varieties Tanzania (Shiferaw et al., 2008), cowpea varieties in Nigeria (Kristjanson et al., Citation2005) and maize varieties in Tanzania (Kaliba et al., Citation2000).
Contact with extension agents explained the variation in adoption to agricultural technology significantly.
The result indicates that distance to market has significant and negative influence on adoption of improved technologies by less than 1% Level. This implies that improved farm technology adoption by farmer’s increases with increased access to market. The probable reason for this was that farmers who have more access to input and output markets had access to market information such as price information on different production input and output. Besides, they were accessing agricultural inputs to purchase and utilize. On the other hand distance from the market of the farm households is expected to directly affect the transaction cost on input purchase and output marketing. The higher the distance from the market, the higher the transaction cost and lower the likelihood of adoption haricot beans variety and fertilizer. Results of similar previous studies (Mulugeta & Bekele, Citation2014; Ogada et al., Citation2014) also supported existence of inverse relationships between distance of the farmers from market and the likelihood of adoption of new crop variety.
Similarly, soil fertility perception of the farm households on the crop is significantly (negatively) related to status of adoption of improved farm technologies specifically chemical fertilizer.
Being a member of a cooperative institution was found to positively influence adoption of improved farm technologies. Other factors remain constant, being members of cooperatives were found to affect farmers’ likelihood of the technology adoption by the factor of 1.5, and the result was statistically significant at 5% level. The result conveys the message that cooperatives are among the strongest social institutions that play crucial roles in adoption of improved agricultural technologies, and also findings from the studies by Tesfay et al. (Citation2005), Abebawand Haile (Citation2013) and Ahmed (Citation2015) are consistent with this result.
All variables capturing access to extension information (number of contacts with extension workers) returned significant coefficients with expected positive signs at 10% level suggesting that farmers who have more contacts with extension personnel are significantly more likely to know of the existence of improved farm inputs than farmers that had fewer contacts with extension personnel.
4.2. Efficiency analysis using SFA method
4.2.1. Test of hypothesis
This section tries to evaluate the extent of farm households’ TE of haricot bean production in the study area. Keeping in mind the widely accepted way of carrying out SFA in efficiency analysis, it was opted for executing multiple inputs and one output and it is possible to test various hypotheses using maximum likelihood ratio test, which were not possible in non-parametric models.
In order to choose an appropriate model for further analysis and interpretation, hypotheses tests are critical before discussing about parameter estimates of production frontier function and the inefficiency effects. Because of this, three hypotheses were tested, to select the correct functional form for the given data set, for the existence of inefficiency and for variables that explain the difference in efficiency.
The first test identifies an appropriate functional form between restrictive Cobb Douglas and the more flexible Translog production function which specifies that square and cross terms are equivalent to zero. The Translog frontier function turns into Cobb-Douglas when all the square and interaction terms in the translog are zero. The test is made based on the value of likelihood ratio (LR) statistics, which can be computed from the log likelihood value obtained from estimation of Cobb-Douglas and Translog functional specifications. Then, this computed value is compared with the upper 5% critical value of the χ2 at the degree of freedom equals to the difference between the numbers of explanatory variables used in the two functional forms (in this case df = 21). For the sample households, the estimated log likelihood values of the Cobb-Douglas and Translog production functions for total sample households were −163.5 and −152, (It is −97 and −86.7 for adopters and −58.92 and −47.7 for non-adopters) respectively. The computed value of likelihood ratio (LR) = 23 for total householders (20.6 for adopters and 22 for non-adopters) is lower than the upper 5% critical value of the χ2 with its respective degree of freedom (Table ). Thus, the null hypothesis that all coefficients of the square and interaction terms in Translog specification are equal to zero was not rejected. This implies that the Cobb-Douglas functional form adequately represents the data.
Table 1. Selected districts and sample distribution
Table 2. Definitions efficiency measurement variables
Table 3. The maximum likelihood estimates of the logistic model
Table 4. Generalized likelihood ratio tests of hypothesis for the parameters of the SPF
The second null hypothesis was H0: γ = 0, which specifies that the inefficiency effects in the SPF were not stochastic, i.e. haricot bean producing farms are efficient and have no room for efficiency improvement. After the appropriate production function is selected, the next step is a test for adequacy of representing the data using SPF over the traditional mean response function, OLS. The null hypothesis, H0: γ = 0, which specifies that the inefficiency effects are absent from the model (that is all haricot bean producers are fully efficient). Whereas, the alternative hypothesis, H1: γ > 0, states that there is inefficiency in production of haricot bean in the study area. Since this study is using the STATA version 13 computer programs, after fitting the function with the required defined variables the computer output displays results which include the test of null hypothesis about inefficiency component. From this computer program output it is found that, log likelihood value = 184.14, (χ2 (01)-value = 13.29 and p = 0.001) for total sample households (but it is log likelihood value = 97, (χ2 (01)-value = 19.11 and p = 0.025 for adopters and log likelihood value = 58.92, (χ2 (01)-value = 8.21 and p = 0.04 for non-adopters). Therefore, the decision of null hypotheses H0: γ = 0, which specifies that the inefficiency effects are absent from the model is rejected at 1% level of significance for the total sampled households (but it is 5% level for both adopters and non-adopters).
The coefficient for the discrepancy ratio (γ) could be interpreted in such a way that for the total sampled households about 85.40% (90% for adopters and 80.20% for non-adopters) of the variability in haricot bean output in the study area was attributable to technical inefficiency effect, while the remaining 14.6% variation in output was due to the effect of random noise (10% for adopters and 19.80% non-adopters). This implies presence of scope for improving output of haricot bean by first identifying those institutional, socioeconomic and farm attribute factors causing this variation. Therefore, this data can be better represented by the stochastic production frontier than the average response function. The null hypothesis was rejected (Table ). This implies the traditional average production function does not adequately represent the data. Therefore, the inclusion of the technical inefficiency term is an important issue to the model.
The third null hypothesis that the explanatory variables associated with inefficiency effects are all zero (H0: δ 1 = δ 2 … = δ21 = 0) was also tested. To test this hypothesis likewise, LR (the inefficiency effect) was calculated using the value of the Log-Likelihood function under the stochastic production function model (a model without explanatory variables of inefficiency effects: H0) and the full frontier model (a model with explanatory variables that are supposed to determine inefficiency of each: H1).
For the total sample households, the calculated value LR = −2(184.14–203.3) = 38.92 (for adopters LR = −2(97–135.52) = 77.04 and for non-adopters LR = −2(58.92–81.8) = 45.76) is greater than the critical value of 32.67 at 21 degree of freedom (Table ) the value of LR implying that, the null hypothesis (H0) that explanatory variables are simultaneously equal to zero was rejected at 5% significance level. Hence, these variables simultaneously explain the sources of efficiency differences among sample farmers in the study area. Thus the observed inefficiency among the haricot bean farmers in Hadiya zone could be attributed to the variables specified in the model and the variables exercised a significant role in explaining the observed inefficiency. Therefore, the result confirms as the null hypothesis was rejected, implying that there is at least one variable that explains the difference in efficiency.
4.2.2. Estimation of parameters of production function model
The output variable was crop production defined as quantity of haricot bean harvested in quintals whereas the inputs were land, labor, seed, fertilizer, oxen and agrochemicals.
The result of the Cobb-Douglas stochastic production frontier for the total sampled householders showed that inputs like chemical fertilizer (at 1% significance level), seed (at 5% significance level) and agrochemicals (at 10% significance level) allocated for haricot bean were found to positively and significantly explained the level of efficiency of haricot bean production (Table ), which are important variables in shifting the frontier output to the right. This indicated that at each and every unit of these variables there is a possibility to increase the level of output. But the increase of land, labour and oxen is insignificant.
Table 5. Maximum likelihood estimate of stochastic production frontier model (House holders)
In the case of improved technology adopters the result showed that inputs such as both chemical fertilizer and improved haricot bean seed at 1% significance level and agrochemicals at 5% significance level explained the level of efficiency of haricot bean production positively (Table ), the remaining inputs like land, labour and oxen does not affect the production system insignificantly. On the other hand the amount of labor and oxen allocated for haricot bean production were found to positively and significantly contribute at 1% significant level and amount of seed used affect the producers efficiency negatively at 1% significance level of non-adopters. The reason for the negative impact of seed usage is that significant amount of non-adopter farmers used local seed with broadcasting system which is exposed for over population of seedlings and high completion for the limited amount of nutrients. The labor and agrochemicals allocation has insignificant effect on non-adopters of haricot bean producers (Table ).
Table 6. Maximum likelihood estimate of stochastic production frontier model (Adopters)
Table 7. Maximum likelihood estimate of stochastic production frontier model (Non-Adopters)
Out of total inputs allocated for haricot bean production, the elasticity of fertilizer is very high implying that these have more effect in determining the output level at the best practice (the maximum technical efficiency score). The positive coefficients of inputs indicate a 1% increase in fertilizer, seed and agro chemicals yields 0.2948%, 0.1265% and 0.1105% increase in haricot bean output improvement, respectively for the sampled householders; in the case of improved farm technology adopters 1% increase in fertilizer and seed causes 0.4481% and 0.1351% increments on haricot bean yield. But for the non-adopters 1% increase in human labor, oxen and local seed provides 0.1931% and 0.1367% increments and 0.1440% decrement on haricot bean output, respectively. In other words, as indicated on the above tables if all the inputs are improved by 1%, haricot bean output would increase by 1.4653% for total sampled householders (1.54% for adopters and 0.72% for non-adopters). The results showed that improved farm technology adopters are operating in the stage one of production process (increasing return to scale) and have ample opportunities to increase output by improving their efficiencies.
Even though nonnegative and less than one value of the sum of elasticity of non-adopters imply that producers are operating in the stage two of the production process (decreasing return to scale), they are not efficient in allocation of resource this implies production is inefficient moreover there is a room to increase production with a decreasing rate. But to break decreasing return to scale, the only way is that non-adopters are promoted to adopt new technologies that improve productivity of haricot bean farm. The reason is that in the long run improving the existing level of technical efficiency of non-improved farm technology adopter households alone may not lead to significant increment in the level of haricot bean output.
Another essential outcome in the analysis is the variance ratio parameter γ which found to be significant at 1% level expressing that about 85.4% of haricot bean output for the total respondents (90% for adopters and 80.2% for non-adopters) deviations are caused by differences in farm level technical efficiency as opposed to the random variability that are outside their control of the producers. In order to decrease inefficiency (technical as well as noise) specifically for non-technology adopters it is advisable to internalize external technologies like improved seed and chemical fertilizers to boost productivities.
4.2.3. Technical efficiency scores of sample households
According to the SFA model results, there exists a difference in efficiency scores among the haricot bean farmers in the study area. TE scores range from 11.7% to 91.9% (from 67.6% to 84.1% for adopters and from 45% to 93% for non-adopters). Such low efficiencies in production indicated potential for improvements in haricot bean production given the current levels of technology among the farmers.
The SFA model results indicated that the average farm households’ TE of 59.8% (it is 77.4% for adopters and 78.8% for non-adopters), indicating farm households are producing 40.1% less (that means 22.6% for adopters and 21.2% for non-adopters) of potential output given their prevailing level of technology and input use. Alternatively, the farmers could still produce their current outputs of these crops with fewer inputs if they were more efficient. Under the assumption of CRS, the efficiency scores remain the same in both input orientation (input minimization) and output orientation (output maximization).
Thus, if we had chosen to keep inputs constant and measure efficiency in output increasing direction the efficiency score is also indicating that outputs should be increased by 40.1% to become efficient (it became 22.6% % for adopters and 21.2% % for non-adopters).
Therefore, this result shows the existence of significant technical inefficiency in haricot bean production among smallholder farmers in the study area. The mean levels of efficiencies were comparable to those from other similar studies in Ethiopia (Seyoum et al., Citation1998; Alene & Zeller, Citation2005; Debebe et al., Citation2015; EndriasGeta et al., Citation2010; Haji, Citation2008).
4.2.4. Estimation Results of Sources of Inefficiency
Understanding the sources of TE and its extent is very important for policy making to address the problem of farm households. In this regard, demographic, socio-economic farm and farmer-specific and institutional variables were hypothesized to affect level of inefficiency of haricot bean growing farmers in the study area. Accordingly, the inefficiency model parameters were estimated by MLE method using one-stage estimation procedure (Table ). After measuring levels of households’ efficiency and determining the presence of efficiency differences among households, finding out factors causing inefficiency disparity among households was the next most important step of this study. To see this, inefficiency levels of sample households were regressed on factors that were expected to affect inefficiency levels using a MLE estimation procedure. The marginal effects of changes in explanatory variables from regression were computed for the purpose of interpretation. That is, the derived values for the significant explanatory variables indicated that the effects of a unit change in those variables on the unconditional expected value of TE and expected value of TE conditional upon being between 0 and 1, and probability of being between 0 and 1.
The result of the model showed that TE total sampled householders were significantly influenced by access to training, distance to market, sex of householders, distance to FTC, crop diversification and livestock holdings. In the same manner access to training, distance to market, distance to FTC, total livestock holdings and crop diversification were important factors influencing TE of improved farm input technology adopters in the study area. Non adopters were also significantly influenced by sex of householders and market distance and oxen holdings (Table ).
Table 8. Summary statistics of estimated technical efficiencies of sample households
Table 9. Maximum likelihood estimates of the factors determining technical inefficiency
There were significant differences in technical efficiencies among male-headed and female-headed farm households. Male-headed households are more likely to be efficient than female-headed farm households. This is due to the fact that female-headed households have additional responsibilities within the household, such as caring for children and this may affect the relative crop productivity of females.
Livestock holdings (excluding ox) had a positive and significant effect on TE in haricot bean production. Farmers who owned large number of livestock were technically more efficient in crop production. This is because livestock provides manure fertilizer and also a source of income that can be used to purchase agricultural inputs. Possessing a large number of livestock is crucial to increase TE in crop production in the study areas. Thus, a unit increase in the value of TLU would have an overall increase in the level of TE. The current result is consistent with the findings of Abdulahi and Eberlin (Citation2001), Ahmed et al. (Citation2002), Bamlaku et al. (Citation2004), Beyene (Citation2008), Tesfay et al. (Citation2005), Asfaw et al. (Citation2011), and Debebe et al. (Citation2015) in their respective studies with different crops.
Oxen holdings had a positive and significant relationship on TE in crop production. This is because oxen provides a draught power, manure fertilizer and is a source of income. Thus, possessing a large number of oxen is crucial to increase TE in crop production in the study areas. This result is consistent with the findings of EndriasGeta et al. (Citation2010) on maize efficiency.
Another factor worth considering, as a variable affecting TE, is proximity to factor markets. The hypothesis in this study is that households located near markets are expected to have higher TE than those located in remote areas. This might be due to the fact that as farmers are located far from market, there would be limited access to input and output markets and market information. The other possible argument can be access to markets may increase the non-farm employment opportunities with higher returns than from farming, leading them to reallocate labour from farm to non-farm activities. More importantly, longer distance from market leads to higher transaction cost that reduces the efficiency of crop-producing farmers. Similar result was found in the work of Bamlaku et al. (Citation2004), EssaChanie (Citation2011), and Hasen (Citation2013).
Farmers’ access to training is expected to provide farmers with new information, training, and demonstrations to encourage best farming practices. Thus, the study considered access of training as one factor of efficiency and there were statistically significant positive relationship between access to training and technical efficiencies among farm households.
Farm households in the study area experienced to grow diversified crops to minimize drought risks resulted from shortage of rainfall and small land holding. However, the result revealed that having more number of diversified crops negatively and significantly influencing farm households’ technical efficiency. The result confirmed that if a typical household add one more crop to grow from the existing crops, then there would have an overall decline in the probability and level of TE. A plausible reason for this observation can be attributed to the fact that under diversified cropping systems, farm households needs to make use of more inputs like fertilizer, seeds and labour, which implies increasing cost pressure more than attaining productivity that contributes the existence of substantial technical and economical inefficiency.
5. Conclusion
The agricultural sector in Ethiopia would continue to be the main source of economic development and the growth of this sector would originate from smallholder farmers. But in the current food insecurity is aggravated by rapid population growth and low productivity. The main reason for low productivity is the low adoption of new improved farm inputs and weak technical efficiency of the sector. The possibility of expanding cultivable land is almost exhausted; this problem could be solved only through improving agriculture sector performance specifically small-scale farmers technical efficiency, in complementary with breaking through the resistance to new technologies, and expansion of modern improved farm inputs adoption to exploit the maximum yield on existing cultivated land.
Empirical analysis of improved farm inputs of smallholder farm households computed by logistic model pointed out different determinants impacting the improved farm inputs adoption. To this effect, result of the model showed that the adoption of agricultural technology for a household is affected positively with extension service, information access and membership on agricultural cooperatives but negatively influenced with attitude towards soil fertility and market distance.
Econometric analysis of technical efficiency of smallholder farm households (adopters and non-adopters) computed by SFA model pointed out that 85.40% (90% for adopters and 80.20% for non-adopters) of the variability in haricot bean output in the study area was attributable to technical inefficiency effect, while the remaining 14.60% variation in output was due to the effect of random noise (10% for adopters and 20% non-adopters).The major significant factors determining technical inefficiencies for total sampled householders were access to training, distance to market, sex of householders, distance to FTC, crop diversification and livestock holdings. In the same manner access to training, distance to market, distance to FTC, total livestock holdings and crop diversification were for adopters and sex of householders, market distance and oxen holdings were for non-adopters.
Out of six only three inputs like chemical fertilizer, seed and agrochemicals allocated for haricot bean were found to positively and significantly explained the level of efficiency of haricot bean production (fertilizer, seed and chemicals have significant positive impact for adopters but labor and oxen have positive and seed have negative effect for non-adopters); In other words, if all the inputs are improved by 1%, haricot bean output would increase by 1.4653% for total sampled householders (1.54% for adopters and 0.72% for non-adopters). The SFA model results indicated that the average farm households’ technical efficiency (TE) of 59.8% (it is 77.4% for adopters and 78.8% for non-adopters), indicating farm households are producing 40.1% less (that means 22.6% for adopters and 21.2% for non-adopters) of potential output given their prevailing level of technology and input use.
Additional information
Funding
Notes on contributors
Tesfaye Merra
Mr. Tamirat Beyene (Msc in Economic policy analysis) is a full time lecturer at Wolkite University for the last three years and yet now. Dr. Wondaferahu Mulugeta (PhD, Associate professor of Economics) is a full time professor at college of business and economics, Jimma University, Ethiopia. He has more than 10 years of teaching experience in total. His areas of research interest are macro issues like impact of monetary and fiscal policies analysis, on exchange rate instabilities etc. Mr. Tesfaye Melaku (Assistant professor of Economics, Msc in Economics) has worked more than 7 years at college of Business and Economics, Jimma University, Ethiopia. His research interests are program impact analysis, microfinance, poverty, and adoption of improved farm inputs. Further, Mr Tesfaye Melaku is a full member of Ethiopian economic association.
References
- Abdulai, A., & Eberlin, R. (2001). Technical efficiency during economic reform in Nicaragua: Evidencefrom farm household survey data. Economic Systems, 25(2), 113–21. https://doi.org/10.1016/S0939-3625(01)00010-3
- Abebaw, D., & Haile, M. (2013). The impact of cooperatives on agricultural technology adoption: Empirical evidence from Ethiopia. Food Policy, 38(C), 82–91.
- Ahmed, B., Haji, J., & Geta, E. (2013). Analysis of Farm Households’ Technical Efficiency in Production of Smallholder Farmers: The Case of Girawa District.
- Ahmed, M. (2015). Adoption of multiple agricultural technologies in maize production of the Central Rift Valley of Ethiopia
- Ahmed, M. M., Berhanu, G., Benin, S., and Ehui, S. (2002). Measurement and source of technical efficiency of land tenure contracts in Ethiopia. Environmental and Development Economics, 7, 507–528.
- Aigner, D. J., Lovell, C. A. K., & Schmidt, P. (1977). Formulation and estimation of stochastic frontier production function models. Journal of Econometrics, 6(1), 21–37. https://doi.org/10.1016/0304-4076(77)90052-5
- Alene, A., & Zeller, M. (2005). Technology adoption and efficiency in multiple crops production in eastern Ethiopia: A comparison of parametric and non-parametric distance functions. International institute of tropical agriculture.
- Amare, M., & Bekele, S. (2016). Determinants of adoption of wheat production technology package by smallholder farmers: Evidences from eastern Ethiopia
- Arega, A., Poonyth, D., & Hassan, R. M. (2010). Determinants of adoption and intensity of use of improved maize varieties in the central highlands of Ethiopia: A tobit analysis, Agrekon. Agricultural Economics Research, Policy and Practice in Southern Africa, 39, 633–643.
- Asfaw, S., Shiferaw, B., Simtow, F., & Mekbib, G. (2011). Agricultural technology adoption, seed access constraints and commercialization in Ethiopia. Journal of Development and Agricultural Economics, 3(9), 436–447.
- Bamlaku, A., Nuppenau, A., & Boland, H. 2004. Technical efficiency of farming systems across agro-ecological zones in Ethiopia: An application of stochastic frontier analysis [PhD Dissertation presented to department of environmental management and market research]. Justus Liebig University of Giessen.
- Battese, G. E., and Coelli, T. J. (1995). A model for technical inefficiency effects in a stochastic frontier production function for panel data. Empirical Economics, 20, 325 – 332
- Beyene, H. (2008). Adoption of improved tef and wheat production technologies in crop- livestock mixed system in Northern and Western Zones of Ethiopia (A Dissertation). The University of Pretoria.
- Bifarin, J. O., Alimi, T., Baruwa, O. I., & Ajewole, O. 2010.Determinants of Technical, Allocative and Economic Efficiencies in the Plantain (Musa spp.). Production Industry.
- Biftu, A., Diriba, B., Bayisa, T., Getachew, F. (2016). Participatory demonstration and evaluation of bread wheat technologies: The experience of FRG/FREG approach in Bale and West Arsi zones of Oromia national regional state, Ethiopia. Scientific Journal of Crop Science, 5(3), 90-103. http://www.sjournals.com/index.php/SJCS/article/view /2146 (January,2017)
- Chilot, Y., Shahidur, R., Befekadu, B., & Solomon, L. (2010). Pulses Value Chain Potential in Ethiopia IFPRI report
- CSA. (2012). Agricultural sample survey 2011/2012. Volume I. Area and production of crops. Statistical Bulletin. Central Statistical Agency, Addis Ababa Ethiopia.
- CSA. (2014). Agricultural sample survey 2013/14 (2006 E.C.). Volume IV: Report on land utilization by private peasant holdings. Statistical Bulletin. number 446.Central Statistical Agency, Addis Ababa, Ethiopia.
- CSA (Central Statistical Authority). (2011). Agricultural sample survey 2010/11 (2003 E.C) (Sep-Dec 2010). Vol I. Report on area and production of major crops (private peasant holdings, Meherseason). Statistical Bulletin, Addis Ababa, 12–96.
- Debebe, S., Haji, J., Goshu, D., & Abdi, A. K. (2015). Technical, allocative, and economic efficiency among smallholder maize farmers in South western Ethiopia: Parametric approach. Journal of Development and Agricultural Economics, 8, 283–292.
- Dercon, S., & Hill, R. V. (2009). Growth from agriculture in Ethiopia: Identifying key constraints (Background Paper). http://www.futureagricultures.org/pdf%20files/Ethiopia%20paper.pdf.
- Edriss, A. K. 2013. Pearls of applied statistics. Theory and STATA application (BEECSI SERIES). Publication International i-publishers.
- Edward, M., Alexander, N. W., Prince, M. E., & Mathias, F. (2013). Fertilizer Adoption and Use Intensity Among Smallholder Farmers in Northern Ghana: A Case Study of the AGRA Soil Health Project.
- EndriasGeta, B., Kassa, A. B., & Elias, E. (2010). Productivity and efficiency analysis of smallholder maize producers in Southern Ethiopia. Journal of Human Ecology, 41(1), 67–75.
- EPPA Ethiopian Pulses ProfileAgency. (2011). Ethiopian Export Promotion Agency Product.Development & Market Research Directorate Ethiopia.
- EssaChanie. (2011). Resource use efficiency of smallholder crop production in the central highlands of Ethiopia [M.Sc Thesis Presented to Department of Agricultural Economics and Business Management]. Egerton University.
- FAOSTAT. (2012). Food and agricultural organization of the United Nations. Retrieved September 16, 2015, from http://www.fao.org/agriculture/seed/cropcalendar/cropcalendar.do
- FekaduGelaw, 2007. Analysis of technical efficiency of wheat production: A study in MachakelWoreda, Ethiopia [M.Sc. Thesis Presented to School of Graduate Studies of Alemaya University].
- Hadiya Zone Report 2017
- Hailu, B. K., Abrha, B. K., & Kibrom, A. (2014). Adoption and impact of agricultural technologies on farm income: Evidence from Southern Tigray, Northern Ethiopia. International Journal of Food and Agricultural Economics, 4, 91–106.
- Haji, J. 2008. Economic efficiency and marketing of vegetable production in eastern and central parts of Ethiopia. Doctoral Dissertation, University of Swedish.
- Haruna, I. M. (2011). Growth and yield of sesame (Sesamumindicum L.) as affected by poultry manure, Nitrogen and Phosphorus at Samaru, Nigeria. The Journal of Animal & Plant Sciences, 21(4), 1018–7081.
- Hasen, M. 2013. Economic efficiency of smallholder farmers in maize production: The case of ArsiNegelle district, Oromia National Regional State, Ethiopia [M.Sc. Thesis]. http://www.researchconnections.org/childcare/datamethods/experimentsquasi.jsp
- IFPRI (International Food Policy Research Institute). (2010). Maize Value Chain in Ethiopia: Constraints and Opportunities for Enhance the System.
- Jaleta, M. (2007). Econometric analysis of horticultural production and marketing in central and eastern Ethiopia (PhD Diss.). Wageningen University.
- Kaliba, A., Verkuijl, H., & Mwangi, W. (2000). Factors affecting adoption of improved maize seeds and use of inorganic fertilizer for maize production in the intermediate and Lowland Zones of Tanzania. Journal of Agricultural and Applied Economics, 32(1), 35–47.
- Katungi, E., Farrow, A., Mutouki, T., Gebeyehu, S., Karanja, D., Alemayehu, F., Sperling, L., Beebe, S., Rubguyo, J. C., & Buruchara, R. (2010). Improving common bean productivity: An analysis of socio-economic factors in Ethiopia and Eastern Kenya.Baseline Report (Tropical Legumes II.Centro International de Agriculture). Tropical-CIAT.
- Kristjanson, P., Okike, I., Tarawali, S., Singh, B. B., & Manyong, V. M. (2005). Farmers‘ perceptions of benefits and factors affecting the adoption of improved dual-purpose cowpea in the dry savannas of Nigeria. . Agricultural Economics, 32(2), 195–210. https://doi.org/10.1111/j.0169-5150.2005.00338.x
- Meeusen, W., & van den Broeck, J. (1977). Efficient estimation from cobb-douglas production functions with composed error. International Economic Review, 18(2), 435–444. https://doi.org/10.2307/2525757
- Mekonnen, E., Geta, E., & Belaineh, L. (2015). Production efficiency of sesame in selamago district of south omo zone, southern Ethiopia. Current Research in Agricultural Sciences, 2(1), 8–21.
- MoFED (Ministry of Finance and Economic Development). (2006). Ethiopia: Building on Progress a Plan for Accelerated and Sustained Development to End Poverty (PASDEP) (2005/06-2009/10).Volume I: Main text, Ministry of Finance and Economic Development, September, 2006, Addis Ababa.
- MoFED (Ministry of Finance and Economic Development). (2010). Five years Growth and Transformation Plan (GTP): The Federal Democratic Republic of Ethiopia.
- Mulugeta, T., & Bekele, H. (2014). Impacts of Adoption of Improved Wheat Technologies on Households’ Food Consumption in Southeastern Ethiopia.
- Mwangi, W. (2014). Adoption of Improved Bread Wheat Varieties and Inorganic Fertilizer By Small-Scale Farmers in YelmanaDensa And Farta Districts Of Northwestern Ethiopia. Zonal report, 2016/17).
- NGIO (North Gondar Investment Office). (2014). Sesame seed production investment quarter report.
- Ogada, M. J., Mwabu, G., & Muchai, D. (2014). Farm technology adoption in Kenya: A simultaneous estimation of inorganic fertilizer and improved maize variety adoption decisions. Journal of Agricultural and Food Economics, 2:12.
- Ogisi, O. D., Chukwuji, C. O., & Okeke, D. C. (2012). Efficiency of resource use by rice farmers in Ebonyi state, southeastern Nigeria: A data envelopment analysis. Asian Journal of Agricultural and Rural Development, 2(2), 149–154.
- Okpe, I. J., Abur, C. C., & Omniyi, S. O. (2012). Resource use efficiency and rice production in Guma local government area of Benue state: An application of stochastic frontier production function. International Review of Social Sciences and Humanities, 1(3), 108–116. Accessed July 25, 2015 http://www.irssh.com
- Rashid, S., Nigus, S., Minot, N., & Gezahengn, A. (2013). Fertilizer in Ethiopia: an assessment of policies, value chain, and profitability (IFPRI Discussion Paper). Addis Ababa.
- Seyoum, E., Battese, G., & Fleming, E. (1998). Technical efficiency and productivity of maize producers in Eastern Ethiopia: A study of farmers within and outside the Sasakawa- Global 2000 project. Journal of Agricultural Economics, 19, 341–348
- Shumet, A. (2011). Analysis of technical efficiency of crop producing smallholder farmers in Tigray, Ethiopia (MPRA Paper Number 40461). Retrieved October, 2016, from https://mpra.ub.uni-muenchen.de/40461/1/MPRA_paper_40461.pdf
- Silverman, B. (2005). Statistics for functional data.
- Spielman, D. J., Dawit, K., & Dawit, A. (2011). Seed, fertilizer, and agricultural extension in Ethiopia (ESSP Working paper). Addis Ababa.
- Tadese, G., Francesconi, G. N., & Kinde, G. (2014). Impact of agricultural cooperatives on smallholders‘ technical efficiency: Empirical evidence from Ethiopia. Annals of Public and Cooperative Economics, 85(2), 257–286. https://doi.org/10.1111/apce.12035
- Teame, H. (2011). Factors determining fertilizer adoption of peasant farm sector in Northern Ethiopia, Tigray [MSc. Thesis]. Norwegian Unversity of Life Sciences, department of economics and resource management.
- Tesfay, G., Ruben, R., Pender, J., & Kuyvenhoven, A. (2005). Resource use efficiency on own and sharecropped plots in Northern Ethiopia: determinants and implications for sustainability.
- Umar, U. A., Mahmud, M., Abubakar, I. U., Babaji, B. A., & Idris, U. D. (2012). Effect of nitrogen fertilizer level and intrarow spacing on growth and yield of sesam (Sesamumindicun L.) varieties. International Journal of Agronomy and Plant Production, 3(4), 139–144.
- Yu, B., Nin-Pratt, A., Funes, J., & Sinafikeh, A. (2011). Cereal production and technology adoption in Ethiopia. Ethiopia strategy support program II (ESSP II) (ESSP II Working Paper 31). International Food Policy Research Institute.