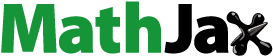
Abstract
This paper aims to analyze factors explaining the capital structure puzzle in the banking sector of Pakistan. Capital Adequacy Ratio (CAR) is used as a proxy for bank capital structure. Secondary data has been collected from the publications of the State Bank of Pakistan and the annual reports of banks from 2006–2017. The data is analyzed by applying the pooled OLS, fixed effect, and the GMM estimator. The determinants are grouped in (i) bank financial performance (ii) bank risk-based and (iii) industry level. Using bank financial performance indicators, the alternate cost of capital is significant, and management quality is an insignificant determinant of capital structure. Similarly, using bank risk-based variables, default risk, and credit risk is statistically significant whereas, bank risk index is statistically insignificant in explaining capital structure. Furthermore, using the industry-specific variables, both the average CAR of the banking sector and the market competitiveness proxied by the Herfindahl-Hirschman Index (HHI) is significantly predicting capital structure. Overall the bank risk-based and the industry-specific indicators are explaining bank capital structure more significantly compared to other indicators in the study. Finally, this paper included the financial crisis to observe any exogenous shocks while studying capital structure which is found to be significant in the current study.
PUBLIC INTEREST STATEMENT
This research empirically investigates explore the relationship of numerous bank-specific as well as industry-specific characteristics with banks’ capital adequacy in Pakistan. Scheduled banks are divided into conventional banks charging interest on loans and Islamic banks potentially interest-free but sharing profits and risk with borrowers. Pakistan has witnessed a transition by giving way and transforming policies to allow private commercial banks in the last two decades. Despite some challenges and inconsistent policies, the banking industry of Pakistan is one of the top growing sectors of the economy. Following internationally recognized standards and being part of the global banking community, the Pakistani banking sector needs to know and get familiarity with the determinants of its capital structure. The findings indicate the Pakistani banking sector should consider the alternate cost of capital, the risk that borrowers may not pay in time or cannot pay for its loans, and market competition from other banks.
1. Introduction
The financial system is composed of financial markets and financial intermediaries. According to Merton and Bodie (Citation1995), financial systems facilitate resource allocation, across space and time, in an uncertain environment as their primary function. Whereas Levine (Citation1999) divided the role of financial systems into five functions like facilitating trading, hedging, diversifying and pooling of risk, allocation of resources, and monitoring managers & exercising corporate control, mobilizing savings, and facilitating the exchange of goods & services.
The banks act as financial intermediaries and facilitate the exchange of payments between individuals, corporations, and governments. According to Dietrich and Wanzenried (Citation2011), the efficiency of the banking sector is essential to smoothly run the economic activities of any country. Athanasoglou et al. (Citation2008) emphasized that to mitigate economic shocks, the banking sector must be a profitable one.
This research is based on Pakistani scheduled commercial banks which operate in a dual banking system since 2002Footnote1 and are considered to be an integral part of the financial system and the overall economy. Over the last several decades, the banking industry in Pakistan has witnessed a dramatic transition, with the dominance of government banks giving way to private banks. All of the country’s private banks were nationalized in the 1970s. In 1990, government shareholding in the banking sector was 93% which declined to 22% in 2004.
Despite inconsistent policies, the banking industry of Pakistan is one of the top growing sectors of the economy. The total assets of the Pakistani banking sector recorded a year on year growth of 15.9% to Rs 18.3 trillion during the calendar year (CY) 2017 with bank assets to GDP ratio of 43.14 percent in 2017, while the bank deposits to GDP for Pakistan rose to 32.6 percent in 2017. Capital Adequacy Ratio (CAR) was recorded at 15.8% as of the end of December 2017 is stronger and higher than the minimum required level of 11.275%. Similarly, asset quality has also improved, and non-performing loans to loan ratio came down from 10.1% in CY16 to 10 years low 8.4% in CY17 (CitationPakistan Economic Survey, 2017–18).
Historically, the banking sector is efficiently maintaining capital adequacy (Table ) but it still might face liquidity challenges due to systematic and unsystematic risks. This fact is evident when the Basel Committee for the banking sector updated regulations to Basel-III and the State Bank of Pakistan (SBP) implemented Basel III through a phase-in arrangement in Pakistan. The implementation started with a Total Capital plus Capital Conservation Buffer (CCB) of 10% in the years 2013 and 2014 followed by 10.25% in the year 2015. The requirement for year 2016 rose to 11.25%, 11.875% for 2017, and 12.5% thereafter (SBP, Citation2013).
Table 1. Capital Adequacy Ratio of Commercial Banks
Table reveals that on average the sample scheduled banks of the study conform to the BASEL-III minimum capital requirements set by the SBP for all sample years. Two banks namely, the Bank of Punjab and Silk Bank Limited were not fulfilling the minimum requirements of 10% for 2013. Furthermore, for years 2014 and 2015, Silk Bank Limited and Small and Medium Enterprise (SME) Bank were not meeting the requirements for the years 2014 and 2015 respectively. In 2016, the Dubai Islamic Bank Limited was short of the minimum requirements, this was their first year of operation and Silk Bank Limited also did not maintain the minimum capital requirements in both years 2016 and 2017.
The financial system of any economy revolves around commercial banks which play an imperative role in capital mobilization through extending the services of financial intermediation and mobilization of savings. The financial institution’s key role of monetary intermediation involving changing investments maturity state and offering insurance to depositors prospective liquidity needs makes banks more fragile (Diamond & Dybvig, Citation1983). The economic significance of commercial banks stability was also endorsed during the crises faced by the global financial sector in 2008–09, which extends many interesting queries related to the stability and performance of financial institutions and the damages instigated to the global economy.
Globally, this topic has received great attention especially after the global banking liquidity crisis of 2008. A review of existing literature shows that many countries including Pakistan remain unexplored. To increase the understanding of the current topic, policymakers and academicians need to conduct comprehensive studies on this important topic in unexplored markets like Pakistan.
No extensive study to the best of the author’s knowledge is carried out in Pakistan regarding the optimal capital structure of scheduled banks in the context of risk and industry-specific factors. Therefore, the primary aim of the study is to empirically investigate the conundrum of banks’ capital structure. Using the data of twenty-four scheduled commercial banks in Pakistan from 2006–2017, the study attempts to answer the question that what factors (bank-specific, risk-based, and industry-specific) determine the commercial banks’ capital adequacy in Pakistan?
The current body of this empirical study is focused on the banking sector of Pakistan considered an important emerging Asian market to unveil the conundrum of bank capital structure. This empirical research has several significant contributions to the existing literature for banks’ capital adequacy ratio. The main contribution of this study in the literature is that it is a pioneer empirical research to the best of authors’ knowledge which has focused on the risk behavior of Pakistani commercial banks concerning the capital adequacy ratio.
Second, the research study estimated and used the Herfindahl-Hirschman Index to measure the competitiveness among banks as an industry-specific variable and also analyzed the effects of the global financial crises 2007–2008 in determining the factors affecting banks’ capital adequacy ratio.
Third, from the methodological perspective, this study applied the Arellano-Bond (AB) linear dynamic panel-data GMM estimator for the first time in the Pakistani context to empirically examine simultaneously the bank-specific, risk-based, and industry-specific determinants of banks’ capital adequacy ratio, which is different from the empirical work of Bokhari et al. (Citation2013), who applied the weighted-average on the data for carrying out the empirical analysis and Masood and Ansari (Citation2016), who applied the fixed-effect estimator in their empirical work.
The study is structured in the following manner: Section 2 reviews the existing literature on banks’ capital adequacy ratios followed by section 3 which presents the data, methodology, variables of the study, and development of hypotheses. Section 4 of this chapter details the results and discussion of the study, and finally, the chapter ends with the conclusion and policy recommendations.
2. Literature review
The capital structure decisions of banks which are an integral part of the financial sector of an economy are primarily different from firms in the non-financial sector because banks use “deposits” as a major source of financing which is not an option of financing for non-financial firms. Another prominent difference is that financial institutions especially commercial banks hold buffer capital for safety reasons in compliance with the regulations imposed by the central banks.
Capital adequacy provides resilience against risk and is considered as one of the most important characteristics of the banking sector. It provides a shield to the banking sector against bad investment and unpredicted incidents. Resilience is a capability to carry on in life despite any uncertainties that come on one’s way and to be resilient people should have access to several institutions which are the main pillars of economic growth (Van Oudheusden et al., Citation2015).
In a regulated economy, the central banks require commercial banks to maintain a minimum CAR. Even in the unregulated economy, banks would still prefer to hold capital because the markets force them to do so as a posture of financial strength (Berger et al., Citation1995). This capital is kept as a cushion and it may absorb unforeseen losses to some extent. In situations, where unanticipated losses exceed the cushion amount, the failure of banks takes place. All countries practice the prevalence of regulated capital ratios by commercial banks because bank failures may prove contagious for the economy.
The empirical study of Hahn (Citation1966) is considered to be the pioneer in the studies on the factors affecting the Capital Adequacy Ratio (CAR) of banks. Hahn (Citation1966) analyzed the factors that affect the capital adequacy of banks in the United States. The regulated capital requirements have been proved unsuccessful in controlling the benefits of risk aversion (Hovakimian & Kane, Citation2000; Kahane, Citation1977; Koehn & Santomero, Citation1980). The bank shareholders may take advantage of this abridged scrutiny by the increase in the banks’ leverage as well as the volatility in earnings due to increased risk, shifting the risk towards bank guarantors and creditors (Hovakimian et al., Citation2003).
Several empirical studies have focused on analyzing the risk-taking behavior of banks with their CAR besides other variables. For example, In an empirical analysis of Swiss banks, Bichsel and Blum (Citation2004), investigated the association of risk and leverage, taking into account accounting as well as market data for capital and risk from 1990 to 2002. They found a positive relationship between the level of capital held and risk, while concluded that bank stability cannot be achieved merely with the leverage restriction imposed by regulatory bodies.
In a study of the Malaysian banking sector, Ahmad et al. (Citation2009) tested the empirical model including the capital ratio as the dependent variable. They used non-performing loans, risk index of a bank, bank management quality, regulatory requirements, bank leverage, bank size, and liquidity as predictors. The findings reveal that non-performing loans, bank leverage, and bank liquidity are positively associated with banks’ capital ratio whereas bank size, management quality, and bank risk index are insignificant using the FE model with the FGLS estimator.
In another study on European banks from 1995–2007 analyzed the association of risk and capital in one model and efficiency and capital in another model by applying the Granger causality model. The results show that inefficient banks were more prone to risk compared to efficient banks. Additionally, efficiency increased as the opportunity cost of capital increases (Fiordelisi et al., Citation2011).
Most recently, in a study of 28 Islamic banks, Kalifa and Bektaş (Citation2018) found that size, credit risk, leverage, ROE, and ROA among the bank-specific factors were significantly associated with banks’ capital adequacy ratio, whereas among the macroeconomic variables, namely exchange rate, market capitalization, and inflation are significantly predicting CAR.
2.1. Empirical works on banks’ capital adequacy
Thoa et al. (Citation2020) investigated the capital adequacy ratio of Vietnamese banks using dimensional banking factors. The study used seven years of data from 2009–2015. Bank size, liquidity, profitability, loan loss reserve, and loan were studied as determinants of capital adequacy ratio. To evaluate the data feasible generalized least square method was used. The findings indicate that liquidity and bank size are significantly predicting capital adequacy ratio negatively whereas other variables were insignificant in the study.
Bambang et al. (Citation2019) employed data from 2014 to 2017 by taking bank size, bank efficiency, capitalization of banks, and loan to deposit ratio was studied as predictors of Indonesian banks’ capital adequacy ratio. The fixed-effect model was used to examine the data and it was concluded that the bank size and loan to deposit ratio are predicting CAR significantly having a negative relationship, while on the contrary, bank equity was positively predicting CAR. While other variables namely, bank efficiency, bank capitalization were insignificant in the study.
In a study of Kuwaiti banks, Hewaidy and Alyousef (Citation2018) found that bank liquidity, asset quality, management quality, and bank size are among the bank-specific variables that are significantly predicting the bank’s CAR. Moreover, bank liquidity, asset quality, and bank size are negatively related to bank’s CAR, whereas, management quality is positively related. All macroeconomic variables are found insignificant in the model. Moussa (Citation2018) in their study for Tunisian banks also found that management quality, return on assets, inflation, and private ownership is affecting positively, whereas liquidity is negatively related to bank capital.
The subsidiaries of foreign banks were investigated to examine how the bank’s home country regulatory environment and economic conditions impact the CAR of foreign banks. The study used 310 subsidiaries and 265 branches using data period from 2000 to 2010. The results suggest that the factors affecting the CAR of developed and developing countries vary. The findings of the study further indicate that specific factors concerning the parent bank have a greater effect on foreign banks (Mili et al., Citation2017).
Klepczarek (Citation2015) examined the capital adequacy determinants of European banks. Specifically, the study examined the common equity tier-1 ratio factors. The data from twenty-two European countries were examined that consisted of forty-nine banks for the year 2013 data. The OLS regression model was used to analyze the data. The results suggest that size and risk indicators such as share of loans in total assets and a risk-weight asset to total asset ratio had a significant impact on the bank’s capital adequacy ratio.
The Egyptian banking sector was examined from 2004 to 2013 to study the determinants of CAR. Liquidity, profitability, earning asset ratio, bank size, deposit asset ratio, loan loss provision, net interest margin growth, and loan asset ratio were studied as factors significantly predicting capital adequacy ratio. The international financial crisis 2007–2007 was also considered while examining the data. The results suggest that before the financial crisis profitability, asset quality, and size were the most significant factors the affect CAR. While, after the crisis period, results suggest that credit risk, management quality, asset quality, size, and liquidity were the variables that significantly affected the CAR. Moreover, for the complete sample from 2004 to 2013, the results conclude that size, liquidity, and management quality were the most statistically significant predictors of CAR (El-Ansary & Hafez, Citation2015).
In an empirical study of the Indonesian banking sector, Abusharba et al. (Citation2013) using data from the period of 2009–2011 found that the CAR of Islamic banks was explained by operational efficiency, profitability, deposit structure, asset earning quality, and liquidity. Results also reveal that liquidity and profitability are positively associated with CAR while asset earning quality was negatively associated. Depositor’s funds and operational efficiency were insignificant in their model.
In the Pakistani context, researches like Bokhari et al. (Citation2013), used bank-level data from 2005–2009 and applied the weighted-average least square for carrying out the empirical analysis. The findings reveal that capital ratio requirement, portfolio risk, and the average capital ratio had a weak, while return on equity and share of deposits had a strong association with banks’ capital adequacy ratio.
Schaeck and Cihak (Citation2012) studied the association of competition and capital ratios by taking bank-level data to test whether greater levels of capital ratios are kept by the banks when they face strong competition. The findings indicate that banks operating in a competitive environment they hold more capital compared to a less competitive environment.
Büyüksalvarci and Abdioğlu (Citation2011) used loan loss reserves, bank size, deposits, loans, ROE, ROA, leverage, bank margin, and liquidity to analyze the determinants of CAR in Turkey. The results depict that ROA and loan loss reserves were positively predicting bank’s CAR, whereas ROE, leverage, and loans were negatively associated. The study also found that bank margins, deposits, size, and liquidity are insignificant with banks’ CAR. In an earlier study of the Turkish banking system, Asarkaya and Özcan (Citation2007) employed the lagged capital adequacy ratio (CAR), level of risk, size of assets, ROE, liquidity, the average CAR, economic growth, and regulatory pressure as factors determining the CAR of Turkish banks. The findings suggest that liquidity was negatively associated, while the average CAR, lagged CAR, risk, ROE, economic growth were positively related to the CAR of Turkish banks.
Romdhane (Citation2011) took capital ratio as the dependent variable while risk, interest margin rate, the cost of equity, demand deposit variability, intermediation rate for the bank, the average CAR, and bank size as predictors and concluded that Net Interest Margin (NIM) and risk are affecting the capital ratio significantly.
In a study of American firms, Frank and Goyal (Citation2009) concluded that market leverage was better explained by few reliable factors, namely, tangibility, inflation, asset size, the market-to-book value of assets, tangibility, profits, and the median leverage of the industry. Tangibility, inflation, asset size, and the median industry average affected leverage positively while the market-to-book value of assets and profits impacted leverage negatively.
Inderst and Mueller (Citation2008) argued that banks should have adequate leverage to attain the advantages to provide new loans to their borrowers. Banks that are completely equity financed are unproductively outdated regarding their lending decision making. The presence of reasonable leverage encourages banks to assume greater risks to achieve higher profits.
In an empirical study of Hong Kong’s banking sector, Wong et al. (Citation2008) took the quarterly data from 1992 to 2004 for thirty-one banks. The study employed the lagged CAR, regulatory capital requirement, risk, size of assets, GDP growth, ROE, average CAR of the sector, the ratio of interbank borrowing to total borrowing, and the financial crisis dummy as independent variables to determine the CAR. The results reveal that the lagged CAR, regulatory capital requirement, size of assets, GDP growth, ROE, average CAR of the sector, the ratio of interbank borrowing to total borrowing are significantly predicting CAR, whereas risk and the financial crisis dummy are statistically insignificant.
Gropp and Heider (Citation2007) found a noteworthy consistency in sign, significance, and economic magnitude for the factors affecting the bank capital structure compared to firm capital structure. They took leverage as the regressand, whereas profitability, collateral, bank size, and a dummy for dividend payers were the regressors. All the regressors were found significant in the study.
In an empirical analysis for building societies and banks in the United Kingdom (UK), Alfon et al. (Citation2005) found that size, risk, economic cycle, the value of assets were negatively related with capital ratios, while found actual individual capital requirements to be positively related to capital ratios for building societies and banks in the UK.
Hussain and Hassan (Citation2005) employed size, profitability, current provisions for loan losses to bad debts, bonds, liquidity, inflation, per capita GDP growth rate, foreign investment, level of financial development, regulatory pressure variable, and year dummy to analyze the relationship of regulatory capital requirements with capital ratios of the commercial banks. The results revealed that capital requirement regulations did not influence the capital ratios of commercial banks.
The factors that affect capitalization in developing countries are the same as the factors for developed countries (Booth et al., Citation2001). Theories of corporate financial leverage were tested using cross country comparison and the results show a persistent difference in factors of capital structure across countries which indicate that different country factors play a role in determining capital structure.
In their study, Shrieves and Dahl (Citation1992) found risk and capital variation to be positively related implying that the greater the risk the higher the capital held to safeguard against any uncertain event. Their finding is factual for banks that maintain a higher capital compared to the minimum regulatory requirements supporting the conclusion that bank principals and agents work for their self-interest to reduce the total risk exposure. The overall findings of the study support that due to the presence of risk-based variables affecting CAR, the capital held by banks also varies.
3. Data and method
3.1. Data
Data for the present empirical study is taken from multiple sources like the annual publications of banking data by the State Bank of Pakistan which includes accounts of scheduled commercial banks and the 5-year financial statement analysis published on an annual basis. The data related to capital adequacy ratio has been derived from the annual reports of individual commercial banks. The small balanced panel dataset consists of twenty conventional commercial banks, while 4 full-fledged Islamic commercial banks operating in Pakistan. Since the study has utilized a balanced panel for the entire sample period from 2006–2017, therefore, no missing values are present in the data of scheduled commercial banks.
Banks with missing data for the sample period, branches of foreign banks, and specialized banks were dropped from the analysis. The branches of foreign banks namely, the Citi Bank and the Deutsche bank were dropped for analysis for the study because the Citi bank has its branch operations only in Karachi, Lahore, and Islamabad, while the Deutsche bank has a single branch operation in Karachi only.
In addition to foreign banks, specialized banks namely, the Industrial Development Bank Limited (IDBL), the Punjab Provincial Cooperative Bank Limited (PPCBL), and the Zarai Taraqiati Bank Limited (ZTBL) is also dropped because of limited branch operations of IDBL in two cities only, the PPCBL is a Punjab centric bank, and ZTBL works for the development of the agriculture sector of Pakistan only.
The rationale for selecting 2006–2017 as the sample period in the current study is manifold first, the study intended to analyze the impact of the global financial crises of 2007–2008 on the capital adequacy ratio of Pakistani scheduled commercial banks and second secondary data for maximum scheduled banks were available for the sample period.
3.2. Methodology
3.2.1. Model specification
The following empirical model is estimated to test the relationship between the bank-specific, bank risk-based, and industry-specific variables that determine commercial banks’ capital adequacy ratio for Pakistani banks.
Where CARit denotes the banks’ capital adequacy ratio of bank i in year t and is the first lagged criterion variable.
represents the intercept term, while
and βk represents partial slope coefficients of the predictors used in the study; the explanatory variables categorized as bank-specific variables include management quality (BM), and the alternate cost of capital (ROE), while bank risk-based predictors include default risk (NPL), credit risk (LTA) and bank risk index proxied by the Z-score (Z). The dynamic panel model also contains HHI as a proxy for market competitiveness and the average CAR of the sector (PEER) constituting the industry-specific variables, and finally a dummy variable (DUMMY) for financial crises of 2007–08. µi denotes the unobservable bank-specific effects, while εit expresses the error term.
3.2.2. Estimation approach
To empirically test the regression model, EquationEquation (1)(1)
(1) is estimated by applying pooled ordinary least square (OLS), Fixed-Effects (FE) estimator, and dynamic panel regression analyses using the GMM estimator also used in previous empirical studies on banks’ capital adequacy ratio (Kalifa & Bektaş, Citation2018).
Extensive literature in the field of econometrics signifies that the estimation of panel regression equations based on the pooled OLS estimators lead to coefficient estimates of regressors that are biased and inconsistent because it does not consider the time-invariant unobserved individual bank effects (μi) as well as the possible endogeneity inherent in the lagged value of the criterion variable (Wintoki et al., Citation2012). In contrast to pooled OLS, the panel regression analysis is more advantageous as it helps researchers to overcome omitted variables issues (Studenmund & Johnson, Citation2017). The Random Effect (RE) and Fixed Effect (FE) estimators limit the researcher to use the observable information of previous periods in their panel models because they are static, whereas, the GMM estimator based on the dynamic panel models possesses the quality of using the past and the current information regarding the regression model. The FE estimator eliminates μi, but produces parameters that are biased in case T is fixed and the FE estimator like pooled OLS does not address the possible issues of endogeneity of the lagged criterion variable (Nickell, Citation1981). Therefore, to overcome the presence of endogenous explanatory variables in EquationEquation (1)(1)
(1) which may lead to estimation biases, a widely-used technique i.e. the Arellano-Bond (AB) GMM estimator will be used in the present study using STATA. The use of GMM tackles the existence of unobservable bank-specific effects by taking the first-lag of predictors presented in EquationEquation (1)
(1)
(1) . The use of GMM estimation also takes care of the probability of endogeneity issues in terms of regressors related with dynamic models.
The following section provides the empirical definitions of predictors and hypotheses formulated post extensive review of the literature.
3.3. Dependent variable
The commercial banks’ CAR is employed as the outcome variable in the study. Berger et al. (Citation1995), emphasized that banks would still hold capital even in an unregulated economy because the markets compel them to do so as a part of the market capitalization requirement. All countries practice the prevalence of regulated capital ratios by commercial banks because bank failures may prove contagious for the economy.
The following proxy is used to measure CAR:
3.4. Independent variables
The study employs eight predictors which are characterized by bank-specific factors, namely management quality (BM), and the alternative cost of capital (ROE). Further bank risk-related variables include default risk (NPL), credit risk (LTA), and bank risk index (Z). The industry-specific variables include the average CAR of banks (PEER) and a variable to proxy market concentration (HHI). Finally, a financial crisis dummy (DUMMY) is also used in the study.
3.4.1. Management quality (BM)
Management quality has been proxied by the ratio of a bank’s net interest margins in the present study. NIM is usually termed to be a key indicator of the bank’s level of efficiency in terms of its fundamental role in financial intermediation. Banks play an imperative role in a country’s economic growth by directing funds from the surplus units of the economy to the deficit ones. Due to the dual banking system in Pakistan, the present study used the term Bank Margins (BM) because Islamic banks which are a part of the sample banks work on their fundamental principle of interest-free banking. Bank margins are calculated as the difference between interest earned on loans and interest paid for deposits divided by total assets for conventional commercial banks, while calculated as the difference between markup earned and markup expensed divided by total assets for pure Islamic commercial banks in the present empirical research.
Banks are capable to increase their capital base with an increase in exposure to risk with the help of adequate bank margins to create sufficient income (Angbazo, Citation1997). According to the charter value theory by Keeley (Citation1990), there is a positive association of bank management quality proxied by NIM and capital held by banks, whereas Yu (Citation2000) argues that a more profitable bank has a lower chance of failure so bank management may decrease their capital buffers if they assume that risk of default has reduced, therefore, the relationship between bank margins and capital might be negative as hypothesized by Ahmad et al. (Citation2009). Romdhane (Citation2011) predicted a positive relationship of management quality with CAR whereas Ahmad et al. (Citation2009) found the relationship to be negative. Moussa (Citation2018) found an insignificant relation of management quality with CAR. Therefore, this study postulates a two-tailed hypothesis.
H1: Management quality is significantly associated with a bank’s CAR.
The following proxy is used to measure BM in this study:
3.4.2. Alternate cost of capital (ROE)
The second predictor employed in the study is the alternate cost of capital measured by ROE which is the most appropriate indicator for banks. Holding excess capital is costly for banks if the ROE is higher suggesting ROE and CAR be negatively related as found in many empirical studies (Büyüksalvarci & Abdioğlu, Citation2011; Francis & Osborne, Citation2010; Kalifa & Bektaş, Citation2018; Romdhane, Citation2011; Wong et al., Citation2008).
H2: The alternative cost of capital is significantly related to banks’ CAR.
The following proxy is used to measure alternative cost of capital in this study:-
3.4.3. Default risk (NPL)
The present study takes the ratio of NPL to measure default risk. The SBP calculates this ratio in the category of asset quality ratios. The higher the ratio the more risk faced by banks. It is suggested that a banks’ net worth decreases due to an increase in default risk and thus compelling banks maintain high CAR (Watanabe, Citation2010). Ahmad et al. (Citation2009) and Kalifa and Bektaş (Citation2018) also found a positive association of NPLs with CAR. Following their results, the present study will test the following hypothesis:
H3: Default risk is positively related to banks’ CAR.
The following proxy is used to measure default risk in this study:
3.4.4. Credit risk (CR)
The next risk-based factor employed in the study is credit risk following Büyüksalvarci and Abdioğlu (Citation2011), the hypothesis formulated for this predictor is:
H4: credit risk has a negative relationship with banks’ CAR.
3.4.5. Bank risk index (Z)
The third risk variable employed in the study is the bank risk index from Hannan and Hanweck (Citation1988) accounting model. The index is used by Ahmad et al. (Citation2009), in their empirical work as a factor in the determination of bank capital ratios. Ahmad et al. (Citation2009) hypothesized a negative association of bank risk index with the capital ratio of banks. This empirical study used the bank risk index as a predictor in the model by using the following proxy:
Where ROA represents the return on assets, EQTA is the ratio of total equity to total assets, and is the standard deviation of return on assets.
H5: Bank’s risk index has a negative relationship with a bank’s CAR.
3.4.6. Average CAR of banking industry (PEER)
According to Alfon et al. (Citation2005), high capital adequacy may be used by the banks as a signal to modify the sensitivity of creditors, investors, and credit rating companies in the market positively. In the Pakistani context Bokhari et al. (Citation2013), hypothesized a positive relationship of PEER with CAR and found the relationship to be insignificant.
Previous empirical studies have found a positive relationship of PEER with banks’ CAR (Alfon et al., Citation2005; Asarkaya & Özcan, Citation2007; Romdhane, Citation2011; Wong et al., Citation2008). Thus following hypothesis is formulated:
H6: The average CAR of the sector is positively associated with the bank’s CAR.
Following Alfon et al. (Citation2005), the following proxy is used to measure PEER in this study:
3.4.7. Market concentration (HHI)
HHI is taken as a proxy of market concentration of banks which can also depict the drift in the competitive structure of the banking industry. In this study, the HHI is calculated by summing the squares of the market share in terms of banking assets of each bank competing in the market.
H7: There is a significant association of the Herfindahl-Hirschman Index with banks’ CAR.
3.4.8. Financial crises dummy
A dummy variable to measure the effects of the global financial crisis is finally added to the model. The following hypothesis is formulated:
H8: The financial crisis has a negative relationship with banks’ CAR.
4. Empirical results and discussion
4.1. Descriptive statistics
Table presents the results of descriptive for the present study. Data for the period of 2006–2017 from a total of twenty-four scheduled banks operating in Pakistan making 288-panel observations. On average commercial banks hold a CAR of 18.8 % which is pretty higher than 11.875 % which is set as the minimum by SBP based on BASEL-III for the year 2017 and it is evident from results that the commercial banks of Pakistan are conservative and are holding additional capital buffers to counter any liquidity shocks (Table ).
Table 2. Descriptive Statistics
Table also reveals that the average CAR of the banking sector for the sample period is 18.6% which is slightly less than the average CAR with the minimum value of 15.4% to a maximum value of 22.2%. The return on equity, which is taken as a proxy for the alternate cost of capital has an average value of 8.1% for the sample period of study. The risk-based variables reveal that the risk of default (NPL) has an average value of 12.9 % with a standard deviation of 14.9 % indicating a significant variation across banks; the credit risk has an average value of 43.2 % with a 10.7 % standard deviation.
The Pairwise correlations results reported in Table reveals that management quality (BM), the alternate cost of capital (ROE), default risk (NPL), Herfindahl-Hirschman Index (HHI) is positively correlated to banks’ CAR, whereas the average CAR of the banking sector (PEER), credit risk and bank risk index are negatively correlated.
Table 3. Correlations
4.2. Estimation results
To empirically analyze the dynamic panel linear regression model, first, the pooled OLS estimator was applied to the data. Secondly, the unobserved effects in the data were accounted for by applying the FE static panel estimation method. The RE and FE estimators limit the researcher to use the observable information of previous periods in their panel models because they are static and to overcome the presence of endogenous explanatory variables in EquationEquation (1)(1)
(1) which may lead to estimation biases, a widely-used technique i.e. the Arellano-Bond (AB) GMM will be used in the present study using STATA to empirically test the regression model. The baseline findings reported in Table for the regression results are estimated using pooled OLS, FE, and the GMM estimators.
Table 4. Estimation Results
4.2.1. Empirical results from pooled OLS estimator
To check for stationarity in the data, Harris and Tzavalis (Citation1999) test is applied on the data and the results reveal that all predictors employed in the empirical model are stationary which indicates that OLS models are appropriate for the current study (Shrestha & Bhatta, Citation2018).
The results derived after applying the pooled OLS estimator are listed in column 2 Table . The findings for the bank-specific variables indicate that management quality and the banks’ alternate cost of capital has a positive association with banks’ CAR.
Among the bank risk-based variables, the default risk is predicting the bank’s CAR positively, while credit risk is negatively predicting the bank’s CAR. Finally, the bank risk index is insignificant in the model based on pooled OLS estimation.
The results for the industry-specific variables reveals that the average CAR of the banking sector has a negative association with the banks’ CAR, whilst, the proxy for market concentration, HHI has a significant positive relationship with banks’ CAR. At last, the financial crisis (2007–2008) dummy is negatively predicting the bank’s CAR.
4.2.2. Empirical results from fixed-effects estimator
To decide between the application of a pooled OLS and panel estimation technique, the Breusch and Pagan (Citation1980) test is applied. The result of the BP test indicates a chi-square test statistic value of 168.07 and a p-value 0.000, it is concluded that the variances across entities are different than zero indicating the existence of a panel effect and making it a consistent estimator for the present study. In comparison to OLS, the panel regression analysis is more advantageous as it helps researchers to overcome omitted variables issues (Studenmund & Johnson, Citation2017).
The Hausman (Citation1978) test is applied to the sample data to decide whether FE or RE estimator is preferred. The test results indicate that the FE method is appropriate for the current study [Chi-sq (7) = 36.38; p-value = .000]. The FE estimator is applied to control for time-invariant unobservable characteristics across banks.
A test proposed by Baum (Citation2013) was applied to check for heteroscedasticity in the FE estimator and the test results confirm the presence of heteroscedasticity. The Wooldridge (Citation2010) test of autocorrelation was then applied, and the results confirmed the presence of the first-order serial correlation in the model. Due to the presence of serial correlation and heteroscedasticity simultaneously in the model, clustered standard errors were chosen to analyze the model as it corrects for heteroscedasticity and serial correlation (Rogers, Citation1994).
The results derived after applying the FE estimations are presented in columns 3 of Table . The findings indicate that the banks’ alternate cost of capital has a positive association with banks’ CAR, while management quality is insignificant in the model among the bank-specific variables. The next set of variables categorized as the bank risk-based variables namely default risk and the bank risk index are all significantly predicting the banks’ capital adequacy ratio having a positive relationship, whereas, the credit risk is predicting banks’ CAR negatively using the FE estimator.
Among the industry-specific variables, the average CAR of the banking sector has a negative relationship with the banks’ CAR, while the HHI is positively associated with the banks’ capital adequacy ratio. The financial crisis (2007–08) dummy is negatively predicting the bank’s CAR as hypothesized.
4.2.3. Empirical results from GMM estimator
The analysis based on testing EquationEquation (1)(1)
(1) using the AB GMM approach is reported in column 4 of Table . The results confirm the correct specification of the model as the values of the autoregressive parameter is less than 0.8 (Moshirian & Wu, Citation2012). The assumption of no autocorrelation in the errors in the levels is tested and the results reveal that the null hypothesis of no second-order autocorrelation is not rejected as suggested by the p-value of 0.326 which is less than 0.05 for AR (2), and it is evident that the assumptions of the AB model are satisfied and the application of a dynamic panel model is validated by the results. Table reveals that the partial slope coefficient of the lagged CAR of banks has a positive relationship with the banks’ CAR at a 1-percent level of significance, which implies that the lag capital adequacy ratio has an impact on the current capital adequacy.
For the bank-specific variables, the banks’ alternate cost of capital has a positive association with banks’ CAR, while management quality is found insignificant in the model estimated based on the GMM. Among the bank risk-based variables, the default risk has a positive relationship with banks’ CAR, credit risk has a negative significant relationship with the bank’s CAR, while the bank risk index is insignificant in the model.
The average CAR of the banking sector categorized as the industry-specific variable has a negative relationship with the banks’ CAR, while HHI proxied for measuring market concentration has a positive relationship with CAR. Finally, the financial crises dummy is significantly predicting CAR negatively based on the GMM estimator.
4.3. Discussion
The first two hypotheses were based on testing the relationship of bank-specific variables with the banks’ CAR. The first hypothesis tested the relationship of management quality with the banks’ CAR. The findings for the bank-specific variables based on the pooled OLS estimator indicate that the partial slope coefficient of management quality has a positive sign indicating a positive association with CAR at a 1% level of significance (β = 1.865; p-value = .000). The results are consistent with several previous empirical works (Hewaidy & Alyousef, Citation2018; Moussa, Citation2018; Romdhane, Citation2011). Management quality is insignificant based on the model estimated using the FE and GMM estimators. It is evident from columns 2 and 3 of Table that the statistical significance of the estimated partial slope coefficient on management quality vanishes when unobserved bank-effects are considered implying that the result derived from the pooled OLS estimation method is probably determined by omitted bank-level characteristics.
The second hypothesis was formulated to test the relationship of the alternate cost of capital proxied by the return on equity with the banks’ CAR. The alternate cost of capital ROE was statistically significant across pooled OLS, FE, and GMM estimation techniques as depicted in columns 2, 3, and 4 of Table . The banks’ alternate cost of capital (ROE) has a positive association with banks’ CAR at a 5% significance level (β = 0.007; p-value = .037) based on the results of the pooled OLS estimator, whereas, it is statistically significant at a 10% and 1% level of significance based on the FE (β = 0.003; p-value = .067) and GMM (β = 0.016; p-value <.01) estimators respectively. The result is consistent with previous empirical studies that found a positive association of ROE with banks’ CAR (Alfon et al., Citation2005; Asarkaya & Özcan, Citation2007). The significance of the relationship has improved by applying the GMM estimator in comparison to the pooled OLS and FE estimator for alternate cost of capital.
Hypothesis 3 is formulated to empirically examine the relationship of default risk with the banks’ CAR tested in the third hypothesis and a positive significant relationship is derived across pooled OLS, FE, and GMM estimators. The default risk proxied by the ratio of non-performing loans to total loans is predicting the bank’s CAR positively at a 1% significance level (β = 0.395; p-value = .000) based on the results of the pooled OLS estimator, 10% significance level (β = 0.178; p-value = .09) based on the results of the FE estimator, and is statistically significant at a 5% level (β = 0.247; p-value <.05) based on the GMM estimator results for the sample period of study. The result is consistent with the empirical studies of Ahmad et al. (Citation2009) and Kalifa and Bektaş (Citation2018).
The fourth hypothesis is constructed to identify the relationship of credit risk with banks’ CAR. The estimation results in Table reveal a negative significant relationship of credit risk with banks’ capital adequacy ratio across pooled OLS, FE, and GMM estimators. Credit risk is statistically significant at a 1% level based on the pooled OLS estimator (β = −0.384; p-value = .000), the FE estimator (β = −0.221; p-value = .006), and the GMM estimator (β = −0.165; p-value <.01) simultaneously. The result implied that credit risk like any risk will hinder the CAR and the finding is consistent with that of Büyüksalvarci and Abdioğlu (Citation2011) in the Turkish context.
The empirical relationship of the last bank risk-based predictor, the Hannan and Hanweck (Citation1988) bank risk index is tested in the fifth hypothesis. The predictor is insignificant based on a pooled OLS and GMM estimate, while it is significantly predicting banks’ CAR positively using the FE estimator at a 1% level of significance (β = 0.005; p-value = .000). The result based on the FE estimator is different as compared to the study conducted by Ahmad et al. (Citation2009), who found a negative relationship of bank risk index with CAR in the Malaysian context.
The industry-specific variables, average CAR of the banking sector (PEER) and HHI to proxy for market concentration are tested in hypotheses 6 and 7. The average CAR is predicting banks’ CAR negatively, whereas, HHI has a positive relationship with banks’ CAR. Both the predictors are statistically significant across all estimation techniques (pooled OLS, FE, and GMM).
The results for the average CAR of the banking sector in column (2) of Table based on pooled OLS estimate reveal that the average CAR of the banking sector (PEER) has a negative association with the banks’ CAR at a 1% significance level (β = −2.979; p-value = .000), column (3) of Table indicates that PEER is statistically significant at a 5% level of significance (β = −1.618; p-value = .023) based on the FE and the GMM estimator (β = −0.65; p-value <.05).
For measuring market concentration, the Herfindahl-Hirschman index was used in the model. HHI is statistically significant at a 1% level (β = 0.240; p-value = .000) based on a pooled OLS estimator, 5% level of significance (β = 0.108; p-value = .023) based on the FE estimator, while significant at a 10% level (β = 0.113; p-value <.10) based on the GMM estimation results.
The present research study employed a dummy variable to assess the effects of the global financial crisis 2007–08 in the dynamic panel model in EquationEquation (1)(1)
(1) . The financial crisis dummy has a negative association with banks’ CAR across all estimation methods. It is statistically significant a 1% significance level based on the results of the pooled OLS (β = −0.049; p-value = .000), and the FE estimator (β = −0.019; p-value = .008), whilst, the financial crisis (2007–2008) dummy is statically significant at a 5% level based on the results of the GMM estimator. The finding conforms to the empirical work of Kalifa and Bektaş (Citation2018). During the sample period of study 2006–2017, it is evident from the results that the commercial banks of Pakistan are conservative and are holding additional capital buffers to counter any liquidity shocks.
5. Conclusions and recommendations
This research investigates the capital structure determinants of Pakistani scheduled commercial banks which operate in a dual banking system since 2002 and are considered to be an integral part of the financial system and the overall economy. Despite inconsistent policies, the banking industry of Pakistan is one of the top growing sectors of the economy.
The current study analyzed the bank-specific, the bank risk-based, and industry-specific determinants of banks’ CAR. The dynamic panel regression model also incorporated a dummy variable to check the effects of the global financial crises on the capital adequacy of commercial banks operating in Pakistan. No extensive study has been carried out in Pakistan regarding the conundrum of bank structure even after the importance this sector has in the country’s economic growth as well as the recent financial crisis the sector has faced globally. This area of study has received great attention after the world banking liquidity crisis across the globe, but still many countries remain unexplored and there is much room for the topic for research studies to be conducted on.
The results derived after applying the pooled OLS estimator indicate that management quality, the banks’ alternate cost of capital, the default risk, credit risk, the average CAR of the banking sector, the HHI, and the financial crisis (2007–2008) dummy significantly predicting the banks’ capital adequacy ratio, whereas, the bank risk index is insignificant in the model.
The results derived after applying the FE estimation technique indicates that the banks’ alternate cost of capital, default risk, credit risk, the bank risk index, the average CAR of the banking sector, the HHI, and the financial crisis (2007–08) dummy are amongst the significant predictors of banks’ CAR. The management quality is insignificant in the model among the bank-specific variables.
The findings based on the results of the GMM estimator depict that among the bank-specific variables, only the alternate cost of capital is a significant determinant of CAR which aligns with the findings of previous researchers (Alfon et al., Citation2005; Asarkaya & Özcan, Citation2007). The management quality is found to be statistically insignificant in the dynamic panel model. For the bank risk-based variables, default risk, and credit risk are statistically significant with banks’ CAR, while the bank risk index is insignificant. Default risk has a positive relationship with banks’ CAR for the sample period of study which is consistent with the result of previous empirical works of Ahmad et al. (Citation2009) and Kalifa and Bektaş (Citation2018). The credit risk has a negative significant relationship with the bank’s CAR and the finding is in line with that of Büyüksalvarci and Abdioğlu (Citation2011) in the Turkish context.
Among the industry-specific variables, the average CAR of the banking sector and the market competitiveness proxied by the Herfindahl-Hirschman Index (HHI) is significantly predicting CAR. Moreover, the dummy variable for the global financial crisis is also found significant in the current study and the finding conforms to the empirical work of Kalifa and Bektaş (Citation2018).
5.1. Summary of hypotheses tested
Table presents a summary of the hypotheses formulated in the study. The present research study tested 8 hypotheses in total, 6 of them were accepted (significant), while 2 hypotheses were not accepted based on the GMM estimator.
Table 5. Summary of Hypotheses Tested
5.2. Policy recommendations
The levels of capital adequacy ratio epitomize the extent of resilience banks possess to take care of idiosyncratic risks and it provides a shield to the banking sector against failing investments and unpredicted events. Policymakers and regulators working at the State Bank of Pakistan are regulating banks with the implementation of BASEL-III through a phase-in arrangement in Pakistan after the Basel Committee for the banking sector updated regulations.
The government should continuously regulate the banking industry to ensure that banks are maintaining the minimum capital requirements set by the SBP on the guidelines laid in the BASEL-III framework. This will boost investor confidence in the banking sector and enhance investment in the industry.
The data suggest that Pakistani banks have devoted more funds towards maintaining the capital adequacy ratio as on average commercial banks are holding a CAR significantly higher compared to the benchmark value set by SBP based on BASEL-III indicating that the commercial banks of Pakistan are conservative and are holding additional capital buffers to counter any liquidity shocks.
The empirical findings of the present study have imperative implications for regulators, policymakers, investors, and bank management. Based on the results derived, the author recommends that first of all, the management of banks must focus on enhancing their net income which will increase the return on equity and enable the banks to maintain the minimum CAR. These measures will help attract depositors and investors of the banking sector to shape their investment decisions.
Second, commercial banks must ensure a cutback in their default risk appetite because a non-performing loan is termed as being close to default or is in default leading to reduce the banks’ net income as well as compel the banks to hold more funds as loan loss reserves to cater the default risk as a part of their lending strategies. Banks must establish a robust mechanism to appraise the creditworthiness of customers to minimize the likelihood of customer default.
Finally, the bank managers should devote more resources to attracting deposits from potential and current customers as they are a cheap funding source. The greater the deposit base banks hold, the greater will be the chance to achieve more net income.
5.3. Future research
The financial sector of Pakistan comprises several groups of actors that jointly constitute the Pakistani financial sector. The author recommends that future studies may be directed towards empirically analyzing the factors affecting the capital structure of the residual players of the financial sector, for example, Development Finance Institutions (DFIs), insurance companies, microfinance banks, investment banks, leasing companies, and Modaraba companies operating in Pakistan.
Furthermore, empirical studies in the future may be focused on for the banking sectors in the Asian context which lack empirical research in the area of capital structure. Country-specific empirical research can be done in the Pakistani context by focusing on the macroeconomic variables affecting bank capital structure.
Additional information
Funding
Notes on contributors
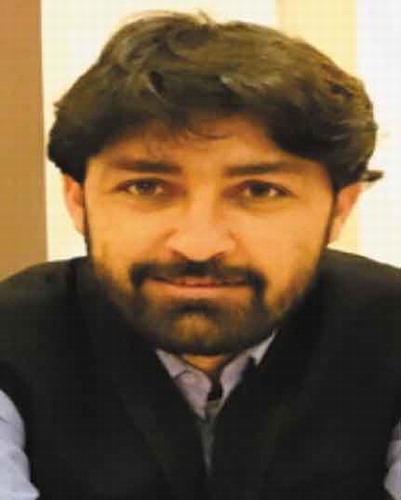
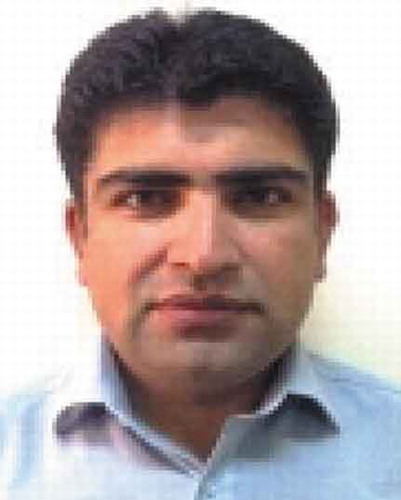
Bilal Sarwar
Dr. Bilal Sarwar is a Ph.D. in Finance and currently working as an Assistant Professor at the Department of Management Sciences Balochistan University of Information Technology, Engineering and Management Sciences (BUITEMS) Quetta, Pakistan. His areas of interest include Banking and Finance, Financial Econometrics, and Corporate Finance. (Email: [email protected]).
Dr. Noor Muhammad earned his Ph.D. in Finance from The University of Waikato, New Zealand. He is currently working as an Associate Professor at the Department of Management Sciences, BUITEMS, Quetta, Pakistan. He has published research papers in renowned journals like the Journal of Cleaner Production, Journal of Business Ethics, Corporate Social Responsibility & Environmental Management, and Financial Innovation.
Dr. Nadeem Uz Zaman is an Assistant Professor at the Department of Management Sciences, BUITEMS, Quetta, Pakistan.
Zia Ur Rehman is an Assistant Professor at the Department of Management Sciences, BUITEMS, Quetta, Pakistan.
Notes
1. Meezan Bank Limited was the first Islamic bank of Pakistan established in 2002.
References
- Abusharba, M. T., Triyuwono, I., Ismail, M., & Rahman, A. F. (2013). Determinants of capital adequacy ratio (CAR) in Indonesian Islamic commercial banks. Global Review of Accounting and Finance, 4(1), 159–20. http://docplayer.net/35761294-Determinants-of-capital-adequacy-ratio-car-in-indonesian-islamic-commercial-banks.html
- Ahmad, R., Ariff, M., & Skully, M. J. (2009). The determinants of bank capital ratios in a developing economy. Asia-Pacific Financial Markets, 15(3–4), 255–272. https://doi.org/10.1016/S0378-4266(96)00025-8
- Alfon, I., Argimón, I., & Bascuñana-Ambrós, P. (2005). How individual capital requirements affect capital ratios in UK banks and building societies. BANCO DE ESPAÑA, Madrid. https://repositorio.bde.es/bitstream/123456789/6828/1/dt0515e.pdf
- Angbazo, L. (1997). Commercial bank net interest margins, default risk, interest-rate risk, and off-balance sheet banking. Journal of Banking & Finance, 21(1), 55–87. https://doi.org/10.1016/S0378-4266(96)00025-8
- Asarkaya, Y., & Özcan, S. (2007). Determinants of capital structure in financial institutions: The case of Turkey. Journal of BRSA Banking and Financial Markets, 1(1), 91–109. https://doi.org/10.1.1.605.2556
- Athanasoglou, P., Brissimis, S., & Delis, M. (2008). Bank-specific industry-specific and macroeconomic determinants of profitability. Journal of International Financial Markets, Institutions and Money, 18(2), 121–136. https://doi.org/10.1016/j.intfin.2006.07.001
- Bambang, S., Elen, P., Yeye, S., Sri, S., & Udin, U. (2019). Determinants of capital adequacy ratio: An empirical study on the conventional banks in Indonesia. International Journal of Innovation, Creativity and Change, 9(7), 115–127. https://www.ijicc.net/images/vol9iss7/9709_Sudiyatno_2019_E_R.pdf
- Baum, C. (2013). Modified Wald statistic for groupwise heteroskedasticity in fixed effect model. dokument za pomoč v programskem paketu STATA.
- Berger, A. N., Herring, R. J., & Szegö, G. P. (1995). The role of capital in financial institutions. Journal of Banking & Finance, 19(3–4), 393–430. https://doi.org/10.1016/0378-4266(95)00002-X
- Bichsel, R., & Blum, J. (2004). The relationship between risk and capital in Swiss commercial banks: A panel study. Applied Financial Economics, 14(8), 591–597. https://doi.org/10.1080/0960310042000233881
- Bokhari, I. H., Ali, S. M., & Sultan, K. (2013). Determinants of capital adequacy ratio in banking sector: An empirical analysis from Pakistan. Academy of Contemporary Research Journal, 2(1), 1–9. http://citeseerx.ist.psu.edu/viewdoc/summary?doi=10.1.1.403.4663
- Booth, L., Aivazian, V., Demirguc‐Kunt, A., & Maksimovic, V. (2001). Capital structures in developing countries. The Journal of Finance, 56(1), 87–130. https://doi.org/10.1111/0022-1082.00320
- Breusch, T. S., & Pagan, A. R. (1980). The Lagrange multiplier test and its applications to model specification in econometrics. The Review of Economic Studies, 47(1), 239–253. https://doi.org/10.2307/2297111
- Büyüksalvarci, A., & Abdioğlu, H. (2011). Determinants of capital adequacy ratio in Turkish Banks: A panel data analysis. African Journal of Business Management, 5(27), 11199–11209. https://doi.org/10.5897/AJBM11.1957
- Diamond, D. W., & Dybvig, P. H. (1983). Bank runs, deposit insurance, and liquidity. Journal of Political Economy, 91(3), 401–419. https://doi.org/10.1086/261155
- Dietrich, A., & Wanzenried, G. (2011). Determinants of bank profitability before and during the crisis: Evidence from Switzerland. Journal of International Financial Markets, Institutions and Money, 21(3), 307–327. https://doi.org/10.1016/j.intfin.2010.11.002
- El-Ansary, O., & Hafez, H. (2015). Determinants of capital adequacy ratio: An empirical study on Egyptian banks.
- Fiordelisi, F., Marques-Ibanez, D., & Molyneux, P. (2011). Efficiency and risk in European banking. Journal of Banking & Finance, 35(5), 1315–1326. https://doi.org/10.1016/j.jbankfin.2010.10.005
- Francis, W. B., & Osborne, M. (2010). On the behavior and determinants of risk‐based capital ratios: Revisiting the evidence from UK banking institutions. International Review of Finance, 10(4), 485–518. https://doi.org/10.1111/j.1468-2443.2010.01112.x
- Frank, M. Z., & Goyal, V. K. (2009). Capital structure decisions: Which factors are reliably important? Financial Management, 38(1), 1–37. https://doi.org/10.1111/j.1755-053X.2009.01026.x
- Gropp, R., & Heider, F. (2007). What can corporate finance say about banks’ capital structures.
- Hahn, P. J. (1966). Factors determining adequacy of capital in commercial banks. The Journal of Finance, 21(1), 135–136. https://doi.org/10.1111/j.1540-6261.1966.tb02969.x
- Hannan, T. H., & Hanweck, G. A. (1988). Bank insolvency risk and the market for large certificates of deposit. Journal of Money, Credit, and Banking, 20(2), 203–211. https://doi.org/10.2307/1992111
- Harris, R. D., & Tzavalis, E. (1999). Inference for unit roots in dynamic panels where the time dimension is fixed. Journal of Econometrics, 91(2), 201–226. https://doi.org/10.1016/S0304-4076(98)00076-1
- Hausman, J. A. (1978). Specification tests in econometrics. Econometrica: Journal of the Econometric Society, 46(6), 1251–1271. https://doi.org/10.2307/1913827
- Hewaidy, A. M., & Alyousef, H. Y. (2018). Bank-specific and macroeconomic determinants of capital adequacy ratio: Evidence from Kuwaiti banks. European Journal of Economics, Finance and Administrative Sciences, (99), 5-20. https://www.europeanjournalofeconomicsfinanceandadministrativesciences.com/issues/PDF/EJEFAS_99_01.pdf
- Hovakimian, A., & Kane, E. J. (2000). Effectiveness of capital regulation at US commercial banks, 1985 to 1994. The Journal of Finance, 55(1), 451–468. https://doi.org/10.1111/0022-1082.00212
- Hovakimian, A., Kane, E. J., & Laeven, L. (2003). How country and safety-net characteristics affect bank risk-shifting. Journal of Financial Services Research, 23(3), 177–204. https://doi.org/10.1023/A:1024699811875
- Hussain, M. E., & Hassan, M. K. (2005). Basel capital requirements and bank credit risk taking in developing countries. Department of Economics and Finance Working Papers, 1991–2006, 1–40. https://scholarworks.uno.edu/econ_wp/34/
- Inderst, R., & Mueller, H. M. (2008). Bank capital structure and credit decisions. Journal of Financial Intermediation, 17(3), 295–314. https://doi.org/10.1016/j.jfi.2008.02.006
- Kahane, Y. (1977). Capital adequacy and the regulation of financial intermediaries. Journal of Banking & Finance, 1(2), 207–218. https://doi.org/10.1016/0378-4266(77)90007-3
- Kalifa, W., & Bektaş, E. (2018). The impacts of bank-specific and macroeconomic variables on the capital adequacy ratio: Evidence from Islamic banks. Applied Economics Letters, 25(7), 477–481. https://doi.org/10.1080/13504851.2017.1340559
- Keeley, M. C. (1990). Deposit insurance, risk, and market power in banking. The American Economic Review, 80(5), 1183–1200. https://www.jstor.org/stable/2006769
- Klepczarek, E. (2015). Determinants of European banks’ capital adequacy. Comparative Economic Research, 18(4), 81–98. https://econpapers.repec.org/article/vrscoecre/v_3a18_3ay_3a2015_3ai_3a4_3ap_3a81-98_3an_3a5.htm
- Koehn, M., & Santomero, A. M. (1980). Regulation of bank capital and portfolio risk. The Journal of Finance, 35(5), 1235–1244. https://doi.org/10.2307/2327096
- Levine, R. (1999). Financial development and economic growth: Views and agenda. World Bank Group.
- Masood, U., & Ansari, S. (2016). Determinants of capital adequacy ratio: A perspective from Pakistani banking sector. International Journal of Economics, Commerce and Management, 4(7), 247–273. http://ijecm.co.uk/wp-content/uploads/2016/07/4716.pdf
- Merton, R. C., & Bodie, Z. (1995). A conceptual framework for analyzing the financial system The global financial system: A functional perspective. Harvard Business School Press.
- Mili, M., Sahut, J.-M., Trimeche, H., & Teulon, F. (2017). Determinants of the capital adequacy ratio of foreign banks’ subsidiaries: The role of interbank market and regulation. Research in International Business and Finance, 42, 442–453. https://doi.org/10.1016/j.ribaf.2016.02.002
- Moshirian, F., & Wu, Q. (2012). Banking industry volatility and economic growth. Research in International Business and Finance, 26(3), 428–442. https://doi.org/10.1016/j.ribaf.2012.01.004
- Moussa, M. A. B. (2018). Determinants of bank capital: Case of Tunisia. Journal of Applied Finance and Banking, 8(2), 1–15. http://www.scienpress.com/Upload/JAFB/Vol%208_2_1.pdf
- Nickell, S. (1981). Biases in dynamic models with fixed effects. Econometrica: Journal of the Econometric Society, 49(6), 1417–1426. https://doi.org/10.2307/1911408
- Pakistan Economic Survey. (2017–18). The Ministry of Finance, Government of Pakistan. http://www.finance.gov.pk/survey/chapters_18/Economic_Survey_2017_18.pdf
- Rogers, W. (1994). Regression standard errors in clustered samples. Stata Technical Bulletin, 3(13). https://www.stata.com/products/stb/journals/stb13.pdf
- Romdhane, M. (2011). The Determinants of Banks’ Capital Ratio in Developing Countries: Empirical Evidence from Tunisia. European Journal of Business and Management, 3(11), 52–64. https://www.iiste.org/Journals/index.php/EJBM/article/view/715/614
- SBP. (2013). Guidelines on basel III implementation in Pakistan. Banking Policy & Regulations Department, State Bank of Pakistan. http://www.sbp.org.pk/bsrvd/pdf/DCGuidelines/Draft%20Basel%203%20Guidelines%20(BPC).pdf
- Schaeck, K., & Cihak, M. (2012). Banking competition and capital ratios. European Financial Management, 18(5), 836–866. https://doi.org/10.1111/j.1468-036X.2010.00551.x
- Shrestha, M. B., & Bhatta, G. R. (2018). Selecting appropriate methodological framework for time series data analysis. The Journal of Finance and Data Science, 4(2), 71–89. https://doi.org/10.1016/j.jfds.2017.11.001
- Shrieves, R. E., & Dahl, D. (1992). The relationship between risk and capital in commercial banks. Journal of Banking & Finance, 16(2), 439–457. https://doi.org/10.1016/0378-4266(92)90024-T
- Studenmund, A. H., & Johnson, B. K. (2017). A practical guide to using econometrics. Pearson Education Limited.
- Thoa, P. T. X., Anh, N. N., & Minh, N. K. (2020). The determinant of capital adequacy ratio: Empirical evidence from Vietnamese banks (a panel data analysis). Afro-Asian Journal of Finance and Accounting, 10(1), 60–70. https://doi.org/10.1504/AAJFA.2020.104406
- Van Oudheusden, P., Klapper, L., Demirguc-Kunt, A., & Singer, D. (2015). The global findex database 2014: Measuring financial inclusion around the world. World Bank.
- Watanabe, W. (2010). Does a large loss of bank capital cause evergreening? Evidence from Japan. Journal of the Japanese and International Economies, 24(1), 116–136. https://doi.org/10.1016/j.jjie.2010.01.001
- Wintoki, M. B., Linck, J. S., & Netter, J. M. (2012). Endogeneity and the dynamics of internal corporate governance. Journal of Financial Economics, 105(3), 581–606. https://doi.org/10.1016/j.jfineco.2012.03.005
- Wong, J., Choi, K.-F., & Fong, T. P.-W. (2008). Determinants of the capital level of banks in Hong Kong. Palgrave Macmillan Studies in Banking and Financial Institutions: Palgrave Macmillan.
- Wooldridge, J. M. (2010). Econometric analysis of cross section and panel data. MIT press.
- Yu, H. C. (2000). Banks’ capital structure and the liquid asset–policy implication of Taiwan. Pacific Economic Review, 5(1), 109–114. https://doi.org/10.1111/1468-0106.00093