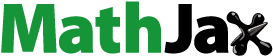
Abstract
The sluggish and sometimes negative growth in sub-Saharan Africa has defined the objectives of most studies seeking to explain the sources of its slow growth. I contribute to this inquiry by estimating how state fragility influences the effect of exchange rate misalignment on economic growth. Since exchange rate misalignment captures the distortionary effects of inappropriate macroeconomic policies in the main, my hypothesis is that resilient and less fragile states cope better with macroeconomic imbalances making misaligned exchange rates less likely to have serious effects on growth in such countries. In testing this hypothesis, I first measure misalignment as deviations of the actual exchange rate from an estimated equilibrium level using the dynamic ordinary least squares method. I then insert this variable and its interaction with state fragility in a growth specification. In line with my hypothesis, results from the system generalised method of moments and data on 13 sub-Saharan countries observed between 2009 and 2018 show a significantly negative effect of exchange rate misalignment on growth that increases with state fragility. Based on this evidence, I urge countries in this region to improve state resilience as an effort to reduce the negative effect of exchange rate misalignment on economic growth.
PUBLIC INTEREST STATEMENT
Generating sustained growth in sub-Saharan Africa remains a developmental challenge and a necessity for alleviating extreme poverty in the region. This policy challenge has occupied the hearts and minds of academic researchers interested in growth and development in Africa. Much of the research attention in sub-Saharan Africa has however gone to factors such as limited investment, terms of trade volatility, external debt, and inappropriate economic policies. In this paper, I focus primarily on the effect of real exchange rate misalignment on economic growth in this region and proceed to test how state fragility influences this relationship. Fragile states are generally marred by weak governance, conflict, weak institutions, and lack of effective public service delivery by the state. I provide evidence that real exchange rate misalignment is likely to have a more severe effect in countries exhibiting these features.
1. Introduction
Sub-Saharan Africa (SSA) has a poor record of economic performance and continues to be one of the poorest regions of the world. Most countries in this region record slow, if not negative, growth in real per capita income and are exposed to extreme poverty. Owing to this economic calamity, several scholars from both sides of the Atlantic have dedicated time and effort to understand sources of its slow growth in the last three or so decades. Common explanations for the region’s economic mishap range from the effect of drought, poor macroeconomic policies, adverse terms of trade shocks, corruption, and limited foreign credit to weak institutions. Ghura and Grennes (Citation1991), an influential paper by citations in particular, attributes SSA’s abysmal performance largely to high levels of exchange rate misalignment; a conclusion that is now shared by many including the International Monetary Fund (IMF).
Several recent studies have confirmed the same narrative of a negative relationship between exchange rate misalignment and economic growth at the empirical quantitative level. These studies include, but are not limited to, Elbadawi et al. (Citation2012), Toulaboe (Citation2011), Dai et al. (Citation2017), and Jehan and Irshad (Citation2020). Interesting from these studies is the growing tendency to explore macroeconomic factors that moderate and ameliorate the negative effects of exchange rate misalignment. This tendency represents a shift from earlier studies of estimating the direct effect of chronic exchange rate misalignment on economic growth oblivious of moderating factors. Elbadawi et al. (Citation2012) and Jehan and Irshad (Citation2020) in particular acknowledge the importance of moderating factors by primarily focusing on the role of financial sector development in the context of sub-Saharan Africa and Pakistan, respectively. A conclusion reached in these two studies is that a robust financial sector mitigates the detrimental effect of exchange rate misalignment on economic growth.
The explanation is generally that financial sector developments provide better hedging tools to safeguard against the risk and uncertainty attached with real exchange rate misalignment. While credit is due for this empirically tested explanation, there still remains an empirical gap as far as understanding the moderating factors of exchange rate misalignment is concerned. In particular, there is a strong possibility that state fragility can dictate and influence the way exchange rate misalignment hampers economic performance. In the main, state fragility refers to weak or failing government structures and to situations where the social contract is broken due to the state’s incapacity or unwillingness to deal with its basic functions such as responsibilities regarding service delivery, management of resources, rule of law, equitable access to power, security and safety of the populace and protection and promotion of citizens’ rights and freedoms. Accordingly, fragile states have characteristics that substantially impair their economic and social performance. These include weak governance, limited administrative capacity, chronic humanitarian crises, persistent social tensions, and often, violence or the legacy of armed conflict and civil war.
On the other hand, real exchange rate misalignment measures how the actual real exchange rate (RER) deviates from an ideal equilibrium level dictated by macroeconomic fundamentals. It primarily comes in two forms namely undervaluation which occurs when the actual RER falls below the equilibrium level (Özyurt, Citation2013) and overvaluation which occurs when the actual RER lies above the equilibrium level (Abida, Citation2011; Béreau et al., Citation2012; Glüzmann et al., Citation2012). In countries pursuing flexible exchange rate policies, real exchange rate misalignment captures the extent to which authorities (or the market itself) respond to correct external and internal shocks that may force the real exchange rate to wander away from its equilibrium value. Under pegged exchange rate systems, however, it demonstrates the limited ability of monetary authorities to use the exchange rate as a policy instrument to address economic disequilibrium, therefore, causing real exchange rate misalignment.
This paper improves existing literature in two important ways. First, it contributes by estimating the impact of real exchange rate misalignment on growth at different levels of state fragility in SSA. My conjecture is that real exchange rate misalignment can have varying effects on economic growth at different levels of state fragility. For lower levels of state fragility, for example, the intuition is that real exchange rate misalignment may have a miniscule effect on economic growth since the government has some discernible capacity to intervene in the exchange rate market and defend the local currency. For higher levels of state fragility, government, and policymakers in particular are generally inept to make an effective policy intervention hence a real exchange rate misalignment is capable of having severe effects on economic growth. Given the stark lack of studies exploring this intuitive inquiry, this paper seeks to contribute to the existing body of knowledge by estimating the interactive effect of real exchange rate misalignment and state fragility on economic growth in SSA. Second, it uses recent data by focusing exclusively on the 2010–2018 sampling period. This sampling period is crucial in so far as it allows us to capture the relationship between real exchange rate misalignment, state fragility and economic growth in sub-Saharan Africa post the 2008/09 global financial crisis.
Sub-Saharan Africa is a region well known for widespread conflict, weak governance, civil wars, humanitarian crises, and poor service delivery which are all ingredients of state fragility. It is also a region that has witnessed chronic exchange rate misalignment over the past three decades (see Elbadawi et al., Citation2012). Since by definition, a country’s fragility is closely associated with, albeit partly, its state capacity to make and implement public policy and respond effectively to challenges and crises, it is crucial to establish whether or not the effect of exchange rate misalignment increases or decreases with state fragility.
In the main, exchange rate misalignment generally hampers economic growth primarily through the erosion of a country’s economic competitiveness (Mazorodze & Tewari, Citation2018). An overvalued real exchange rate in particular makes a country’s exports expensive and imports cheaper leading to a negative trade balance. A misaligned exchange rate also leads to capital flight, reduced investment, currency crises and unemployment (Abida, Citation2011; Couharde & Sallenave, Citation2013; Razin & Collins, Citation1997; Yan & Yang, Citation2012). The question asked in this paper is, is this potentially negative effect of exchange rate misalignment on growth worse in countries that are fragile and therefore incapable of making effective policy interventions to crises? If, as argued by Ebaidalla (Citation2014), exchange rate misalignment captures the consequences of distortionary monetary and exchange rate policies, are fragile states therefore less likely to make effective policy interventions required to correct these distortions?
In answering the above question, I benefit heavily from two strands of literature. The first strand includes studies on exchange rate misalignment and economic growth. These studies (Dubas, Citation2009; Elbadawi et al., Citation2012; Razin & Collins, Citation1997; Sallenave, Citation2010; Toulaboe, Citation2011), essentially measure the values of exchange rate misalignment and subsequently plug them in a growth specification as an explanatory variable. In the majority of cases, a negative effect of exchange rate misalignment on economic performance is confirmed. The second strand of literature which includes Chuku and Onye (Citation2019) treats state fragility as a determinant of growth. Since I rely on these two strands of literature, the study’s methodology section is systematically designed. In particular, it begins with measuring exchange rate misalignment and later turns to state fragility.
Cognisant of the fact that exchange rate misalignment and state fragility can be causes or consequences of slow growth, I methodologically rely on the System Generalized Method of Moments (SysGMM). This estimator deals with endogeneity of this sort in the cases of large N and fixed T panel datasets. The results are both interesting and revealing. I am able to replicate the harmful effect of exchange rate misalignment on economic growth observed in Elbadawi et al. (Citation2010) for sub-Saharan Africa. I proceed to show, however, that the negative effect is less severe in countries that are relatively less fragile and is more pronounced in fragile states. This latter result is crucial in so far as it demonstrates the importance of state resilience in mitigating the negative effects of real exchange rate misalignment on economic growth in sub-Saharan Africa. From a policy perspective, the moderating effect of state resilience calls for countries in this region to pay a close attention to factors behind state resilience (or lack thereof). From the literature, these factors include peace, inclusive politics, and effective governance. In particular, it is essentially the establishment of inclusive political arrangements, strengthening of institutions, and fostering of investment that primarily achieve state resilience.
The rest of the paper is organised as follows: Section 2 provides the theoretical model used to estimate the equilibrium real exchange rate and its associated misalignment values. The empirical models are specified in Section 3 while results are presented and discussed in Section 4. Section 5 then provides some concluding remarks.
2. Data Description and model specification
I have data on 13 sub-Saharan countries, namely, Burundi, Cote d’Ivoire, Cameroon, Gabon, Ghana, Gambia, Equatorial Guinea, Lesotho, Malawi, Nigeria, Togo, Uganda, and South Africa observed between 2010 and 2018. Selection of the sampling period and the 13 countries is solely based on data availability. All data used in this paper are sourced from the World Development Indicators (WDI).
The entry point of the paper derives the model used to measure exchange rate misalignment. Generally, studies have measured exchange rate misalignment using either the purchasing power parity approach (PPP), the black-market premium approach, or the equilibrium model approach. In the PPP framework, the change in the bilateral exchange rate between the two currencies over time is determined by changes in relative prices in the two countries and misalignment is then defined as deviations of the real exchange rate from the parity in a selected year. The shortfall of this method is embedded in accounting only for monetary sources of exchange rates while neglecting contemporary exchange rate fluctuations attributed to real factors. Also, it treats the real exchange rate as an immutable number yet in practice the real exchange rate responds to movements in economic fundamental variables. On the other hand, the black-market premium approach defines misalignment as deviations between the parallel market and the official rate. The limitation of this measure is that it is sensitive to temporary shocks that may have little or no effect on the underlying fundamental real equilibrium exchange rate (Ghura & Grennes, Citation1993).
The recent and most used approach to measuring the equilibrium real exchange rate and its associated misalignment values is the model-based measure. This approach has its theoretical foundation in the so-called dependent model developed by Swan (Citation1956) where real exchange rate is defined as the relative price of tradable to non-tradable goods. This method involves the determination of fundamental factors that affect the real exchange rate. The long-run equilibrium exchange rate is then compared with the actual real exchange rate to come up with the misalignment measure.
In this paper, I follow a model by Baffes et al. (Citation1997) which assumes a small economy that is exposed to external competition producing and consuming two goods, namely, tradable and non-tradable. Aggregate demand for non-tradables is composed of private consumption () and government consumption (
). By letting
signify aggregate private spending (measured in tradables),
as the spending that goes into traded goods, and
as the total supply of non-tradables under the assumption of full employment of resources, the state of equilibrium in the non-tradable sector market can be expressed as:
From this expression, the real exchange rate is theoretically defined as the price ratio of tradables to non-tradables
and
respectively. This measure of the real exchange rate describes and explains the internal exchange rate that allocates resources in the domestic economy across tradable and non-tradable sectors. A depreciated real exchange rate can, for instance, signal profitability in non-tradables which facilitates a movement of resources from the non-tradable sector towards the tradable sector.
With respect to the external environment, equilibrium is attained if a change in the long-run position of international reserves collapses to zero. This equilibrium can also be defined as the gap between the capital account and the current account. The current account balance in the model is defined as the sum of 1) trade balance
, 2) net unilateral transfers and 3)
and net investment income
. The trade balance component is expressed as the gap between the supply of tradables
and the sum of consumption on tradables in the private sector
and government consumption on tradables (
). Net investment income is then defined by the product of net foreign assets (
) and real yield on the foreign assets
. External balance can be expressed as:
Combining the first and the second equation, assuming external balance holds in the long-run, the real exchange rate
that equilibrates the non-tradable sector and the external sector can simultaneously be written as:
For small open economies facing a binding credit ceiling, the trade balance can be treated as exogenous so that Equationequation 3(3)
(3) can be re-expressed as:
It is also important to consider the influence of an economy’s terms of trade as well as the trade policies. Since most sub-Saharan economies are too small to influence world prices, the foreign price of exportables ( and importables (
) is exogenously determined. In this respect, the corresponding prices in the domestic economy are given by:
where represent export and import tax rates, respectively, while E is the nominal exchange rate. The domestic relative price of exports and imports is given by:
where:
The dynamics of terms of trade denoted ( and the trade policy
will influence the real exchange rate for both the imports and exports. In addition to the fundamental variables in Equationequation 4
(4)
(4) , one can view the equilibrium real exchange rate as a function of the trade policy and terms of trade variables which can be represented by:
Based on recent literature, this model can be improved by accommodating a technological progress variable so that the final model takes the form:
The fundamental variables represent mainly four economic stances and these are (1) domestic supply-side factors particularly the Balassa–Samuelson effect that arise from rapid productivity growth in the tradable sector relative to the non-tradable sector; (2) fiscal policy, such as budget deficits and the change in the proportion and composition of government expenditure between tradable and non-tradable goods, (3) the external environment that includes capital inflows, and terms of trade and 4) the country’s commercial policy such as trade liberalisation in the form of a reduction in export subsidies and import tariffs.
Empirically from Equationequation (5)(5)
(5) , I first considered the issue of non-stationarity which is customary when estimating the equilibrium real exchange rate model. To test for panel non-stationarity of the real exchange rate and its fundamental determinants, I employ both the first- and second-generation panel unit root tests for robustness purposes. The first-generation tests in particular are due to Levin and Lin (Citation1993), Harris and Tzavalis (Citation1999), and Im et al. (Citation2003), Maddala and Wu (Citation1999), Choi (Citation2001) and Hadri (Citation2000). Second-generation tests include Bai and Ng (Citation2004), Pesaran (Citation2007) and Breitung and Das (Citation2005).
As will be shown in the results section, the results from panel unit root tests suggest non-stationarity of variables in level form but stationarity after first difference. Given that variables are integrated of order one (I (1)), the next step was to test for a cointegration among the variables. Again in the interest of reaching a solid conclusion, I employ several cointegration tests which include the Fischer-Johansen test by Maddala and Wu (1999), the residual-based tests for cointegration by Pedroni (Citation2001) and Kao (Citation1999) and the error correction-based tests proposed by Westerlund (Citation2007), and Persyn and Westerlund (Citation2008).
Since cointegration is mostly confirmed, I consider estimating the long-run relationship from a pool of candidates that include the panel ordinary least squares (OLS), Johansen maximum likelihood, the dynamic ordinary least squares (DOLS), fully modified ordinary least squares (FMOLS), and the Pooled Mean Group (PMG). Monte Carlo results in Chiang and Kao (Citation2002) find the DOLS superior in small sample sizes. Against this background, the DOLS estimator was preferred in this paper.
Technically, the DOLS estimator includes leads and lags of differenced explanatory variables and addresses endogeneity, regressor simultaneity and serial autocorrelation through the generalized least squares procedure. It is derived from the following specification.
where is the logarithm of real effective exchange rate empirically measured as the nominal effective exchange rate (a measure of the value of a currency against a weighted average of several foreign currencies) divided by a price deflator or index of costs,
is a vector of coefficients of leads and lags and
is a vector of what drives the real effective exchange rate as specified in Equationequation (5)
(5)
(5) . Empirically, these fundamentals include government consumption, technological progress, capital inflows, terms of trade and trade openness.
Government consumption expressed as a percentage of GDP is used to capture the stance of the fiscal policy. Due to unavailability of data on government consumption on tradable and non-tradable goods (
, total government consumption is used instead. A rise in government consumption of non-tradable goods causes the real exchange rate to appreciate while the real exchange rate depreciates if excess consumption is concentrated on tradable activities. Either sign is therefore expected.
With respect to technological progress, the Balassa-Samuelson theory postulates that productivity growth in tradable sectors versus the non-tradable sectors due to technological advancement leads to real exchange rate appreciation by stimulating demand in the non-tradable sectors. The challenge, however, lies in finding an appropriate proxy variable for technological advancement. Earlier studies such as Ghura and Grennes (Citation1993) captured the Balassa-Samuelson effect by a simple time trend. Others such as Razin and Collins (Citation1997) proxied the Balassa-Samuelson effect by growth of GDP per worker. Recent studies have mostly used GDP per capita growth rate. None of these proxies is not without its own limitations, but Toulaboe (Citation2011) asserts that rapid growth in GDP per capita is very much associated with technological advancement. In line with this argument, this paper uses GDP growth rate as a proxy for technological progress. A positive sign is expected.
Trade openness is also included calculated as the ratio of sum of imports plus exports to GDP. This measures the degree to which a country’s real exchange rate is affected by the external environment. It is expected that trade openness exerts a downward pressure on the relative price of tradable to non-tradable goods, thereby leading to a depreciation of the real exchange rate.
Terms of trade, another fundamental is defined as the foreign price of exports divided by the foreign price of imports. The effects of terms of trade on real exchange rate are ambiguous. An improvement in terms of trade has both income and substitution effects in the sense that, on one hand, it leads to an increase in real wages allowing an intersectoral shift of mobile factors of production to the tradable sectors. This, in the context of the Dutch disease theory, will cause an appreciation of the real exchange rate. On the other hand, the substitution effect is explained as follows; an improvement in terms of trade lowers the cost of imported inputs in the production of non-traded goods causing real exchange rate depreciation. Either sign is therefore expected.
Lastly, capital inflow is defined as the ratio of the difference between real exports and real imports to real GDP following Toulaboe (Citation2011). Bhattacharya et al. (Citation1997) argue that when net capital inflows exceed debt service obligations, the real exchange rate may appreciate while Baffes et al. (Citation1997) justify the negative sign of the variable on its associated increases in domestic absorption, particularly in the non-tradable sector. With these fundamentals, the coefficient of a lead or lag of the first differenced regressors and the estimated coefficient of the DOLS is given by:
where = [
—
, Δ
… Δ
] is
embeds the above defined fundamentals. Using the Schwarz information criterion (SIC), 1 lead and 1 lag were selected in the estimation process. The equilibrium real exchange rate (
) for each time period is then obtained by multiplying the estimated elasticities by the values of the fundamental variables. Following Baffes et al. (Citation1997) and Toulaboe (Citation2011), real exchange rate misalignment is then computed as
where is the actual real effective exchange rate and
is the equilibrium real exchange rate. The computed misalignment variable (not presented here for brevity sake) shows that the real effective exchange rate was generally overvalued in Burundi, Cameroon, Gabon, Ghana, Gambia, Lesotho, Malawi, Togo, and Uganda and undervalued in South Africa, Nigeria, Equatorial Guinea, and Cote d’Ivoire.
Having computed the misalignment variable, I then considered the conditional convergence growth theory which captures the exchange rate misalignment, state fragility, and other growth determinants. Relative to endogenous growth theories, the neoclassical conditional convergence theoretical framework allows us to unravel answers to a number of puzzling empirics such as the disparities in country performance in terms of growth and the rate at which poor countries can catch up with richer ones. The conditional convergence theoretical model takes the following form.
where denotes the growth rate in country
,
is a vector of factors that govern the long-run steady state,
is the rate of convergence (which can be equivalently represented by
where T is the time lag) and
is a random error term. What enters
are called growth fundamentals which essentially represent a set of factors that tell us where each country is heading in the long run. While this set of fundamentals is large in principle, only key factors explained later are considered based on existing literature. From Equationequation (7)
(7)
(7) , the specific econometric model takes the following form.
where represents annual real per capita GDP growth of country
in period
,
is initial income expressed in natural logarithm,
is a convergence parameter,
is as defined before, SFI is a state fragility index,
is a vector of standard controls with their corresponding slope coefficients embedded in vector
and
is an idiosyncratic disturbance term.
The vector of controls includes government consumption proxying the fiscal policy, inflation capturing the importance of monetary policy, investment, urbanization, trade openness, and terms of trade which capture external developments. Government consumption, terms of trade and trade openness which have already featured in the equilibrium real exchange rate model are as defined before. In addition to these already defined variables, urbanization refers to people living in urban areas as defined by national statistical offices. Inflation as measured by the consumer price index reflects the annual percentage change in the cost to the average consumer of acquiring a basket of goods and services. Investment is proxied by gross capital formation which, by measurement, consists of outlays on additions to the fixed assets of the economy plus net changes in the level of inventories.
State fragility, which is one of the variables of interest, is an index sourced from the center for systemic peace. By measurement, the state fragility index is based on both effectiveness and legitimacy in four performance dimensions namely security, political, economic, and social with scores attached to each category. The state fragility index, then, produces scores ranging from 0 “no fragility” to 25 “extreme fragility.”
In the literature, real exchange rate misalignment is generally found to harm economic growth primarily through its negative effect on the tradable sector (Zakaria, Citation2010). Therefore, is expected to be negative. State fragility signals weak governance and ineffective policy making which is detrimental to economic growth. As a result,
is expected to be negative. Since fragile states are known for ineffective policy responses to crises, our hypothesis is that real exchange rate misalignment is likely to have a detrimental effect on growth that increases with state fragility. Therefore, I expect both
and
to be significantly negative.
The challenge with estimating Equationequation (8)(8)
(8) Footnote1 is that state fragility and real exchange rate misalignment can be both causes and consequences of slow growth. In this case,
and
from the pooled least squares will be biased and inconsistent. In addition, state fragility, real exchange rate misalignment, and economic growth can be simultaneously affected by third factors nested in the disturbance term raising serious endogeneity concerns. The standard fixed effects estimator with country-specific dummies only succeeds in purging out endogeneity stemming from time-invariant heterogeneity through M-1 country dummies or the within transformation but fails to address endogeneity arising from omitted time-varying factors.
To address the latter kind of endogeneity, a standard solution in the literature uses instrumental variables via the 3 stage least squares (3SLS) technique. Success of this approach lies in finding a variable correlated with the endogenous regressor (real exchange rate misalignment and state fragility in this particular case) but uncorrelated with the error term. The endogenous regressor(s) will then be exogenised using this instrument and later plugged back into the growth equation. In our case, finding an appropriate external instrument is a formidable task given the difficulty of imagining a variable that can be correlated with the real exchange rate and state fragility without having an independent effect on economic growth.
When faced with the above challenge, a natural solution suggested in panel data studies takes advantage of lagged values of the variables as instruments in the Generalised Methods of Moments (GMM) framework proposed by Arellano and Bond (Citation1991). This is the approach applied in this paper primarily because of its ability to pin down endogeneity issues using internal instruments. The Panel GMM is more appropriate in case of T < N. In this paper, T = 9 < N = 13. In terms of mechanics, the GMM estimator is based on differencing regressions and/ or instruments to account for unobserved effects and using lagged dependent and independent variables as instruments. It is based on a dynamic model of the following sort.
Since I am working with a panel dataset, country heterogeneity needs attention even in this dynamic setting (Cameron & Trivedi, Citation2005). In the context of GMM estimators, this unobserved heterogeneity is removed by first differencing Equationequation (8)(8)
(8) as follows
where is a first difference operator. First differencing our specification this way wipes away country-specific effects but requires instrumentation to control for endogeneity of the regressors and the correlation between the new error term
and the lagged depended variable
. In a panel data setup, initial values of regressors as well as the lagged response variable are utilized as instruments and this constitutes the difference GMM estimator. Blundell and Bond (Citation1998) show however that the difference GMM estimator is exposed to weak instruments which distort both the small sample and asymptotic performance of the difference estimator. A solution to this problem is proposed by Arellano and Bover (Citation1995) and Blundell and Bond (Citation1998) via the system GMM and it combines the first differenced equations with the equation in which the level regressors are instrumented by their first differenced terms. Blundell and Bond (Citation1998) through Monte Carlo simulation find the system GMM estimator relatively more efficient than the first difference estimator. For this reason, this paper applies the Blundell and Bond system GMM estimator.
The Blundell and Bond system GMM can be estimated using one-step or two-step. The one-step system GMM estimator employs a variance covariance matrix while the two-step system GMM estimator capitalizes on the residual values generated from the first estimation step for variance covariance matrix, , which is utilized in the second estimation stage. Both the one-step and two-step estimators can be performed with heteroscedasticity corrected standard errors.
The two-step system GMM estimator is relatively more efficient as compared to the one-step estimator with a heteroscedastic error-structure. Nonetheless, when the size of (cross-sectional members, countries in this particular case) is small relative to the number of instruments used, the standard errors from the two-step system GMM estimator will be downward biased (Blundell & Bond, Citation1998). The bias on the standard errors will later compromise and distort statistical inferences on the parameters of our explanatory variables. In such a case where
is smaller than the number of instruments, the one-step system GMM estimator will be relatively more reliable (Blundell & Bond, Citation1998). In the present case, I am only working with a handful of sub-Saharan countries and therefore the above-mentioned issue is quite relevant if more lags are used as instruments. Mindful of this fact, the one-step system GMM estimator is employed.
It is critical to test the validity of certain assumptions that underpin the success of the system GMM estimation technique. These tests must essentially address the validity and relevance of the instruments used in the system. The first diagnostic test is the Sargan test for over-identifying restrictions. This diagnostic test allows us to determine exogeneity or validity of the instruments. The second diagnostic test is the Arellano and Bond test for AR autocorrelation.
Time effects are customarily included in panel data regressions to absorb technical changes and the effects of common shocks on growth over time. Their inclusion is determined by their joint significance in the model. If the F-test produces a significant result, then time dummies will be necessary. In this paper, however, time dummies entered the system insignificantly; hence, they are not included to save degrees of freedom and to avoid unnecessary model overfitting.
An important issue that that needs to be tested is endogeneity of the regressors, particularly real exchange rate misalignment and state fragility. In spite of the theoretical arguments leading to suspicion of endogeneity of the two covariates, the use of the system GMM technique to control this estimation problem must well be balanced with the loss of efficiency if the regressors happen to be exogenous (Wooldridge, Citation2003). It is therefore in this regard important to perform a formal test of endogeneity in order to determine whether or not the GMM technique is in the first place required instead of the pooled least squares. To achieve this in this paper, the Durbin—Wu—Hausman (DWH) test of regressor endogeneity is employed. The null hypothesis of the DWH test holds that the variables are exogenous.
In addition to endogeneity, an equally important aspect in our case relates to contemporaneous correlation. Contemporaneous correlation exists when the idiosyncratic disturbance terms are correlated across individuals. If this is the case, hypothesis testing will be grossly compromised leading to distortion of statistical inference (Everaert and De Groote, Citation2016). It is important in this paper to test for contemporaneous correlation because the GMM estimation strategy assumes autocorrelation within the individual units’ error terms but not across them (Baum et al., Citation2012). In this case, I suspect cross-sectional dependence that may emanate from spillover effects among the selected sub-Saharan countries. To test for cross-sectional dependence in this paper, I use a method developed recently by Pesaran (Citation2015). The null hypothesis of this test holds that the residuals are weakly cross-sectional dependent and it is rejected if the null hypothesis is less than 10 percent.
3. Results and discussion
I estimated separately first-generation tests () and second-generation tests (). In total, I performed 6 panel unit root tests, with first-generation and second-generation tests having 3 apiece. The former tests do not accommodate potential cross-sectional dependence among countries while the latter is quite robust to this potential problem. In both cases however, variables seem to be non-stationary in levels and stationary after first differencing. I considered specifications with and without a trend and intercept, but for brevity, only results for specifications with the intercept are presented in . Important is that inclusion and exclusion does not significantly change the outcome of the tests.
Table 1. First-generation panel stationarity tests
Table 2. Second-generation panel stationarity tests
Second-generation panel unit root tests are presented in . With the exception of the Hadri test, all the panel unit root tests have a null of a unit root. Included in the table are test-statistics for each test. These test statistics were compared against the 1, 5, and 10 percent critical values and a test statistic above any of these critical values would warrant rejection of the null in favour of the alternative. As indicates, most tests do not reject the null of non-stationarity in levels and only reject them after first differencing (1st ∆). The Hadri test, on the other hand, strongly rejects the null of stationary panels in levels. We can therefore conclude from the results that we are dealing with non-stationary panels overall and that cross-sectional dependence is not very important in influencing the outcome of the results.
With non-stationary panels at hand, I proceed with cointegration tests to determine whether or not these variables move together in the long run. In line with the methodology, reports results from 4 cointegration tests. The results are quite comforting. All 4 tests suggest the existence of a cointegrating relationship among the variables.
Table 3. Pedroni test for cointegration
Table 4. Westerlund test for cointegration
Table 5. Kao test for cointegration
Table 6. Johansen and Fischer test for cointegration
The null hypothesis of no cointegration under the Pedroni test is rejected at the 10 percent significance level in 6 out of 7 cases. Similarly, the null of no cointegration is strongly rejected under the Kao test pointing to a long-run relationship among the non-stationary variables. The Westerlund test also rejects at 1 percent level the null of no cointegration which confirms the results of the Kao and Pedroni tests.
Lastly, the first null of no cointegration is strongly rejected under the Johansen and Fischer test. The second null hypothesis of at most 1 cointegrating relationship is also rejected at the 1 percent level. In the third case, the null of at most 2 cointegrating relationship cannot be rejected at the 10 percent level both under the trace test and the max-eigen test. Combined together, all the cointegrating tests are pointing to a long-run relationship among the variables paving way for the DOLS estimator.
therefore presents the results from the estimated real exchange rate model. All the exchange rate fundamentals have the expected signs. The capital inflows variable is positive and statistically significant at 5 percent indicating that inflows of capital in the selected sub-Saharan countries appreciate their real exchange rates. The technological variable proxy is positive and significantly corroborating the Balassa effect in which productivity growth tends to be concentrated in the tradable sector leading to a real appreciation of the currency. The government consumption variable is statistically negative suggesting that a high proportion of government spending was devoted to tradable goods in these countries leading to an appreciation of the real exchange rate. The terms of trade variable, on the other hand, are positive and highly significant indicating an income effect in which improvements in the terms of trade increase real national income and the demand for both tradable and non-tradable goods, causing a real appreciation that works through an upward pressure on the relative price of non-tradables. This is consistent with results in Elbadawi et al. (Citation2012).
Table 7.: Equilibrium real exchange rate model
The next step is to check whether or not the countries are cross-sectionally independent and also whether the regressors are indeed endogenous to warrant the use of the system GMM estimation strategy. The former was performed by Pesaran cross-sectional dependence test while the latter was performed by the Durbin Wu-Hausman test. For the sake of brevity, both tests are reported in .
Table 8. Endogeneity and cross-sectional dependence
The null hypothesis of the Durbin Wu-Hausman test is that the variables are exogenous. The probability value is less than 5 percent hence the null of variable exogeneity can be rejected. This means that the regressors are endogenous. With respect to the test for cross-sectional dependence, the null hypothesis is that the countries are cross-sectionally independent. The probability value is way above the maximum significance level; hence, the null cannot be rejected. This means that selected sub-Saharan countries are cross-sectionally independent. For robustness checks, additional tests for cross-sectional dependence are considered. In particular, Breusch-Pagan LM test and its corresponding scaled versions, namely scaled Breusch-Pagan LM, and the bias-adjusted version proposed by Baltagi et al. (Citation2012). Like the Pesaran CD test, these additional tests do not reject the null hypothesis of no cross-sectional dependence.
The system GMM estimation strategy maintains the assumption of cross-sectional independence and is particularly appropriate in cases of endogenous regressors. Given that the variables are endogenous and cross-sectionally independent, we can safely proceed with the system GMM estimation strategy. presents the main results. It comprises four regression variants. Variants (1) and (2) are parsimonious in the sense of including only the variables of interest. Variants (3) and (4) then control for other relevant drivers of economic growth in these countries as guided by literature.
Table 9.: Misalignment, state fragility, and growth
All variables have the expected signs. The initial income coefficient is significantly negative demonstrating conditional convergence in which higher initial income reduces subsequent growth. Government consumption and inflation are both negative and statistically significant reflecting the importance of prudent fiscal and monetary policies, respectively. This result echoes the general consensus that inflation control should be a precondition for economic growth as testified by the growing number of countries pursuing inflation targeting policies. Similarly, it is now commonly believed that persistent government spending can be a hindrance to economic growth, particularly if it comes in form of unproductive spending. Barro (Citation1991) in particular shows a detrimental effect of government consumption on economic growth which is consistent with the result in . Here, a percentage point increase in government consumption and inflation is estimated to reduce the rate of economic growth by 0.098–0.104 and 0.099–0.113 percent, respectively.
The investment coefficient is significantly positive in both variants (3) and (4) reinforcing capital accumulation as an important growth process in sub-Saharan Africa. A percentage point increase in the share of gross capital formation on GDP is estimated to raise the rate of economic growth by about 0.1 percent on impact of all else being equal. This compares with the 0.15–0.17 observed in Toulaboe (Citation2011) from a set of developing countries. Looking at the data from WDI, gross capital formation in sub-Saharan Africa declined from 43.6 percent of GDP in 1981 to 21.8 percent of GDP in 2019. This is essentially a 50 percent drop in gross capital formation, and it suggests that countries in this region could be struggling to boost growth partly as a result of declining investment.
The urbanization coefficient is negative and statistically significant at 1 percent level. This is not a surprising result Kenya, a sub-Saharan country, went through significant urbanization without witnessing positive growth (see Fay & Opal, Citation2000). In theory, one would expect urbanization to influence economic growth positively as part of a structural transformation in which the rural population migrates to cities. This transformation comes with a reallocation of labour from the low productivity agricultural sector to a more productive industrial sector setting the stage for higher economic growth. When job creation is a challenge in cities, however, as has often been the case for sub-Saharan Africa, growth in urban population can put pressure on limited urban resources in a manner that can be detrimental to economic growth.
Trade and terms of trade variables both enter positively which is consistent with Toulaboe (Citation2011). In Toulaboe (Citation2011) however, the coefficient in terms of trade is insignificant despite being positive and slightly similar in terms of size, i.e. 0.04 and 0.07. The difference in significance could be attributed to the difference in our sampling periods. Toulaboe (Citation2011) focused on the 1985–99 period, an era of trade liberalization. Since the benefits of a favourable external environment may be dynamic and long term, it may be less surprising to find the effect of terms of trade significant during the 2010–18 sampling period. In terms of interpretation based on , a percentage point increase in the share of trade on GDP is estimated to raise growth by about 0.08 percent while a percentage increase in terms of trade enhances growth in the range of 0.05–0.06 percent. Clearly, these two results demonstrate how crucial the external environment is in fostering growth in sub-Saharan Africa.
Turning to the variables of interest, evidence points to a negative and statistically significant effect of state fragility on economic growth across all the four variants. An increase in state fragility lowers annual economic growth significantly controlling for investment, inflation, urbanization government consumption, terms of trade and trade openness. This is in line with a recent study by Chuku and Onye (2019) and it reiterates the relevance of state effectiveness (or lack thereof) as a determinant of economic growth in SSA. As expected, our misalignment variable enters with a significantly negative coefficient across all the four regression variants. A marginal increase in real exchange rate misalignment is accompanied by a significant decline in economic growth on impact. This is consistent with earlier studies (Dubas, Citation2009; Ebaidalla, Citation2014; Toulaboe, Citation2011) in which currency overvaluation is found to hurt economic growth.
From variant (2) to variant (4), real exchange rate misalignment is interacted with the state fragility index to appreciate how the latter influences its effect on economic growth. The coefficients of this interaction terms are negative and statistically significant at 1 percent level across the three variants. This result confirms our conjecture that real exchange rate misalignment can have a negative effect on economic growth which increases with state fragility. In other words, the negative effect of real exchange rate misalignment on economic growth is more detrimental in countries that are more fragile and less so in countries that are robust and resilient. Our explanation for this empirical result is that fragile states are ineffective in making policy interventions that effectively realign the real exchange rate with the equilibrium level dictated by its long run fundamentals. These countries are characterised by weak governance, political turmoil, weak institutions, a breakdown of the social contract, conflict, civil wars, and corruption in some instances. An exchange rate overvaluation is expected to have a persistently negative and large effect on growth in these countries due to the state’s ineffectiveness to intervene and correct macroeconomic imbalances in the event of an exchange rate misalignment. Similarly, the negative effect of real exchange rate misalignment on economic growth is likely to be ameliorated by state resilience owing to state’s effectiveness in correcting macroeconomic imbalances.
The estimated system Generalized Method of Moments (GMM) was subjected to a battery of diagnostic tests which include the Arellano and Bond first-order correlation (which is expected due to the lagged dependent term) and second correlation tests and the Sargan tests test for overidentifying restrictions. Rejecting the null hypothesis of no second-order correlation would imply that the moment conditions are invalid. Results presented in the lower part of show the presence of first order and not second-order autocorrelation. In addition, the Sargan test is insignificant across all the specifications. These results collectively suggest that the moment conditions from the estimated system GMM are valid.
4. Conclusion
Sub-Saharan Africa remains one of the world's most developmental challenges of our time and papers seeking answers for its slow growth have expanded rapidly in the last three decades. Most accounts generally link it to drought, corruption, adverse terms of trade, poor infrastructure, open conflict, and exchange rate misalignment. In this paper using evidence from 13 sub-Saharan countries, I add another important explanation that exchange rate misalignment whose negative effect on growth is now widely accepted, is more detrimental in fragile states. In other words, evidence shows a negative effect of real exchange rate misalignment that increases with state fragility. Based on this evidence, I urge countries in this region to improve state effectiveness as an effort to reduce the negative effect of exchange rate misalignment on economic growth. Pinpointing precise efforts to improve state fragility would be over-reaching the data. Therefore, I leave this for future studies.
Additional information
Funding
Notes on contributors
Brian Tavonga Mazorodze
Brian Tavonga Mazorodze is an economist and a current research fellow with the University of Zululand whose research covers trade, development and industrial economics.
Notes
1. The presence of an initial income coefficient (the convergence term) also raises concerns of the Nickell bias (Nickell, Citation1981).
References
- Abida, Z. (2011). Real exchange rate misalignment and economic growth: An empirical study for the Maghreb countries. Zagreb International Review of Economics and Business, 14(3), 87–19. DOI: 10.5539/ijef.v3n3p190
- Arellano, M., & Bond, S. (1991). Some tests of specification for panel data: Monte Carlo evidence and an application to employment equations. The Review of Economic Studies, 58(2), 277–297. https://doi.org/10.2307/2297968
- Arellano, M., & Bover, O. (1995). Another look at the instrumental variable estimation of error-components models. Journal of Econometrics, 68(1), 29–51. https://doi.org/10.1016/0304-4076(94)01642-D
- Baffes, J., Elbadawi, I. A., & O’connell, S. A. 1997. Single-equation estimation of the equilibrium real exchange rate, World Bank Working Paper No. 1800, Development Research Group.
- Bai, J., & Ng, S. (2004). A Panic attack on unit roots and cointegration. Econometrica, 72(4), 1127–1177.
- Baltagi, B. H., Feng, Q., & Kao, C. (2012). A lagrange multiplier test for cross-sectional dependence in a fixed effects panel data model. Journal of Econometrics, 170(1), 164–177. https://doi.org/10.1016/j.jeconom.2012.04.004
- Barro, R. J. (1991). Economic growth in a cross section of countries. The Quarterly Journal of Economics, 106(2), 407–443. https://doi.org/10.2307/2937943
- Baum, M. A., Poplawski-Ribeiro, M. M., & Weber, A. (2012). Fiscal Multipliers and the state of the Economy (No. 12–286). International Monetary Fund.
- Béreau, S., Villavicencio, A. L., & Mignon, V. (2012). Currency misalignments and growth: A new look using nonlinear panel data methods. Applied Economics, 44(27), 3503–3511. https://doi.org/10.1080/00036846.2011.577022
- Bhattacharya, R. (1997). The trade balance and the real exchange rate: evidence from a VAR for the United States. Journal of Economic Insight, 23(1), 59–71.
- Blundell, R., & Bond, S. (1998). Initial conditions and moment restrictions in dynamic panel data models. Journal of Econometrics, 87(1), 115–143. https://doi.org/10.1016/S0304-4076(98)00009-8
- Breitung, J., & Das, S. (2005). Panel unit root tests under cross‐sectional dependence. Statistica Neerlandica, 59(4), 414–433. https://doi.org/10.1111/j.1467-9574.2005.00299.x
- Cameron, A. C., & Trivedi, P. K. (2005). Microeconometrics: Methods and applications. Cambridge university press.
- Chiang, M.-H., & Kao, C. A. (2002). Nonstationary panel time series using NPT 1.3–A user guide. Center for Policy Research, Syracuse University.
- Choi, I. (2001). Unit root tests for panel data. Journal of International Money and Finance, 20(2), 249–272. https://doi.org/10.1016/S0261-5606(00)00048-6
- Chuku, C., & Onye, K. (2019). Macroeconomic consequences of state fragility in sub-Saharan Africa. Review of Development Economics, 23(3), 110–1140.
- Couharde, C., & Sallenave, A. (2013). How do currency misalignments’ threshold affect economic growth? Journal of Macroeconomics, 36(1), 106–120. https://doi.org/10.1016/j.jmacro.2012.11.002
- Dai, P. V., Delpachitra, S., & Cottrell, S. (2017). Real exchange rate and economic growth in East Asian countries: The role of financial integration. The Singapore Economic Review, 62(1), 163–177. https://doi.org/10.1142/S0217590816500168
- Dubas, J. M. (2009). The importance of the exchange rate regime in limiting misalignment. World Development, 37(10), 1612–1622. https://doi.org/10.1016/j.worlddev.2009.02.003
- Ebaidalla, E. M. (2014). Real exchange rate misalignment and economic performance in Sudan. African Review of Economics and Finance, 6(2), 115–140.
- Elbadawi, I., & Soto, R. (2010). Exchange Rate and Monetary Policy for Sustainable Post-conflict Transition (No. 392).
- Elbadawi, I. A., Kaltani, L., & Soto, R. (2012). Aid, real exchange rate misalignment, and economic growth in Sub-Saharan Africa. World Development, 40(4), 681–700. https://doi.org/10.1016/j.worlddev.2011.09.012
- Everaert, G., & De Groote, T. (2016). Common correlated effects estimation of dynamic panels with coss-sectional dependence. Econometric Reviews, 35(3), 428–463.
- Fay, M., & Opal, C. A. 2000. Urbanization without growth: A not-so uncommon phenomenon. Policy Research Working Paper No. 2412, World Bank, Washington, DC.
- Gala, P. (2008). Real exchange rate levels and economic development: Theoretical analysis and econometric evidence. Cambridge Journal of Economics, 32(2), 273–288. https://doi.org/10.1093/cje/bem042
- Ghura, D. and Grennes, T. J. 1991. The impact of real exchange rate misalignment and instability on macroeconomic performance in Sub-Saharan Africa (No. 943-2016-74603)
- Ghura, D., & Grennes, T. J. (1993). The real exchange rate and macroeconomic performance in Sub-Saharan Africa. Journal of Development Economics, 42(1), 155–174. https://doi.org/10.1016/0304-3878(93)90077-Z
- Glüzmann, P. A., Levy-Yeyati, E., & Sturzenegger, F. (2012). Exchange rate undervaluation and economic growth: Díaz Alejandro (1965) revisited. Economics Letters, 117(3), 666–672. https://doi.org/10.1016/j.econlet.2012.07.022
- Hadri, K. (2000). Testing for stationarity in heterogeneous panel data. The Econometrics Journal, 3(2), 148–161. https://doi.org/10.1111/1368-423X.00043
- Harris, R. D., & Tzavalis, E. (1999). Inference for unit roots in dynamic panels where the time dimension is fixed. Journal of Econometrics, 91(2), 201–226. https://doi.org/10.1016/S0304-4076(98)00076-1
- Im, K. S., Pesaran, M. H., & Shin, Y. (2003). Testing for unit roots in heterogeneous panels. Journal of Econometrics, 115(1), 53–74. https://doi.org/10.1016/S0304-4076(03)00092-7
- Jehan, Z., & Irshad, I. (2020). Exchange rate misalignment and economic growth in Pakistan: The role of financial development. The Pakistan Development Review, 59(1), 81–99. https://doi.org/10.30541/v59i1pp.81-99
- Kao, C. (1999). Spurious regression and residual-based tests for cointegration in panel data. Journal of Econometrics, 90(1), 1–44. https://doi.org/10.1016/S0304-4076(98)00023-2
- Levin, A., & Lin, C.-F. 1993. Unit root tests in panel data: New results. University of California at San Diego, Economics Working Paper Series.
- Levy-Yeyati, E., & Sturzenegger, F. (2003). To float or to fix: Evidence on the impact of exchange rate regimes on growth. The American Economic Review, 93(4), 1173–1193. https://doi.org/10.1257/000282803769206250
- Maddala, G. S., & Wu, S. (1999). A comparative study of unit root tests with panel data and a new simple test. Oxford Bulletin of Economics and statistics, 61(S1), 631–652.
- Mazorodze, B. T., & Tewari, D. D. (2018). Real exchange rate undervaluation and sectoral growth in South Africa. African Journal of Economic and Management Studies, 4(4), 462–476. https://doi.org/10.1108/AJEMS-06-2017-0132
- Nickell, S. (1981). Biases in dynamic models with fixed effects. Econometrica: Journal of the Econometric Society, 49(6), 1417–1426. https://doi.org/10.2307/1911408
- Özyurt, S. (2013). Currency undervaluation and ECONOMIC REBALANCING TOWARDS SERVICES. Is China an Exception? China & World Economy, 21(1), 47–63. https://doi.org/10.1111/j.1749-124X.2013.12008.x
- Pedroni, P. (2001). Purchasing power parity tests in cointegrated panels. Review of Economics and Statistics, 83(4), 727–731. https://doi.org/10.1162/003465301753237803
- Persyn, D., & Westerlund, J. (2008). Error-correction-based cointegration tests for panel data. Stata Journal, 8(2), 232–241. https://doi.org/10.1177/1536867X0800800205
- Pesaran, M. H. (2007). A simple panel unit root test in the presence of cross‐section dependence. Journal of Applied Econometrics, 22(2), 265–312. https://doi.org/10.1002/jae.951
- Pesaran, M. H. (2015). Testing weak cross-sectional dependence in large panels. Econometric Reviews, 34(6–10), 1089–1117. https://doi.org/10.1080/07474938.2014.956623
- Razin, O., & Collins, S. M. (1997). Real exchange rate misalignments and growth No. w6174. National Bureau of Economic Research.
- Sallenave, A. (2010). Real exchange rate misalignments and economic performance for the G20 countries. Economie internationale, 59–80.
- Swan, T. W. (1956). Economic growth and capital accumulation. Economic Record, 32(2), 334–361. https://doi.org/10.1111/j.1475-4932.1956.tb00434.x
- Toulaboe, D. (2011). Real exchange rate misalignment and economic growth in developing countries. Southwestern Economic Review, 33(1), 57–74.
- Westerlund, J. (2007). Testing for error correction in panel data. Oxford Bulletin of Economics and Statistics, 69(6), 709–748. https://doi.org/10.1111/j.1468-0084.2007.00477.x
- Wooldridge, J. M. (2003). Cluster-sample methods in applied econometrics. The American Economic Review, 93(2), 133–138. https://doi.org/10.1257/000282803321946930
- Yan, H.-D., & Yang, C.-L. (2012). Does an undervalued currency merit economic growth?: Evidence from Taiwan. Panoeconomicus, 59(1), 37–57. https://doi.org/10.2298/PAN1201037Y
- Zakaria, M. (2010). Exchange rate misalignment and economic growth: Evidence from Pakistan’s recent float. The Singapore Economic Review, 55(3), 471–489. https://doi.org/10.1142/S0217590810003857