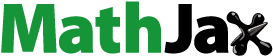
Abstract
This paper focuses on determining the factors influencing investors’ risk-taking through empirical evidence from Vietnam. This study investigates risk perception, expected return and herding behavior, and other determinants such as historical volatility and subjective financial risk attitude; according to previous studies, these are the main components affecting risk-taking behavior among investors. Overconfidence (better than average, miscalibration, and excessive optimism) is also taken into consideration. We employ pooled-OLS and quantile regression to overcome the shortage of research models in this field. In addition, we demonstrate how risk-taking behavior can be affected by those factors with the application of measures across four different investment channels. This study suggests implications for investors who wish to control risk.
PUBLIC INTEREST STATEMENT
This paper explains the relationship between the determinants and risk-taking behaviors in the Vietnamese sample. By collecting 85 response surveys from investors, we explored three main findings. First, risk perception, expected return and herding behavior, and other determinants such as historical volatility and subjective financial risk attitude contribute to the risk-taking behavior. Second, Overconfidence (better than average, miscalibration, and excessive optimism) is the important factor. Finally, our results hold robust when replacing the different econometric models, suggesting to confirm what we found before.
1. Introduction
On over the world, financial behavior attracts researchers’ attention by its judgment and theory used a lot in practice. However, in Vietnam, research on financial behavior or psychology of investors as making their decisions for investing cases is still limited. One of the researchers has contributed to the development of financial behavior when combining the psychology and finance is Gabriel Tarde, then there has been further research on the application of psychology to finance and there is a significant development of appearing this study in more schools of study to exploit different aspects of the psychology of human behavior. One of the most important decisions of investors is to decide to take the risk under consideration of implicit risk owned by each kind of asset (Wongchoti et al., 2020). And knowing that investment projects having a high probability of risk often brings more profit than the safer ones. In addition, investors are usually willing to take a risk in exchange for a higher rate of return. Therefore, researching the possibility of risk-taking of investors to find out the factors affecting their decision for taking a risk is really urgent in the context of increasing a variety of diversified investment channels that are appealing to investors.
The person who has a stronger achievement motive tends to set his level of aspiration in the intermediate zone where there is moderate risk. He has the motive to avoid failure, and he will voluntarily choose activities that maximize his own anxiety about failure. Three factors are motive, expectancy, and incentive impact on the level of investors’ risk-taking differently (Atkinson, Citation1957).
Weber and Hsee (Citation1998) define the preference for an asset or risky option affected by risk perception and expected return to be “within each expected value group the Willingness to pay or risk preference increase with the option probability of loss which is positively correlated with the magnitude of gains”.
Weber et al. (Citation2005) conclude that Risk perception is forecasted through forecasting tools such as asset name, historical volatility, and historical return. The studies lay the groundwork for financial behavior is Amos Tversky and Daniel Kahneman, Richard H.Thaler and Robert Shiller, especially the famous book “Irrational Exuberance” (Citation2000), which correctly predict the collapse of the global stock market, has created a great turning point for the behavioral finance researchers as constantly offering new study. In particular, the study of Weber and Blais (Citation2006) who contribute to researches of financial behavior DOSPERT scale to not only measure the risk-taking ability of people in various sectors in the field of investment but also explain somewhat human psychology when introducing into a particular situation in life to measure risk perceptions of each person toward a special situation and make their own decisions. Nosić and Weber (Citation2010) accept that the influence of Subjective Financial Risk Attitude on risk-taking in case of stock and lotteries by providing a situation and ask the participant to invest their budget (assumed 10,000 euros) in the risk-free asset with a fixed rate of return or the implicit risk asset with the historical price performed. The measurement of risk-taking, in this case, is the amount of money participants accept to trade-off invest in risky assets instead of investing in a risk-free asset. Broihanne (Broihanne et al., Citation2014) figured out that the overconfidence determinants such as better than average, optimism and miscalibration are significant risk perception and overconfidence strongly affect the risk-taking behaviors of professionals. This result confirms the strong role played by risk perception of stocks with respect to risk-taking behavior. The identification of risk-taking by the degree of herding should, in his view, be adopted only with some caution since the relation of herding and experience is relatively unambiguous (Menkhoff et al., Citation2006). Hence, as considering factors like experience, knowledge, and bravery, there should be huge differences between student groups and investors. Because of the fact that students who have not participated in the market to trade will give inaccurate answers.
In addition to the diversity of investment channels, we also focus on investors who actively and independently make their investment in assets rather than in mutual or traditional funds as the funds in Vietnam are not as popular as other markets. Traditional funds have more abnormal (superior as well as inferior) selectivity performance than their ethical counterparts. Restricted investment opportunities because of screening criteria require managers of the ethical funds to be more efficient and disciplined in picking “winners” and avoiding “losers” to keep their transaction costs low and portfolio return reasonably high. The enlarged boundary of the unrestricted feasible investment opportunity set makes managers of traditional equity funds wander around unsuccessfully searching for mispriced assets and thereby generates too many transaction costs that drive down their portfolio returns (Li et al. Citation2018). That is also the reason we do not include investment in the fund in this study.
As regards the Objectives of our research, in this study, the authors inherit from the previous studies from both international and domestic journals regarding the ability to take a risk so as to continue developing and providing closer empirical analysis to the real market in Vietnam. Specifically, in this research, the survey will be designed to measure the risk-taking ability of the investor toward four assets namely securities, real estate, foreign currencies, and gold based on the previous literature review. Each asset has different characteristics on price movements over time, so based on these distinct features, we can know the psychology of investors for each asset precisely. Furthermore, previous studies often focus on the measurement and analysis of psychological factors affecting behavior on the stock market in Vietnam. In this study, we want to expand more other investment channels to get a well-rounded overview of the possibility of risk perceived by investors through methods of viewing the price charts over the period and the annual rate of return performed in the column chart of the investment channel. The objectives of our study as following:
- Identifying the factor affecting the decision to accept the risk of investors in Ho Chi Minh City and Hanoi, the threshold of risk-taking of investors for each investment channel.
- Being able to measure the overconfidence of investors by considering their expression for the asset. Although the overconfidence is an important factor affecting the risk-taking ability, the authors focus on analyzing the impact on the behavior of investors and offering solutions for better assessment as well as more accurate decision-making.
- Helping investors to have more evidence to adjust the threshold of risk-taking and ability to take a risk for popular investment channels assisting for management operation and producing a wise investment portfolio. For instance, the advisory service, the brokers can have a better understanding of their customer’s sentiment, the ability of their trade-off when participating in the investment, from which they may have the strategy and consistent advice to customers.
We focus on objects had invested over the real market, so the investors will have a more subjective view and based on experience combined with their knowledge and ability to analyze before making any decisions in situations where groups have built survey from, there may find out the factors affecting the risk-taking of investors. Besides the risk-taking, the preference for each asset in the situation which we design for the participant to allocate their budget in four investment channels is retested by regressing the preference with independent variables such as expected return and risk judgment as Weber and Hsee (Citation1998). Compared to Nosić and Weber (Citation2010), we remain the measurement for subjective expected return and also use additional expected return in a new way, the expected return that investors desired which is calculated by a percentage of profit compared with the initial invested amount by looking at four types of asset’s return, is different from previous studies. Furthermore, this study uses quantile regression to classify the ability of risk-taking into three groups by investors risk appetite (low, medium, and high risk-taking) by that way we can see more detail on each favor of risk-taking which factors affect the investors’ behavior and regarding separate favor of risk-taking with different characteristic and investment strategy, some suitable and helpful pieces of advice will be proposed to the investors. Thus, the results should not only contribute to the literature that examines the analysis of the ability of risk-taking by Vietnamese investors but also help people work in management in investment field understand some of the factors that drive investors’ behavior toward the risk-taking and the preference for a particular asset with its figures performed by price and historical return chart.
The rest of the paper is well structured as follows: Section 2 will acknowledge the existing literature while Section 3 presents the characteristics of data. In section 4, we would like to discuss research model before demonstrating our results and findings in section 5. We will close our paper with conclusions and implications in section 6.
2. Background literature
There has been a great deal of previous research in this area. One common finding is that people with stronger achievement motives tend to set the intermediate zone, where there is moderate risk. Such people are motivated to avoid failure, and will voluntarily choose activities that maximize anxiety about failure. Three relevant factors on investors’ differential risk-taking are motive, expectancy, and incentive (Atkinson, Citation1957). Research by Weber and Hsee (Citation1998) suggests that the preference for risky options is affected by risk perception and expected return. In the same vein, the risk behaviors might be the determinants of uncertainties (Strobel et al., Citation2018), which might change the financial well-being of investors (Mahdzan et al., Citation2020). Therefore, when understanding the individual risk perception, we can interpret how the institutions behave under the risking context (Ngo et al., Citation2019)
In addition, the work of Weber et al. (Citation2005) concludes that risk perception is predicted through characteristics and features such as asset name, historical volatility, and historical return. In particular, the study of Weber and Blais (Citation2006), who also contribute to financial researchers’ DOSPERT scale not only measures risk-taking in various investment sectors, but also offers insights into human psychology. Nosić and Weber (Citation2010) accept the influence of Subjective Financial Risk Attitude (SFRA) on risk-taking in the cases of stock and lotteries by asking study participants to invest their budget (10,000 euros) in a risk-free asset with a fixed rate of return or an implicitly risky asset on the basis of historical price performance data. The measurement of risk-taking, in this case, is the amount of money that participants accept to trade-off invest for the risky asset instead of investing in the risk-free asset.
Broihanne (Broihanne et al., Citation2014) discovers that the overconfidence determinants such as better than average, optimism, and miscalibration, are significant; risk perception and overconfidence strongly affect investors’ risk-taking behavior. To achieve predetermined targets, we conduct research based on three well-known hypotheses. Firstly, the preference for a choice will be influenced by two main factors: expected profit and risk perception. When choosing among options, investors use information and knowledge to compare and evaluate among choices. Risk perception attitude can be positive or negative and is spread across a weight distribution (C.K. Hsee & Weber, Citation1998). Secondly, people generally look at past events to predict the future. In addition, characteristic elements such as trends, seasonality, random noises, and cyclical changes are often treated as foundations for forecasting. Investors try to catch up with what happens in history, relying on the past and the present to make predictions for the future (Weber et al., Citation2005).
Our results also suggest that, in line with theoretical models (Odean, Citation1998), behavioral biases such as overconfidence and excessive optimism significantly affect risk behavior. Investment advisors could try to incorporate some of these findings in their advisory process by correcting for investors’ erroneous beliefs (Nosić & Weber, Citation2010). Based on our literature review, we have developed a survey to measure investors’ risk-taking tendencies concerning four assets: securities, real estate, foreign currencies, and gold. Each asset has different characteristics and price movements over time, therefore, investors’ psychological interaction to risk-taking with each asset in distinct ways.
Our paper shares similarities and differences in the study of Phan et al. (Citation2018) in some aspects. There are also the same patterns to measure factors in both two papers such as overconfidence, risk tolerance, and other personal characteristics. However, we evaluate the “miscalibration” and “optimism” in a section of overconfidence by two different approaches based on the study of Nosić and Weber (Citation2010). In addition, Phan et al. (Citation2018) indicate how to rate the willingness to take financial risks by asking participants to choose the appropriate scale from 0 (risk-averse) to 10 (risk-prone). Thus, this viewpoint is similar to us in a variable of “Subjective Financial Risk Attitude” by questioning for risk-taking behavior. However, we only use the scale from 1 (very afraid of risk) to 5 (very risk-seeking). Distinguishingly, we investigate which determinants of risk-taking behavior whereas Phan et al. (Citation2018) shed further light on factors of overtrading and under-diversification behavior. Finally, both papers contribute the empirical evidence from one emerging market with a sample of Vietnamese investors selected randomly.
Similarly, the outcomes of research from Lin (Citation2005) indicate that after a period of stock gains, overconfident traders tend to title their investment toward smaller-cap and growth stocks. Since these two kinds of stocks are riskier, these findings are consistent with the prediction of the overconfident hypothesis that as investors become overconfident, they underestimate risk and thereby trade more of riskier stocks. Interestingly, Rahman et al. (Rahman et al., Citation2017) emphasize the roles of ethical behaviors in the mutual funds market in the United States with different quantitative techniques. It also supports our existing literature review.
Previous studies often focus on the measurement and analysis of psychological factors affecting behavior in the Vietnamese stock market. In this study, we expand our view and research scope, studying how investors perceive risk when they view price charts and the annual rate of return in the column chart of the investment assets.
2.1. Prospect theory and heuristics
Daniel Kahneman (Kahneman & Tversky, Citation2013) indicates that “development theory expectations” (prospect theory) creates a solid foundation on which to build behavioral finance. Depending on the framing of a prospect, human behavior tends to express risk aversion but sometimes risk tolerance. The theory also points out that individual investors simultaneously search for risks and avoid risk.
Prospect theory is a major milestone in behavioral finance, and it is widely accepted today. Kahneman and Tversky (Kahneman & Tversky, Citation2013) suggest that prospect theory describes how people make decisions when confronted with choices under uncertainty. They point out obvious asymmetries between the values that people place on gains and losses. These asymmetries are called risk aversion.
A heuristic is a decision-making principle that utilizes a subspace of the information set. In most prospects, people have to economize and cannot analyze all contingencies; as such, we rely on heuristics, often without realizing it. For example, if you hear a strange sound while walking down the hallway, your tendency may be to run away from it until analysis can be undertaken. There is no thought here: command-and-control is entirely in the primitive emotional recesses of the brain. Later, you look around and ascertain whether the sound is a threat (Ackert & Deaves, Citation2010). The use of heuristics is often unavoidable, but often effective as well.
In the empirical literature, the risk is usually measured in two ways: either by the standard deviation of returns or by the degree of herding behavior. Based on theoretical justifications in Diamond (Diamond, Citation1989), Hirshleifer and Thakor (Citation1992); this interpretation has been used in the empirical studies of Graham (Citation1999) and Hong et al. (Citation2000). Following the prior trade of better-informed investors (Bikhchandani et al., Citation1992), if one investor is skeptical about his reasoning, he will exchange information with others or observe other investors. Through this exchange, investors will observe their own decisions. To determine the herding behavior of the participants in the survey, we provided the following questions:
1. How important are colleagues from your own company to you as a source of information?
2. How important are other market participants (not from your own company) to you as a source of information?
3. How intensively do you use the momentum strategy?
4. How intensively do you use other strategies like contrarian and buy-and-hold?
To measure questions 1 and 2, Menkhoff et al. (Citation2006) use a Likert scale to estimate how survey participants rate the level of importance of information from their colleagues (from their own company) and other market participants (not from their own company) with a scale from 1 (very important) to 6 (not important). In questions 3 and 4, participants doing this survey are given a percentage of their understanding of investment strategy, which shows proportion in each strategy. Therefore, this survey illustrates its inclination in what strategy is considered as a herding measure by Menkhoff. Menkhoff’s paper on herding suggests that the less inclined an investor is to engage in herding behavior, the more risk she is willing to take. In this study, we do not include an experience variable in the model; we want to focus on herding behavior separately to see how intensively it impacts risk-taking. As such, we change Menkhoff’s question, because his question does not apply to many investors in Vietnam.
2.2. Recent research on risk-taking
In finance, the risk of an option is equal to its variance. Weber (Weber, Citation1997; Weber & Hsee, Citation1998) measures risk perception as a variable in risk in psychology model-profit. This methodology is different between the subject as a function of the content and context of an identified situation. The risk behavior or the risk-taking on the preference of option X would be represented as following with the general trade-off model between expected benefit (hope) to option X and the perceived risk (fear of loss) of option X.
where parameter a describes the effect of the marginal value of the expected benefit of option X on risk behavior or risk-taking preference, parameter b describes the marginal effect of the perceived risk of option X on risk-taking preference and c represents the error term. This decomposition of preference provides for different methods in which the resulting domain can affect people’s choices under risk (Weber & Milliman, Citation1997).
Preferences and choices may be recognizable as results of the fact that risks and returns of similar magnitude are considered by the decision-maker across different domains; the decision-maker likes risk (positive perceived-risk coefficient b) in one domain (e.g., recreational risks) and dislikes it (negative perceived-risk coefficient) in the other domain (e.g., financial risks) (Weber et al., Citation2005). The theory of reasonable action (Ajzen & Fishbein, Citation1977) and its construction of planned behavior theory (Ajzen, Citation1991) were the most well-known models of the relationship. Attitude is defined as the logical integration of expectations and values, as in the risk-and-return-weighted integration in equation (Equation1(1)
(1) ) above. Preference for choosing X in our theoretical framework thus correlates with an attitude towards risk in option X in Ajzen and Fishbein’s theory. As described in equation (Equation1
(1)
(1) ), dispersing is the preferred choice for X (or attitudes towards choosing X, in social psychology terms). The attitude of the perception of risk (“attitude” in the theoretical framework of risk and profit) can be positive or negative, depending on the weighted allocation of the risk perception.
The phenomenon confirmed by Weber’s study is that people refer to the past performance of investment options to estimate future performance; that is, historical returns are used to estimate future returns and their likely volatility or risk. If this is correct, the format in which historical returns are presented might influence predictions of future performance. In addition, people can use information such as macroeconomic indices, expected trends, or company-specific facts to arrive at estimations of the risks and returns of investment options. Weber’s survey design a variety of conditions to test these possibilities.
Following Broihanne (Broihanne et al., Citation2014), investors are overconfident in both general and financial fields. In addition, investors are overconfident in predicting future stock prices. Broihanne only focuses on the miscalibration of probabilities and the better-than-average effect. He also interviewed 64 high-level experts and conducted face-to-face interviews to ensure that respondents did not utilize the Internet to verify their answers to the knowledge questions. The questionnaire includes 10 questions on topics, such as risk attitude, risk perception, risk-taking, overconfidence, and expectations. The questionnaire structure is close to that of Nosić and Weber (Citation2010) although adjusted for the sample of experts. The first question investigates risk perceptions, risk-taking behaviors, and risk attitudes outside of a market-related context. The Likert scale ranging from 0 to 10 is used to predict risk perception. The next questions measure the overconfidence and self-evaluation of competencies. Two sequences of 10 questions are asked, the first (second) of which contains general (finance) questions. Furthermore, participants are also required to provide the intervals within which they think the right answer is located with a 90% probability. Respondents then guess the number of answers that are contained in the intervals they provide (N_myself) and the medium number of answers contained in the intervals provided by other respondents (N_others). The better-than-average score is N_myself—N_others (Alpert & Raiffa, Citation1982; Russo & Schoemaker, Citation1992). The final questions examine predictions about future stock prices. Participants are then asked to scale their risk perception of the four assets (from 0 to 10) and to propose a portfolio allocation (between one stock and a 3% risk-free asset). Then, three prices (low, medium, high) are also used to predict the subjective expected earnings and volatility of the respondents.
Broihanne’s regression equation is written as:
where k is the number of independent variables. The study states that risk-taking is down following two measures of risk perception and respondents’ experience. Overconfidence measure has a significant positive influence on risk-taking while expected subjective volatility has a negative impact on risk-taking, optimism increases risk-taking; therefore, expected subjective returns has a significant influence on risk-taking in all models via the optimism variable. Risk perception and overconfidence strongly affect the risk-taking behaviors of experts (Broihanne et al., Citation2014).
2.3. Overconfidence and performance
Overconfidence can be understood as an overdeveloped belief about what the future will hold—and in our ability to control it. Lam et al. (Citation2010, Citation2012) indicate that investors are overconfident when they possess some private information. In these two studies, the expanded models use a pseudo-Bayesian approach, which well reflects investors’ behavioral biases. Especially, these papers demonstrate an estimation of their future investors’ acts with a strong belief to control, known as overconfidence. From the updated literature review in two scenarios including under- and overreaction, our variables, such as Miscalibration, Better than average and Excessive optimism, are based on the basic concept of market anomalies as well as asymmetric information in the market. In addition, Guo et al. (Citation2017) have extended the theory for overconfidence to include a financial crisis. During the crisis period, the volatility of assets might be higher, which leads to different investors’ behavior through their historical and expected volatility in the calculation. From another perspective to study overconfidence, Fabozzi et al. (Citation2013) also evaluate the under- and overreaction through the empirical US equity market. Then, overconfidence is one of the psychological reasons that influence investor behavior. More interestingly, the feeling of overconfidence might result in both directional and magnitude effect, which is tested by price movement. As a result, when achievement is exceptionally high, people may calculate their own performances inaccurately. They may also underestimate others more than they do normally.
- Miscalibration: when a person overestimates the accuracy of their knowledge. More precisely, if someone is asked large numbers of questions with a confidence interval of x %—then estimating correctly pointed out that x% confidence interval containing the correct answer. For example, the respondents are asked to answer these questions with multiple answers. In the next step, these people are asked about the accuracy of their answers. If they say that they believe they have answered correctly 70%, but they only achieve 55% of the correct answers, then this is the form of overconfidence, miscalibration.
- Better than average: Believing that you are better than average, especially when the definition of excellence or ability is unclear. In a survey of a group of students, 82% of people think that they among the best 30% of drivers.
- Excessive optimism: When people assess the probabilities of favorable returns too highly (or adverse returns too low), given historical experience or reasoned analysis. For example, students often expect their future test scores will be higher than their score histories would predict. After graduation, students often overestimate the number of job offers they will receive. The same tendency applies to stock market investors.
Recently, Wong et al. (Citation2018) has pointed out that the investors’ trading behavior has linked with conservative and representative heuristics in the Hong Kong market. In addition, this research emphasizes the overconfidence factor, risk-tolerant by the empirical approach of the questionnaire survey. More importantly, these authors represent that the investors will be overconfident about the past information on stocks, which leads to our fundamental estimation by looking at the price chart to predict upper- and lower bound at the defined time.
2.4. The impact of risk perception on risk-taking
In reality, risk-taking assessment is approached from many angles and motivations over time, (Atkinson, Citation1957). Risk perception measurement might be preferred as a variable in models according to Coombs Portfolio theory (Coombs, Citation1969; Coombs & Meyer, Citation1969). However, the “risk” measured in Coombs Portfolio theory is not characterized; it has since been treated as a separate question and has stimulated a long stream of research on the measurement of perceived risk. Although perceived risk might be regarded as a vague and subjective notion, empirical studies have demonstrated that people are consistently able to order lotteries with respect to their riskiness, and that risk judgment satisfies some basic axioms (e.g., De Bondt,). Perception of risk increases when there is an expansion in range, variance, or expected loss, recognition of risk increases if the returns of a lottery with zero-mean are multiplied by a positive constant greater than one (Coombs & Meyer, Citation1969). Perceived risk increases if a lottery with a mean of zero is repeated many times (Coombs & Meyer, Citation1969). These empirically verified properties provide basic guidelines for developing and evaluating measures of perceived risk (Jia et al., Citation1999). There are some updated concepts of risk perception on risk-taking, which help our literature review to build the estimation hereinafter. Firstly, Wong (Citation2007) illustrates the mean-variance criterion, which strongly links with stochastic dominance. Additionally, Guo and Wong (Citation2016) contribute the essential framework multivariate stochastic dominances for the investors’ risk preferences. Therefore, these approaches are mainly mentioned risk-taking on risk-averse and risk-loving decision-makers when investors can make a comparison between return and loss. Hence, this is the core motif for us to focus on the questionnaire survey on Vietnamese investors for rating.
3. Data
The primary data are generated by interviewing investors directly and via an electronic survey. The online survey is posted on social networks such as Facebook and investors’ forums. We collect 102 total answers for the online and offline survey, and we ultimately selected 85 qualified observations. While the current empirical literature also uses the small sample set (e.g., Rieger et al. (Citation2020) with 96 participants in online platform; Huynh (Citation2020f) with around 50 Vietnamese students per treatment), we also acknowledge that our dataset is quite small. However, there are three worth-mentioning points from this view. First, this is the first study to collect the data from real investors, who actually trade in the market. Second, having practitioners would have some certain merits for us to interpret the findings and results. For the offline survey, we approach the stock exchanges as well as real estate agency companies, banks, and other investment-related firms. Six years of secondary data (1/1/2010–1/1/2016) were chosen to form the survey. Because our survey time was 2016, the historical data for the investors to guess as well as deliver their perspective should be from 2010 to 2016. Therefore, our secondary data on survey is appropriate for investors’ evaluation. We also rely on sources such as Thomson Reuters. The stock we chose is KDC because KDC trading volume and price have high correlation with the market at the same period which is over 50%.Also, KDC is the leading company in the industry in term of market share and stock price. Regarding the price of gold, we gather information from the international XAU/VND. USD is chosen as the foreign currency; the exchange rate we use is provided by the Joint Stock Commercial Bank for Foreign Trade of Vietnam. The survey in behavioral finance research is based on previous studies such as Biais (Biais et al., Citation2005), Glaser and Weber (Citation2007) but mainly based on the study of Nosić and Weber (Citation2010) with appropriate corrections for Vietnamese markets. As the study by Nosić and Weber (Citation2010) focused on overconfidence and miscalibration factors which we want to highlight for variety of type of asset in Vietnamese markets.
We built a questionnaire to measure variables such as risk-taking (RT), risk perception (RP), expected return, subjective financial risk attitude (SFRA), better than average (BTA), miscalibration (MIS), subjective expected return (SER), subjective expected volatility (SEV), optimism (OPTI) and preference (PREF) of all four assets: gold, real estate, stocks, and foreign currency.
4. Research models
The questionnaire consists of eight pages and is divided into three main parts. Part 1 is about demographics. In part 2, respondents have presented four historical price charts and four return charts. Then, they make predictions about each asset’s price and level of risk, as well as about the allocation of assets. In part 3, we test respondents’ social knowledge and use their answers—together with the results of our survey—to measure the level of herd mentality among investors.
Part 1: The demographic variables including age, gender, monthly average income, and investors’ previous asset investments.
Part 2: In this section, the authors want to know the price predictions for individual investors in different investment channels based on historical price charts and return charts for four asset categories. Based on the given chart, participants provided 3 one-year price predictions. To ensure the objectivity of the survey, participants did not know which asset they were assessing. Similar to the study by Glaser and Weber (Citation2007), we provide asset data that fluctuated (increased, decreased, or remained unchanged) over the period. represents the importance of using the predictability of future return.
Table 1. Important results for predictability of future returns
We request the respondents make three kinds of price predictions: upper-bound price predictions, lower-bound price predictions, and their highest-confidence price predictions corresponding to r(0.95), r(0.05), and r(0.5). We use these predictions to measure subjective expected volatility (SEV) and subjective expected return (SER), following the research of Weber and Nosić (Nosić & Weber, Citation2010). For the chart of asset price volatility and the bar chart of return for each asset, we ask participants in the survey to give the lower bound, the upper bound, and a guess about whether their estimations were wrong. We use the following three prompts: “The upper bound of this type of financial asset (This is the price that you predicted; the actual price of this type of asset cannot be higher than this price in December 2016)”,“The lower bound of this type of financial asset (This is the price that you predicted; the actual price of this type of asset cannot be lower than this price in December 2016)” and “The median price (The price of the asset will fall within the two limits that are the most likely to occur in December 2016).” In addition, the researchers asked “How do you assess the risk of the asset on a scale from 0 (no risk) to 10 (very risky)?” to evaluate participants’ risk perception (RP) for each asset class. This methodology had also been used in Nosić and Weber (Citation2010). Through the charts, we also asked participants to give their expected returns for the four main assets. The expected return reflects the return that investors anticipate receiving when investing in one or more assets. We measure investors’ expected return by asking them “What is your expected return when investing in this investment” (%). To measure risk-taking, participants are offered two investment opportunities. The first choice is a chance to invest in an asset class with a risk-free interest rate of 3%/year (Nosić & Weber, Citation2010). The second choice is to invest in an investment vehicle that we provide. The risk-taking variable (RT) value ranges from 0 to 100; this figure corresponds to the ratio of the amount that the survey participants are willing to invest in this asset.
With regard to the better than average (BTA) variable, we ask subjects two questions: “For how many assets above did you predict the price correctly? (0–4)” and “In your opinion, what is the average number of correct answers for the price of assets across all respondents? (0–4)”. This variable is measured by taking the number of answers that survey participants predict correctly (N_myself) minus the average number of answers, they thought all respondents answered correctly (N_others) (Alpert & Raiffa, Citation1982; Russo & Schoemaker, Citation1992). This method is also used in the study of Broihanne (Broihanne et al., Citation2014). We also want to know the investment strategy and which asset they prefer to others. Preference (PREF) is a dependent variable in the regression model; it is used to confirm the equation of C.K. Hsee and Weber (Citation1998). We assume that surveyed participants have a budget of 100 million dong for investment and they would distribute this money among four asset choices. The PREF variable ranges from 0 to 100 corresponding to the amount of money the investor pays for each type of asset.
Finally, we evaluate subjective financial risk attitude (SFRA) by asking participants to rate their willingness to take financial risks on a scale from 1 (very afraid of risk) to 5 (very risk-seeking) (Nosić & Weber, Citation2010). The classification methodology is simple, fast, and commonly used in investment consulting. We measure these variables to evaluate the correlation between cognitive ability to take risks and risk-taking behaviors in practice. We calculate two variables—the subjective expected return (SERi) and the subjective expected volatility (SEVi)—based on a formula proposed by Keefer and Bodily (Citation1983):
Following Nosić and Weber (Citation2010), with the subjective expected volatility (SEV) we also measure an easily interpretable and standardized measure of miscalibration (MIS) by dividing the estimated one-year volatility (HV) by minus one times the historical volatility. This miscalibration measure is close to 0 for excessively overconfident subjects and equal to −1 for perfectly calibrated subjects. Below is the formula:
Furthermore, we calculate a subject’s optimism (OPTI) regarding the return of each asset as the difference between the subjective expected return (SER) and the historical return (HR) (Nosić & Weber, Citation2010) which is also a crucial factor in overconfidence variables set. The higher the value is, the greater the level of optimism is. The formula is given below:
In our survey, assets one through four are stock, gold, real estate, and foreign currency. We do not show the participant the name of the assets. Based on four different features of nameless assets, we can measure investors’ behavior more objectively and reflect on how they approach risk.
Part 3: In this section, the authors give 10 questions about social knowledge such as “The inventor of the telephone, Alexander Graham Bell, is from which country?” In addition, we give reference answers. To the above question, we give the four following results: 67.5% of chose answer A (England), 7.5% chose B (France), 11.3% chose C (America) and 13.7% chose D (Germany). If the interviewees use the reference results, they would mark X in the “Yes” box; if they did not, they would mark X in the “No” box. If the participants use and copy the reference answers, they are influenced by herding behavior. Then, the number of answers that participants use the reference results is evaluated as herding behavior variable (HB).
For each asset, we conduct regressions with variable risk-taking (RT) on each asset, and the independent variables were risk perception, expected return, and Subjective Financial Risk Attitude (SFRA). This model measures the impact of awareness of risks in the process of risk-taking; it is mentioned in the study by Weber et al. (Weber et al., Citation2005). We also input more independent variables such as Subjective Expected Return (SER), Subjective Expected Volatility (SEV), and Better Than Average (BTA). These variables are also put into Broihanne’s model (Broihanne et al., Citation2014) to estimate Risk-taking. Finally, the herding behavior (HB) variable is also put into our model to determine whether it affects risk-taking or not. summarizes our main variables that can be used in the following models. Therefore, we have four models from regressing four times across four assets:
Table 2. Main variables in the research model
Model 1: RTi = β1+ β2*RPi + β3*RETURNi + β4*BTA + β5*SFRA+ β6*HB + β7*SEVi + β8*SERi + εi .
Weber (Weber & Hsee, Citation1998) suggests that in the next step, we should test the model. The Preference (Pref) of each asset would be affected by two variables: Risk Perception (RP) and Expected Return (RETURN) (C.K. Hsee & Weber, Citation1998):
Model 2: PREFi = β1+ β2*RPi + β3*RETURNi + εi .
We integrate these regression models with all kinds of assets. The dependent variables of this model are Risk-taking (RT), Subjective Expected Return (SER), Subjective Expected Volatility (SEV), Risk Perception (RP). In addition, we use the set of independent variables representing overconfidence such as Miscalibration (MIS), Optimism (OPTI), and Financial Risk subjective Attitude (SFRA) through the model based on the study of Weber et al. (Citation2005) and Vrecko et al. (Citation2009). Lastly, Better Than Average (BTA) is an element demonstrating for the herding behavior (HB) in accordance with the research of Broihanne (Broihanne et al., Citation2014) as follows:
Model 3: RTi = β1+ β2*RPi + β3*RETURNi + β4*BTA + β5*SFRA+ β6*HB + β7*SEVi + β8*SERi + β9*MISi + β10*OPTIi + εi .
In this research, we just put more attention on the regression for each asset rather than the integrated regression model for all assets. In our model, we want to synthesize all the elements of investor confidence (OPTI, MIS, BTA). The overconfidence values were estimated from the survey to see if the confidence factor affects the decision to invest or not.
Model 4: RTi = β1+ β2*BTA + β3*MISi + β4*OPTIi + εi .
5. Empirical results
5.1. Description of statistics
Before discussing the descriptive statistics of variables, we would like to summarize our analysis of respondent demography.
5.2. Descriptive statistics of age
In the survey, the research team approaches interviewees whose ages are ranging from 20 to 51 years old. Overall, according to the statistic table, the average age of investors interviewed is about 30 years old, and we undertake all the interviewees are the investor who invested yet at least one asset. In addition, this range of age provides us the various investor’s style because we do not only have the experienced investor we also have the newbie investors (aged 20). Thus, it does not occur in the representative phenomenon in our survey result, which means that there is no group of characters than others. In addition, at the age of 30, people are usually at work and looking for an investing channel for extra return exceptionally the monthly salary.
5.3. Descriptive statistics of monthly average income, gender, and asset investor had invested
According to the result generated from investors’ information. It can be seen that the largest number of investors earn more than 8,000,000 VND per month accounting for 67% out of the total number. There is only about 4.7% of investors having less than 3,000,000 VND of income monthly. In terms of the gender of interviewees, it is shown that nearly 70% of investors are men and the rest of about 30% are women. It can be inferred that the proportion of female investors are more than women investors in our survey and generally in Vietnam. When conducting the survey in the realistic company as joint securities companies, real estate transaction companies, etc. We realized almost the participants are male, because men are usually interested in the financial market, regardless that their occupations are, they want to join the market and look for chances to invest. Participants in our survey are included the company’s staff, they do not only give advice and consult to customers but they are also investors trading in the real market. Out of four types of assets, namely, stock, gold, real estate, and foreign currency, investors tend to spend money on stock the most (76.5%), this may be the most common channel for investors to approach easily. Besides, foreign currency with the figure standing at approximately 43.5% can be another way to invest. In addition, the two other asset investors should consider as making a decision to invest are gold and real estate which make up about 30% and 24%, respectively, among 4 kinds of assets, according to the present information from the table above. present the description of the statistics for the variables we used in our paper.
Table 3. Descriptive statistics of age
Table 4. Descriptive statistics of monthly average income, gender, and asset
Table 5. Description of statistics of variables
Descriptive statistics of stock: Investors’ willingness to take risk is in the medium range at nearly 39%; the amount of money that people invest in stock is about 40%. Investors are willing to take a risk for the probability of return—26% on average. In addition, the mean of risk perception is quite high: 6.7 on average, respectively, while the maximum levels are 10. For the risk perception and subjective financial risk attitude variables, the statistical table shows an average positive effect of more than 50% on the decision to invest in a stock.
Descriptive statistics of gold: Approximately 36% of the money that investors want to trade-off and take a risk to invest in gold instead of a risk-free asset. Investors perceive the riskiness of gold as average (5), so the expected return and the preference for gold investment are quite high on average (17%, 26%). Some investors have a negative view of the price of gold, expecting the minimum return as −8.3%. We can conclude that the investors who are interested in gold will invest considerable amounts, leading to a high average preference (26%).
Descriptive statistics of real estate: The average risk-taking rate of investors is quite low—about 24%—despite the fact that they do not perceive the risk in this asset as very high. Perhaps they do not take a risk for assets like real estate that do not provide high profits. From the chart on real estate, it is clear that volatility is not high; stable prices during the period made this investment choice less attractive to investors.
Descriptive statistics of foreign currency: the average risk perception for this asset is not high. In comparison with other assets, foreign currency is more attractive to investors; the rate of risk-taking is highest and the preference for this asset is high—approximately 33%.
5.4. Risk-taking for each asset
Risk-taking in stock: Risk recognition has significance at 10% level (p-value <10%); risk perception factors negatively affect investors’ risk-taking. Specifically, the higher risk the investors perceive, the less money they want to invest in stock. SFRA variable shows a positive influence on risk-taking at significance level 1% (p-value<1%). The subjective assessment of investors in financial risk situations only affects their behavior in stock channel.
Risk-taking in gold: In terms of gold, the dependent variables in the model are explained by the independent variables 10.89%. Investors’ risk recognition has a negative impact on their willingness to take risks. Gold is considered one of the most attractive investment because of its necessity and worldwide usability. When confront with the price chart, however, investors do not know which asset is which, so their behavior reflects the situation: selecting an asset when risk perception is low. The more risk they perceive, the less they want to invest in gold. Moreover, the expected return and the prediction of investors cannot account for their risk-taking behavior in gold.
Risk-taking in real estate: With regard to real estate, the expected return and subjective expected return have statistical significance at 1% and 5%, respectively (p-value <1%), expected return factors that positively impact investors’ willingness to take risks. Surprisingly, in this case, the investors take the risk regardless of their awareness of their assets’ hazard.
Risk-taking in foreign currency: With regard to risk-taking in foreign currency, the estimated results of the OLS regression model showed a p-value <0.05; therefore, the model is applicable and statistically significant at a 5% significance level. As for the final results, risk perception has significance at 1% level (p-value<1%), and this impact is inverse: awareness of risk factors negatively impact investors’ willingness to take risks.
There is no multicollinearity because the variance inflation factor (VIF) is under the threshold (normally 10) in . Only two of the four models suffer from heteroskedasticity. Therefore, we propose the quantile regression for the error model in order to correct this phenomenon and evaluate the influence on each quantile clearly.
Table 6. Result of OLS regression on risk-taking for each asset
5.5. Result of preference for each asset
Preference for stock: As we can see from the , the result of White’s test shows that p-value 0.0006 < 0.05, so we reject H0: homoskedasticity. The regression model for preference for stock suffers from heteroskedasticity.
Table 7. Result of OLS regression on preference to each asset
Preference for gold: According to the estimated results of the OLS model, p-value > 0.05; therefore, the model is not significant. The preference for gold cannot be explained by risk perception and expected return in this model.
Preference for real estate: According to the estimated results of the OLS model, p-value <0.05; therefore, the model is statistically significant at a 5% level. The adjusted R2 is 7.19%, meaning that the independent variables in the model explain the dependent variable. Next, considering the impact of the independent variables on the dependent variable, the expected return is significant at 1% (p-value <1%). The expected return has a positive impact on investors’ preferences.
Preference for foreign currency: Risk perception and expected return have statistical significance at 5% and 10%, respectively. Awareness of risk factors has a negative impact on investors’ willingness to take risks, whereas the expected return has a positive effect on risk-taking.
The preference for stock and gold cannot be explained by the expected return and risk perception in this case. In the preference to the investment, the channel is not always impacted by concurrent risk perception and expected return except the foreign currency channel. The results of the quantile regression models () with three levels of decile τ = 0.25, 0.5, 0.75 provide more detail about investors’ willingness to accept risk. Within each group of investors, we can segment into three groups (τ = 0.25: low risk-taking group; τ = 0.5: medium risk-taking group; τ = 0.75: high risk-taking group).
Table 8. Result of quantile regression on risk-taking with τ = 0.25; 0.5; 0.75
Table 9. Results of quantile regression on risk-taking with variables in overconfidence
5.6. Result of three levels of risk-taking
Within the quantile regression analysis in , τ = 0.25 is a surprise: it represents the segment of investors who tend to seek safer investments. The variable HB: herd mentality is also statistically significant when put into the model for risk-taking, and shows a positive impact on willingness to take the risk. To be more detailed, the more herding-oriented investors are willing to pay more money for a given asset. To put it in another way, people who tend to incline toward others’ ideas have a tendency to take less risk than others. This was confirmative research done by (Dickason & Ferreira, Citation2018) who also found that conservative investors with low-risk tolerance levels focus on mental accounting bias which is represented as expected return variable.
At τ = 0.5, two factors impact risk-taking: awareness about risk and return expectations. This group of investors may have negative attitudes toward investing in high-risk assets, but if they believe that the rate of return will increase in the future, they are willing to take a risk and invest more. At τ = 0.75, investors’ behavior is quite similar to that of the medium risk-taking group. They are willing to take risks at high rates with the assets we provided, which means they do not prefer the free risk-taking asset over the asset with implicit risk. These investors are open to riskier investments; return expectations also positively impact risk-taking in this group (p-value < 1%). Investors who are interested in risky investments also believe that their investments will earn a profit, which is the reason they are willing to invest.
The group of overconfidence factors including better than average, miscalibration, and excessive optimism for all three levels of risk-taking in . We discover the miscalibration is the main factor that contributes to the willingness to take risks. In addition, excessive optimism also impacts risk-taking for medium- and high-risk investors. Therefore, optimism about risky assets can positively affect risk-taking, which means that investors’ risk-taking behavior takes little account of the historical status of the asset; investors believe that there will be a large price change in the future. According to the results quantile regression, the BTA variable is not significant at all which can be justified for our measure for the BTA variable that may not be appropriate or the form of overconfidence or better than average factor is really not the determinant impacting the risk-taking ability of investors. This result is in line with conclusion by Zia et al. (Citation2017) that the relation between loss realization and overconfidence bias due to excessive trading in financial market.
6. Conclusions and implications
The expected return is the crucial factors that all three risk-level investors consider for investment while herding behavior is exclusively useful for risk-taking decision by safe-oriented investors. Investors’ profit-related goals also influence the decision to accept risks. We also find evidence that most investors in Vietnam exhibit risk-averse behavior; they are generally unwilling to invest in risky assets. They avoid that chance to seek for another safer option; the coefficient of perceived risk is negative, which means they are risk-averse (C.K. Hsee & Weber, Citation1998). The results also show that when we add the asset’s return chart or reformat the information display, Vietnamese investors’ risk-taking is still not much affected; in fact, they are affected by even fewer factors comparing with previous studies.
For most assets, risk perception is the main determinant affecting investors’ risk-taking. Stock, currency, and gold have high volatility, and this is visually evident on the price and return charts, so investors had good reason to consider these assets risky. In the case of gold, however, prices are more stable; this likely creates trust among investors. Risk perception for this asset is also relatively low. Gold investors also tend to believe that upward trends in the past will continue in the future. Unlike gold, results of real estate indicate that investors are more likely attracted by return regardless of their perceived risk on this asset meaning the risk perception does not play role in decision-making, the price chart of real estate only makes investors’ behavior tempted by the expected return.
Investors’ asset preferences are really affected by two determinants: expected return and risk perception. For real estate, the only expected return factor impacts investors’ decisions. For foreign currency, investor’s willingness to invest is influenced by both risk perception and expected return, as in the model proposed by C.K. Hsee and Weber (Citation1998). Although investment preferences are not always impacted by both risk perception and expected return, our results show that these relationships generally hold.
Overconfidence—especially miscalibration and excess optimism—affects risk-taking enormously. The level of overconfidence differs across Vietnamese investors; in general; however, risk-taking is influenced by overconfidence factors. With regard to excess optimism, investors overvalue the future. While they are difficulty predicting the future or making an investment decision, they usually believe that a positive scenario will take place. The behavior of low-risk-taking investors could be affected by herding behavior; brokers or salesmen in the investment field should focus on demonstrating the credibility and potential of investments. The reference price chart is not the way to display the data; clearly, we have to manage overconfidence levels in other, more effective ways.
Our results indicate that risk perception is the main determinant and negatively impacts risk-taking, which is in line with previous researches by Nosić and Weber (Citation2010) conducted in the German market, Broihanne (Broihanne et al., Citation2014) conducted in the French market. Compared to Nosić and Weber (Citation2010), we remain the measurement for subjective expected return and also use additional expected return in a new way, and our empirical findings that these factors also have a positive impact on risk-taking of Vietnamese investors in this specific case. With respect to overconfidence, we find that miscalibration and optimism have a positive and significant impact (consistent with the results of Nosić and Weber (Citation2010). However, in Broihane’s findings (Broihanne et al., Citation2014), Optimism also has a positive impact, while miscalibration has a negative impact because it is only based on knowledge questions. The presence of the herding behavior variable also has a positive impact on investors’ risk-taking. The insignificant subjective expected volatility variable cannot explain the risk-taking behavior and also differs from previous studies’ results. To sum up, determinants impacting the Vietnamese investors’ risk-taking are different from other markets, especially considering subjective expected return and volatility, subjective financial risk attitude though the overconfidence factors show the persistent impact on risk-taking across different markets. We also suggest further research in this behavioral economics by expanding the samples of interviews, adding more factors regarding herding with a more logical way of determining behavior. Although the expansion of four investment channels determines factors in each specific case, there is a variety of measurement of risk-taking and impacting determinants as well. We need to consider the diversifying approach and calculation for each variable for each asset not as only a given price chart as our research. We also acknowledge our limitation of small sample dataset. The future research in risk perception for Vietnamese investors can be extended up to nearly 500 respondents (Ha et al., Citation2020). Another limitation is that the different variables’ measure scales could lead to bias results. We leave this avenue for the future directions to construct the consistent scale, which could improve the estimated results by comparing the similarities and differences in different range of measured scales.
Finally, the future research can also be emphasized how the risk perception has changed due to the appearance of newly disease, COVID-19 pandemic (Huynh, Citation2020b, Citation2020c; T. L. Huynh, Citation2020a), the role of cultural dimension (Huynh, Citation2020d) or the new financial classes, Bitcoin, (Burggraf et al., Citation2020). It will be a fruitful avenue for those who explain the relationship between risk-perception and asymmetric information (Huynh et al., Citation2020e) as well as the honest behavior (Huynh, Citation2020f) to mitigate the fraud in financial markets.
Acknowledgements
The authors would like to thank Thong Trung Nguyen, Phuoc Huu Le, Tuyet Anh Vuong, Hanh Thi Dinh, and Nga Thi Thanh Nguyen for their helpful comments, suggestions, and encouragement. The first author acknowledges the support from Foreign Trade University (Vietnam). The third author would like to thank Robert B. Miller and Howard E. Thompson for their continuous guidance and encouragement. This research is funded by Foreign Trade University, University of Economics Ho Chi Minh City, Asia University, China Medical University Hospital, The Hang Seng University of Hong Kong, the Research Grants Council of Hong Kong (Project Numbers 202809, 12502814, and 12500915), and Ministry of Science and Technology (MOST, Project Numbers 106-2410-H-468-002 and 107-2410-H-468-002-MY3), Taiwan.
Additional information
Funding
Notes on contributors
Linh Duy Bui
Linh Duy Bui is the lecturer of Foreign Trade University (Hanoi). His research area is Supply Chain and Logistics, International Trade and Microeconomics. He published on the Asian Journal of Shipping and Logistics, International Journal of Economics and Business Research. Chi Trung Le graduated Faculty of Finance, Banking University and Anh Huynh Ngoc Quang is staff at School of Banking, University of Economics Ho Chi Minh City (Vietnam). He published a paper at International Journal of Economics and Business Research. Professor WONG, Wing Keung obtained his PhD from the University of Wisconsin-Madison, USA with major in Business Statistics (Statistics and Finance) and obtained his Bachelor’s degree from the Chinese University of Hong Kong, Hong Kong, with major in Mathematics and a double minor in Economics and Statistics. Currently, he is a Chair Professor at the Department of Finance, Asia University. He was Full Professor at the Department of Economics, Hong Kong Baptist University and Deputy Director in at Risk Management Institute, National University of Singapore.
References
- Ackert, L. F., & Deaves, R. (2010). Decision-making and markets. Behavioral Finance Psychology, 425, 86–23. USA: Cengage Learning. https://www.cengage.com/c/behavioral-finance-psychology-decision-making-and-markets-1e-ackert/9780324661170PF/
- Ajzen, I. (1991). The theory of planned behavior. Organizational Behavior and Human Decision Processes, 50(2), 179–211. https://doi.org/10.1016/0749-5978(91)90020-T
- Ajzen, I., & Fishbein, M. (1977). Attitude-behavior relations: A theoretical analysis and review of empirical research. Psychological Bulletin, 84(5), 888–918. https://doi.org/10.1037/0033-2909.84.5.888
- Alpert, M., & Raiffa, H. (1982). A progress report on the training of probability assessors. In D. Kahneman, P. Slovic, & A. Tversky (Eds.), Judgment under uncertainty: Heuristics and biases (pp. 294–305). Cambridge University Press.
- Atkinson, J. W. (1957). Motivational determinants of risk-taking behavior. Psychological Review, 64(6, Pt.1), 359–372. https://doi.org/10.1037/h0043445
- Biais, B., Hilton, D., Mazurier, K., & Pouget, S. (2005). Judgemental overconfidence, self-monitoring, and trading performance in an experimental financial market. Review of Economic Studies, 72(2), 287–312. https://doi.org/10.1111/j.1467-937X.2005.00333.x
- Bikhchandani, S., Hirshleifer, D., & Welch, I. (1992). A theory of Fads, fashion, custom, and cultural change as informational cascades. Journal of Political Economy, 100(5), 992–1026. https://doi.org/10.1086/261849
- Broihanne, M. H., Merli, M., & Roger, P. (2014). Overconfidence, risk perception and the risk-taking behavior of finance professionals. Finance Research Letters, 11(2), 64–73. https://doi.org/10.1016/j.frl.2013.11.002
- Burggraf, T., Huynh, T. L. D., Rudolf, M., & Wang, M. (2020). Do FEARS drive Bitcoin? Review of Behavioral Finance, ahead-of-print(ahead–of–print). https://doi.org/10.1108/RBF-11-2019-0161
- Coombs, C. H. (1969). Portfolio theory: A theory of risky decision making. La Decision, Centre National de la Recherche Scientifique.
- Coombs, C. H., & Meyer, D. E. (1969). Risk-preference in coin-toss games. Journal of Mathematical Psychology, 6(3), 514–527. https://doi.org/10.1016/0022-2496(69)90021-2
- Diamond, D. (1989). Reputation acquisition in debt markets. Journal of Political Economy, 97(4), 828–862. https://doi.org/10.1086/261630
- Dickason, Z., & Ferreira, S. (2018). Establishing a link between risk tolerance, investor personality and behavioural finance in South Africa. Cogent Economics & Finance, 6(1). https://doi.org/10.1080/23322039.2018.1519898
- Fabozzi, F. J., Fung, C. Y., Lam, K., & Wong, W. K. (2013). Market overreaction and underreaction: Tests of the directional and magnitude effects. Applied Financial Economics, 23(18), 1469–1482. https://doi.org/10.1080/09603107.2013.829200
- Glaser, M., & Weber, M. (2007). Overconfidence and trading volume. Geneva Risk Insurance Review, 32(1), 1–36. https://doi.org/10.1007/s10713-007-0003-3
- Graham, J. R. (1999). Herding among investment newsletters: Theory and evidence. Journal of Finance, 54(1), 237–268. https://doi.org/10.1111/0022-1082.00103
- Guo, X., McAleer, M., Wong, W. K., & Zhu, L. X. (2017). A Bayesian approach to excess volatility, short-term under reaction and long-term overreaction during financial crises. North American Journal of Economics and Finance, 42, 346–358. https://doi.org/10.1016/j.najef.2017.08.001
- Guo, X., & Wong, W. K. (2016). Multivariate stochastic dominance for risk averters and risk seekers. RAIRO - Operations Research, 50(3), 575–586. https://doi.org/10.1051/ro/2016026
- Ha, T. M., Shakur, S., & Pham Do, K. H. (2020). Food risk in consumers’ eye and their consumption responses: Evidence from Hanoi survey. Journal of Asian Business and Economic Studies, ahead-of-print(ahead–of–print), Vol. ahead-of-print No. ahead-of-print. https://doi.org/10.1108/JABES-12-2019-0126
- Hirshleifer, D., & Thakor, A. V. (1992). Managerial conservatism, project choice, and debt. Review of Financial Studies, 5(3), 437–470. https://doi.org/10.1093/rfs/5.3.437
- Hong, H., Kubik, J. D., & Solomon, A. (2000). Security analysts career concerns and herding of earnings forecasts. RAND Journal of Economics, 31(1), 121–144. https://doi.org/10.2307/2601032
- Hsee, C. K., & Weber, E. U. (1998). Cross-cultural differences in risk perception,but cross-cultural similarities in attitudes towards perceived risk. Management Science, 44(9), 1205–1217.
- Huynh, T. L. (2020a). The COVID-19 risk perception: A survey on socioeconomics and media attention. Econ. Bull, 40(1), 758–764. http://www.accessecon.com/Pubs/EB/2020/Volume40/EB-20-V40-I1-P64.pdf
- Huynh, T. L. D. (2020b). Data for understanding the risk perception of COVID-19 from Vietnamese sample. Data in Brief, 30(June), 105530. https://doi.org/10.1016/j.dib.2020.105530
- Huynh, T. L. D. (2020c). “If you wear a mask, then you must know how to use it and dispose of it properly!”: A survey study in Vietnam. Review of Behavioral Economics, 7(2), 145–158. https://doi.org/10.1561/105.00000121
- Huynh, T. L. D. (2020d). Does culture matter social distancing under the COVID-19 pandemic? Safety Science, 130(October), 104872. https://doi.org/10.1016/j.ssci.2020.104872
- Huynh, T. L. D. (2020f). Replication: Cheating, loss aversion, and moral attitudes in Vietnam. Journal of Economic Psychology, 78(June), 102277. https://doi.org/10.1016/j.joep.2020.102277
- Huynh, T. L. D., Wu, J., & Duong, A. T. (2020e). Information asymmetry and firm value: Is Vietnam different? The Journal of Economic Asymmetries, 21(June), e00147. https://doi.org/10.1016/j.jeca.2019.e00147
- Jia, J., Dyer, J. S., & Butler, J. C. (1999). Measures of perceived risk. Management Science, 45(4), 519–532. https://doi.org/10.1287/mnsc.45.4.519
- Kahneman, D., & Tversky, A. (2013). Prospect theory: An analysis of decision under risk. In Handbook of Fundamentals of Financial Decision Making, Part I (pp. 99–127). Worldscientific.
- Keefer, D. L., & Bodily, S. E. (1983). Three-point approximations continuous random variables. Management Science, 29(5), 595–609. https://doi.org/10.1287/mnsc.29.5.595
- Lam, K., Liu, T. S., & Wong, W. K. (2010). A pseudo-Bayesian model in financial decision making with implications to market volatility, under- and overreaction. European Journal of Operational Research, 203(1), 166–175. https://doi.org/10.1016/j.ejor.2009.07.005
- Lam, K., Liu, T. S., & Wong, W. K. (2012). A new pseudo Bayesian model with implications to financial anomalies and investors behaviors. Journal of Behavioral Finance, 13(2), 93–107. https://doi.org/10.1080/15427560.2012.680993
- Li, B., Wang, J., Huang, D., & Hoi, S. C. (2018). Transaction cost optimization for online portfolio selection. Quantitative Finance,18(8), 1411–1424.
- Lin, M. C. (2005). Returns and investor behavior in Taiwan: Does overconfidence explain this relationship? Review of Pacific Basin Financial Markets and Policies, 8(3), 405–446. https://doi.org/10.1142/S0219091505000476
- Mahdzan, N. S., Zainudin, R., Abd Sukor, M. E., Zainir, F., & Ahmad, W. M. W. (2020). An exploratory study of financial well-being among Malaysian households. Journal of Asian Business and Economic Studies, 27(3), 285–302. https://doi.org/10.1108/JABES-12-2019-0120
- Menkhoff, L., Schmidt, U., & Brozynski, T. (2006). The impact of experience on risk taking, overconfidence, and herding of fund managers: Complementary survey evidence. European Economic Review, 50(7), 1753–1766. https://doi.org/10.1016/j.euroecorev.2005.08.001
- Ngo, T. T., Le, M. Q., & Ngo, T. P. (2019). Incorporating risk into technical efficiency for Vietnam’s and ASEAN banks. Journal of Asian Business and Economic Studies, 26(1), 2–16. https://doi.org/10.1108/JABES-10-2018-0083
- Nosić, A., & Weber, M. (2010). How riskily do i invest? The role of risk attitudes, risk perceptions, and overconfidence. Decision Analysis, 7(3), 282–301. https://doi.org/10.1287/deca.1100.0178
- Odean, T. (1998). Volume, volatility, price, and profit when all traders are above average. Journal of Finance, 53(2), 1887–1934. https://doi.org/10.1111/0022-1082.00078
- Phan, T. C., Rieger, M. O., & Wang, M. (2018). What leads to overtrading and under-diversification? Survey evidence from retail investors in an emerging market. Journal of Behavioral and Experimental Finance, 19, 39–55. https://doi.org/10.1016/j.jbef.2018.04.001
- Rahman, S., Lee, C.-F., & Xiao, Y. (2017). The investment performance, attributes, and investment behavior of ethical equity mutual funds in the US: An empirical investigation. Review of Quantitative Finance and Accounting, 49(1), 91–116. https://doi.org/10.1007/s11156-016-0581-1
- Rieger, M. O., Nguyen, T. M., Schnur, B., & Wang, M. (2020). Taking more risk tomorrow: Time horizons and investment decisions. Applied Economics Letters, 28(6), 1–5. https://doi.org/10.1080/13504851.2020.1761522
- Russo, J. E., & Schoemaker, P. J. (1992). Critical IT (information technology) issues: The next ten years.. Sloan Management Review, 33(4), 7–17.
- Shiller, R. C. (2000). Irrational exuberance. Philosophy and Public Policy Quarterly,20(1), 18–23.
- Strobel, J., Salyer, K. D., & Lee, G. S. (2018). Uncertainty, agency costs and investment behavior in the Euro area and in the USA. Journal of Asian Business and Economic Studies, 25(1), 122–143. https://doi.org/10.1108/JABES-04-2018-0007
- Vrecko, D., Klos, A., & Langer, T. (2009). Impact of presentation format and self-reported risk aversion on revealed skewness preferences. Decision Analysis, 6(2), 57–74. https://doi.org/10.1287/deca.1090.0141
- Weber, E. U. (1997). The utility of measuring and modeling perceived risk. In A. A. J. Marley (Ed.), Choice, Decision, and Measurement: Essays in Honor of R. Duncan Luce (45–57). Erlbaum. https://www8.gsb.columbia.edu/researcharchive/articles/12859
- Weber, E. U., & Blais, A. R. (2006). A Domain-Specific Risk-Taking (DOSPERT) scale for adult populations. Judgment and Decision Making, 1(1), 33–47. http://journal.sjdm.org/06005/jdm06005.htm
- Weber, E. U., & Hsee, C. K. (1998). Cross-cultural differences in risk perception, but cross-cultural similarities in attitudes towards perceived risk. Management Science, 44(9), 1205–1218. https://doi.org/10.1287/mnsc.44.9.1205
- Weber, E. U., & Milliman, R. (1997). Perceived risk attitudes: Relating risk perception to risky choice. Management Science, 43(2), 122–143. https://doi.org/10.1287/mnsc.43.2.123
- Weber, E. U., Siebenmorgen, N., & Weber, M. (2005). Communicating asset risk: How name recognition and the format of historic volatility information affect risk perception and investment decisions. Risk Analysis, 25(3), 597–609. https://doi.org/10.1111/j.1539-6924.2005.00627.x
- Wong, W. K. (2007). Stochastic dominance and mean-variance measures of profit and loss for business planning and investment. European Journal of Operational Research, 182(2), 829–843. https://doi.org/10.1016/j.ejor.2006.09.032
- Wong, W. K., Chow, S. C., Hon, T. Y., & Woo, K. Y. (2018). Empirical study on conservative and representative Heuristics of Hong Kong small investors adopting momentum and contrarian trading strategies. International Journal of Revenue Management, 10(2), 146–167. https://doi.org/10.1504/IJRM.2018.091836
- Wongchoti, U., Tian, G., Hao, W., Ding, Y., & Zhou, H. (2020). Earnings quality and crash risk in China: An integrated analysis. Journal of Asian Business and Economic Studies, 28(1), 2–19. https://doi.org/10.1108/JABES-02-2020-0012
- Zia, L., Sindhu, M., Hashmi, S., & Zhang, X. (2017). Testing overconfidence bias in Pakistani stock market. Cogent Economics & Finance, 5(1), 1289656. https://doi.org/10.1080/23322039.2017.1289656.