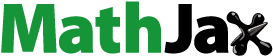
Abstract
This research examines whether Google search volume index (GSVI), a proxy of investor attention, can predict the excess returns and abnormal trading volumes of TPEx 50 index constituents. It also explores the motive underlying GSVI based on positive or negative shocks to stock prices. The empirical data include 48 companies from TPEx 50 index constituents and cover a period from 1 September 2016 to 31 August 2019. The empirical results present that (1) lagged GSVI negatively affects current excess returns, perhaps due to the characteristics of TPEx, in which there are a higher proportion of retail investors, smaller listed companies, and a higher information asymmetry problem. (2) Lagged GSVI can positively affect abnormal current trading volumes. (3) If GSVI is driven by positive shocks, then it can predict excess returns and abnormal trading volumes positively.
Public interest statement
The Google Trends tool provides google search volume index (GSVI) for one or more keywords. Existing studies suggest that retail investors’ attention can be gauged by GSVI and that GSVI can forecast stock returns and trading volumes. Our paper aims to examine the effects of GSVI on stock returns and trading volumes in a market dominated by retail investors, i.e. the Taipei Exchange (TPEx). In contrast to most literature, we find that GSVI negatively affects future returns (1-12 weeks later). That could be because when retail investors with lagging information pay attention to stocks, stock returns are likely to reverse. With respect to trading volume, when investors receive favorable news and generate searches, they can make buying decisions according to the search results, resulting in abnormal growth in share trading volume. In contrast, investors may not be used to shorting in response to unfavorable news, therefore no apparent growth occurs in stock trading volume.
1. Introduction
A traders market in a stock market is classified as institutional investors or retail investors whose investment styles greatly differ. Institutional investors usually represent informed traders who are sophisticated about industry trends. By contrast, with thousands of listed companies and a vast amount of information, understanding the fundamentals of all companies is challenging for most retail investors. Barber and Odean (Citation2008) note that retail investors tend to buy stocks that often appear in the news, stocks with abnormally high trading volumes, or stocks with extremely high returns, meaning that retail investors prefer to buy stocks of companies that attract attention.
How to measure investors’ attention has accordingly been an important issue over the last decade. On the basis of website search behavior, Da et al. (Citation2011) first use the Google search volume index (GSVI) as a proxy variable of investor attention to study the relationship between investor attention and stock returns.Footnote1 Ben-Rephael et al. (Citation2017) also directly define GSVI as the proxy variable of retail investor attention, which is used to distinguish it from the attention of institutional investors.Footnote2 Thus, GSVI has become a well-accepted proxy variable of investor attention in the literature (Chen et al., Citation2021; Rizkiana et al., Citation2019; Sifat & Thaker, Citation2020).
The present research further explores the relationship between GSVI, stock returns, and trading volume of TPEx 50 Index constituents in Taiwan. As aforementioned, GSVI can gauge retail investor attention instead of institutional investor attention. This paper thus chooses the Taiwan stock market as our target market because there is a very high proportion of retail investors in Taiwan (Chang, Citation2021). Accordingly, we suggest that the effect of GSVI would be more pronounced in a retail investor dominant market. Moreover, TPEx 50 Index constituents are the top 50 largest companies listed on the main board of Taipei Exchange (TPEx), formerly called the over-the-counter (OTC) market in Taiwan. TPEx is the second large market in comparison to Taiwan Stock Exchange (TWSE). The structure of TPEx, such as investors and listed companies, greatly differs from that of TWSE. More specifically, from 2020 statistics the proportion of institutional investors in TWSE accounted for 37.8%, while domestic retail and institutional investors accounted for 87.8% of the total trading volume in the TPEx market. This means that the TPEx market is clearly favored by local investors, and the trading proportion of domestic retail investors is as high as 78.8%.
The listed stocks in the two markets are also different. The stocks in the TPEx mainly belong to small- and medium-sized companies with relative young and illiquid characteristics. The media exposure of TPEx is extremely lesser than that of the large-capitalization stocks in TWSE. The average daily trading volume of TPEx during 2010–2019 was only 24.5% that of TWSE. We consider that information on TPEx stocks is relatively uncirculated and therefore refer to Da et al. (Citation2011) by using GSVI as the proxy variable of investor attention to observe whether it can predict excess returns and abnormal trading volumes of TPEx 50 Index constituents, which are listed in a stock market dominated by retail investors in Taiwan.
This paper benefits the related literature in two ways. First, to our best knowledge, this paper is the first to examine the impact of GSVI in a market with an extremely large proportion of retail investors. It is believed that investor attention affects stock returns and trading volumes when most investors are uninformed traders and trade behaviorally. Second, this study further explores the factors behind changes in GSVI by distinguishing between positive shocks and negative shocks caused by the increase in SVI use. This is to verify whether its changes, caused by various factors, have an asymmetric effect on excess returns and abnormal trading volumes.
Subsequent sections are arranged as follows. Section 2 summarizes domestic and foreign literature related to the use of GSVI to predict stock prices and proposes possible gaps. Section 3 explains the analysis used herein. Section 4 presents the empirical results. Section 5 contains the conclusion and recommendations for future studies.
2. Literature review
Ginsberg et al. (Citation2009) employ GSVI keywords to track the prevalence of influenza, and the results reveal that searches highly correlate with doctor visits. In addition, GSVI is able to accurately estimate flu incidence rates one week later, suggesting that Google search data are effective predictors. The results have inspired academia, and the number of studies applying SVIs has multiplied in various fields. For instance, Goel et al. (Citation2010) use the Yahoo SVI to predict movie box office receipts of weekend premier feature films, the initial sales volume of video games, and the Billboard Hot 100, demonstrating that SVI effectively predicts consumer behavior.
With respect to behavioral finance studies, Da et al. (Citation2011) first investigate the relationship between GSVI and Russell 2000 Index stock returns. GSVI captures changes in investor attention more effectively than the proxy variables used in the literature, and the response is more immediate. The results indicate that GSVI predicts positive changes in short-term returns and that the effect reverses within a year. Preis et al. (Citation2013) collect GSVI changes of 98 keywords (e.g., “debt,” “stocks,” and “unemployment”) and conclude that the subsequent drop of the Dow Jones Industrial Average Index can be predicted when the SVI of certain keywords increases. Among them, the trading strategy constructed with the keyword “debt” received 326% returns during 2004–2011, which outperformed the 16% return of the buy-and-hold strategy. Da et al. (Citation2015) utilize Google Trends to generate an SVI for 118 keywords (e.g., “recession,” “unemployment,” and “bankruptcy”) and select the 30 words with the largest negative t-statistic to construct the Financial and Economic Attitudes Revealed by Search (FEARS) Index as a new evaluation criterion for market sentiment. The index exhibits a significant negative effect for the returns of t day of the S&P500 index and various exchange-traded funds. However, the return rate on t + 1 day exhibits a significantly positive effect. More recently, Padungsaksawasdi and Treepongkaruna (Citation2021) use “Coronavirus” as the search keyword and confirm that the global stock markets slump down when investors pay more attention to COVID-19 pandemic.
Various empirical studies have also been conducted outside the U.S. market. Takeda and Wakao (Citation2014) take Japan’s Nikkei 225 Index as the research object to explore the relationship between GSVI, stock returns, and trading volume. They note that GSVI presents a strong positive effect on short-term future stock trading volume, but shows a weakly positive significant relationship with the return rate of small-capitalization stocks. They conclude that this phenomenon is caused by the search engine most commonly used by Japanese people being Yahoo rather than Google. Gao et al. (Citation2018) collect SVI from China’s local search engine (Qihoo 360) to study the effect of SVI on information dissemination and information asymmetry in the China stock market. The results indicate that an increase in SVI accelerates information dissemination and reduces information asymmetry. Sifat and Thaker (Citation2020) examine the relationships between GSVI and market indices in five ASEAN countries. They employ vector error correction model (VECM) and wavelet analysis and indicate that an increase of GSVI is induced by high market returns but GSVI is negatively associated to future market returns. The paper provides a contrary conclusion to most existing literature using data in advanced countries.
Based on individual stock data, Rizkiana et al. (Citation2019) construct a composite sentiment index using three internet-based source data, i.e. social media comments, GSVI, and news. They find that GSVI is positively related to the composite sentiment index and GSVI can lead composite sentiment index as well as news for most cases in Indonesia. However, the paper does not find a consistent conclusion that GSVI can predict stock returns. Using a large sample of retail investors’ trading accounts, Desagre and D’Hondt (Citation2021) present that retail trading activity is positively associated with GSVI no matter which sides (purchase or sale shares) retail investors trade. They also find bidirectional causality relationship between investor attention and trading activity.
For the Taiwan stock market, Huang et al. (Citation2016) use GSVI and the number of new reports as proxy variables for investor attention and explore the effect of the two on the weekly returns of stock prices of companies listed in TWSE. The paper suggests that the increase in investor attention lead to higher stock price returns overall, with mid-capitalization stocks presenting the strongest effect. They also observe that the effect of investor attention disappeared during the financial crisis. Li et al. (Citation2017) explore the effect of GSVI on stock returns and the trading volume of the Taiwan Mid-Cap 100 Index. They report that GSVI positively affects both excess return and abnormal volume of the constituents of Taiwan Mid-Cap 100 Index.
As mentioned above, GSVI effectively influences or predicts related variables in various fields, including financial markets. Nevertheless, in the literature on Taiwan’s financial market, no study has focused on whether GSVI predicts the returns and trading volume of OTC stocks listed in a retail investor dominant market. Moreover, both positive and negative shocks on stock prices may attract investor attention and prompt searches for company information. Therefore, this study explores GSVI’s reliability as a predictor of OTC stock movement and whether increases in GSVI are caused by positive or negative shocks. The study intends to predict the effect of positive and negative shocks on the stock returns and trading volume of the TPEx 50 Index by considering the driving factors of the attention change.
3. Method
3.1. Data
This paper selects the research period covering from 1 September 2016 to 31 August 2019. The research objects are the constituents of TPEx 50 Index. The TPEx 50 Index is chosen, because its constituents are ordered by market value and due to its fundamentals, public float, and liquidity, in which the companies selected are representative of the TPEx stock market. We note that this study chooses the constituents at the beginning of the research period with the data frequency being weekly, totaling 155 weeks. However, two companies are no longer listed in TPEx over the whole research period. One company was acquired by ASML in late-2016, and the other one is delisted by TPEx due to corporate governance problems. Hence, the final research sample contains 48 companies.
The GSVI used in this study is premised on the traditional Chinese names of the 48 sample companies as the search keywords, and the region is set as Taiwan. All categories are included, and the GSVI generated by Google web search is selected. Finally, the GSVI for 48 companies is obtained, and each company has 155 weeks of data, with a total of 7,440 company-week observations. The remaining stock trading information is obtained through the Taiwan Economic Journal. In addition, the regression model considers to control the five-factor variables of Fama and French (Citation2015). This part of the data is calculated by authors according to Fama and French’s (Citation2015) approach.
3.2. Empirical model and variable definition
The first purpose of this research is to discuss whether GSVI has a predictive effect on weekly excess returns under the control variables of each factor in the five-factor model. Accordingly, the regression model uses (weekly excess returns) as the dependent variable,
(natural logarithm of SVI in the previous week) as the independent variable, and the five factors of the Fama and French (Citation2015) model and the previous week’s excess return
as the control variables, as shown in EquationEquation (1)
(1)
(1) :
where is the return rate of company i in week t minus the risk-free interest rate (the one-year fixed deposit interest rate is converted into weekly interest rate);
is the market risk premium factor for week t;
and
are the size effect and value premium factors, respectively; and
and
are the profitability and investment factors for week t in the five-factor model, respectively. A panel data regression model is adopted to estimate the parameters and to discuss the coefficient of
. In addition, because GSVI is between 0 and 100, the natural logarithm must be applied, as the value will change to 1 if the initial value is 0, and the value remains 0 after the calculation.
Substantial stock price changes (i.e., a positive or negative shock) may attract retail investors’ attention. Retail investors heed certain companies and initiate a search action, which eventually increases the GSVI value. Specifically, GSVI only measures the number of searches, but factors that drive investor searches often arouse more concern in the financial market. After all, both positive and negative shocks may generate attention among retail investors and prompt them to search for company names, which will lead to an increase in GSVI.
This paper therefore further defines two dummy variables—positive shock (POSit) and negative shock (NEGit)—to capture whether the jump of GSVI is triggered by a positive or negative shock. POSi is defined as follows: to judge whether it is a positive shock, if the weekly return of company i in week t is greater than the average weekly return plus one standard deviation, then the dummy variable = 1; otherwise, the dummy = 0. To judge whether it has suffered a negative shock, NEGit is defined as follows: if the weekly return of company i in week t is less than the average weekly return minus one standard deviation, then the dummy variable = 1, otherwise it is 0. Subsequently, EquationEq. (1)(1)
(1) is added with the two interaction terms to examine whether investor attention as measured by SVI changes under conditions of positive or negative shocks can have an asymmetric predictive effect on stocks’ weekly excess returns. The regression model is shown in EquationEq. (2)
(2)
(2) :
In addition to exploring the effect of SVI on excess returns, this study analyzes the effect of changes in SVI on trading volume. A company’s stock trading volume is calculated to obtain abnormal trading volume (AVol). The calculated abnormal trading volume is used as the dependent variable, and GSVI of the previous week is used as the independent variable to observe whether retail investor attention leads to abnormal trading volume. Moreover, because stock returns have a significantly positive relationship with trading volume, the current return rate is included as the control variable, with the regression model shown in EquationEq. (3)(3)
(3) :
The calculation method of is as follows:
where is the abnormal trading volume of shares of company i in week t and refers to the rate of change in the weekly share trading volume of company i in week t compared with its volume average over the previous 12 weeks.
This study also extends the discussion of using GSVI to predict the abnormal trading volume of stocks by adding an interaction term between positive shock and GSVI and negative shock and GSVI. Two interaction terms are used to explore whether changes in GSVI under the positive and negative shock conditions lead to abnormal stock trading volume. This study aims to observe the effect of GSVI changes caused by positive and negative shocks on the abnormal trading volume of stocks. The regression equation is shown in EquationEquation (4)(4)
(4) :
These equations use a panel data regression model to estimate the parameters. Regarding the fixed effects model, random effects model, or least square method, the F-test, Breusch–Pagan LM test, and Hausman test are used for model testing to decide the most suitable model.
Finally, the media and analyst coverages of TPEx 50 index constituents are not as popular as stocks listed in TWSE (particularly those in the Taiwan 50 Index and the Taiwan Mid-Cap 100 Index). This paper considers that the familiarity of the constituents may affect the relationship between GSVI and excess returns (or abnormal trading volume). Next, we use the coefficient of variation of GSVI to divide 48 constituents into two groups. Although GSVI of all companies is between 0 and 100, the coefficient of variation of GSVI for companies that are usually noticed by investors is smaller than that of companies not noticed by investors, which means the standard deviation is small or the mean is large. For instance, in the research sample, the mean and standard deviation of the GSVI of LandMark Optoelectronics Corporation (symbol: 3081.TWO) are 53.6 and 17.0, respectively; and the mean and standard deviation of TTY Biopharm Company (4105.TWO) are 12.6 and 24.0, respectively, indicating that the degree of attention received by these two companies significantly differs. Therefore, this study further analyzes the effect of GSVI on the returns and trading volume of high-attention and low-attention stocks by using subsamples.
4. Empirical results
4.1. Descriptive statistics
provides descriptive statistics of the three main variables. During the study period, the average weekly excess return on the stocks of the sample companies is 0.177%, and the standard deviation is 5.411%. The maximum and minimum values indicate that excess returns rise by 47.483% in one week and fall by 28.445% in another. The GSVI of the samples (natural logarithm) has a mean of 2.877, a standard deviation of 1.306, and maximum and minimum values of 4.605 and 0, respectively. The average weekly stock abnormal trading volume is 0.099 with a standard deviation of 1.004. The maximum and minimum values present that the abnormal trading volume of the sample companies increases by 16 times in the highest week and decreases by nearly 100% in the lowest.
Table 1. Descriptive statistics
According to the correlation coefficient matrix (), the weekly excess return is significantly positively correlated with the GSVI, signifying that the weekly excess return is larger when GSVI is higher. This phenomenon is consistent with the literature. However, the weekly excess return is significantly negatively correlated with GSVI of the previous week, implying that a larger GSVI can lead to a lower excess return rate the following week. This phenomenon differs from the literature and is further explored through regression analysis. Regarding abnormal trading volume and GSVI in the same or the previous period, a significantly positive correlation is present, which can be explained as an increase in GSVI attracting investor attention, which in turn increases the company’s share trading volume. This finding corresponds to that of related studies.
Table 2. Correlation matrix
4.2. Effect of Google Search Volume Index on stock returns
This section discusses whether GSVI has a predictive effect on weekly excess returns. Therefore, EquationEquations (1)(1)
(1) and (Equation2
(2)
(2) ) are used to analyze whether the difference between the two considers the positive and negative shocks of stock prices. The regression results of EquationEq. (1)
(1)
(1) without considering the effects of positive and negative shocks are presented in . Six regression results are in the table, with each one using a different independent variable or control variable or using a subsample. The analyses are estimated using the pooled ordinary least squares (OLS) model, because the results of the F-test and Lagrange Multiplier (LM) test are insignificant. Thus, the fixed or random effects model is not used, and the Hausman test is no longer necessary. In addition, the six regression models do not have the problem of collinearity.
Table 3. Regression results for GSVI prediction of weekly excess returns
Models (1) to (3) in are analyzed using the total sample (48 companies in the TPEx 50 Index). For Model (1) with the five-factor model’s control variables, the result indicates that the current GSVI has a significantly positive effect on the weekly excess return of the current period. This result is consistent with the findings of related studies. Model (2) adds the excess return of the previous period as a control variable, and the result suggests that the excess return of the previous and current periods have a significantly negative relationship, which means that the stock returns in the research sample have a strong short-term reversal effect. Model (3) inputs the main independent variables into the regression model, and the result demonstrates that GSVI of the previous period has a negative effect on the weekly excess return of the current period. More precisely, a higher GSVI leads to a lower weekly excess return the following week.
We may restate this as follows. SVI increases when investors pay attention to the news and use Google to search for the company’s Chinese name in the previous week (indicating investors are beginning to focus on the company), and it reduces the weekly excess returns in the current week. Although the estimated coefficient is insignificant, the literature generally supports the positive relationship between investor attention and weekly excess return, which obviously contradicts the results of this study.
Two possible reasons for the above phenomenon are suggested. First, excess return in the research sample has a short-term reversal effect, and it has a positive relationship with GSVI over the same period. Therefore, the effect of GSVI from the previous period is negative (a significantly negative correlation can be observed from the correlation coefficient in ). However, after the short-term reversal effect has been controlled in Model (3), the effect of GSVI from the previous period becomes insignificant. Second, as in the data presented in Section 1, nearly three-quarters of Taiwan’s OTC investors are assumed to be so-called retail investors, which is much higher than in other stock markets. Coupled with the small market capitalization of the stocks on the TPEx market and the small trading volume, this adds to the problem of information asymmetry. Therefore, when retail investors with lagging information pay attention to OTC stocks, it is usually the time for stock returns to reverse.
Model (4) in explores the effect of GSVI on weekly excess returns when the samples are present in the TPEx 50 Index during the entire study period. This study asserts that some stocks will be removed, because the apparent decline in stock prices has caused the market capitalization to be insufficient for inclusion in the TPEx 50 Index list. This may affect the aforementioned findings. For this reason, the stocks listed in the TPEx 50 Index from the beginning of the research period until 31 August 2019 are used as the screening basis. In total, 33 companies are taken as subsamples, and a five-factor model is used for verification. The regression results indicate that GSVI of the previous period also has a negative effect on the excess return of the current period, but the effect remains insignificant.
Model (5) takes high attention (low coefficient of variation group in GSVI) stocks as the analysis object; such stocks are frequently noticed by investors. Model (6) uses low attention (high coefficient of variation group) stocks as the analysis object; such stocks are seldom noticed by investors, but may start to attract attention due to news dissemination. The results of Model (5) indicate that GSVI of the previous period has a negative effect on the excess return of the current period, but the coefficient is insignificant. However, the results of Model (6) suggest that GSVI of the previous period has a significantly negative effect on the excess return of the current period. Because this group is low-attention stocks, the effect is an indication of information asymmetry. More precisely, the stock reversal effect is activated when retail investors with lagging information begin to pay attention to stocks that they usually do not follow.
Because this study uses weekly data, analyzing only the effect of GSVI of the previous week may be insufficient. Therefore, this study also analyzes the effect of GSVI for the previous period to the first twelve periods (about three months) on the excess returns of the current period. The results appear in .
Table 4. The longer effect of GSVI on excess returns
Regarding the total sample regression results in , GSVI levels for the two periods of t-4 and t-6 (approximately 1 to 1.5 months prior) have a significant effect on the excess returns of the current period. In addition, the Wald test is further used to test whether the cumulative effect is not zero. Among them, only the cumulative effect of the first four periods is significantly negative, indicating that a higher GSVI in the past month can result in lower current excess returns. The samples of the low coefficient of the variation group are generally high-attention stocks. The coefficients of the two periods of t-4 and t-7 are significantly negative, and the results of the three Wald tests are significant, denoting that GSVI has a negative effect on excess returns for the ensuing 1 to 3 months. Although depicts that GSVI has no effect on the short-term (one-week) return in the high attention group, it does depict a significant effect when extended to one quarter.
With regard to the low attention group, the coefficients for the five periods of t-1, t-4, t-7, t-11, and t-12 are significant, and most have negative effects. In the Wald test, the cumulative effect of the first four periods is significantly negative. Although the cumulative effect of the first eight periods is insignificant, the cumulative effect of the first twelve periods is significantly negative, indicating the negative effect of GSVI lingers when the period is extended to one quarter. Accordingly, GSVI has a predictive effect on excess returns, and the effect lasts at least one month.
As mentioned above, this study differs from related studies, because it further explores whether the factors behind GSVI’s increase indicate a positive or negative shock on the stock prices, and whether the shock has an asymmetric effect on the weekly excess returns of stocks. Thus, the study uses EquationEq. (2)(2)
(2) for regression analysis of the direction and significance of the coefficients of the two interaction terms; the results are presented in . The total sample regression adopts a fixed effects model (F-test is significant and LM test is insignificant), and the regressions of the other two subsamples are estimated using the pooled OLS model.
Table 5. Distinguishing the effect of GSVI on excess returns under positive and negative shocks
In the estimation results for the total sample, the coefficient of GSVI of the previous period is significantly negative, indicating that under the general situation (without a positive or negative shock), the current excess return is lower when GSVI in the previous period is higher. The interaction between a positive shock and GSVI is significantly positively correlated, which means that when the stock price suffers a positive shock and rises by more than one standard deviation of the average return, the search volume of retail investors increases and offsets the initial unfavorable effect on stock prices (the overall effect of and
is not different from zero). However, the interaction between a negative shock and GSVI of the previous period is positive, but non-significant for the coefficient of weekly excess returns, and the sum of the coefficients of
and
is not different from zero. This implies that when a negative shock results in a sharp fall of more than one standard deviation of the average return, the decline causes the search volume of retail investors to increase, and the stock price does not continue to fall the following week. Because retail investors obtain information relatively slowly, they sell or short shares in panic after obtaining unfavorable news from newspapers or other media. Generally, this effect is accompanied by the situation that the stock price stops falling the following week.
contains the regression results for the high attention group. GSVI of the previous period and the two interaction terms are insignificant, indicating the stocks that normally attract high attention. Regardless of whether the stock price is affected, GSVI has no effect on excess returns in the next period. In addition, the results for the low attention group indicate that the effect of GSVI of the previous period is generally negative on excess returns. Moreover, the interaction between a positive shock and GSVI is significantly positively correlated, which implies that when the excess return is greater than one standard deviation, the overall effect of GSVI switches to become positive, but remains insignificant. Furthermore, the interaction between a negative shock and GSVI is negatively correlated. Thus, the overall effect of GSVI remains negative. Nonetheless, because the F-statistic is insignificant, the stock price does not have an apparent fall the following week. Note that the positive and negative shocks exhibit a significant asymmetric effect in the high coefficient of the variation group. Investor attention caused by positive shocks can increase returns, but investor attention caused by negative shocks has no effect on returns.
4.3. Effect of Google Search Volume Index on abnormal trading volume
When investors notice a company because of news dissemination, they use the Google search engine to search for more company information. This causes GSVI to increase, which represents investor attention, and raises the question of whether investor attention can lead to abnormal changes in stock trading volume. Subsequently, this subsection explores whether changes in GSVI have a predictive effect on abnormal stock trading volume. Both positive and negative events may attract investors’ attention, causing them to use the Google search engine to search for relevant information about events, which in turns boosts GSVI. Therefore, this study also analyzes whether GSVI under the positive or negative shock of stock prices has an asymmetric effect on abnormal stock trading volume. EquationEquations (3)(3)
(3) and (Equation4
(4)
(4) ) are used as regression models, and the parameter estimation results are listed in .
Table 6. Effect of GSVI on abnormal trading volume
According to Model (1) in , GSVI of the previous period has a significantly positive effect on abnormal stock trading volume, which can be explained by the current week’s trading volume indeed having more significant growth compared with the average trading volume of the previous 12 weeks when GSVI in the previous week is higher. Specifically, the increase in GSVI can predict abnormal trading volume. This may be due to the information initially obtained by investors coupled with the use of information obtained using the Google search engine as the basis for stock trading, resulting in an obvious increase in trading volume. This result is consistent with those reported in the literature, where an increase in investor attention drives the subsequent increase in stock trading volume (Barber & Odean, Citation2008).
Model (2) adds the interaction terms (positive and negative shocks) of GSVI. In the findings, the predictive effect of GSVI of the previous period on abnormal trading volume disappears, but is replaced with the interaction term of a positive shock and GSVI. The coefficient of this interaction term is significant and positive; when GSVI of the current week is soaring due to a surge in stock prices, the trading volume the following week grows more apparently than the average trading volume of the previous 12 weeks. This likely relates to the trading habits of general retail investors. When retail investors receive favorable news and generate searches, they can make buying decisions according to the search results, resulting in abnormal growth in stock trading volume. However, the coefficient of a negative shock and GSVI is negative and insignificant, which is explained as stock trading volume not growing significantly after investors are aware of the sharp drop in stock prices, because they are not accustomed to short selling. In addition, investors are unwilling to sell stocks that have suffered losses due to the disposition effect, which may be one reason for the trading volume not growing significantly.
further divides the samples into a low and high coefficient of variation groups. The regression results for the two groups are consistent—that is, regardless of whether stocks are high- or low-attention, the abnormal trading volume in the following week increases significantly as long as the stock price is subject to a positive shock and the occurrence of search behavior. By contrast, abnormal trading volume of the following week does not change significantly if the stock price is subjected to a negative shock. The Wald test in also indicates that positive and negative shocks have an asymmetric effect on abnormal trading volume. As mentioned, this asymmetric effect should come from investors’ different behaviors after perceiving that stock prices rise and fall.
4.4. Robustness analysis
This study aims to explore the asymmetric effect of Google search behavior caused by shocks in stock prices on excess returns and abnormal trading volume. No method is consistently adopted to measure positive and negative shocks. This study defines the stock price subject to positive and negative shocks on the basis of the average return plus or minus one standard deviation as noted above. To improve the robustness of the research results, this subsection adopts two other measurement methods—namely, (1) the average return plus or minus 0.5 times the standard deviation, and (2) the average return plus or minus two standard deviations—to verify the robustness of the results.
The regression estimation results for excess returns are in . Measuring the total sample, when the shock threshold is set to 0.5 times the standard deviation, only GSVI of the previous period exhibits a significantly negative effect on excess returns, whereas the other two interaction terms have no effect. When the shock threshold is set to two standard deviations, the positive shock and GSVI are significantly positively correlated, and the overall effect ( +
) is significantly greater than zero, indicating that GSVI is high when the positive shock is large, which results in higher excess returns the following week. This outcome is consistent with findings in the literature.
Table 7. Robustness analysis of shock thresholds and excess returns
With respect to the regression results for the high-attention group, when the threshold is set to two standard deviations, the negative shock significantly positively correlates with GSVI, and the overall effect (+
) is also positive but insignificant. This indicates that the stock price exhibits a reversal effect after the sharp drop during the previous period has attracted the attention of investors. Finally, in the regression results of low-attention group, when the threshold value is set to two standard deviations, the positive shock significantly positively correlates with GSVI, and the overall effect is greater than zero; the asymmetric effect is also significant. This denotes that low-attention stocks are more susceptible to the effect of GSVI. According to the results in , because OTC stocks are less noticed by investors than stocks listed in TWSE, a larger stock price shock is required to attract their attention.
In , different thresholds are used to define positive and negative shocks, and the table presents the results of regression analysis of abnormal trading volume. In short, the regression results for the total sample and high- and low-attention groups are rather consistent—that is, abnormal trading volume increases significantly the week following a stock price rise due to a positive shock. By contrast, abnormal trading volume in the week following a negative shock that causes the stock price to fall sharply drops or remains unchanged. These results are consistent with the conclusions in . In addition, when the threshold value is higher, the abnormal trading volume increases more obviously (the coefficient becomes larger), which can also be explained as a higher positive shock on stock prices attracting more investor attention and increasing trading volume.
Table 8. Robustness analysis of shock thresholds and abnormal volumes
The thresholds of positive and negative shocks are adjusted in this section, but the robustness analysis results of are greatly consistent with , suggesting that the research results have a certain degree of robustness. Two points are worth noting. First, when the threshold is more extreme, the effect of GSVI is more distinct. Second, if the stock is originally a low-attention stock, then its share price and trading volume are more obviously affected by changes in GSVI.
Our results also provide some practical implications for investors. First, present a significant reversal effect on Taiwan’s OTC stocks, implying that the price momentum strategy may be unprofitable in short-term. Second, show that when the current excess return is larger than a certain threshold, the overall effect of GSVI on future excess return is positive, especially for low attention group. Therefore, this finding implies a potential trading strategy based on the interaction of positive shock and GSVI. For example, according to the last column of , the predicted future excess return is 0.647% when we hold all independent variables at sample means except for excess return and then let excess return is equal to sample mean plus two standard deviations (positive shock on price). The fact that the securities transaction tax is 0.3% and the common commission fee is 0.0855% for buying or selling a share, indicating that the total transaction cost for one trade is 0.471%.Footnote3 Therefore, we suggest that if investor attention is triggered by a large positive shock, investors can buy this stock to gain a weekly return of 0.176%.Footnote4
5. Conclusion Remarks
GSVI is widely adopted as a proxy variable that measures investor attention. Empirical evidence also supports the positive relationships between GSVI and future stock returns and trading volume. Unlike related studies, this study focuses on stocks in the OTC market, i.e., the TPEx 50 index constituents, under the assumption that TPEx and TWSE markets have obvious differences in market structure and the structure of listed companies in Taiwan. Hence, this study aims to address a lack of discussion in the literature by analyzing how GSVI affects the excess returns and abnormal trading volume of stocks in TPEx 50 Index. In addition to adopting different research objects, the study distinguishes whether changes in GSVI are caused by positive or negative shocks to stock prices to explore whether the increase in search volume caused by different sources of information has an asymmetric effect on subsequent excess returns and abnormal trading volume.
In the empirical results, GSVI of the previous period has a negative effect on the weekly excess returns of the current week when the five-factor model is used for the control variables. In particular, the effect on stocks with low attention is more obvious, and this negative effect may last for more than one month. One inference is that when retail investors with lagging information begin to pay attention to a stock, it has already entered a reversal period. After distinguishing the factors behind the SVI increase, the positive shock and GSVI of the previous period have a significantly positive effect on excess returns. Particularly when the positive shock reaches the average excess return plus twice the standard deviation, the overall effect of GSVI is significantly positive, indicating that a stock price rise is accompanied by the SVI increasing and that the upward trend may continue.
Regarding the prediction of abnormal stock trading volume by GSVI, GSVI of the previous period has a significantly positive effect on abnormal stock trading volume. This finding is similar to the conclusions of related studies. After identifying the reasons behind the increase in SVI, the results reveal that the stock price surge in the current week is accompanied by an increase in search volume, and the subsequent stock trading volume has more obvious growth compared with the average trading volume in the previous 12 weeks. Nevertheless, after a sharp drop in stock price and the increased search volume the previous week, the subsequent share trading volume does not have obvious changes. This may be related to the habits of general retail investors. When these investors receive favorable news and generate searches, they can make buying decisions according to the search results, resulting in abnormal growth in share trading volume. By contrast, when they receive unfavorable news and are not accustomed to shorting, no apparent growth may occur in stock trading volume.
Different from relevant studies, this study explores whether a greater GSVI results from a positive or negative shock on stock prices, with the hope that the empirical results can interpret the relationship between the initial factors of investor use of Internet searches and the excess returns and trading volume of stocks. For general retail investors, this study suggests paying attention to certain OTC stocks for at least one month to detect the short-term reversal effect, instead of blindly trading. However, investors can consider buying the stock if an abnormal rise in stock price is accompanied by increased investor attention, particularly for OTC stocks with low attention. The study encounters limitations, however. For example, positive and negative shocks are defined only by abnormal changes in stock prices. Future studies are recommended to use text mining to analyze the information source causing abnormal stock prices in order to clarify the relationship between GSVI increases and financial news.
Acknowledgements
Financial supports from the Ministry of Science and Technology [grant number 108-2410-H-224 −010; 109-2410-H-224 −015] are gratefully acknowledged.
Disclosure statement
No potential conflict of interest was reported by the author(s).
Additional information
Funding
Notes on contributors
Huei-Hwa Lai
Huei-Hwa Lai is an assistant professor at the Department of Business Administration, Chaoyang University of Technology, Taiwan. She obtained her PhD in Finance from National Yunlin University of Science and Technology, Taiwan. Her research interests cover corporate finance, corporate government, and stock market.
Tzu-Pu Chang is an associate professor at the Department of Finance, National Yunlin University of Science and Technology, Taiwan. He obtained his PhD in Management from National Chiao-Tung University, Taiwan. His research interests include investment and data analysis. Hence, he recently studies machine learning in the finance field. The other two authors, Cheng-Han Hu and Po-Ching Chou, are respectively master and PhD students supervised by Tzu-Pu Chang. They assist this research in data collection and data processing.
Notes
1. The Google Trends tool was launched in 2006 to provide GSVI, which contains statistical data for keywords. Users can observe the search popularity trend of a keyword in a country in any period. The data are gathered from every user who uses the Google search engine, and any use of the engine leaves a trace in Google Trends.
2. Ben-Rephael et al. (Citation2017) use Bloomberg news search as the proxy variable of institutional investor attention, explore the changes in stock returns when institutional investors identified a certain company’s stock, and compare it with GSVI.
3. In Taiwan, the upper limit of commission fee is 0.1425% for buying or selling a share. However, most brokerage firms give a discount of 40 percent in order to shoot for a higher market share. Even some brokerage firms can provide a discount of 70 percent to grab more customers.
4. We suggest that future studies can examine the profitability of this strategy by using more rigorous methodologies.
References
- Barber, B. M., & Odean, T. (2008). All that glitters: The effect of attention and news on the buying behavior of individual and institutional investors. Review of Financial Studies, 21(2), 785–18. https://doi.org/10.1093/rfs/hhm079
- Ben-Rephael, A., Da, Z., & Israelsen, R. D. (2017). It depends on where you search: Institutional investor attention and underreaction to news. Review of Financial Studies, 30(9), 3009–3047. https://doi.org/10.1093/rfs/hhx031
- Chang, T. P. (2021). Buy low and sell high: The 52‐week price range and predictability of returns. International Review of Finance, 21(1), 336–344. https://doi.org/10.1111/irfi.12263
- Chen, Z., Schmidt, A., & Wang, J. (2021). Retail investor risk-seeking, attention, and the January effect. Journal of Behavioral and Experimental Finance, 30, 100511. https://doi.org/10.1016/j.jbef.2021.100511
- Da, Z., Engelberg, J., & Gao, P. (2011). In search of attention. Journal of Finance, 66(5), 1461–1499. https://doi.org/10.1111/j.1540-6261.2011.01679.x
- Da, Z., Engelberg, J., & Gao, P. (2015). The sum of all FEARS investor sentiment and asset prices. Review of Financial Studies, 28(1), 1–32. https://doi.org/10.1093/rfs/hhu072
- Desagre, C., & D’Hondt, C. (2021). Googlization and retail trading activity. Journal of Behavioral and Experimental Finance, 29, 100453. https://doi.org/10.1016/j.jbef.2020.100453
- Fama, E. F., & French, K. R. (2015). A five-factor asset pricing model. Journal of Financial Economics, 116(1), 1–22. https://doi.org/10.1016/j.jfineco.2014.10.010
- Gao, Y., Wang, Y., Wang, C., & Liu, C. (2018). Internet attention and information asymmetry: Evidence from Qihoo 360 search data on the Chinese stock market. Physica A: Statistical Mechanics and Its Applications, 510, 802–811. https://doi.org/10.1016/j.physa.2018.07.016
- Ginsberg, J., Mohebbi, M. H., Patel, R. S., Brammer, L., Smolinski, M. S., & Brilliant, L. (2009). Detecting influenza epidemics using search engine. Nature, 457(7232), 1012–1014. https://doi.org/10.1038/nature07634
- Goel, S., Hofman, J. M., Lahaie, S., Pennock, D. M., & Watts, D. J. (2010). Predicting consumer behavior with web search. PNAS, 107(41), 17486–17490. https://doi.org/10.1073/pnas.1005962107
- Huang, T. L., Chen, M. L., Kuo, H. J., & Lai, K. L. (2016). How do web search activity and financial media coverage affect asset pricing? Journal of Financial Studies, 24(1), 25–53. https://doi.org/10.6545/JFS.2016.24(1).2
- Li, Y. L., Tu, Y. C., & Wang, W. H. (2017). Google search volume index and its effects on stock return and trading volume of Taiwan stock market. Journal of Management and Systems, 24(4), 565–590. https://doi.org/10.29416/JMS.201710_24(4).0004
- Padungsaksawasdi, C., & Treepongkaruna, S. (2021). Chasing for information during the COVID-19 panic: The role of google search on global stock market. Cogent Economics & Finance, 9(1), 1930669. https://doi.org/10.1080/23322039.2021.1930669
- Preis, T., Moat, H. S., & Stanley, H. E. (2013). Quantifying trading behavior in financial markets using google trends. Scientific Reports, 3(1), 1684. https://doi.org/10.1038/srep01684
- Rizkiana, A., Sari, H., Hardjomidjojo, P., & Prihartono, B. (2019). The development of composite sentiment index in Indonesia based on the internet-available data. Cogent Economics & Finance, 7(1), 1669399. https://doi.org/10.1080/23322039.2019.1669399
- Sifat, I. M., & Thaker, H. M. T. (2020). Predictive power of web search behavior in five ASEAN stock markets. Research in International Business and Finance, 52, 101191. https://doi.org/10.1016/j.ribaf.2020.101191
- Takeda, F., & Wakao, T. (2014). Google search intensity and its relationship with returns and trading volume of Japanese stocks. Pacific-Basin Finance Journal, 27, 1–18. https://doi.org/10.1016/j.pacfin.2014.01.003