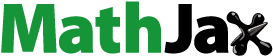
Abstract
The main objective of the study was to investigate the impact of microfinance on smallholder resettled sugarcane farmers’ productivity and technical efficiency. The study evaluated the impact of microfinance on technical efficiency of resettled sugarcane smallholders as well as the determinants of their technical efficiency. The study used Transcendental Logarithmic (Translog) Stochastic Frontier Analysis. Data from a household level survey of 2018 was collected using questionnaires in a multi-stage sampling technique. The hypothesis tests confirmed the adequacy of Translog SFA frontier over Cobb–Douglas together with the appropriateness of SFA over OLS. The results revealed that both microfinance and intensity of participation significantly improve technical efficiency. Extension services, secondary education, tertiary education, experience, and farming assets were among statistically significant determinants of observed variation in technical efficiency. Estimated technical efficiency scores from the truncated normal distribution model with heteroscedasticity and exogenous determinants were on average 64.4% and 33.6% for treatment and control groups, respectively. Bank participants were more efficient (65.4%) than MFIs participants (63.3%). The results confirmed that microfinance promote efficient utilization of agricultural inputs. Policy suggestions include expansion and sufficient disbursement of microfinance.
PUBLIC INTEREST STATEMENT
This study concerns the impact of microfinance (microcredit) on technical efficiency of A2 smallholder resettled sugarcane farmers. Technical efficiency is critical aspect in agricultural total factor productivity and output growth in agro-based economies. Smallholder farmers gained significance after land reform, but their productivity significantly declined likely due to existing technical inefficiencies. This study contributes to the current debate through pointing smallholder farmers’ productivity strategies, highlighting key policy interventions (complementary agricultural finance mechanisms) since smallholders hardly access formal credit due to lack collateral (assets and title deeds). Homogeneity of challenges faced by smallholder farmers makes the findings applicable to various agribusiness specialisations. Sugarcane output responds positively to the traditional production factors. Efficiency therefore could be improved through government and private sector interventions (in promoting access to production factors including agricultural finance and ensuring better and more reliable agricultural extension services which will to restore Zimbabwe’ breadbasket of Africa status).
1. Introduction
Increasing agricultural production and productivity in Zimbabwe requires timely and adequate supply of agricultural inputs including agricultural finance. Smallholder farmers need financial support to meet the expenses on various agricultural activities (Wadud, Citation2013). A large number of smallholder farmers in Zimbabwe are dependent on microfinance of different forms. As marginal and smallholder farmers have little to no access to mainstream financing mechanisms, microfinance provides smallholder resettled farmer with timeous access to factors of production and technology adoption. Access to and participation in microfinance is crucial for smallholder agricultural productivity growth. Appropriate amounts and quality of agricultural microfinance (microcredit) are crucial for realising the full potential of agriculture as a profitable economic activity (Wadud, Citation2013).
Agricultural production is strongly conditioned by the fact that inputs are transformed into outputs with considerable time lags (Conning & Udry, Citation2005), causing rural household to struggle in balancing their budgets during the off-season. With limited access to finance, balancing the budget within a season becomes a binding constraint to agricultural productivity growth. Binding liquidity constraints result in suboptimal input combinations by farmers, thereby restraining optimum production choices. With the majority of smallholder farmers lacking direct access to formal financial system in Zimbabwe, microfinance (microcredit) becomes their next best alternative.
With strategies to revitalize and restore the agriculture contribution to GDP in Zimbabwe involving Operation Maguta,Footnote1 presidential input schemes (mainly seed and fertilizer), command agriculture,Footnote2 and Pfumvudza.Footnote3 Much effort is directed towards food security directly linked crops such as maize and other small grains. Sugarcane is generally considered to have an indirect link to food security though it has and is receiving little to no government attention, though critical since many smallholder resettled sugarcane farmers solely depend on it as a source of livelihoods. The Zimbabwe vision 2030 (attaining middle income country status) can only come to be if all corners of the agricultural sector receive significant attention they deserve. The full potential of sugarcane on employment creation, export promotion, import substitution, and food and energy security will therefore be fully realised if sufficient funding is directed towards the smallholder resettled farmers.
The terms “Microfinance” and “microcredit” are often used interchangeably though they are not precisely the same. Microfinance (which entails financial inclusion—micro-savings, micro-insurance, financial literacy, and management training and money transfer services) is wider than microcredit (though microcredit is the critical pillar of microfinance). The above misconception of microfinance was also narrowed to microcredit by Christen et al. (Citation2003) and Microfinance Gateway (Citation2008). For the sake of this study, microfinance was proxied to microcredit.
Productivity is the growth in output not accounted for by changes in factor inputs, measured as the ratio between output(s) and input(s), while farm productivity is the ratio of output (s) to input (s) (Coelli, Rao, O’Donnell, and Battese, Citation2005). This study is mainly centred on Technical Efficiency (TE). Technical efficiency (TEFootnote4) is the ability of a decision-making unity (DMUFootnote5) to attain the best production from a given set of inputs (output-increasing). It may also be defined as the measure of the ability to use the minimum feasible amount of inputs to produce a certain level of output (input-saving). Koopmans (Citation1951) and Cooper et al. (2007) posited that a DMU is fully technical efficient if, and only if, it is not possible to increase inputs or output without making some inputs or output worse off. Debreu (Citation1951) defined TE as one minus maximum equiproportionate reduction (expansion) in all inputs (output) that still allows the production process to continue. Efficiency measurement relies on the specification of a production frontier, which represents the maximum potential output produced from a given input vector (S. C. Kumbhakar & Lovell, Citation2000).
Smallholder resettled A2Footnote6 sugarcane farmers contribute immensely towards the national productivity through utilising previously underutilised and unutilised land. Growth in sugarcane production increases the chances of producing biofuels. This therefore contributes significantly towards sustainable development and poverty reduction through various environmental and economic advantages over fossil fuels. The benefits of biofuels to the Zimbabwean economy may include but not limited to enhanced energy security, improved trade balance by reducing oil imports (import substitution), creation of new export opportunities (export promotion), and the potential to help tackling climate change through reduced emissions of greenhouse gases and other air contaminants. Growth in sugarcane production therefore enhance energy security. Also, strategically supporting sugarcane production can significantly contribute towards reducing unemployment in Zimbabwe because its production is labour intensive.
Land redistribution can be an effective tool in fighting poverty and promoting agricultural productivity growth and ensuring food security (World Bank, Citation2006). However, empirical evidence linking land redistribution, microfinance (microcredit), agricultural productivity (technical efficiency) in Zimbabwe is still scanty. Also, studies which compare technical efficiency impacts of microfinance from different sources (MFIs versus Bank microfinance) are still limited. In addition, previous studies did not consider the possible influence of random shocks like measurement errors and other noises in the data Battese and Coelli Citation1995) due to the use of nonparametric methods-DEAFootnote7 as done in Tahir and Tahrim (Citation2015). Non-parametric approaches result in overstatement of TE. For those who used parametric approaches, there is a strong bias towards the less flexible Cobb–Douglas stochastic frontier which is also flawed by considering factors of production as substitutes rather than complements. This study therefore implored the more flexible Translog Stochastic Frontier (with increased number of estimated parameters (cross-elasticities)).
The aims of the study are to examine the impact of microfinance on TE together with its determinants on smallholder resettled farmers in Zimbabwe. In achieving set objectives, answers to the following pertinent questions will be provided: Could it be that smallholder resettled sugarcane farmers who participate in microfinance are more efficient than those who do not participate?, Could it be that farmers who participate in microfinance through MFIs are more efficient than those who participate through the mainstream financial institutions? What are the determinants of TE for smallholder resettled sugarcane farmers? From a policy perspective, answers to these questions are very important given the decreasing agricultural production in Zimbabwe and the results are applicable to other smallholder cash crop production (tobacco, soya beans, and cotton). The answers will help in the development of comprehensive and complementary microfinance and land resettlement policies despite smallholder farmers’ varying socioeconomic and agro-ecological settings. All stakeholder smallholder farmer intervention strategies and schemes will be aided by the findings through the development of productive lending schemes. The rest of the study is organized into the following sections, literature review (section 2), sampling, definition and measurement of technical efficiency, research methodology (section 3), presentation of results, interpretation and discussion (section 4), then conclusions and policy recommendations (section 5).
2. Literature review
Several studies have been carried out to investigate the link between agricultural credit and productivity in both developing and developed countries. These studies have used different approaches in the domain of cross sectional and panel data and also of nonparametric and parametric approaches. Of the studies that have used parametric approaches, very few have applied the flexible Translog Stochastic Frontier model-TLSFM.Footnote8 Of the very few that have used the Translog model, on distributional assumptions, very few have applied the Truncated normal distribution Technical Inefficiency model that caters for Heteroskedasticity and exogenous determinants. What follows is a snapshot of some of the studies that have been carried out within the domain of this study.
Wadud (Citation2013) examined the impact of microcredit on farm performance, output and food security using farm level survey data of northern Bangladesh on 682 (450 treatment and 232 control) farms. Cobb–Douglas stochastic frontier model and data envelopment analysis along with inefficiency effects model were used. Inefficiency effects model revealed that microcredit, experience, and education help farmers to efficiently utilise inputs. Level of technical efficiency of participants was on an average, higher than the control group. Suggested policies include the expansion, timely, and fair distribution of microcredit to small farmers.
Ambali (Citation2013) examined the effect of microcredit on technical efficiency of rural farm households in Egba in Nigeria using a multistage sampling procedure to select 160 farmers. The stochastic frontier production function was used. Results revealed that farm output increases with farm size and labour. The inefficiency model revealed age, farming experience, education, household size, and credit to decrease technical inefficiency. The mean technical efficiency of 69% implied that there still exist room for improvement in technical efficiency. Policy suggestions included the reinforcement of farmers’ education and increased awareness of microcredit benefits on farmers’ productivity.
Anang et al. (Citation2016) examined the impact of agricultural microcredit on technical efficiency of smallholder rice farmers in Northern Ghana using farm household survey data. A stochastic frontier production function was used. Micro-credit-participating households had TE of 63% compared to 61.7% percent for non-participants. Conventional farm inputs revealed significant effects on rice production except labour and capital. The significant determinants of inefficiency included microcredit, age, sex, educational status, distance to the nearest market, herd ownership, access to irrigation, and specialisation in rice production. Recommendations were that microcredit should be availed to farmers.
Dessale (Citation2019) analysed the level of TE of smallholder wheat producers and identified the determinants of TE in Ethiopia. Cobb–Douglas Stochastic Frontier Model was used. Wheat output was positively and significantly influenced by area, fertilizer, labour and number of oxen. Estimated average TE was 82%. The estimated inefficiency parameters showed that age, education, improved seed, training, and credit were negative and significant determinants of technical inefficiency.
Bangwayo-Skeete et al. (Citation2010) examined whether Zimbabwe’s Fast Track Land Reform Programme (FTLRP) farms are more technically efficient than the traditional communal farms. The study used data from FTLRPFootnote9 beneficiaries and a control group of unsuccessful communal applicants. To account for possible systematic selection into FTLRP, they employed a probit selection equation and estimated a corrected Cobb–Douglas stochastic frontier function model. Inefficiency model estimates revealed that FTLRP beneficiaries were more technically efficient than the traditional communal farmers. The average efficiency for the FTLRP beneficiaries was 24% higher than that of communal farmers group.
Dube and Guveya (Citation2014) investigated the technical efficiency of smallholder out-grower tea farmers of Chipinge in Zimbabwe. DEA was used on 50 smallholder outgrower farmers. The estimates of technical efficiency ranged from 37% to 100%, while the mean technical efficiency was 79% suggesting 21 % tea output loss due to inefficiency. Experience, area cultivated, fertiliser, extension services, and extent of farm commercialization significantly affected TE.
There have been generalisations of the assessments of the smallholder farmers’ technical efficiency in considering agricultural production of many crops limiting relevance and applicability of the studies given the heterogeneous demands of various crops (maize, cotton, tobacco, and cotton) and nothing yet in line with smallholder A2 resettled sugarcane farmers. It has therefore become imperative to fill the absurd gap on the both scarcity of the literature on the impact of microfinance on smallholder (A2/commercial) resettled farmers and the methodological shortcomings of nonparametric and less flexible parametric approaches. The study also wish to provide pointers on the critical routes to take when crafting microfinance, agriculture finance, and land resettlement policies in Zimbabwe.
2.1. Population, sampling frame, sampling procedure, and data collection
The target population of the study were the smallholder A2 resettled sugarcane farmers. Only farmers who specialise in sugarcane farming were selected. Again, only FTLRP beneficiaries in the sugarcane growing areas of Chiredzi resettlement schemes (Hippo Valley, Mkwasine and Triangle) constituted the target population. Chiredzi in Masvingo province of Zimbabwe was the study area. It is one of the few areas where farmers gained medium sized farms for smallholder farming purposes. Also, Chiredzi surrounding areas constitute the total commercial sugarcane production in Zimbabwe hence it was selected under this study. Farmers resettled under the A2 smallholder commercial/outgrower schemes who specialises in sugarcane growing were selected since they share common socio-economic characteristics. Over and above being FTLRP beneficiaries, bank (AgribankFootnote10) and MFI (Getbucks) beneficiaries of microfinance (microcredit) were considered together with non-beneficiary smallholder A2 resettled sugarcane farmers.
A multi-stage sampling technique was employed. First stage involved the stratification of the Chiredzi resettlement area into the three resettlement schemes namely Mkwasine, Hippo valley, and Triangle. Second stage followed the purposive selection of microfinance participants (micro-borrowers) with the assistance of the baseline survey data from the microfinance service providers. Third, farmers in each stratum were randomly selected and interviewed using a structured and researcher administered questionnaire. Both microfinance beneficiaries from MFI (Getbucks) and bank (Agribank) together with non-beneficiaries of microfinance in the selected areas were interviewed. Non-participants included both unsuccessful applicants and those who never applied. Farmers of the three resettlement areas constituted a sample of 370 smallholder resettled farmers.
Stratified random sampling procedure was selected for its ability to provide a better representation of the target population by ensuring that every subgroup within the total sample is properly represented, thereby providing better coverage of the population since the researcher have control over the sub-categories. Keeping in mind the objectives and methodology of the study, a detailed questionnaire was prepared for data collection. A pre-tested, structured, and comprehensive questionnaire was designed with the aim of gathering relevant, reliable, and valid data. The questionnaire included questions about household characteristics microfinance (microcredit) participation, input (land, labour, fertiliser, seed and irrigation), and output information of farm activities and their prices.
2.2. Efficiency measurement—parametric versus nonparametric approaches
In the literature, there are two widely used methods of measuring technical efficiency: the nonparametric Data Envelopment Analysis (DEA) and the parametric Stochastic Frontier Analysis (SFA). The choice between parametric and nonparametric depends on production frontier specification and treatment of measurement errors. Non-parametric method has no parameters to be estimated and therefore does not account for measurement or sampling errors (Kathuria et al., Citation2013). Non-parametric techniques make no attempt to distinguish stochastic noise from technical inefficiency and notably the Stochastic Frontier Analysis (SFA) takes care of that. In other words, all deviations from the frontier are considered inefficiency in DEA, whereas this is decomposed into inefficiency and random errors in SFA (Battese & Coelli, Citation1995; Dorfman & Koop, Citation2005; Färe et al., Citation1990). Through econometric estimation, a statistical error term is added to account for deviations from the frontier independent of inefficiency (S. Kumbhakar & Wang, Citation2015).
The assumption of considering all deviations from the frontier as inefficiency becomes very difficult gives the inherent variability of agriculture production in developing countries due to exogenous shocks (Battese & Coelli, Citation1995), which include factors like weather shocks triggered by climate change in Zimbabwe such as droughts, cyclones (cyclone Elini and cyclone Gloria that reached Zimbabwe in the year 2000 and cyclone Idai of 2019), floods, pests, and diseases (army worms of 2016/2017). Since the data used in this study is obtained from responses of farmers who base on mental accounting (inability to do proper accounting (low levels of education and lack of accounting knowledge)), SFA (accounts for data noises) is reasonably preferred to DEA because it measure efficiency in the presence of statistical noise.
2.3. Measurement of technical efficiency and the theoretical model
Technical efficiency measurement can be either output or input oriented. Technical efficiency that relate to the ability to minimize inputs given output (Input-Oriented) and that which relates to the ability to maximize output from given input vector (Output-Oriented; S. C. Kumbhakar & Lovell, Citation2000). In the output-oriented approach, interest is by how much output could be expanded from a given vector of inputs, which is output-shortfall. In the case of single output-multiple inputs, the efficiency index is that of the output divided by summed weighted inputs according to Färe et al. (Citation1994). The best practice or frontier function shows the ability of a DMU to produce maximum attainable output from a given mix of inputs (Islam 2011). Debreu (Citation1951) and Farrell (Citation1957) described a feasible output-input vector technically efficient if, and only if, no increase in output or decrease in input is feasible. It follows then that technical inefficiency occurs if a producer is producing below the frontier.
Building from early theoretical work by Debreu (Citation1951), Farrell (Citation1957), Aigner et al. (Citation1977), Meeusen and van Den Broeck (Citation1977), and Battese and Corra (Citation1977), in triple simultaneous and independent papers, introduced models that allowed technical inefficiency alongside a stochastic or random shock. Introducing the random shock as an error term in (5.1) produced a stochastic production frontier expressed as:
where is a two-sided error term which captures individual specific noise. Therefore, TE in a stochastic frontier specification for a cross sectional data set becomes:
For this study, we have a cross sectional data set hence the need to specify technical efficiency in a cross sectional stochastic frontier model form that is:
where represents time period.
2.4. Functional forms of the stochastic frontier production models
In this section, the discussion is based on two commonly used frontier functions which are the Cobb–Douglas Production Function enroute to the Translog Stochastic Frontier Production Function.
2.4.1. The Cobb–Douglas stochastic production frontier model-functional form
According to Greene (Citation2010), the key assumptions underlying a Cobb–Douglas production function are that of positive and diminishing marginal products to inputs, constant returns to scale, and competitive product and inputs markets. The perfectly competitive factor and output markets assumption is self-defeating (factor and output markets for sugarcane are not perfectly competitive) due to the overregulated agriculture sector markets like sugarcane production in Zimbabwe.
The stochastic frontier production function is founded on the neoclassical production function:
Changes in K (capital) and L (labour) lead to changes in output (Y). However, output may change without changes in inputs and this constitutes technical progress (Grosskopf, Citation1993) as:
where represents all the exogenous variables that account for change in output with capital and labour being constant; therefore,
is the level of technical efficiency/inefficiency, which (allows) prohibits an entity from producing its maximum feasible output. EquationEquation (5)
(5)
(5) can then be is expressed as
Aigner et al. (Citation1977), Meeusen and van Den Broeck (Citation1977), and Battese and Corra (Citation1977) added , to Equationequation (6)
(6)
(6) to develop the stochastic frontier production function, which captures the typical measurement errors and statistical noise in regression translating into:
where is a two-sided independently and identically distributed (iid) error term with mean zero and variance,
, thus
.
Statistical operationalization of the TE term gives:
where measures the output distance to the frontier and follows a non-negative truncated normal distribution with mean zero and variance
, that is
.
Substituting Equationequation (8)(8)
(8) into Equationequation (7)
(7)
(7) and expressing it in a multiplicative Cobb–Douglas production function yields:
where and
are interpreted as production elasticities. Taking logs on Equationequation (9)
(9)
(9) and expressing in per capita terms (Y/L) yields the following:
Expressing the production function in per capita terms transforms it from an output function into a productivity function S. Kumbhakar and Wang (Citation2015). The dependent variable is now output per worker, or labour productivity. In general, denoting as output per unit of production and
as a vector of inputs and
as parameters to be estimated:
With the guidance of the Neo-Classical Cobb–Douglas production function (CDPF), this study included Land (Ln), Labour (Lr), Seed (SEE), Fertilizer and Chemicals (FC) and Irrigation. If presented in a cross-sectional data model, it becomes:
The shortcomings of the Cobb–Douglas production function motivated the development of more flexible functions such as the Transcendental-Logarithmic (Translog) production function discussed below. The study applied the more flexible and parametric Stochastic Frontier Analysis (SFA) (Transcendental-Logarithmic (Translog) Production Function).
2.4.2. Transcendental-logarithmic (translog) production function (TLPF)
Christensen et al. (Citation1971) improved the CDPF into the TLPF that address weaknesses of the Cobb–Douglas Production Function mainly its rigidity, strong separability (additivity) and homogeneity of Cobb–Douglas and Constant Elasticity of Substitution (CES) production functions into a relatively flexible TLPF. TLPF model does not assume perfect substitutability among inputs and perfect competition in factor markets. Flexibility in this case increases with the number of estimated parameters.
2.5. Model specification
2.5.1. Translog SFM specification
This study adopted and modified the specifications by Hossain and Rahman (Citation2012) and S. Kumbhakar and Wang (Citation2015). The likelihood ratio test confirmed the adequacy of the TLPF. The estimated model was derived from Equationequation (12)(12)
(12) and specified as below:
where —land,
—seed,
- labour,
—fertilizer and chemicals,
)—irrigation water, while
are the parameters estimated including the cross elasticities of the combined inputs.
2.5.2. Determinants of technical inefficiency
Since the study used the one stage MLE estimation of the stochastic frontier model, technical inefficiency scores will be calculated for each farmer and regressed against the technical inefficiency variables specified as below:
3. The dependant variable
is the respective technical inefficiency scores estimated in the first stage of the TLPF and regressed against independent variables indicated in Equationequation (14)
(14)
(14) .
4. Results presentation
4.1. Technical efficiency distribution
The TE distribution has been estimated using the flexible Wang (Citation2002) model modified from, KGMHLBCFootnote11 (Kumbhakar, Ghosh, and McGuckin (Citation1991); Reifschneider and Stevenson (Citation1991), Huang and Liu (Citation1994), and Battese and Coelli (Citation1995) model of truncated normal distribution assumption model, which caters for heteroskedasticity and exogenous determinants of technical inefficiency. The estimated TE results are presented in .
Table 1. Summary of technical efficiency distribution for the pooled sample
From the pooled sample (370), the frontier/best and least efficient farmers were 100% and 20%, respectively. On average (51.43%), the results generally show that the majority of the smallholder resettled sugarcane farmers are operating below their full potential. For the microfinance participants (214 farmers), the best farmer was 100% technically efficient (frontier farmer), the least efficient was 38.4%, and the mean efficiency level was 64%. For the non-participants (156 farmers), the best farmer was 65.13%, worst farmer 20.28% and mean TE levels of 33.58%. Furthermore, the results revealed that under the two main categories of microfinance participation, the best or most efficient farmer under MFI (Getbucks) beneficiaries was found to be 100% efficient, the least with 38.4%, and the mean TE level of 63.25%. For the Bank (Agribank) microfinance participants, the best farmer was 94.57% efficient; the least efficient was 43.65% efficient and mean TE level of 65.38%.
4.1.1. Technical efficiency scores distribution for the microfinance participants
From , mean TE for microfinance participants was 64.44%. Only 6.54% of microfinance participants operating below 40% TE, 22.43% operated between 41% and 60% level of TE, 33.18% operated between 61% and 80% level of TE, and a larger proportion (37.85%) operated above 80% level of TE.
Table 2. Technical efficiency distribution for smallholder resettled sugarcane farmers
4.1.2. Technical efficiency scores distribution for the microfinance non-participants
From the TE distribution results for the non-participants presented in , the mean TE was 33.58% and this was way below the average TE level of the microfinance participants (64.44%). A greater percentage (44.23%) of farmers (microfinance non-participants) operated below 40% level of TE, 33.97% operated between 41% and 60% levels of TE, 13.46% operated between 61% and 80% levels of TE, and a few (8.34%) operated above 80% level of TE. For the two main categories, it was concluded that participating in microfinance significantly increased TE levels for smallholder resettled sugarcane farmers (64.44% against 33.58% mean TE).
4.1.3. Technical efficiency distribution for MFI (Getbucks) and bank (Agribank) participants
From , TE distribution of MFI microfinance participants, mean TE was 63.25%, about 7.27% of the farmers in this sub-category operated below 40%, 23.64% operated between 41% and 60%, the majority (40%) operated between 61% and 80%, while about 29.09% operated at over 80% levels of TE. However, for Bank microfinance participants, subcategory, 5.77% of farmers operated below 40% level of TE, 21.15% of 104 Bank participants operated between 41% and 60% levels of TE, 25.96% operated between 61% and 80%, while the majority (47.12%) operated above 80% level of TE.
Despite both sources of microfinance generally resulting in increased TE compared to non-participants, mean TE for Bank participants of 65.38% was greater than that of MFIs microfinance participants of 63.25%. This suggest that participating in microfinance through the formal financial institutions (Banks) increases TE compared to participating in microfinance through MFIs despite the frontier farmer being an MFI participant. However, on average, Bank microfinance participants were 2.13% more efficient (technically) than MFIs microfinance participants. Banks engage in other non-financial services such as monitoring the borrowers’ (farmers) progress. This is because many banks have been able to finance agriculture including plantation (sugarcane) farming from the onset of the agricultural seasons (funding land preparation, planting, weeding and even equipment purchase or initial hiring costs) and be able to wait for the whole sugar-cane growing season. The grace or waiting period in sugarcane farming can stretch up to 14 months depending at the stage at which the farmer borrowed. There are risks such as crop-failure and default risk, which resulted in the financial (Banking institutions) designing some farmer progress monitoring schemes thereby significantly contributing towards judicious, productive and efficient utilization of borrowed funds (microcredit).
In addition to the TE variations between Bank beneficiaries and MFI beneficiaries, banks are able to cover any possible risks associated with micro-lending through other products like formal credit and other banking products such as accepting deposits which MFIs cannot; hence, they can spread their risks and offer some non-financial microfinance services such as farmer monitoring and advisory services. Also, credit risk (specifically default risk) is less prevalent to banks who offer microcredit because farmers usually borrow from the financial (banking) institutions they normally bank with (for other mainstream financial services) unlike MFIs who normally create new and short-term relationships with farmers hence they tend to be more stringent and not fully financed to offer other microfinance services. MFIs therefore tend to be more stringent on both the amounts they lend, administration and processing charges than the formal banking institutions where relationships already exist. Banks ride on their existing clients and also to borrow, farmers are obliged to open bank accounts with the banks they want to borrow from thereby by creating a lasting relationship, which also reduces credit/default risk and also paves way for monitoring by banks so that the microcredit is put into productive use.
4.2. Results of hypothesis tests
Two hypotheses were tested first to check for the presence of inefficiency to confirm whether the frontier model specification was justified or not. Second, applicable model specification was checked between the Cobb–Douglas stochastic frontier and the Translog model specifications (by checking for the significance of the interaction terms in affecting output of sugarcane (cross-elasticities)). Both hypothesis tests were contacted using the mixed chi-square table of Kodde and Palm (Citation1986), as shown in
Table 3. Log-likelihood tests for model
4.3. Critical values were adopted from Kodde and Palm (Citation1986)
From the results, the null hypothesis was that inefficiency effects are not present (
) against the alternative hypothesis that inefficiency effects are present (
). The null hypothesis of no inefficiency effects was rejected at 1 percent level of significance meaning the traditional OLSFootnote12 was not an adequate representation of the model. This implied that the variation of the total production among the resettled smallholder sugarcane farmers was due to the differences in inefficiencies.
From the second hypothesis, results indicated that square and interaction terms in the translog model specified are significantly different from zero; thus, the translog model could not be reduced to the Cobb–Douglas specification. The second hypothesis embraced the validity of translog over the Cobb–Douglas specification within the ML specifications using null hypothesis :
. The degrees of freedom of 8 and critical value of 25.370 is smaller than the LR statistic of 71.775, so the null hypothesis was rejected at all levels of significance meaning
. Given that, the translog model specification was more appropriate than the Cobb–Douglas form.
4.4. Translog stochastic frontier model results
This was conducted to test the responsiveness of sugarcane output to a change in input, (percentage change, double change and cross changes). From , Land, seed, labour, and irrigation had positive and statistically significant coefficients as expected and significance levels by t-statistics of 1.88, 1.85, 3.92, and 3.34, respectively, conforming to empirical findings of Mango et al, (Citation2015), Dessale (Citation2019), and Haji and Tegegne (Citation2018). The output elasticities of the four factors of production are positive with labour having the largest of 156%, followed by seed with 122%, while land and irrigation have 112% and 36%, respectively. As the theory of production acknowledges that an increase in one production factor attracts an increase in output, sugarcane output was positively related to land, labour, seed, and irrigation. Thus, the results suggest that, farmers were operating below the full employment level of factors of production thereby justifying the existence of technical inefficiency.
Table 4. Output elasticity of inputs (MLEa of the translog stochastic production frontier model)
Second-order derivatives have been chosen to acknowledge the fact that factors of production are non-competing together with the factor markets according to Hossain and Rahman (Citation2012). This was also selected to increase the flexibility of the model through the increase in the parameters estimated as asserted in S. Kumbhakar and Wang (Citation2015).
The results of the SFA analysis show that most of the interaction coefficients turned out to be highly significant indicating that the usage levels of the five inputs were interdependent on each other. Given that the majority of the second-order coefficients (,
,
,
,
,
,
,
, and
) are significant in the Translog SFA under the truncated normal distribution assumption with heteroskedasticity and exogenous determinants. This was in the exception of land squared; land and labour; land and seed; land and irrigation and fertiliser; and chemicals and irrigation (
,
,
,
, and
). On another note, labour squared, labour and fertilizer and chemicals and irrigation (
,
, and
) had significant but, negative coefficients meaning these inputs can be viewed as substitutes in sugarcane production. The other seven own and cross elasticities (
,
,
,
,
, and
) had positive and significant influence on sugarcane output. Therefore, these inputs can be considered as complements in production economic theory. The majority of the cross elasticities exhibited the existence of general interdependent/complementary nature of inputs. Agriculture inputs should not always be treated as competing/substitutes.
4.5. Technical inefficiency model
The focus of the analysis was to provide an empirical analysis of the determinants of productivity and inefficiency gaps among A2 smallholder resettled sugarcane farmers in Zimbabwe. Having knowledge that farmers were technically inefficient is not sufficient until the sources of technical inefficiency are identified. Thus, the study further investigated farm and farmer-specific attributes that impact on smallholder resettled farmers’ TE. This was therefore estimated using the Truncated Normal model with Heteroskedasticity and Exogenous determinants (Wang (Citation2002) model as in S. Kumbhakar and Wang (Citation2015)). The model was selected to cater for homoscedasticity in a truncated normal model according to Battese and Coelli (Citation1995). The model also caters for exogenous determinants according to KGMHLBC. Combined in the Wang (Citation2002) model, the selected model allows a further estimation of the marginal effects. Given the simultaneous estimation of the translog SFA and the prediction of technical efficiency scores, the technical efficiency scores were then simultaneously regressed against a set of independent variables (Technical inefficiency determinants).
From , given that the dependant variable is technical inefficiency, a positive sign of the coefficient shows the negative effect of that variable on TE. Microfinance participation, magnitude of participation, household farming assets, age, secondary education, tertiary education, off-farm income, and extension visits/services were found to be negative and significantly responsible for TE variations amongst the smallholder resettled sugarcane farmers. The results further showed that, former estate worker was a positive and significant determinant of TE variation.
Table 5. Determinants of technical inefficiency
Microfinance participation was found to be a negative and statistically significant determinant of technical inefficiency that is by participating in microfinance (microcredit), farmers increase their TE levels. On average, a one percent increase in microfinance participation result in a 9.7% increase in TE as indicated by the marginal effects of the mean (musigmas). Participating in microfinance also increases the certainty of technical TE 5.3% as indicated by the marginal effects of the usigmas. The results, therefore, were found to be consistent with the empirical works of Islam et al. (Citation2011), Asefa (Citation2012), Wadud (Citation2013), and Sarker et al. (Citation2016) who also found positive relationship between TE and microfinance (microcredit).
The magnitude of participation (MF) representing the amount borrowed proved to be a positive and significant determinant of TE. From the results, a percentage increase in the magnitude of participation (amount borrowed) simultaneously increases, on average, the level of TE (by 3.22%) and the certainty of TE (by 3.5%). As the amount borrowed increases, the level of TE increases as hypothesised. In other words, participating with smaller amounts does not significantly increase technical efficiency. The results therefore conformed to the findings of Wadud (Citation2013); and Dessale (Citation2019) who found a positive relationship between microfinance (microcredit) and TE.
Household farming assets (HFA) had a negative and statistically significant coefficient meaning it helps in explaining TE variations among the smallholder resettled sugarcane farmers. Thus, a percentage increase in the ownership of farming assets simultaneously increases, on average, the level of TE for the smallholder resettled sugarcane farmers by (8.77%) and the certainty of the TE (by 3.4%). This is because being in ownership of such assets reduces the time lags, inconveniences, and production lags associated with hiring equipment (timeous transportation of inputs and output) thereby increasing TE.
Following the treatment and behaviour of age (of farmer) as in Asefa (Citation2012) guided by Wittenberg (Citation2010), age was squared (for optimal age) and used in conjunction with age. Both proved to significantly (positively at 5% and negatively at 10% levels of significance, respectively) explain TE variation among smallholder A2 resettled sugarcane farmers despite the null hypothesis of negative impact on TE. A percentage increase in the farmer’s age result in the farmer’s TE increasing (due to farming experience, knowledge, skills and physical capability). However, after a farmer reaches a certain age interval specifically at 67 years for this study, age tended to have negative influence on TE symbolising an inverted u-shaped (nonmonotonic) relationship between age and TE. The findings were consistent to Asefa (Citation2012) who also found an inverted u-shaped relationship between age and TE (though at a different age turning point).
Household head’s highest education qualification was decomposed into no schooling, primary education, secondary education, and tertiary education. The results revealed that secondary and tertiary education has negative and statistically significant coefficients in explaining TE variations amongst the smallholder resettled sugarcane farmers. Secondary and tertiary education qualifications aids farmers to produce higher output from efficiently utilising the existing recourses. A percentage increase in a farmer’s education result in TE efficiency increases. For instance, improvement from primary to secondary education increases average TE (by 6.1%) and also increases the certainty of the TE (by 0.2%). Improvement from primary education qualification to tertiary education qualification increases TE (by 9.3%) and reduces the uncertainty of technical inefficiency (by 1.24%). The results were consistent with Haji and Tegegne (Citation2018); and Dessale (Citation2019).
Experience was also found to be a negative and statistically significant determinant of technical inefficiency. The findings indicated that as experience of the smallholder farmer increases, the level of TE increases. Experienced farmers are more efficient than inexperienced farmers. A percentage increase in a farmer’s experience simultaneously increases on average; TE (by 1.52%) and the certainty of TE (by 0.22%). The results (positive relationship between farming experience and TE) conforms to Wadud (Citation2013); and Prasanna and Lakmali (Citation2016). Likewise, former estate worker (FEW) exhibited a negative and statistically significant relationship with technical inefficiency. Allocating land to former sugar estate workers simultaneously increases the level of TE (by 0.29%) and the certainty of TE (by 0.3%). The positive influence of former estate workers on TE is via experience earned during the period worked for the Estates (Tongaat Hullets). For efficient utilisation of sugarcane farming resources, land for sugarcane farming should be allocated to estate workers where their estate working experience will be valid and utilised on their own farms meaning their working experience in the same environment counts.
Extension visits also have a negative and statistically significant relationship with technical inefficiency. As extension visits per sugarcane growing season increases, TE increases. A percentage increase in extension visits simultaneously increases TE (by 4.61%) and the certainty of TE (by 0.11%). This was found to be consistent with Mango et al.et al (Citation2015), Dube and Guveya (Citation2014) and Haji and Tegegne (Citation2018) who also found a positive relationship between extension visits and TE for farmers. However, off-farm income was found to be positive and statistically significant (not as hypothesised). Increases in off-farm income result in an increase in technical inefficiency due to increase off-farm activities (dedication and sugarcane farming value reduced). A percentage increase in off-farm income simultaneously decreases the level of TE (on average by 4%) and the certainty of TE (by 0.4%). These findings are consistent to Haji and Tegegne (Citation2018) and Asefa (Citation2012) who found off-farm income to negatively affect TE. This might be due to the fact that growth in off-farm income emanates from increases in off-farm activities hence there will be diversion of attention to the off-farm activities at the expense of sugarcane farming activities.
4.6. Conclusion
Smallholder A2 resettled sugarcane farmers under their existing levels of technology are not technically efficient implying a wide variation of output below frontier output. There is vast potential for increasing TE with proper allocation of their existing resources. Microfinance plays a significant role in increasing the TE of the smallholder resettled sugarcane farmers. Microfinance improves the existing levels of input use (technical efficiency) and decreases the existing levels of input wastage (technical inefficiency). Furthermore, the microfinance (microcredit) provided by the formal financial institutions (Banks) is slightly more efficient enhancing than microfinance provided by semi-formal institutions (MFIs). Sugarcane production in Zimbabwe can be increased if integrated development efforts such as agriculture financing mechanisms (microfinance (microcredit)) are embraced. For agro-based economies like Zimbabwe, microfinance therefore promotes inclusive economic growth. This will be through promoting the participation of the previously marginalised (smallholder farmers) in the economic development discourse (inclusive growth).
4.7. Policy recommendations
Connected to the foregoing findings of the study, policy makers should make efforts in strengthening financial institutions like MFIs. Banking institutions should also be encouraged to establish microfinance units to serve the marginalised smallholder farmers niche.
Farmers are generally recommended to participate in microfinance (microcredit) initiatives and further recommended to take advantage of available microfinance service providers (Banks and MFIs) for better and maximum utilisation of resources.
Specialised farming and farm budgeting is also recommended.
The government also encouraged (through the Reserve Bank of Zimbabwe) should constantly review the maximum thresholds for microfinance taking cognisance of the dynamic macroeconomic trends (inflation and exchange rate dynamics).
4.8. Implications/recommendations for future land resettlement programmes
Microfinance—Given the significance of both microfinance and the size and magnitude of participation, there should be plant/crop specific complementary financing mechanisms before or on the onset of any land redistribution exercise.
Extension services—Prior land resettlement exercises, the government (through the Ministries of agriculture and Higher and tertiary education) should avail extension support mechanisms (train and provide adequate extension workers) for the newly resettled farmers.
Household farming assets (HFA)—Land resettlement exercises by the government should give first priority to farmers who own farming assets or who are capable of purchasing and or vary land size accordingly.
Experience and former estate worker—Resettlement exercises should also prioritize former farm workers given that their experience gained as a farm worker counts on TE.
Age—Households/potential farmers over and closer to 67 years of age should not be prioritized since they will be over and closer their maximum level of productivity and TE. Therefore the young and middle aged should be given the first priority and or this can be catered for through land sizes.
Education—Secondary and Tertiary educational qualifications of farmers/potential farmers should be the minimum requirement for land allocations and or be catered for through varying land sizes (increasing land size with educational qualifications).
Disclosure statement
No potential conflict of interest was reported by the author(s).
Additional information
Funding
Notes on contributors
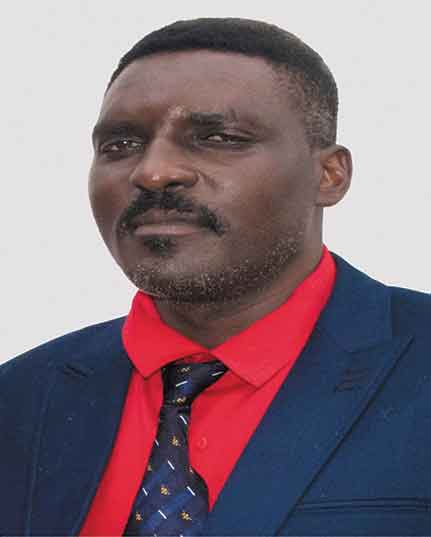
Simion Matsvai
Simion Matsvai ([email protected]) is a PhD holder in Agriculture Economics from the University of Fort Hare (South Africa) and a lecturer at Great Zimbabwe University (Department of Economics) with interdisciplinary research interests in Agriculture economics, Productivity & Efficiency Analysis, Micro-econometrics, Development Economics, Financial Economics, International and Applied Economics. His career goal is to contribute positively to the above disciplines of Economics.
Notes
1. Operation Maguta means bumper harvest and it was a programme launched in 2005 earmarked to boost food security and was spearheaded by the Joint Operations Command (JOC) comprising the army, police, prisons and the intelligence.
2. Command agriculture is a Zimbabwean agricultural scheme of 2016 aimed at ensuring food self-sufficiency.
3. Pfumvudza is a climate smart conservation agriculture aimed at boosting food security in Zimbabwe.
4. Technical Efficiency.
5. Decision Making Unit in which refer to farmer/household/farm in relation to this study.
6. Smallholder commercial farmers’ scheme of the FTLRP with slightly bigger pieces of land than the A1 scheme and it involves land allocations greater than 5 ha per household. This is the type of land allocation scheme carried out in sugarcane producing areas around Chiredzi in Zimbabwe.
7. Data Envelopment Analysis which is a nonparametric approach to the measurement of efficiency.
8. Transcendental Logarithmic Stochastic Frontier Model commonly referred to as the Translog Stochastic Frontier Model.
9. Fast Track Land Reform Programme which involved the redistribution of land through the smallholder farming (A1), smallholder commercial (A2) and the large-scale commercial farm allocation schemes.
10. Agricultural Development Bank of Zimbabwe formed to provide agricultural finance to the agribusiness sector through both long-term and short-term (microcredit) finance.
11. The estimation of TE incorporating the submissions of Kumbhakar, Ghosh, and McGuckin (Citation1991); Reifschneider and Stevenson (Citation1991); Huang and Liu (Citation1994); and Battese and Coelli (Citation1995).
12. Ordinary Least squares estimation technique.
References
- The Microfinance gateway, (2008).
- Aigner, D. J., Lovell, C. A. K., & Schmidt, P. (1977). Formulation and estimation of stochastic frontier production function models. Journal of Econometrics, 6(1), 21–23. https://doi.org/10.1016/0304-4076(77)90052-5
- Ambali, O. I. (2013).Microcredit and technical efficiency of rural farm households in Egba Division of Ogun State Nigeria. Journal of Agriculture and Sustainability, 2(2), 196–211.
- Anang,T. B., Bäckman, S., & Sipiläinen, T. (2016). Agricultural microcredit and technical efficiency: The case of smallholder rice farmers in Northern Ghana. Journal of Agriculture and Rural Development in the Tropics and Subtropics, 117(2), 189–202. http://hdl.handle.net/10138/233240
- Asefa, S. (2012). Who is technically efficient in crop production in Tigray Region, Ethiopia? Stochastic Frontier Approach. Department of Economics, Wollega University. Ethiopia.
- Bangwayo-Skeete , P. F., Bezabih, M., & Zikhali, P. (2010). Are Zimbabwe’s fast track land reform farms more technically efficient than communal farms? Quarterly Journal of International Agriculture, 49(4), 319–339. https://www.researchgate.net/publication/289430217
- Battese, G. E., & Coelli, T. J. (1995). A model for technical inefficiency effects in a stochastic frontier production function for panel data. Empirical Economics, 20(2), 325–332. https://doi.org/10.1007/BF01205442
- Battese, G. E., & Corra, G. S. (1977). Estimation of a production frontier model: With application to the pastoral zone of eastern Australia. Australian Journal of Agricultural Economics, 21(3), 169–179. https://doi.org/10.1111/j.1467-8489.1977.tb00204.x
- Christen, R. P., Lyman, T. R., & Roesenburg, R., (2003). Microfinance consensus guidelines: Guiding principles on regulation and supervision of microfinance. Consultative group to Assist the Poor, World Bank.
- Christensen, L. R., Jorgenson, D. W., & Lau, L. J. (1971). Conjugate duality and transcendental logarithmic function. Econometrica, 39(4), 255–256.
- Coelli, T. J., Rao, D. S. P., O'Donnell, C. J., and Battese, G. E. (2005). An introduction to efficiency and productivity analysis. 2nd ed. New York, NY, United States: Springer. https://doi.org/10.1007/b136381
- Conning, J., & Udry, C. (2005). Rural financial markets in developing countries, economic growth centre. Discussion Paper No. 914, Economic Growth Center, Yale University.
- Debreu, G. (1951). The Coefficient of resource utilization. Econometrica, 19(3), 273–292. https://doi.org/10.2307/1906814
- Dessale, M. (2019). Analysis of technical efficiency of small holder wheat-growing farmers of Jamma district, Ethiopia. Agriculture & Food Security, 8(1), 1. https://doi.org/10.1186/s40066-018-0250-9
- Dorfman, J. H., & Koop, G. (2005). Current developments in productivity and efficiency measurement. Journal of Economics, 126(2), 233–240. https://doi.org/10.1016/j.jeconom.2004.05.001
- Dube, L., & Guveya, E. (2014). Technical efficiency of smallholder out-grower tea (Camellia sinensis) farming in Chipinge District of Zimbabwe. Greener Journal of Agricultural Sciences, 4(8), 368–377. https://doi.org/10.15580/GJAS.2014.8.091114354
- Färe, R., Grosskopf, S., & Lee, H. (1990). A nonparametric approach to expenditure-constrained profit maximization. American Journal of Agricultural Economics, 72(2), 574–581. https://doi.org/10.2307/1243026
- Färe, R., Grosskopf, S., and Lovell, C.A.K. (1994). Production Frontiers. Cambridge University Press, Cambridge.
- Farrell, M. J. (1957). The measurement of productive efficiency. Journal of the Royal Statistical Society, Series A, 120(III), 253–281. https://doi.org/10.2307/2343100
- Greene, W. H. (2010). A stochastic frontier model with correction for sample selection. Journal of Productivity Analysis, 34(1), 15–24. https://doi.org/10.1007/s11123-009-0159-1
- Grosskopf, S. (1993). Efficiency and productivity. In H. O. Fried, C. A. K. Lovell, & S. S. Schmidt (Eds.), The measurement of productive efficiency (pp. 160–194). Oxford University Press.
- Haji, J., & Tegegne, B. (2018). Technical efficiency of sorghum production: The case of smallholder farmers in Konso District, Southern Ethiopia. Journal of Agricultural Economics, Extension and Rural Development, 6(7), 772–793. https://doi.org/10.20448/journal.523.2018.31.1.15
- Hossain, M. E., & Rahman, Z. (2012). Technical efficiency analysis of rice farmers in Naogaon district: An application of the stochastic frontier approach. Journal of Economics and Development Studies, 1(1), 1–20.
- Huang, C., & Liu, J.-T. (1994). Estimation of a non-neutral stochastic frontier production function. Journal of Productivity Analysis, 5(2), 171–180. https://doi.org/10.1007/BF01073853
- Islam, K. M. Z., Bäckman, S., & Sumelius, J. (2011). Technical, economic and allocative efficiency of microfinance borrowers and non-borrowers: Evidence from peasant farming in Bangladesh. European Journal of Social Sciences, 18(3), 361–377. http://hdl.handle.net/10138/158534
- Kathuria, V., Raj, R. S. N., & Sen, K. (2013). Productivity measurement in Indian manufacturing: A comparison of alternative methods. Journal of Quantitative Economics, 11(1/2), 148–179. doi:10.4236/tel.2017.76124
- Kodde, D. A., & Palm, F. C. (1986). Wald criteria for jointly testing equality and inequality restrictions. Econometrica, 54(5), 1243–1248. https://doi.org/10.2307/1912331
- Koopmans, T. C. (1951). Efficient Allocation of Resources. Econometrica, 19(4), 455–465. https://doi.org/10.2307/1907467
- Kumbhakar, S. C., Ghosh, S., and Mcguckin, J. T. (1991). A Generalized Production Frontier Approach for Estimating Determinants of Inefficiency in U.S. Dairy Farms, Journal of Business & Economic Statistics, Volume 9, 279–286.
- Kumbhakar, S. C., & Lovell, C. A. K. (2000). Stochastic frontier analysis. Cambridge University Press.
- Kumbhakar, S., & Wang, H.-J. (2015). A practitioner’s guide to stochastic frontier analysis using Stata. Cambridge University Press.
- Mango, N., Makate, C., Mlambo, B. H., Siziba, S., & Lundy, M. (2015). A stochastic frontier analysis of technical efficiency in smallholder maize production in Zimbabwe: The post-fast-track land reform outlook. Cogent Economics & Finance. doi:10.1080/23322039.2015.1117189
- Meeusen, W., & van Den Broeck, J. (1977). Efficiency estimation from Cobb-Douglas production functions with composed error. International Economic Review, 18(2), 435–444. https://doi.org/10.2307/2525757
- Prasanna, R., & Lakmali, N. (2016). Estimating technical efficiency among smallholder potato producers in Welimada, Sri Lanka. Sri Lanka Journal of Economic Research, 4(1), 59–74. https://www.jstor.org/stable/24737403
- Rana, Z., & Bapari, Y (2018). Credit availability and technical efficiency of boro rice in Pabna District, Bangladesh: A stochastic frontier analysis. International Journal of Sustainable Development Research, 4(1), 08–16. https://doi.org/10.11648/j.ijsdr.20180401.12
- Reifschneider, D., & Stevenson, R. (1991). Systematic departures from the frontier: A framework for the analysis of firm inefficiency. International Economic Review, 32(3), 715–723. https://doi.org/10.2307/2527115
- Sarker, M. A. S., Arshad, F. M., Alam, F., Zainal Abidin Mohamed, F. Z. A., & Akhtaruzzaman Khan, M. (2016). Stochastic modeling of production risk and technical efficiency of Thai koi(Anabas testudineus) farming in Northern Bangladesh. Aquaculture Economics & Management, 20(2), 165–184. https://doi.org/10.1080/13657305.2016.1156189
- Tahir, I. M., & Tahrim, C. S. N. (2015). Efficiency and productivity analysis of microfinance institutions in Cambodia: A DEA approach. International Review of Business Research Papers, 11(1), 25–42. https://doi.org/10.21102/irbrp.2015.03.111.03
- Wadud, M. A. (2013) Impact of microcredit on agricultural farm performance and food security in Bangladesh. Dhaka: Institute of Microfinance, Working Paper 14/13.
- Wang, H.-J. (2002). Heteroscedasticity and non-monotonic efficiency effects of a stochastic frontier model. Journal of Productivity Analysis, 18(3), 241–253. https://doi.org/10.1023/A:1020638827640
- Wittenberg, M. (2010). Econometrics through applications: A practical handbook School of Economics and SALDRU. University of Cape Town.
- World Bank (2006) The role of postal networks in expanding access to financial services—Volume I [Discussion Paper]. Global Information and Communication Technologies Group, the World Bank.
Appendix 1
Summarized Results
Table
Appendix 2
Stata Results
Marginal Effects and Exogenous Determinants of Technical Inefficiency estimates
. sf_predict, bc(bc_w) marginal
The following is the marginal effect on unconditional E(u).
The average marginal effect of MFI on uncond E(u) is −.0970158 (see MFI_M).
The average marginal effect of MF on uncond E(u) is −.0321612 (see MF_M).
The average marginal effect of SEX on uncond E(u) is .03417428 (see SEX_M).
The average marginal effect of HFA on uncond E(u) is −.0876534 (see HFA_M).
The average marginal effect of AGE on uncond E(u) is −.0102832 (see AGE_M).
The average marginal effect of AGE2 on uncond E(u) is −.00010449 (see AGE2_M).
The average marginal effect of Edctn2 on uncond E(u) is −.0066044 (see Edctn2_M).
The average marginal effect of Edctn3 on uncond E(u) is −.0605580 (see Edctn3_M).
The average marginal effect of Edctn4 on uncond E(u) is −.09343946 (see Edctn4_M).
The average marginal effect of HHS on uncond E(u) is −.00770858 (see HHS_M).
The average marginal effect of HHSS on uncond E(u) is −.01743677 (see HHSS_M).
The average marginal effect of EXP on uncond E(u) is −.01512843 (see EXP_M).
The average marginal effect of FEW on uncond E(u) is −.02220299 (see FEW_M).
The average marginal effect of NOF on uncond E(u) is .08971299 (see NOF_M).
The average marginal effect of OFY on uncond E(u) is .03998709 (see OFY_M).
The average marginal effect of EXT on uncond E(u) is −.006046 (see EXT_M).
The average marginal effect of MFA on uncond E(u) is −.01281996 (see MFA_M).
The average marginal effect of MS on uncond E(u) is .01262517 (see MS_M).
The following is the marginal effect on uncond V(u).
The average marginal effect of MFI on uncond V(u) is −.0528961 (see MFI_V).
The average marginal effect of MF on uncond V(u) is −.0345063 (see MF_V).
The average marginal effect of SEX on uncond V(u) is −.0061868 (see SEX_V).
The average marginal effect of HFA on uncond V(u) is −.0337769 (see HFA_V).
The average marginal effect of AGE on uncond V(u) is −.00241315 (see AGE_V).
The average marginal effect of AGE2 on uncond V(u) is .00001511 (see AGE2_V).
The average marginal effect of Edctn2 on uncond V(u) is .00736751 (see Edctn2_V).
The average marginal effect of Edctn3 on uncond V(u) is −.00145136 (see Edctn3_V).
The average marginal effect of Edctn4 on uncond V(u) is −.01237477 (see Edctn4_V).
The average marginal effect of HHS on uncond V(u) is .00104193 (see HHS_V).
The average marginal effect of HHSS on uncond V(u) is −.0005041 (see HHS_V).
The average marginal effect of EXP on uncond V(u) is −.00223782 (see EXP_V).
The average marginal effect of FEW on uncond V(u) is −.00293835 (see FEW_V).
The average marginal effect of NOF on uncond V(u) is −.00825001 (see NOF_V).
The average marginal effect of OFY on uncond V(u) is −.00364343 (see OFY_V).
The average marginal effect of EXT on uncond V(u) is −.00104075 (see EXT_V).
The average marginal effect of MFA on uncond V(u) is .00504112 (see MFA_V).
The average marginal effect of MS on uncond V(u) is −.00003525 (see MS_V).