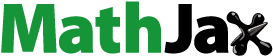
Abstract
Given the impact of the COVID-19 pandemic on the European real economy, European banks are likely expected to be confronted by a wave of non-performing loans. Focusing on a sample of large banks in the EU area over the pre and during the COVID-19 pandemic period, the analysis shows an extended degree of divergence during the pandemic crisis, with GDP, bank profitability and risk being held the responsible factors. The findings imply that potential plans to deal with NPLs should explicitly consider strategies running from bank restructuring and resolutions to recapitalisation or any other rescue measures.
PUBLIC INTEREST STATEMENT
Since the time of the global financial crisis, non-performing loans (NPLs) have been treated as a serious problem for banking systems; the presence of the COVID-19 pandemic is expected to deteriorate the impact of NPLs. This research study explored convergence across EU banks to determine whether NPLs follow homogeneity across EU. The results highlight a divergent pattern in the pandemic period. Responsible factors are economic growth, bank profitability and bank risks, since they behave differently across countries in the pandemic era. The findings recommend that policymakers should ensure that banks assess current loan values realistically, which can be achieved by effective stress tests, adequate accounting rules, and specific inspections that impede banks masking their risk. Moreover, the development of secondary markets for NPLs is also highly recommended, because in future pandemic periods can deal better with information asymmetries and banks’ lacking incentives to sell loans at market prices. This will reduce the need for government bailouts, with future positive spillovers to the real economy.
1. Introduction
In the aftermath of the COVID-19 pandemic crisis, it is more than certain that more challenges will inflict banking system (Beck & Keil, Citation2021). One of these challenges is whether the pandemic crisis will affect non-performing loans (NPLs) on banks’ balance sheets, which of course does not only depend on pure banking/liquidity factors, but also on idiosyncratic cross-sector differences (Demmou et al., Citation2021), the presence of fiscal programs addressing firms and households (Aussilloux et al., Citation2021), and the degree of uncertainty linked to lockdowns (Ornelas, Citation2020).
It is well known that the presence of a high volume of NPLs has detrimental effects on a high stock of non-performing loans may have detrimental effects on banks’ profits, while require high provisions and large managerial resources (Aiyar et al., Citation2015), as well as high funding costs since they are used as an indicator to forecast bank failures (Lu & Whidbee, Citation2013). In addition, NPLs have a negative impact on bank lending, thus disrupting the smooth functioning of the real economy (Accornero et al., Citation2017).
In terms of the European banks, Kasinger et al. (Citation2021) document that aggregated bank capital seems to be large enough to absorb potential NPL losses, especially during and after the pandemic crisis. Nevertheless, their analysis illustrates substantial heterogeneity across European Union (EU) countries, not only in terms of the size of NPLs, but also in terms of the relation of NPLs to bank capital. This cross-country heterogeneity suggests two key problems associated with the functioning of the European banking system, i) less-capitalised banks are substantially vulnerable to credit crunch cases, raising systemic risk, in the event of higher NPLs volumes, and ii) the risk of zombie bank lending, along with high volumes of NPLs and insufficient equity capital, may generate negative spillovers to the banking system’s performance and the real economy.
Based on this discussion, the goal of this paper is twofold. First, to explore the convergence of NPLs across EU over two distinctive periods, the pre-COVID-19 period and during the pandemic, and second, to identify what factors are responsible for any potentially differentiated picture of convergence. The main contribution of this work is that it shows for the first time, to the best of my knowledge, the differentiated convergence picture across banks in the EU over the pre- and during the pandemic period, as well as it identifies certain NPL factors recommended by the literature driving the convergence process.
The results could be useful to determine differentiated levels of systemic risk across EU banks, which may necessitate potential government interventions. Moreover, based on the findings, policymakers can be prepared to prevent upcoming systemic banking crises events that require government bailout programs, either through the banking system, or directly channelling rescue money to viable firms and borrowers.
2. Methodology and data
The empirical analysis uses the Phillips and Sul (Citation2007) modelling approach to investigate whether there is convergence with respect to the time-varying idiosyncratic components after controlling for a common component across the NPLs that share the same convergence pattern. The methodological approach employs a time-varying common factor defined as:
where i = 1, …, N and t = 1, …, T. NPL represents non-performing loans. These NPLs are comprised of a common component, μt, and an idiosyncratic component, δit. This idiosyncratic component measures the distance between NPL and the common component, μ. Next, the relative transition parameter, hit, is defined as follows:
EquationEquation (2)(2)
(2) measures the loading coefficient, δit, relative to the panel average; the transition path for NPL i relative to the panel average. If the factor loadings, δit, converge to a constant, δ, then the cross-sectional mean of the relative transition path for NPLi, hit, converges to unity and the cross-sectional variation, Ht, of the relative transition path converges to zero as t → ∞:
i = 1
The semi-parametric form of δit is presented as:
where δi is fixed; ξit ~ iid(0,1) varies across the NPLs i = 1, 2, …, N, σi describes the idiosyncratic scale parameter, L(t) is a slow varying function, where L(t)→∞ and t→∞; and α represents the speed of convergence, while δit converges to δi for α ≥ 0. In other words, the null hypothesis of convergence is H0: δi = δ and α ≥ 0. The method sets L(t) = logt, so the empirical logt regression can test for convergence. Then convergence clubs are determined as follows:
for , …, T, where r > 0 set on the interval [0.2, 0.3].
The study obtains quarterly data on NPLs for the largest 144 bank banks across EU, spanning the period 2016–2021(March). It excludes banks not reporting complete balance sheet data and those with a ratio between loans to customers and total assets lower than 10%. Data were collected from the Orbis bank database. To avoid potential discontinuities in the balance sheet variables for banks involved in M&A transactions over the period considered, pre-M&A figures are adjusted to consider such processes and ensure comparability. NPLs are measured by the NPLs ratio, defined as dividing total impaired loans by total gross loans to customers. The denominator of this ratio includes mortgage loans, other retail loans, corporate and commercial loans, other loans and reserves for impaired loans, while the numerator represents the impaired loans included in gross loans to customers. For the second part of the analysis, we obtained data on real economic activity (measured by the real GDP), interest rates (measured as the interest rates on 10-year benchmark government bonds), and judicial efficiency (measured by the days required to enforce contracts; Bofondi & Ropele, Citation2011; Ghosh, Citation2015; Jassaud & Kang, Citation2015). The data on the former two variables were obtained from Datastream, while those on latter from the World Bank index, which measures the time necessary to resolve a dispute, from the moment the plaintiff files the lawsuit in court until payment. Data on additional variables were also obtained from the Orbis database: bank profitability (measured as ROA), capitalization (measured by the ratio between the Tier 1 capital and the risk-weighted asset), risk (measured by the risk weighted assets on total assets ratio), and loans (measured by total loans over total assets; Ghosh, Citation2015; Klein, Citation2013; Podpiera & Weill, Citation2008). The EU countries included in the sample, along with the number of banks considered in each country (in parentheses) are: Austria (4), Belgium (5), Bulgaria (3), Croatia (5), Cyprus (3), Czech Rep. (5), Denmark (5), Estonia (3), Finland (6), France (11), Germany (13), Greece (4), Hungary (4), Ireland (5), Italy (8), Latvia (3), Lithuania (3), Luxembourg (6), Malta (2), Netherlands (7), Poland (6), Portugal (5), Romania (4), Slovak Rep. (4), Slovenia (3), Spain (7), UK (14) (we included the country despite the Brexit event).
3. Empirical analysis
reports the results over the pre-COVID-19 period (2016–2020:2) in Panel A, while Panel B presents those during the pandemic period (2020:3–2021:3). The findings in Panel A clearly document that the point estimate of the log-t statistic in the 1st row is −22.619 (with critical value −1.67 at 5%), implying that full convergence is violated. Applying the club-clustering procedure reveals three different clubs (2nd- 4th rows). Belgium, France, Germany, Ireland, Italy and Spain banks form the first club, Austria, Check Rep., Denmark, Estonia, Finland, Latvia, Lithuania, Luxembourg, Malta, Netherlands, Slovak Rep., Slovenia, and UK banks form the second club, while Bulgaria, Croatia, Cyprus, Greece, Hungary, Poland, Portugal and Romania banks form the third club. The picture turns out different over the pandemic period (Panel B) where the full panel convergence hypothesis is also rejected, but this time five clubs are formed, implying that the pandemic crisis has differentiated the impact of COVID-19 conditions on NPLs across EU banks. These results also receive support from the last column which reports the speed of adjustment (or convergence) calculated as the absolute value of b/2. The findings clearly show that in the pre-Covid19 period, the countries in the 3rd club (Bulgaria, Croatia, Cyprus, Greece, Hungary, Poland, Portugal, and Romania) exhibit the lowest speed of adjustment, probably reflecting high rigidities in the banking system to handle fast and properly their NPLs. During the pandemic crisis periods, the countries in the fourth club (Belgium, Czech Rep., Ireland, Slovak Rep., and Slovenia) are those that display the lowest speed of adjustment, indicating that the pandemic has inflicted the efficiency of their banking systems to handle fast their NPLs.
Table 1. Convergence results: NPLs
To test the robustness of the above findings, the analysis considers the likelihood of merging these sub-clubs into larger clubs. Following Phillips and Sul (Citation2009), the analysis focuses on adjacent sub-clubs, and the results are reported in . The null of convergence is rejected across all adjacent cases, as well as across both regimes, implying that all clubs cannot be merged into a larger one.
Table 2. Club-merging convergence
The second part of the analysis will attempt to investigate the role of certain macro and micro variables (described in the data section) in explaining the distinctive convergence clubs across both regimes through an ordered logit model. The findings are shown in and document and interesting picture across the two regimes. A positive coefficient implies increased likelihood for bank NPLs in one country to move to a higher club and vice versa. The estimates highlight that an increase in real GDP, ROA, judicial efficiency, capitalization, and total loans increase the likelihood of joining Club 1 moving from Clubs 2 and 3 in the first regime and from Clubs 2, 3, 4 and 5 in the second regime. The opposite holds in the cases of long-term bond yields and risk. Furthermore, the findings during the pandemic period assign a more substantial role to the factors of GDP, ROA and risk to lead to convergence vis-à-vis those in the pre-COVID-19 regime, implying that economic growth, bank profitability and bank risk factors have been dramatically affected by the pandemic conditions, with their improvement playing a significant role in leading NPLs across European banks and countries to converge.
Table 3. Order logit estimates
4. Conclusion
Given that NPLs are treated as a serious problem in the EU, with the COVID-19 considered as a major threat for the future of the banking system, the analysis explored convergence across EU banks and countries. The findings documented an enhanced and different divergent picture over the pandemic regime with factors, such as economic growth, bank profitability and bank risks being the primary contributors, probably reflecting the high degree of uncertainty concerning the economic consequences of serial lockdowns. The high degree of uncertainty could be a significant reason why policy proposals on NPL resolution should take a scenario-based approach, i.e., be designed as conditional on the events unfolding. Therefore, bank policymakers (European Central Bank) and regulators should ensure that banks assess current loan values realistically, which can be achieved by effective stress tests, adequate accounting rules, and specific inspections that impede banks masking their risk. Hence, realistic loan value assessment is expected to incentivize banks to recognize their NPLs early and to handle them more efficiently, i.e., by internal workouts. Finally, policymakers in future crises periods are well advised to overcome certain obstacles hindering the development of secondary markets for NPLs, given that crises, such as the pandemic period, tend to enhance information asymmetries and banks’ lacking incentives to sell loans at market prices. A coordinated European policy towards NPLs even in stressful times, is expected to substantially mitigate bank risks. As a result, banking “systemic risk” is expected to drop, which will significantly reduce the need for a government bailout and, thus, channelling direct subsidies to viable firms and sectors in the real economy, with future positive spillovers not only for the economy, but also for the viability of the banking system.
Author statement
The author’s research activities explore energy issues, the interaction between financial markets and the macroeconomy, the role of monetary policy in a digitalised environment, and how the recent COVID-19 pandemic has affected certain sectors in the economy, including the banking sector, in terms of its profitability, risk engagement and efficiency. This current empirical work is part of the latter portfolio agenda.
Disclosure statement
No potential conflict of interest was reported by the author(s).
Additional information
Funding
References
- Accornero, M., Alessandri, P., Carpinelli, L., & Sorrentino, A. M. (2017). Non-performing loans and the supply of bank credit: Evidence from Italy. Occasional Papers Banca d’Italia, 374, 1–8. https://www.bancaditalia.it/pubblicazioni/qef/2017-0374/index.html?com.dotmarketing.htmlpage.language=1
- Aiyar, S., Bergthaler, W., Garrido, J. M., Ilyina, A., Jobst, A., Kang, K., Kovtun, D., Liu, Y., Monaghan, D., & Moretti, M. (2015). A strategy for resolving Europe’s problem loans (pp. 15/19). IMF Staff Discussion Note.
- Aussilloux, V., Baïz, A., Garrigue, M., Martin, P., & Mavridis, D. (2021, February 19). Fiscal plans in Europe: No divergence but no coordination. VoxEU.org.
- Beck, T., & Keil, J. (2021, March 11). Bank performance in the time of COVID-19: Evidence from the US. VoxEU.org.
- Bofondi, M., & Ropele, T. (2011). Macroeconomic determinants of bad loans: Evidence from Italian banks. Bank of Italy Occasional Paper Series, 89, 1–40. https://www.bancaditalia.it/pubblicazioni/qef/2011-0089/QEF_89.pdf
- Demmou, L., Calligaris, S., Franco, G., Dlugosch, D., McGowan, M. A., & Sakha, S. (2021). Insolvency and debt overhang following the COVID-19 outbreak: Assessment of risk and policy responses. Covid Economics, 69(1), 87–108. https://www.oecd-ilibrary.org/docserver/747a8226-en.pdf?expires=1640951905&id=id&accname=guest&checksum=9CDB24A577E4BFFECF471D314ECC4512
- Ghosh, A. (2015). Banking-industry specific and regional economic determinants of nonperforming loans: Evidence from US states. Journal of Financial Stability, 20(1), 93–104. https://doi.org/10.1016/j.jfs.2015.08.004
- Jassaud, N., & Kang, K. (2015). A strategy for developing a market for nonperforming loans in Italy (International Monetary Fund Working Paper, No. 24). International Monetary Fund.
- Kasinger, J., Krahnen, J. P., Ongena, S., Pelizzon, L., Schmeling, M., & Wahrenburg, M. (2021). Non-performing loans-new risks and policies? (SAFE White Paper, No. 84). Commissioned by the European Parliament upon request of the Economic and Monetary Committee. Economic Governance Support Unit (EGOV) Directorate-General for Internal Policies.
- Klein, N. (2013). Non-performing loans in CESEE: Determinants and impact on macroeconomic performance (International Monetary Fund Working Paper, No. 72). International Monetary Fund.
- Lu, W., & Whidbee, D. (2013). Bank structure and failure during the financial crisis. Journal of Financial Economic Policy, 5(3), 281–299. https://doi.org/10.1108/JFEP-02-2013-0006
- Ornelas, E. (2020, March 28). Managing economic lockdowns in an epidemic. VoxEU.org.
- Phillips, P. C. B., & Sul, D. (2007). Transition modeling and econometric convergence tests. Econometrica, 75(6), 1771–1855. https://doi.org/10.1111/j.1468-0262.2007.00811.x
- Phillips, P. C. B., & Sul, D. (2009). Economic transition and growth. Journal of Applied Econometrics, 24(7), 1153–1185. https://doi.org/10.1002/jae.1080
- Podpiera, J., & Weill, L. (2008). Bad luck or bad management? Emerging banking market experience. Journal of Financial Stability, 4(2), 135–148. https://doi.org/10.1016/j.jfs.2008.01.005