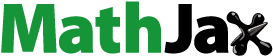
Abstract
The housing market is one of the key drivers of economic growth and social welfare in South Africa. However, the performance of the market depends on housing prices which are also subjected to changes in various socio-economic and political factors. The main purpose of this study is to determine the effects of inflation (consumer price index), interest rate, rental price and political risk on housing prices. To achieve this objective, the study applies Vector Autoregressive (VAR) model, the Johansen test for cointegration, Vector Error Correction Model (VECM) and Granger causality test on monthly data starting from January 2002 to December 2019. Findings of the study suggest that inflation, interest rate, rental and political risk influence the housing prices in both the long run and short run. Changes in political risk and rental price cause a long-run decline in housing prices while high-interest rates and inflation cause an increase in housing prices. Based on findings, researchers propose stability in the inflation rate, interest rate and political situation as a solution that can assist to improve the housing market and create price stability. Additionally, interest rate reduction can generate growth in housing demand resulting in the country’s economic improvement.
PUBLIC INTEREST STATEMENT
This research offers insight for investors, policymakers, government and other relevant stakeholders in the housing markets. With political and macroeconomic instabilities in South Africa, we are presented with a new opportunity to analyse the effect of political risk and macroeconomic fluctuations on price volatility within the South African housing market. To this end, it is indispensable to find an adequate approach that elucidates the housing market behaviour resulting from political and economic uncertainties to provide useful information to investors and policymakers. The finding of this study suggested that investors in the housing business should consider the movement of other factors, besides the housing price, such as housing location, country’s inflation, interest rate and the stability of governing institutions as the latter determine the failure or success of housing business. Having enough information on country risk and macroeconomic status can assist both investors and policymakers in forecasting future prices in the housing market.
1. Introduction
Housing or shelter is one of the elemental needs for the settlement and development of humans. Consequently, the housing market is one of the factors that contributes to any country’s socio-economic development and it is an instrument of economic growth in some of the developed and developing countries through investment growth and job creation (Shi et al., Citation2014). The housing market or real estate market is essential not only for the country’s economy but also for householders, investors, property developers and policymakers (Ahmed, Citation2020). Nonetheless, during the 21st century, housing price levels have become one of the crucial economic challenges and the seriousness of the matter was aggravated by the 2008 global financial crisis (Shi et al., Citation2014). Besides, Barrell and Davis (Citation2008) argue that the housing bubble experienced before the crisis was of causes and instigators of the 2008 financial crisis and yet were also affected by the same crisis.
Housing price is compared to a double-edged sword. On one hand, an increase in housing prices is more desirable as it improves economic conditions through wealth creation. On the other hand, a rise in housing prices reduces demand for houses and creates an increasing rental rate and this is harmful to both economic and social wellbeing. Additionally, an increase in housing prices may lead to growth in households’ expenditure which in return may cause high inflation and macroeconomic instability (Crowe et al., Citation2013; Shi et al., Citation2014). Thus, the negative effect of high prices for houses includes a reduction in wealth effect, decline in township housing and increase in housing investment rather than production improvement (Orren, Citation2003).
Generally, the housing price is associated with and influenced by various macroeconomic variables or factors. Understanding drivers of housing is indispensable not only because housing plays a vital role in a country’s wealth but also because its shocks influence changes in a country’s economy (Miller et al., Citation2005). Factors that influence change in housing price include, among others, economic growth, consumer price index, money supply, interest rate, unemployment rate, political risk and rental price. These aforementioned variables may have either positive or negative effects on housing prices. Knowing to which extend these macroeconomic factors influence housing prices can assist in making genuine investment decisions and formulate adequate policies (Cohen & Karpaviciute, Citation2017).
The South African housing market has been experiencing fluctuations in houses and property demand within different market segments. However, studies on causes of price shocks in the South African housing and properties industry are still limited (Balcilar et al., Citation2011; Burger & Van Rensburg, Citation2008). Against the discussed background, this paper aims to determine the responses of housing prices to shocks in some macroeconomic variables namely inflation rate, interest rate, rental prices and political risk in South Africa.
2. Literature review
South Africa’s housing and real estate market is one of the most healthier on the African continent owing to the country’s sophisticated and free-market economy. In this market furnished and unfurnished houses are sold. A large number of furnished houses is mostly obtainable in Cape Town and Johannesburg cities (Gil-Alana et al., Citation2013). The housing market accounts for a large share of the South African expenditure and the country economic activities. The housing market, in South Africa, is divided into two main categories namely luxury and affordable and their classification or segmentation is based on property size and prices. Thus, houses are classified as large, medium and small. To be more focused, this study focuses only on housing prices and how it is affected selected macroeconomic variables and political risk.
2.1. Development of housing market in South Africa
Ever since the mid-1980s, the South African housing market has experienced a significant transformation. In both the 18th and 20th centuries building societies were the crucial provider of finances in homeownership. These building societies aimed to close the housing gap witnessed during the agricultural and industrial revolutions in the 18th century (Tita & Opperman, Citation2021). To encourage savings and improve access to housing ownership, building societies created other non-profit friendly societies in Port Elizabeth in 1855 and Durban in 1857, in Northern Cape in 1870; and in Gauteng in 1886 correspondingly (Luüs, Citation2005). The discovery of gold in both the Northern Cape and Gauteng served as motivation to extend building societies in these two provinces. The establishment of building societies was based on both temporary and permanent objectives. Temporary societies were established for temporary objectives and once the latter were achieved the building societies had to be terminated. Permanent building societies were established for long-term objectives and once the set objectives were achieved the building societies could be transformed into financial institutions. An example is the transformation of the United Building Society created in 1889 and become the Amalgamated Banks of South Africa Limited (ABSA) in the 1990s (Luüs, Citation2005). Different financial institutions or banks which derived from the aforementioned building societies have become the main providers of mortgage and loans for housing in South Africa. It is important to note that these banks mortgages and loans involve interest rates and the latter depends on the country’s inflation rate, rental rate and country risk. Therefore, country risk, interest rate, inflation rate and rental price are some of the major variables that influence the housing market. The linkage between these variables and housing prices is discussed in section 2.2 through a review of the empirical literature.
In recent decades, the appetite for owning personal property of houses has increased due to a significant development in mortgage access and a cut in interest rate. Easy access to housing loans increased the demand for both loans and houses followed by a significant increase in housing prices and on mortgage finance. Nonetheless, this opportunity offered by financial institutions and banks is currently impeded by low household income as a consequence of growing unemployment and sluggish economic growth and it is intensified by the COVID-19 endemic (Centre for Affordable Housing Finance in Africa, Citation2021).
2.2. Empirical review on the effect of macroeconomic variables on housing prices
Although several economic variables influence the housing market, this study focuses on inflation, interest rate, rental and political risk in South Africa. Several empirical studies were conducted in various periods and different regions to analyse the relationship that may exist between inflation, interest rate, rental, political risk and housing prices. Some of those studies are discussed in the subsequent paragraphs.
Parrikar (Citation2019) conducted a study to analyse the impact of GDP, inflation, housing credit, interest rates and exchange rate on the House Price Index in India from 2010 to 2017. The findings of this study revealed the presence of a positive correlation between inflation, exchange rate, GDP and housing prices. However, a negative correlation was found between housing prices and interest rates. Similar results of an inverse relationship between interest rate and housing prices were revealed in the study of Julius (Citation2012) in Kenya. Another study was conducted by Shi et al. (Citation2014) to determine the effect of interest rates on housing prices in New Zealand for the period from 1999 to 2009. Contrary to Parrikar (Citation2019), findings of the Shi et al. (Citation2014) study suggested a positive relationship between interest rate and housing prices. In other words, an increase in mortgage interest rates causes housing prices to increase. Although these three discussed studies suggested both positive and negative relationships between interest rate and housing prices, the study of Wang and Jiang (Citation2016) found an insignificant relationship between interest rate and housing prices in Shanghai.
Baharuddin et al. (Citation2019) analysed the effect of macroeconomic variables on housing prices in Malesia for a period of 14 years starting from 2005 to 2019. The study findings revealed that both inflation and interest rate negatively influence housing prices in Malesia. The Baharuddin et al. (Citation2019) results oppose those of Mariadas et al. (Citation2016) suggesting that the housing price in Malesia was not necessary to influence by the inflation rate.
Although there might be few studies providing a direct effect of the rental price of the housing market, the study of Liu and Chang (Citation2021) indicated that high rent prices reduce households’ consumption and savings. From this point of view one can conclude that if households are facing savings challenges, it will be difficult for them to buy new housing especially when the interest rate is also high. Kamal et al. (Citation2016) argue that the effect of rent price on the housing market remains indispensable. People will be willing to rent if the monthly rent is lower than the monthly instalment for their own houses, otherwise, they prefer to buy their own houses. Kamal et al. (Citation2016) argue also that investors will be willing to buy houses for rental if the money obtained from renting can generate significant profit to their investment. Nonetheless, the study of Cui et al. (Citation2018) suggested that the linkage between rent price and housing price depend on the usefulness of the house. The high price of the business house may lead to high rental payment whilst an increase in price for a residential house does not necessarily mean growth in the rental price.
In addition to the macroeconomic variables discussed above, political risk is another variable that influences housing prices. A study was conducted by Aha et al. (Citation2018) in the United Kingdom to investigate whether changes in political regimes affect housing prices. Findings indicated that any changes that occur in the country’s political influence price levels in housing markets. Additionally, studies by Monfared and Pavlov (Citation2019) and Muzindutsi et al. (Citation2021) found political risk as one of the major factors that influence housing prices and real estate markets. The study of He et al. (Citation2020) also found that an increase in political uncertainties causes housing and properties price to decline.
3. The datasets and research approach
3.1. Variable datasets
The study was conducted on monthly time series data from January 2002 to December 2019. That dataset consists of macroeconomic variables namely housing prices or demand, rental rate, interest rate and political risk in South Africa. This data was acquired from EasyData-Quantec. Before empirical estimation and interpretation, each data variable was transformed into a natural logarithm. Housing price was employed as a dependent variable while renting price, interest rate and political risk represent independent variables. The sample size and the aforementioned macroeconomic variables were selected based on their impact on housing prices and data availability.
3.2. Research approach
The study utilised the Vector Autoregression (VAR) approach and the following is the sequence followed: initially the Augmented Dickey-Fuller (ADF) unit root test was performed to determine the stationarity level of variables. Secondary the optimal lag length for the VAR model was established through the final prediction error criterion (FPE), Akaike information criterion (AIC), Schwarz information criterion (SIC) and Hannan-Quinn information criterion (HQIC). Thirdly, the roots of characteristics polynomial were estimated to ensure the stability of the selected VAR model. Fourthly, the study proceeds with the Johansen test for cointegration to determine if variables under consideration have a long-run relationship. The existence of a long-run relationship provides the basis for vector error correction model estimation. Subsequently, proceeds with residual tests followed by the Granger causality test. The choice for the aforementioned approaches was stimulated the data sample and the unit root results.
3.3. Model specification
To establish the relationship that exists between housing price and selected macroeconomic variables in South Africa, the vector autoregressive model (VAR) was employed as discussed in the previous section. The following is the VAR model estimated for this study:
Where: LHPI denotes the log of housing price index; is the natural log of inflation; LREN denotes the log of renting price index; LINR denotes the log of interest rate; LPLR denotes the log of political risk, t denotes the sample period;
,
,
and
represent coefficients to be estimated;
,
,
,
denote the intercepts; n denotes the number of lags included in the model while
,
,
,
denote the error terms.
Before the cointegration test, it is imperative to perform a unit root or stability test to ensure that series under consideration are stable to avoid spurious results (Habanabakize & Muzindutsi, Citation2015). The current study employed the Augmented Dickey-Fuller (ADF) unit root test to determine variables integration order. The VAR model expressed in Equations 1–4 is estimated if variables are stationary after the first difference I(1). However, if the ADF test result indicates that variables are not stationary, then the cointegration is estimated to assess if a linear combination of those nonstationary variables is stationary (C.A. Ogbokor, Citation2015). In this study, a long-run relationship amongst variables is assessed using the Johansen (1Citation1988 and Citation1991) test for cointegration. In the case of these variables cointegrate, the next step is the estimation of the vector error correction model (VECM). The following are the VECM equations were derived from the VAR model expressed in equations 1–4:
Where: symbolises variables at the first difference;
,
,
and
denotes coefficients of the error correction used to capture the speed of adjustment towards long-run equilibrium; while
,
,
and
represents the error correction terms.
4. Empirical findings and discussion
4.1. Descriptive statistics
Descriptive statistics summary assists in measuring both central tendency and measures of variability of data set for a given study. For the current study the summary of descriptive statistics is presented in Table . The LPR has the lowest mean of 3.9241 while the means for the remaining variables approximately similar with 4.4051 for LHPI, 4.4326 for LCPI, 4.4052 for LINR and 4.4595 for LREN. Both LHPI and LREN have the highest standard deviation from the mean while the LPR records the lowest standard deviation. Three variables namely LCPI, LINR and LREN are negatively skewed while LHPI and LPR are positively skewed. Lastly, the Jarque-Bera test and its probability suggests that the 0.167591 is the only variables with normally distributed data.
Table 1. Descriptive statistics
4.2. Unit root test
To determine whether series (variables) have a unit root or not, the ADF test was applied to the following hypothesis.
Null hypothesis (
): A series has a unit root, I(1).
Alternative hypothesis (
): A series has no unit root, I(0)
The rejection of the null hypothesis implies that variables are stationary at level, I(0). If the null hypothesis is note rejected, the variable under consideration is tested at the first difference I(1). Results in Table indicate that the null hypotheses were not rejected at a level as the ADF statistics for all variables were smaller than the critical values at a 5 percent level of significance. However, the null hypothesis was rejected at first difference. This implies that all variables are stationary at I(1) and consequently the Johansen cointegration test is appropriate to determine the relationship among variables.
Table 2. ADF unit root results
4.3. Lag length selection
The Johansen test for cointegration is sensitive to the number lag included in the model. The lag selection length selection for the VAR model was performed before the cointegration test to ensure sound results. The lag length results in Table indicated that six lags were appropriate for the model as suggested by LR, FPE and AIC. These six lags were included in the model to estimate the cointegration test whose results are reported in Table .
Table 3. Lag length selection
Table 4. Roots of characteristic polynomial
4.4. Test for stability
To establish sound and accurate results, it is necessary to ensure that the model employed by the study is stable. The VAR model is stable if the value of each root of Characteristic Polynomial has a modulus of less than one (C. A. Ogbokor, Citation2016). Looking at the result in Table , none of the moduli for the employed VAR system is equal or greater than one or outside of the unit circle. Thus, the employed model meets the stability requirement.
4.5. Cointegration analysis
The outcome from the Johansen test for cointegration in Table , both Trace and Eigenvalues indicates the existence of 1 cointegrating equation. In other words, a long-run relationship exists between housing price, interest rate, rental price and political risk. Consequently, it is necessary to estimate the long-run equation and the error correction model.
Table 5. Johansen cointegration results
Cointegration coefficients are represented in Equation 9. Both consumer price index (inflation) and interest rate have a positive sign while rent and political risk and rental price poses a negative sign. In other words, a one percent increase in the inflation rate causes housing price to increase by about 9.02 percent and a one percent increase in the interest rate causes housing prices to increases by approximately 0.95 percent. These results confirm the findings of Baharuddin et al. (Citation2019), Parrikar (Citation2019), and Shi et al. (Citation2014) suggesting that a linear positive relationship exists between inflation, interest rate and housing prices. Nonetheless, this study findings (taking into account the effects of interest rate and inflation rate on housing prices) oppose those of Julius (Citation2012) and Parrikar (Citation2019) suggesting an inverse relationship between interest rate inflation rate and housing prices. Empirical studies on the relationship between interest rate, inflation rate and housing prices generate controversial results, it can be concluded that the effect of both interest rate and inflation rate on housing prices depends on the individual housing market and within a specific region or country.
Contrary to interest rate and an inflation rate that is positively associated with housing prices, the study found that positive changes in political risk and rental price cause a decline in housing prices. Thus, a one percent increase in the political risk index causes housing prices to decrease by 2. 9996LPR while a one percent increase in rental price results in a 9.6384 decline in housing prices. These results may imply that when the political situation improves economic condition improves and people are willing to build their own houses that by and rent as building a house is cheaper than buying and if the rental price is higher or equal to monthly instalment, it better to own a house than to rent.
4.6. Short-run dynamics
The presence of long-run relationships provides evidence to establish a vector error correction model (VECM). This assist in deterring short-run adjustments towards long-run equilibrium. The result of VECM in Table indicates the coefficients of LPR is the only one with negative sign statistically significant at 5 percent level. This implies that the political risk explains the model adjustment to long-run equilibrium after short-run shocks. The error correction term (ECT) of 0.024273 infers that about 2.43 percent of short term shocks are adjusted each quarter. Therefore, changes within independents variables take approximately 41.4 (1/0.024273) quarters to influence housing prices. This result is in line with Muzindutsi et al. (Citation2021) findings suggesting that the South African housing market is very sensitive to political risk.
Table 6. Error correction model
The robustness of the findings was validated through diagnostic checks. Table displays the summary of performed tests. The residuals are normally distributed, with no heteroscedasticity nor serial correlation in residuals. Additionally, as indicated in Table , all AR characteristic polynomial roots have a modulus of less than one. Therefore, the study VECM meets the essential econometric assumptions.
Table 7. VECM diagnostic results
4.7. Pairwise granger-causality test
Test for cointegration test only determines the presence or absence of a long-run run relationship among variables and yet does not provide the causality and its direction between variables. However, when two or more variables cointegrate, there is evidence of at least a unidirectional causality between those variables (Engle & Granger, Citation1987). Results in Table suggest a causality between all independent variables and the dependent variables. While a unidirectional or one-way causality exists between housing price and both interest rate and political risk, bidirectional causation exists between housing price and inflation, housing price and interest rate; and housing and rental price. These results confirm that a short-run relationship exists between exploratory variables and dependent variables.
Table 8. Pairwise granger causality test
5. Conclusion
Using a multivariate approach, the study attempted to determine the relationship between consumer price index or inflation, interest rate, rental price and housing prices in South Africa between January 2002 and December 2019. The Johansen test for cointegration and autoregressive model were employed to determine both long-run and short-run relationships among selected macroeconomic variables. Findings suggested the presence of a long-run relationship among variables under consideration. While inflation and interest rate lead to high prices, rental prices and political risk were found to cause a decline in housing prices. Additionally, all independent variables except for political risk were found to cause changes in housing prices.
The study findings imply that shocks in levels of inflation, interest rate, rental price and political risk substantially affect the housing market and house prices. It is, therefore, important for fiscal and monetary policymakers to carefully manage inflation rates and interest rates to maintain stability in housing markets. Additionally, government and political leaders should strive for political stability as the latter influence positively or otherwise the housing prices. Since this study analysed the effect of macroeconomic and political risk on housing prices, further studies should consider the impact of other components of country risk, namely economic risk and financial risk, on housing prices in South Africa.
Disclosure statement
No potential conflict of interest was reported by the author(s).
Additional information
Funding
Notes on contributors
Zandri Dickason
Prof Zandri Dickason-Koekemoer specialises in financial risk management has obtained her PhD degree in this field. She researches various areas of Risk Management where her main focus area is on financial risk tolerance, depositor behaviour, investor behaviour, behavioural finance, and the financial well-being of investors. This researcher has already published several articles in accredited journals regarding this field of interest. Dr Thomas Habanabakize is an economist specialist. He is currently doing a postdoctoral research fellowship at North-West University, South Africa. His research interests include the intersection between microeconomics, macroeconomics, economic development, labour economics, time series modelling, econometrics and financial economics.
References
- Aha, B., Higgins, D., & Lee, T. (2018). UK political cycle and the effect on national house prices: An exploratory study. European Real Estate Society 25th Annual Conference, 27-30 June.
- Ahmed, D. E. E. (2020). Impact of macroeconomic variables on housing prices in Saudi Arabia: A VAR approach. Preprints. 0715.v1. https://doi.org/10.20944/preprints202012.
- Baharuddin, N. S., Isa, I. N. M., & Zahari, A. S. M. (2019). Housing price in Malaysia: The impact of macroeconomic indicators. Journal of Global Business and Social Entrepreneurship (GBSE), 5(16), 69–11.
- Balcilar, M., Gupta, R., & Shah, Z. B. (2011). An in-sample and out-of-sample empirical investigation of the nonlinearity in house prices of South Africa. Economic Modelling, 28(3), 891–899. https://doi.org/10.1016/j.econmod.2010.11.005
- Barrell, R., & Davis, E. P. (2008). The evolution of the financial crisis of 2007—8. National Institute Economic Review, 206, 5–14. https://doi.org/10.1177/0027950108099838
- Burger, P., & Van Rensburg, L. J. (2008). Metropolitan house prices in South Africa: Do they converge? South African Journal of Economics, 76(2), 291–297. https://doi.org/10.1111/j.1813-6982.2008.00190.x
- Centre for Affordable Housing Finance in Africa (2021). South Africa housing market report – 2020. https://housingfinanceafrica.org/app/uploads/2021/03/Citymark-National-Property-Market-Report-3-March-2021-FINAL.pdf Date of access 17 September 2021.
- Cohen, V., & Karpaviciute, L. (2017). The analysis of the determinants of housing prices. Independent Journal of Management & Production, 8(1), 49–63. https://doi.org/10.14807/ijmp.v8i1.521
- Crowe, C., Dell’Ariccia, G., Igan, D., & Rabanal, P. (2013). How to deal with real estate booms: Lessons from country experiences. Journal of Financial Stability, 9(3), 300–319. https://doi.org/10.1016/j.jfs.2013.05.003
- Cui, N., Gu, H., Shen, T., & Feng, C. (2018). The impact of micro-level influencing factors on home value: A housing price-rent comparison. Sustainability, 10(12), 1–23. https://doi.org/10.3390/su10124343
- Engle, R. F., & Granger, C. W. (1987). Co-integration and error correction: Representation, estimation, and testing. Econometrica: Journal of the Econometric Society, 55(2), 251–276. https://doi.org/10.2307/1913236
- Gil-Alana, L., Ave, G., & Gupta, R. (2013). Testing for persistence in South African house prices. Journal of Real Estate Literature, 21(2), 293–314. https://doi.org/10.1080/10835547.2013.12090367
- Habanabakize, T., & Muzindutsi, P. F. (2015). Time series analysis of interaction between aggregate expenditure and job creation in South Africa. Journal of Governance and Regulation, 4(4), 649–657. https://doi.org/10.22495/jgr_v4_i4_c5_p11
- He, Z., Hu, M., Wang, Z., & Yao, V. (2020). Political uncertainty and asset valuation: Housing prices in Hong Kong. University of Chicago, Becker Friedman Institute for Economics Working Paper, (2020-105).
- Johansen, S. (1988). Statistical anlysis of cointegration vestors. Journal of Economic Dynamics and Control, 12(6), 231–254.
- Johansen, S. 1991. Estimation and hypothesis testing of cointegration vestors in Gaussian vector autoregressive models. Econometrica, 59(6), 1551–1580.
- Julius, S. M. R., 2012. Determinants of residential real estate prices in Nairobi: A research project submitted to the school of business in partial fulfilment of the award of degree in master of science finance university of Nairobi. http://erepository.uonbi.ac.ke/bitstream/handle/11295/12835.
- Kamal, E. M., Hassan, H., & Osmadi, A. (2016). Factors influencing the housing price: Developers’ perspective. International Journal of Humanities and Social Sciences, 10(5), 1676–1682.
- Liu, G., & Chang, X. (2021). The impact of rising housing rent on residents’ consumption and its underlying mechanism: Empirical evidence from China. SAGE Open, 11(2), 21582440211015709.
- Luüs, C. (2005). The ABSA residential property market database for South Africa – Key data trends and implications. BIS Papers, (21), 12.
- Mariadas, P. A., Selvanathan, M., & Hong, T. K. (2016). A study on housing price in Klang Valley, Malaysia. International Business Research, 9(12), 103–109. https://doi.org/10.5539/ibr.v9n12p103
- Miller, N. G., Sklarz, M., & Thibodeau, T. G. (2005). The impact of interest rates and employment on nominal housing prices. International Real Estate Review, 8(1), 27–43. https://doi.org/10.53383/100059
- Monfared, S., & Pavlov, A. (2019). Political risk affects real estate markets. The Journal of Real Estate Finance and Economics, 58(1), 1–20. https://doi.org/10.1007/s11146-017-9619-y
- Muzindutsi, P.-F., Jamile, S., Zibani, N., & Obalade, A. A. (2021). The effects of political, economic and financial components of country risk on housing prices in South Africa. International Journal of Housing Markets and Analysis, 14(3), 523–537. https://doi.org/10.1108/IJHMA-05-2020-0060
- Ogbokor, C. A. (2015). No miracles here: Trade and economic progress. British Journal of Economics, Finance and Management Sciences, 10(2), 167–182.
- Ogbokor, C. A. (2016). Reassessing the export-led growth relationship through cointegration methods. International Journal of Economics and Finance Studies, 8(1), 192–208.
- Orren, T. 2003. House prices and interest rate. https://www.pc.gov.au/inquiries/completed/first-home-ownership/submissions/69/sub069.pdf. Date of access: 10 September 2021
- Parrikar, J. G. P. (2019). The influence of macroeconomic factors on housing prices in India: An empirical study. Indian Journal of Economics and Development, 7(3), 1–7.
- Shi, S., Jou, J. B., & Tripe, D. (2014). Can interest rates really control house prices? Effectiveness and implications for macroprudential policy. Journal of Banking & Finance, 47, 15–28. https://doi.org/10.1016/j.jbankfin.2014.06.012
- Tita, A. F., & Opperman, P. (2021). Understanding the behaviour of house prices and household income per capita in South Africa: Application of the asymmetric autoregressive distributed lag model. Journal of Housing Markets and Analysis, 14(4), 1–21.
- Torres-Reyna, O. 2013. Time series: Data and statistical services. Princeton University. http://www.princeton.edu/~otorres/TS101. Date of access: 15 Jul. 2021
- Wang, Y., & Jiang, Y. (2016). An empirical analysis of factors affecting the housing price in Shanghai. Asian Journal of Economic Modelling, 4(2), 104–111. https://doi.org/10.18488/journal.8/2016.4.2/8.2.104.111