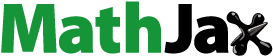
Abstract
This paper examines the direct and spillover effects of exchange rate on foreign direct investment (FDI) inflows in a panel of ASEAN countries from 2001 to 2018. We utilize the spatial econometric approach to accommodate the nature of spatial dependence among ASEAN countries. Our results suggest that the effect of the exchange rate depends on the source-region of FDI, implying the existence of spatial heterogeneity in ASEAN’s FDI. We also show that FDI inflows in ASEAN are not only influenced by the exchange rate of the country itself but also by those of the neighboring countries.
PUBLIC INTEREST STATEMENT
This paper investigates the direct and indirect effect of the exchange rate on foreign direct investment inflow. The data used for this research encompass the economies of ASEAN. The investigation of the relationship between exchange rate and foreign direct investment is carried out in a spatial context. The findings suggest that the effect of the exchange rate depends on the source-region of foreign direct investment. We also show that foreign direct investment inflows in ASEAN are not only influenced by the exchange rate of the country itself but also by those of the neighboring countries.
1. Background
The growing globalization of ASEAN economies has caused FDI to relate to the exchange rate movement strongly. It can be seen through which, for instance, the aftermath of the global financial crisis (GFC), which triggered the Federal Reserves to conduct Unprecedented Monetary Policy (UMP) that led to highly appreciated exchange rates and massive capital inflows in emerging market economies (Bhattarai et al., Citation2018). Under such challenging circumstances, ASEAN economies thus can successfully maintain approximately 2% economic growth in 2009, while the global economic growth had slowed down drastically (Word Bank, Citation2019).
There is, however, still much ongoing debate concerning the impact of changes in exchange rates on the flow of FDI. At least four hypotheses focus on the effect of the real exchange rate on FDI (Kosteletou & Liargovas, Citation2000). The monetary approach, the balance of payments, and the strategic behavior of international firms have demonstrated that the appreciation in the real exchange rate leads to a current account deficit and induces foreign capital inflows (Boateng et al., Citation2015; Campa, Citation1993; Corbo, Citation1985; Cushman, Citation1985; Darby et al., Citation1999; Ffrench-Davis, Citation1983; Kogut & Chang, Citation1996). On the contrary, the imperfect-capital-markets theory and the relative-labor-cost theory predict that a real exchange rate depreciation leads to an increase in inward FDI and vice-versa (i.e., Blonigen, Citation1997; Froot & Stein, Citation1991; Guo & Trivedi, Citation2002; Kiyota & Urata, Citation2004; Klein & Rosengren, Citation1994). On the other hand, de Castro et al. (Citation2013) and Felipe and Llamosas-rosas (Citation2018) had different perspectives where they found no relationship between exchange rates and direct investments for foreign investors in Brazil and Mexican states. Hence, it is crucial to ascertain the relationship of exchange rate movements on FDI inflows as they may have implications for central bank intervention and the forces driving markets (Keleijan & Prucha, Citation2010).
Given the above premise, this paper examines the direct and spillover effects of the exchange rates of neighboring countries on foreign direct investment (FDI) inflows among the ASEAN countries. Besides looking at the effects of exchange rates on ASEAN’s FDI, we also incorporate a spatial analysis to address the regional factors embedded among ASEAN countries. Fujita et al. (Citation1999) suggested that economic integration and cross-border investment would create an agglomeration effect; hence, an analysis of the spatial distribution of neighboring countries is essential as these countries cannot be taken as being homogeneous. This is especially in light of existing bilateral and multilateral agreements that have been established, both with inter- and intra-countries in the ASEAN region, making the geographical interconnections between ASEAN countries even more relevant and inevitable. Therefore, a comprehensive investigation on the spatial framework is required to specify the role of exchange rate movements on ASEAN’s FDI.
Most existing empirical studies on FDI in the ASEAN region frequently utilize a traditional panel and gravity model to explain the determinants of FDI by considering the economic sizes of the countries of origin and destinations and the distances between (e.g., see, Blattner, Citation2006; Eichengreen & Tong, Citation2007; Hattari et al., Citation2014; Hoang, Citation2012; Hoang & Bui, Citation2015; ; Ismail, Citation2009; Masron & Abdullah, Citation2010; Thangavelu & Narjoko, Citation2014). Unfortunately, the traditional panel and gravity model cannot capture the interrelationships or interdependence between countries, especially where the effect of spatial interaction between countries within a particular region is evident.
The spatial econometric model can capture and explain the behavior of FDI influenced by third countries if there is a connection. FDIs are spatially dependent, as portrayed by the relationship between possible forms of FDI on the spatial lag coefficient of FDI and the investment potential of neighboring countries. There are four forms of FDI outlined in the literature: horizontal FDI, vertical FDI, export-platform FDI, and complex vertical FDI ().
Table 1. Predicted signs of the theoretical model explanatory
There is voluminous literature that has examined the determinants of FDI using spatial econometric models, such as Baltagi et al. (Citation2007), Blonigen et al. (Citation2007), van der Ploeg & Poelhekke (Citation2009), Uttama and Peridy (Citation2009), Nwaogu (Citation2012), and Regelink and Paul Elhorst (Citation2015) for the US outward FDI, Garretsen and Peeters (Citation2009) for Dutch outward FDI, Ledyaeva (Citation2009) for Russian inward FDI, Chou et al. (Citation2011) for Chinese outward FDI, Marouane et al. (Citation2019) for MENA Region inward FDI, Fischer et al. (Citation2017) for Austrian outward FDI, Felipe and Llamosas-rosas (Citation2018) for Mexican states’ inward FDI. In the case of ASEAN, Uttama and Peridy (Citation2009) examined FDI originating from the United States (extra-ASEAN), while Hoang and Goujon (Citation2019) investigated the intra-ASEAN and extra-ASEAN FDI using the Spatial Autoregressive Model (SAR) and Spatial Error Model (SEM) respectively.
Given their proven proficiency, this paper uses spatial econometrics to address the issues related to inter- and intra-relationships for FDI and thus follows the works of Hoang and Goujon (Citation2019) and Uttama and Peridy (Citation2009). These research analyses have investigated the determinants for ASEAN inward FDI using spatial econometrics. However, they have not formally addressed the role of the exchange rate and its spatial dependence effect in determining FDI inflows among ASEAN countries. On the other hand, they only used the SAR and SEM, which allow only spatial lag and error analysis of neighboring countries.
In this paper, we incorporated the Spatial Durbin Model (SDM), which, unlike the SAR and SEM, accommodates spatial lag analysis and the spatial effect for independent variables. This model allows us to make empirical investigations on the spatial effect of the exchange rate and simultaneously enables us to study the path of FDI inflows into ASEAN countries. By using SDM, we also could prevent the cost of ignoring spatial dependence in the dependent variable and the independent variables, providing more efficient estimations and unbiased coefficients (Elhorst, Citation2010; J. LeSage & Pace, Citation2009)
By exploiting a dataset based on a panel of ASEAN countries from 2001 to 2018, our empirical investigations have unveiled several crucial findings: First, we find the existence of spatial heterogeneity in FDI form in ASEAN, which is shown by the effect of the exchange rate and macroeconomic variables on foreign direct investment inflows that are varied among different forms of FDI based on the source countries. Second, we find that FDI inflows in ASEAN are influenced by the country’s macroeconomic factors and the spatial effect channel transmitted from a third-country to the particular ASEAN country. Third, we find that the results become susceptible when the structure of matrix W is changed. By implementing the inverse distance matrix, the “Export-platform FDI hypothesis” is established. However, when the spatial weight matrix is the first-order binary contiguity, it becomes quite challenging to prove the existence of the geographical interdependence of FDI inflows, which then leads to the “Pure horizontal FDI hypothesis.”
This paper is organized as follows: Section II discusses the literature that serves as the foundation of this study; section III discusses methodological aspects, including data, variables, theoretical insight, and econometric specification; section III discusses the estimation results; the last section provides the concluding remarks and policy implications.
2. Literature
Two theories are most widely used in linking the effect of exchange rates on FDI, namely Blonigen (Citation1997) and Froot and Stein (Citation1991).
Using an imperfect capital market approach, Froot and Stein (Citation1991) argue that if the exchange rate affects FDI through the wealth channel, it is assumed that external sources of borrowing are more expensive than the company’s internal cost of capital. Thus, currency depreciation will have a positive effect on inbound FDI, as it increases the wealth of foreigners and allows them to make higher bids on assets.
Meanwhile, Blonigen (Citation1997) focuses more on company acquisitions through FDI, assuming market segmentation of goods. He argues that foreign and domestic companies have the same opportunity to buy, but different opportunities to generate asset returns in foreign markets, where the profitability of the branch of a multinational company (MNE) can be increased after acquiring a foreign company, so as to provide company-specific assets. Therefore, currency movements can have an impact on relative asset valuations and a depreciation of the host currency can increase FDI.
A large body of literature has investigated FDI determinants, especially in developing and emerging countries. These studies have identified several country-specific-macroeconomic and also international factors that affect the flow of capital across countries.
Tille and van Wincoop (Citation2010) developed the implications of portfolio choice for net and gross international capital flows in a simple two-country setting using the dynamic stochastic general equilibrium model. They focused on the time-variation in portfolio allocation following shocks and the resulting flows. Their research finds that the endogenous time variation in expected return is the key determinant of capital flows.
De Vita and Kyaw (Citation2008) investigated the relative significance of the determinants of FDI and portfolio flows to developing countries using a sample of five countries from 1976 to 2001 and by employing the structural vector autoregression method. Their research emphasizes the importance of real economic variables, such as output in source and destination country as the most important forces explaining capital flows to developing countries.
In line with De Vita and Kyaw (Citation2008), several other studies have also emphasized the importance of links between countries when studying capital flows. With a focus on emerging economies, Vo (Citation2018) examined the determinants of capital flows by analyzing the flow of FDI into Vietnam by employing a fixed effect panel and dynamic GMM estimator. His research is focused on country characteristics and the bilateral relationship between the source and destination country. His findings highlight the importance of inflationary risk, bilateral trade links, and stock market volatility.
Similarly, by using a dataset from emerging economies, Byrne and Fiess (Citation2016) considered global and national determinants of capital flows. Using panel data estimation, his research found commodity prices, U.S. rates of return, uncertainty, and growth in advanced economies as the global determinants of capital flows. Financial openness and institutions’ quality are the key national factors affecting capital flows.
3. Empirical strategy
3.1. Data sources
This paper analyzes the impact of the exchange rate and macroeconomic variables on FDI inflows in ASEAN, focusing on the spatial dependence of ASEAN foreign direct investment (FDI) inflows and the spatial heterogeneity in FDI forms between 2001 and 2018. The period is selected based on data availability and to avoid significant shocks to the economies caused by the Asian Financial Crisis (which lasted to 2000) and the COVID-19 Pandemic (which started in late 2019). The data includes the 10 ASEAN countries: Brunei Darussalam, Cambodia, Indonesia, Lao PDR, Malaysia, Myanmar, Philippines, Singapore, Thailand, and Vietnam. The Association of Southeast Asian Nations (ASEAN) is composed of 10 economies. Together, the 10 economies of ASEAN account for the fifth-largest GDP in the world in 2019. Population-wise, ASEAN accounts for around 9% of the world population (ASEAN Secretariat, Citation2019). Most of the ASEAN member states are developing economies that value FDI as an important source of growth (Abbes et al., Citation2015). Thus, it is essential for these economies to understand the behavior of FDI.
Based on our data source, ASEANstats, the flows of Inward Foreign Direct Investment (FDI) into ASEAN by Source Country are divided into FDI that enters ASEAN from the world/global, from inside ASEAN itself (intra-ASEAN), and from outside ASEAN (extra-ASEAN).
The FDI (the dependent variable) data are presented as the ratio of the respective country’s GDP (both FDI and GDP are in constant 2010 price). This is to control for the country size and to better represent the focus of this research: The inflow of FDI to economies. The data for other variables are in the logarithmic form. Thus, say the coefficient of an independent variable i, can be interpreted as
increase in the FDI to GDP ratio if variable i increases by 1%.
For the exchange rate, we use the official exchange rate (LCU per the US $, period average)Footnote1 (Hattari et al., Citation2014; Hoang, Citation2012; Hoang & Bui, Citation2015; Marouane et al., Citation2019). We use the real gross domestic product (2010 constant) to measure the market size (Felipe & Llamosas-rosas, Citation2018; Garretsen & Peeters, Citation2009; Hoang & Goujon, Citation2019; Marouane et al., Citation2019; Nwaogu, Citation2012). The consumer price index represents inflation (Boateng et al., Citation2015; Hoang & Bui, Citation2015; Hoang & Goujon, Citation2019; Ismail, Citation2009). We also employ the indicator of Political Stability and Absence of Violence/Terrorism to represent political risk since it is crucial to ascertain the quality of governance (Garretsen & Peeters, Citation2009; Hoang & Goujon, Citation2019; Marouane et al., Citation2019; Nwaogu, Citation2012). We use total export and import (as a percent of GDP) to denote trade openness (e.g., Boateng et al., Citation2015; Chou et al., Citation2011; Garretsen & Peeters, Citation2009; Ismail, Citation2009; Marouane et al., Citation2019). Lastly, we utilize the total number of airports to represent infrastructural development, which is different from Marouane et al. (Citation2019), Nwaogu (Citation2012), and Hoang and Goujon (Citation2019), who use telephone lines as a proxy. Our rationale for this is that technological development will render telephone lines obsolete, and their usage will trend downwards. The airport is one of the infrastructures in the transportation of goods considered by investors’ costs. The exchange rate, market size, and inflation rate are calculated as natural logarithms. The data sources for the exchange rate, market size, political risk, inflation, trade openness are World Bank and IMF. Also, infrastructural development data from ASEAN-JAPAN Transport Partnership. presents the descriptive statistics of the variables.
Table 2. Descriptive statistics
3.2. Spatial econometric model
In this paper, we follow the spatial econometrics model of FDI inflows, developed by Uttama and Peridy (Citation2009) and Hoang and Goujon (Citation2019) to capture the third-country effects of the exchange rate and macroeconomic variables on FDI inflows in the ASEAN region. Our model estimate is thus expressed in the following equation:
Where denotes the direct coefficient of the independent variable, which is indicative of the impact exerted by the macroeconomic variable of the host country on the FDI inflows of the host country (dependent variable).
denotes the spatial exogenous variable coefficient, which indicates the impact exerted by the macroeconomic variable in the neighboring country on the FDI inflows of the host country.
denotes the spatial autoregressive coefficient, which indicates the degree of FDI spatial dependence.
is vector
error-term for the host country
at t-time.
acts as a spatial coefficient of autocorrelation to calculate the impact of FDI shocks from the neighboring country
on the host country
.
stands for the time-period fixed effect,
refers to the spatial unit fixed effect. Meanwhile,
denotes a random error vector, satisfying
.
3.3. Robustness checks
To ensure consistent results, we conduct robustness tests as follows: First, we divide the spatial econometric models for three different interaction effects: (i) Spatial Autoregressive (SAR) Model, which contains only the spatial lag term of the dependent variable, where θ = 0 and λ = 0; (ii) Spatial Error Model (SEM) which contains only the spatial lag term of the error term, where ρ = 0 and θ = 0; and, (iii) Spatial Durbin Model (SDM) which contains both the dependent variable and independent variable spatial lag term, where λ = 0. Second, we propose two weighting methods by adopting alternative spatial weight matrices. Following Peng et al. (Citation2018) and Feng et al. (Citation2019), we construct and employ the general spatial weight matrices based on the geographical correlation, which includes the inverse distance (W1) and the first-order binary contiguity (W2). Specifically, these two spatial weight matrices are constructed as follows:
where denotes the great circle distance between country i and country j. We adopt the normalization procedure of the row-normalized spatial weight matrix of Elhorst (Citation2010), Kelejian & Prucha (Citation2010) to ensure that the rows sum up to 1 and their diagonal elements are set to 0.
4. Results and discussion
4.1. An empirical analysis for the whole sample (FDI Global)
We begin the investigation by estimating the non-spatial panel data based on two spatial weight matrices to select the optimal model for our analysis. First, following Anselin et al. (Citation1996), we use several LM test procedures to examine whether a spatially lagged dependent variable (LM spatial lag) or a spatially autocorrelated error term (LM spatial error) should be included in the model. We then use the LR test to evaluate whether the model has a spatial fixed effect and time fixed effect. From , the LR test of FDI Global result of the spatial fixed effect is significant at the 1% level but not significant for the time fixed effect for all spatial weight matrices, indicating that the model contains only spatial fixed effects. In light of this, LM statistics should be calculated based on the spatial fixed effects model. The estimations of SAR and SEM, indicated by the LM-lag, LM-error and Robust LM-error statistics are significant below 10% levels for W1 and the LM-error, Robust LM-lag and Robust LM-error statistics are significant below 5% levels for W2.
Table 3. Coefficient estimation and LM test of the non-spatial panel model
To select the best data-fit model, we look at alternative approaches, including the spatial Durbin model (SDM) to minimize endogeneity problems that would arise due to omitted variables bias in spatial regressions containing endogenous and exogenous spatial lags (Fingleton and Le Gallo, Citation2010; J.P. LeSage & Pace, Citation2008; Lacombe & Lesage, Citation2015). The Wald or likelihood ratio (LR) test is used to judge whether the SDM can be simplified into the SAR or SEM. The results show that the two effects are significant at 1% levels, indicating we should adopt the SDM rather than SAR or SEM.
Second, we consider the spatial Durbin model specification for the Global FDI reported in . We find that the direct coefficients of the exchange rate (XR) when the spatial weight matrix changes from W1 to W2 are significantly negative at the 10% significance level and 1% significance level, respectively. This result is consistent with Paun et al. (Citation2013) that shows evidence of the presuppositions of the monetary approach of the balance of payments in the emerging market, that is, the depreciation in the real foreign exchange rate generates surpluses in the current account (improving the export-to-import ratio) while at the same time, causes an increase in the capital or financial account deficit. Furthermore, the theory of the strategic behavior of international firms demonstrates that an appreciation of the real exchange rate in a country encourages a large current account deficit and thus attracts international firms to invest in these markets (Kosteletou & Liargovas, Citation2000). These findings support similar views of Boateng et al. (Citation2015), Campa (Citation1993), Darby et al. (Citation1999), Kogut and Chang (Citation1996), and Cushman (Citation1985).
Table 4. Estimation results of spatial panel model in the whole sample (FDI Global case)
As with our results for the exchange rate, we find that the direct coefficients of OPENNESS are significantly negative at the 1% significance level for both spatial weight matrices, indicating that a rise in trade openness in the host country significantly decreases foreign direct investments in the host country. This finding confirms the motives for “tariff jumping” that allows the home country investor undertake foreign direct investment (FDI) in that host country to sidestep trade barriers by relocating production to the destination market and creating benefit for the foreign firms (Blonigen et al., Citation2019; Ghodsi, Citation2020). This confirms Garretsen and Peeters (Citation2009) and Marouane et al. (Citation2019) findings, who found that when trade openness in the host country increases, it encourages FDI discharge from the MENA Region and Dutch outbound FDI into 18 OECD economies, respectively. The increase in inflation in the host country also has a significantly negative effect for FDI inflows at the 10% and 1% significance level, respectively, when the spatial weight matrix changes from W1 to W2. This indicates that the instability and uncertainty of macroeconomic factors in the host country might restrain FDI from flowing into the host country as reflected by the inflation rate.
The direct coefficients of market size are significantly positive at the 1% significance level when the spatial weight matrices are W1 and W2. This reveals that the increase in the host country’s gross domestic product (GDP) has facilitated foreign direct investment (FDI) inflows from the world into the ASEAN countries during the research period. Therefore, the positive effect of MARKET on FDI is consistent with Head and Mayer (Citation2004), Nwaogu (Citation2012), and Hoang and Goujon (Citation2019). Political risk, however, shows no significant effect on FDI inflows in the ASEAN region for W1 but is positive at a 10% significance level for W2, albeit the coefficient is relatively low at 10%. This is consistent with the work of Marouane et al. (Citation2019) and Garretsen and Peeters (Citation2009) who argue that government stability in the host country is not a determining factor for foreign investors entering the host country. The direct coefficients of INFRA are significantly positive at the 1% significance level when the spatial weight matrix is W1, while the coefficient of INFRA is not significant when the spatial weight matrix is W2. The positive effect of this coefficient shows that infrastructure development can be a factor in attracting investors to a host country.
Third, we can see from the results of the estimated coefficients for the spatial autoregressive coefficients for Global FDI in Table , that there are differentials for the estimates of the spatial weight matrices using W1 and W2. When using the SAR model, it is complicated to prove the existence of spatial autoregressive coefficients () for both the spatial weight matrices. However, when we include the spatially lagged macroeconomic variables, we find that the spatial autoregressive coefficients are significantly negative at the 1% and 10% significance level, respectively, when the spatial weight matrix changes from W1 to W2. This implies that the negative spillover effect of foreign direct investment inflows in the neighboring or surrounding countries significantly influences foreign direct investment in a particular country (e.g., Garretsen & Peeters, Citation2009; Ledyaeva, Citation2009; Marouane et al., Citation2019; Regelink & Paul Elhorst, Citation2015). This is an indication of a competitive relationship between countries in ASEAN and that there is a substitution interaction effect for foreign direct investment inflows rather than a complementary one. Therefore, if the third country has better prospects than the host country, foreign capital may likely leave the host country and move to the neighboring economies.
Our analysis also finds the coefficient (W * MARKET) positively significant at 1% significance levels when using W1 and W2. This coefficient which represents the market potential of a neighboring country shows the existence of an export-platform FDI relationship among the ASEAN countries. The positive spillover effects from the market potential confirm that an increase in economic activity in neighboring countries causes higher FDI inflows in a particular country when the spatial econometric model takes into consideration the geographical correlation (e.g., Garretsen & Peeters, Citation2009; Hoang & Goujon, Citation2019; Marouane et al., Citation2019; Nwaogu, Citation2012; Regelink & Paul Elhorst, Citation2015). This finding supports the work of Uttama and Peridy (Citation2009) and Hoang and Goujon (Citation2019) for FDI inflows in ASEAN. Our finding is also consistent with that of Ekholm et al. (Citation2007) who demonstrate that when there are free trade agreements among countries, export-platform FDIs are very likely to occur, as in the case of ASEAN where trade agreements between countries are in existence.
Fourth, the spatial lag coefficient of the exchange rate is positive but does not pass the significance test when the spatial weight matrix is W1, whereas it is significantly negative at the 5% significance level when the spatial weight matrix is W2. This indicates that with the spatial econometric model, which takes into consideration state boundaries, an appreciation of the neighboring country’s currency improves the investment expectation arising from the enhancement of future profits in the host country. However, the spatial spillover effects of the exchange rate on FDI inflows in ASEAN are inconsistent when the spatial weight matrix changes. Similarly, the spatial spillover effect of infrastructure development on FDI inflows in ASEAN is also inconsistent when the spatial weight matrix changes. The spatial lag coefficient of INFRA is significantly positive at the 1% significance level when the spatial weight matrix is W1, but weak when the spatial weight matrix is W2, since the negative coefficient of the spatial lag on INFRA is only significant at 10%. This indicates that infrastructure development in the neighboring country will induce inflows of foreign direct investment in a particular country when the spatial weight matrix considers the distance between the two capitals.
The spatial lag coefficient of trade openness is significantly negative at the 1% significance level when the spatial weight matrix is W1 but does not pass the significance test when the spatial weight matrix is W2. The negative spatial spillover effect which is indicative of a reduction in trade barriers, as depicted by an increase in trade openness in neighboring countries, acts to enhance foreign direct investment in the host country. Ekholm et al. (Citation2007) have shown that a country’s export-platform FDI increases when trade costs between potential destination countries are lower relative to the home country and destination country (third-country). In our study, the spillover effect of POL is negative but does not pass the significance test when the spatial weight matrix is W1. However, it is significantly positive at the 10% significance level when the spatial weight matrix is W2. The empirical evidence for political risk in the neighboring country and FDI inflows in the host country, however, is relatively weak since the positive coefficient of the spatial lag for POL is only significant at 10%.
Lastly, for our robustness check, we compare the SDM with SAR and SEM, and the results are quite similar or identical to the SDM. Our empirical results using SAR and SEM show that FDI Global is significantly responded to by the market size, trade openness, inflation when W1 and W2 are used. Moreover, infrastructure development is significantly positive for the SEM model when using W1. For the spatial autoregressive coefficients, the results show a negative sign at a 10% significance level when the spatial weight matrix is W1 and has no significance when the spatial weight matrix changes from W1 to W2.
4.2. FDI by source country comparison analysis
Generally, the characteristics of foreign direct investment originating from intra-ASEAN and extra-ASEAN are largely different, and likewise with the factors that influence the decisions of investors entering the ASEAN region. The impact of the exchange rate and macroeconomic factors on FDI inflows may differ vastly between countries due to these differences. To that end, we need to examine the existence of spatial heterogeneity in FDI. Therefore, the subsamples of the standpoints of the source country’s FDI (i.e., FDI from intra-ASEAN and extra-ASEAN) are utilized to re-estimate the regressions. Specifically, we adopt three model tests (SAR, SEM, and SDM) in order to determine the most suitable model for FDI from intra-ASEAN and extra-ASEAN. Similar to the selection steps for the whole sample, we have chosen the spatial Durbin model to analyze the spatial fixed effect for FDI inflows of the source country, except for FDI inflows from extra-ASEAN which use the spatial random effect when the spatial weight matrix is the inverse distance (W1).
Table 5. Estimation results of spatial panel model in FDI Intra-ASEAN case
Table 6. Estimation results of spatial panel model in FDI Extra-ASEAN case
We find that the estimation results for these two subsamples have some differences from those of the whole sample. Specifically, there are several distinctions in the characteristics of the factors that influence FDI in intra-ASEAN and extra-ASEAN. First, the direct coefficient of the exchange rate in the intra-ASEAN FDI model is positive but does not pass the significance test when the spatial weight matrix is W1, while it is significantly positive at the 10% significance level when the spatial weight matrix is W2. However, this value is relatively weak because it is only significant at 10% while W1 is not significant. The direct coefficients in the intra-ASEAN FDI model, for market size (MARKET) and political risk (POL) are significant and positive at 10% significance levels when the spatial weight matrix is W1 and significantly positive at the 5% significance levels when the spatial weight matrix is W2. The positive effects of POL reflect an increase in the index, indicative of a decrease in political risks that could encourage investors to set up MNEs. This is consistent with Nwaogu (Citation2012) and Hoang and Goujon (Citation2019). The direct coefficients of infrastructure development are significantly positive at the 1% significance level for both spatial weight matrices, showing that infrastructure development and FDI inflows from intra-ASEAN are complementary. Meanwhile, the direct coefficients of trade openness and inflation have no significant effect on FDI inflows from intra-ASEAN for both spatial weight matrices. The insignificant coefficients for infrastructure development and trade openness may be attributable to the substantial reduction in trade barriers between the source countries and host countries since 1993 when the ASEAN Free Trade Area (AFTA) was implemented.
Second, the direct coefficients of FDI for the extra-ASEAN model are quite different from the FDI for the intra-ASEAN model. In summary, the impact from macroeconomic factors in the host country affecting FDI inflows from extra-ASEAN are different from those from intra-ASEAN. While the effect of inflation and the exchange rate in the host country on intra-ASEAN FDI is weak, we find that for the FDI extra-ASEAN model, inflation and exchange rate in the host country have a significant effect. In other words, inflation and the exchange rate are factors of consideration for investors from outside of ASEAN, while investors from within ASEAN do not place much emphasis on them. The direct coefficient of the exchange rate shows no significant effect when the spatial weight matrix is W1 while it has a significantly negative effect on FDI inflows from extra-region at the 1% significance level when the spatial weight matrix changes from W1 to W2. Similarly, the increase in inflation in the host country has a significantly negative effect on FDI inflows at the 1% significance levels for both spatial weight matrices. As for the variables of market size and trade openness in the FDI extra-ASEAN model, they have effects similar to those for the whole sample (FDI global), where the direct coefficients of market size are significantly positive, and trade openness is significantly negative at the 1% significance level when the spatial weight matrices are W1 and W2.
The negative and significant relationship between trade openness and FDI inflows originating from outside ASEAN shows the substitution relationship between trade openness and FDI in the host country. The geographical distance between the home country and countries outside ASEAN or inside ASEAN represents a cost consideration for investors when they export their products. Nwaogu (Citation2012) recommends the establishment of foreign affiliate companies for closer proximity to customers as well as for the reduction of trade costs. Concentrating on production abroad relative to exporting these products would generate fixed cost savings associated with foreign production facilities. Hattari et al. (Citation2014) observe that there is a negative relationship between exports and FDI, suggesting a substitution effect between trade and FDI, which is similar to our finding. Surprisingly, the direct coefficients for infrastructure development show unexpected negative signs at 1% and 5% significance levels when the spatial weight matrix changes from W1 to W2, which are in contrast to our intra-region FDI model. Similar to the negative effects of trade openness, infrastructure development also seems to have a substitution relationship with FDI inflows originating from extra-ASEAN, indicating that an increase in airport development in the host country facilitates the delivery of raw materials by MNEs to the host country as against the establishment of a company in the host country itself. This suggests that airport development can reduce FDI inflows from outside the ASEAN region as foreign investors could potentially save costs by just exporting their products instead of setting up resource-heavy foreign production facilities. Meanwhile, the direct coefficient of political risk is only positive and significant when using W1 as the spatial weight matrix, suggesting that the more stable the political situation in the host country, the more foreign investors from outside ASEAN will be encouraged to invest in ASEAN.
Third, we prove that the “export-platform FDI hypothesis” occurs in the FDI inflows originating from intra-ASEAN and extra-ASEAN when using W1 as the spatial weight matrix. We find that the spatial autoregressive coefficients of FDI intra-ASEAN and extra-ASEAN are significantly negative at 5% and 1% significance levels while market potential coefficients are significantly positive at 5% and 1% significance levels for W1, which are in line with the full sample results. However, when the spatial weight matrix changes from W1 to W2, the “pure horizontal FDI hypothesis” occurs as the spatial autoregressive coefficient () is not significant with a negative sign for FDI source countries, while there are positive spillover effects from the market potential, confirmed at 1% significance level. Therefore, it is complex to determine whether the characteristics of FDI intra-ASEAN and extra-ASEAN are purely horizontal or export-platform FDI by just looking at the geographical interdependence with the use of country boundaries. In pure horizontal FDI, foreign investors establish MNEs only to gain market access in the host country to sidestep trade barriers, while the market size of neighboring countries is not an issue with pure horizontal FDI.
Fourth, the spillover effect using the great circle distance (W1) is more visible compared to country boundaries (W2) as a spatial weight matrix for FDI intra-ASEAN and extra-ASEAN. Possibly, this is because when using country boundaries, countries that do not share the same boundary are considered to have no spatial influence on the host country. In FDI intra-ASEAN, the spatial lag coefficients of the exchange rate and inflation are significantly negative at the 5% and 1% significance levels for both spatial weight matrices. This is indicative of the importance of maintaining stability in the inflation and exchange rate in the neighboring country for attracting FDI inflows from intra-ASEAN into ASEAN economies. The spatial lag coefficient of trade openness in FDI intra-ASEAN is significantly negative at the 5% significance level when the spatial weight matrix is W1 but does not pass the significance test when the spatial weight matrix is W2. This negative result shows that if the third country is a potential destination that has reduced its trade barriers, this will reduce the interest of investors from intra-ASEAN to invest in the host country, which is in line with the FDI export platform hypothesis. Similarly, the spatial spillover effect of infrastructure development on FDI inflows from intra-ASEAN is also inconsistent when the spatial weight matrix changes. The spatial lag coefficient of infrastructure development in FDI intra-ASEAN is significantly positive at the 1% significance level when the spatial weight matrix is W1, but not significant when the spatial weight matrix is W2. For intra-ASEAN FDI, it appears that political stability is not an important factor, as seen from the two insignificant spatial weight matrices for the spillover effects of political risk.
Fifth, continuing with the spillover effects, we look at the geographic interaction factors that affect FDI inflows from extra-ASEAN. For extra-ASEAN FDI, inflation in the third-country appears to be the factor that most influences foreign investors from outside ASEAN, as gleaned from the spatial lag coefficient of inflation that is significant and negative for both spatial weight matrices at 1% significant levels. Furthermore, the development of airports in the third country has a negative effect on the entry of foreign investments from outside ASEAN into a particular country in ASEAN. The spatial lag coefficients of infrastructure development are negative and significant at 5% and 10% significance levels when the spatial weight matrix changes from W1 to W2. Similar to FDI intra-ASEAN, the spatial lag coefficient of trade openness in FDI extra-ASEAN is significantly negative at the 1% significance level when the spatial weight matrix is W1, but fails to pass when it changes to W2. Comparable to FDI intra-ASEAN, political stability for extra-ASEAN FDI is also not an important factor, evidenced by its insignificance when W1 is used as the spatial weight matrix but is significant and positive at the 10% significance level when the spatial weight matrix is W2. The result shows that the spillover effects of the exchange rate do not induce FDI inflows from extra-ASEAN, as reflected by the insignificant spatial lag coefficient of the exchange rate when the spatial weight matrix is W1 and is only significant at the 10% significance level when the spatial weight matrix is W2.
Lastly, similarly, with FDI Global, we perform the robustness test using the SAR and SEM models for FDI intra-ASEAN and extra-ASEAN. In summary, the SDM estimation results for the subsamples of these two foreign direct investments by source countries are not always consistent for the SAR and SEM models. Our empirical results illustrate that neither the SAR model nor the SEM model for FDI intra-ASEAN is significantly determined by trade openness, political risk, and infrastructure development. For FDI extra-ASEAN, it is significantly determined by market size and trade openness, except for political risk which is significant for SAR and inflation which is significant for SEM, for both spatial weight matrices. However, for the SDM model, we find that the market size, political risk, and infrastructure development affect FDI intra-ASEAN while market size, trade openness, inflation, and infrastructure development affect FDI extra-ASEAN when W1 and W2 are used. Moreover, political risk is significantly positive when using W1 while the exchange rate is significantly positive when using W2. The spatial autoregressive coefficients are not significant when the spatial weight matrix changes from W1 to W2 for FDI intra-ASEAN and extra-ASEAN.
5. Concluding remarks
Our empirical investigations have derived several crucial findings. First, we find that the effects of the exchange rate and macroeconomic variables on foreign direct investment inflows are varied for different forms of FDI based on the source countries. This provides robust support for the establishment of spatial heterogeneity in FDI forms in ASEAN. Second, we find that aside from macroeconomic factors of the host country, foreign investors also contemplate the interlinkages among the ASEAN countries in their quest to invest in the most optimal location. Third, we find that the results are very sensitive to the structure of the matrix W. For our cases, this makes it quite challenging to prove the existence of the geographical interdependence of FDI inflows when the first-order binary contiguity is used as the spatial weight matrix. The implementation of the inverse distance matrix establishes the “Export-platform FDI hypothesis,” but when the spatial weight matrix is the first-order binary contiguity, it is quite challenging to prove the existence of the geographical interdependence of FDI inflows, leading to the “Pure horizontal FDI hypothesis”.
For policy practices, our empirical results have several implications. First, the strong competition for FDI inflows among ASEAN countries means that they must maintain or enhance their competitiveness, investment environment, as well as macroeconomic conditions in their respective economies to attract investments as well as to dissuade investors from moving to greener pastures. Second, to attract FDI, countries must look at the economic policies of other countries besides their own. Third, we also find that the positive spillover effect of market potential leads to an increase of FDI inflows into the host country. The focus should thus be given to the development of economic integration among the ASEAN countries. Fourth, the finding of “tariff jumping” motives in our study indicates that authorities must tread carefully when implementing free-market policies or reducing trade barriers as they can lead to declines in FDI inflows, especially from extra-ASEAN regions. It would thus be very useful to probe deeper into the relationship between trade barriers and FDI inflows into ASEAN countries through more in-depth research. Lastly, the effect of infrastructure development on FDI inflows from extra-ASEAN contrast with FDI inflows of the intra-ASEAN model. From our study, infrastructure development is a complementary factor for FDI inflows from intra-ASEAN, while it is a substitute factor for FDI inflows from extra-ASEAN. The policies for infrastructure development should therefore be tailored to specific situations and conditions, taking into account the aspect of regional interdependence.
Acknowledgements
I thank my research assistants, Ayu Citra Muthia and Militcyano Samuel Sapulette, for their help in the making of this paper.
Disclosure statement
No potential conflict of interest was reported by the author(s).
Additional information
Funding
Notes on contributors
Ferry Syarifuddin
Ferry Syarifuddin is a senior macroeconomic and financial system research specialist at Bank Indonesia. His research activities center on issues of international finance, especially in Southeasts Asia and emerging economies; monetary economics; banks and financial institutions; and Islamic finance. This research is one of his several recent research on financial and economic integration in Southeast Asia.
Notes
1. We do not use the nominal/real effective exchange rate due to incomplete data for Brunei Darussalam, Cambodia, Lao PDR, and Myanmar.
2. The value for the political stability index variable ranges from −2.5 (weak) to 2.5 (strong).
References
- Abbes, S. M., Mostéfa, B., Seghir, G., & Zakarya, G. Y. (2015). Causal Interactions between FDI, and economic growth: Evidence from dynamic panel co-integration. Procedia Economics and Finance, 23, 276–20. https://doi.org/10.1016/s2212-5671(15)00541-9
- Anselin, L., Bera, A. K., Florax, R., & Yoon, M. J. (1996). Simple diagnostic tests for spatial dependence. Regional Science and Urban Economics, 26(1), 77–104. https://doi.org/10.1016/0166-0462(95)02111-6
- ASEAN Secretariat. (2019). ASEAN key figures 2019. ASEAN Key Figures.
- Baltagi, B. H., Egger, P., & Pfaffermayr, M. (2007). Estimating models of complex FDI: Are there third-country effects? Journal of Econometrics, 140(1), 260–281. https://doi.org/10.1016/j.jeconom.2006.09.009
- Bhattarai, S., Chatterjee, A., & Park, W. Y. (2018). EFFECTS OF US QUANTITATIVE EASING ON EMERGING MARKET ECONOMIES. ADBI Work. Pap. Ser. https://doi.org/10.1507/endocrj1954.10.202
- Blattner, T. S. (2006). What drives foreign direct investment in Southeast Asia ? A dynamic panel approach. Outlook.
- Blonigen, B. A. (1997). Firm-specific assets and the link between exchange rates and foreign direct investment. Foreign Direct Investment, 87 3 , 447–465. https://doi.org/10.1142/9789813277014_0003
- Blonigen, B. A., Davies, R. B., Waddell, G. R., & Naughton, H. T. (2007). FDI in space: Spatial autoregressive relationships in foreign direct investment. European Economic Review, 51(5), 1303–1325. https://doi.org/10.1016/j.euroecorev.2006.08.006
- Blonigen, B. A., Tomlin, K., & Wilson, W. W. (2019). Tariff-jumping FDI and domestic firms’ profits. Foreign Direct Investment 37 3 , 473–500. https://doi.org/10.1142/9789813277014_0014
- Boateng, A., Hua, X., Nisar, S., & Wu, J. (2015). Examining the determinants of inward FDI: Evidence from Norway. Economic Modelling, 47, 118–127. https://doi.org/10.1016/j.econmod.2015.02.018
- Byrne J P and Fiess N. (2016). International capital flows to emerging markets: National and global determinants. Journal of International Money and Finance, 61 82–100. 10.1016/j.jimonfin.2015.11.005
- Campa, J. M. (1993). Entry by foreign firms in the United States under exchange rate uncertainty. The Review of Economics and Statistics, 75(4), 614–622. https://doi.org/10.2307/2110014
- Chou, K. H., Chen, C. H., & Mai, C. C. (2011). The impact of third-country effects and economic integration on China’s outward FDI. Economic Modelling, 28(5), 2154–2163. https://doi.org/10.1016/j.econmod.2011.05.012
- Corbo, V. (1985). Reforms and macroeconomic adjustments in Chile during 1974–1984. World Development, 13(8), 893–916. https://doi.org/10.1016/0305-750X(85)90074-9
- Cushman, D. O. (1985). Real exchange rate risk, expectations, and the level of direct investment. The Review of Economics and Statistics, 67(2), 297. https://doi.org/10.2307/1924729
- Darby, J., Hallett, A. H., Ireland, J., & Piscitelli, L. (1999). The impact of exchange rate uncertainty on the level of investment. The Economic Journal, 109(454), 55–67. https://doi.org/10.1111/1468-0297.00416
- de Castro, P. G., Fernandes, E. A., & Campos, A. C. (2013). The determinants of foreign direct investment in Brazil and Mexico: An empirical analysis. Procedia Economics and Finance, 5, 231–240. https://doi.org/10.1016/s2212-5671(13)00029-4
- De Vita G and Kyaw K S. (2008). Determinants of capital flows to developing countries: a structural VAR analysis. Journal of Economic Studies, 35(4), 304–322. 10.1108/01443580810895608
- Eichengreen, B., & Tong, H. (2007). Is China’s FDI coming at the expense of other countries? Journal of the Japanese and International Economies, 21(2), 153–172. https://doi.org/10.1016/j.jjie.2006.07.001
- Ekholm, K., Forslid, R., & Markusen, J. R. (2007). Export-Platform foreign direct investment. Clinical Gastroenterology and Hepatology: The Official Clinical Practice Journal of the American Gastroenterological Association, 5(7), 776–795. https://doi.org/10.1016/j.cgh.2007.05.008
- Elhorst, J. P. (2010). Applied spatial econometrics: Raising the bar. Spatial Economic Analysis, 5(1), 9–28. https://doi.org/10.1080/17421770903541772
- Felipe, J., & Llamosas-rosas, I. (2018). Determinants of FDI attraction in the manufacturing sector in Mexico. Working Papers 2018-07, Banco de México https://ideas.repec.org/p/bdm/wpaper/2018-07.html.
- Feng, Y., Wang, X., Du, W., Wu, H., & Wang, J. (2019). Effects of environmental regulation and FDI on urban innovation in China: A spatial Durbin econometric analysis. Journal of Cleaner Production, 235, 210–224. https://doi.org/10.1016/j.jclepro.2019.06.184
- Ffrench-Davis, R. (1983). The monetarist experiment in Chile: A critical survey. World Development, 11(11), 905–926. https://doi.org/10.1016/0305-750X(83)90054-2
- Fingleton, B., & Le Gallo, J. (2010 Endogeneity in a Spatial Context: Properties of Estimators Páez, A, Gallo, J, Buliung, R, Dall'erba, S eds.). . Progress in Spatial Analysis. Advances in Spatial Science (Berlin: Springer), . https://doi.org/10.1007/978-3-642-03326-1_4
- Fischer, M. M., Pintar, N., & Sargant, B. (2017). Austrian outbound foreign direct investment in Europe: A Spatial Econometric Study. SSRN Electronic Journal. https://doi.org/10.2139/ssrn.2898092
- Froot, K. A., & Stein, J. C. (1991). Exchange rates and foreign direct investment: An Imperfect Capital Markets Approach. The Quarterly Journal of Economics, 106(4), 1191–1217. https://doi.org/10.2307/2937961
- Fujita, M., Krugman, P., & Venables, A. J. (1999). The spatial economy: Cities, regions, and international trade. Southern Economic Journal 67 2 491–493 . https://doi.org/10.2307/1061487
- Garretsen, H., & Peeters, J. (2009). FDI and the relevance of spatial linkages: Do third-country effects matter for Dutch FDI? Review of World Economics, 145(2), 319–338. https://doi.org/10.1007/s10290-009-0018-1
- Ghodsi, M. (2020). How do technical barriers to trade affect foreign direct investment ? Tariff jumping versus regulation haven hypotheses. Structural Change and Economic Dynamics, 52, 269–278. https://doi.org/10.1016/j.strueco.2019.11.008
- Guo, J. Q., & Trivedi, P. K. (2002). Flexible parametric models for long-tailed patent count distributions. Oxford Bulletin of Economics and Statistics, 64(1), 63–82. https://doi.org/10.1111/1468-0084.00004
- Hattari, R., Rajan, R. S., & Thangavelu, S. (2014). Intra-ASEAN FDI flows and the role of China and India: Trends and determinants (pp. 69–88). Issues Governance, Growth Glob. https://doi.org/10.1142/9789814504959_0005
- Head, K., & Mayer, T. (2004). Market potential and the location of Japanese investment in the European Union. Review of Economics and Statistics, 86 4 959–972 https://www.jstor.org/stable/40042982 .
- Hoang, H. H. (2012). Foreign direct investment in Southeast Asia: Determinants and spatial distribution. Depocen, 30, 1–24 http://depocenwp.org/modules/download/index.php?id=146.
- Hoang, H. H., & Bui, D. H. (2015). Determinants of foreign direct investment in ASEAN: A panel approach. Management Science Letters, 5(2), 213–222. https://doi.org/10.5267/j.msl.2014.12.015
- Hoang, H. H., & Goujon, M. (2019). Determinants of intra-region and extra-region foreign direct investment inflow in ASEAN: A spatial econometric analysis. Applied Spatial Analysis and Policy, 12(4), 965–982. https://doi.org/10.1007/s12061-018-9280-8
- Ismail, N. W. (2009). The determinant of foreign direct investment in ASEAN: A semi-gravity approach. Transition Studies Review, 16(3), 710–722. https://doi.org/10.1007/s11300-009-0103-0
- Kelejian H H and Prucha I R. (2010). Specification and estimation of spatial autoregressive models with autoregressive and heteroskedastic disturbances. Journal of Econometrics, 157(1), 53–67. 10.1016/j.jeconom.2009.10.025
- Kiyota, K., & Urata, S. (2004). Exchange rate, exchange rate volatility and foreign direct investment. The World Economy, 27(10), 1501–1536. https://doi.org/10.1111/j.1467-9701.2004.00664.x
- Klein, M. W., & Rosengren, E. (1994). The real exchange rate and foreign direct investment in the United States. Relative wealth vs. relative wage effects. Journal of International Economics, 36(3–4), 373–389. https://doi.org/10.1016/0022-1996(94)90009-4
- Kogut, B., & Chang, S. J. (1996). Platform investments and volatile exchange rates: Direct investment in the U.S. by Japanese electronic companies. The Review of Economics and Statistics, 78(2), 221–231. https://doi.org/10.2307/2109924
- Kosteletou, N., & Liargovas, P. (2000). Foreign Direct Investment and Real Exchange Rate Interlinkages. Open Economies Review, 103 11 , 239–248 doi:10.1023/A:1008383821669.
- Lacombe, D. J., & Lesage, J. P. (2015). Using Bayesian posterior model probabilities to identify omitted variables in spatial regression models. Papers in Regional Science 94 2 , 365–383 http://hdl.handle.net/10.1111/pirs.12070.
- Ledyaeva, S. (2009). Spatial econometric analysis of foreign direct investment determinants in Russian regions. World Economy, 32(4), 643–666. https://doi.org/10.1111/j.1467-9701.2008.01145.x
- LeSage, J. P., & Pace, R. K. (2008). Spatial econometric modeling of origin-destination flows. Journal of Regional Science, 48(5), 941–967. https://doi.org/10.1111/j.1467-9787.2008.00573.x
- LeSage, J., & Pace, R. K. (2009). Introduction to spatial econometrics, introduction to spatial econometrics. Taylor & Francis Group, LLC, 6000 Broken Sound Parkway NW, Suite 300.
- Marouane, Alaya , & . (2019). FDI determinants and geographical interdependence in MENA region. The Economic Research Forum Working Paper, (1361 1–31). https://erf.org.eg/publications/fdi-determinants-and-geographical-interdependence-in-mena-region-2/
- Masron, T. A., & Abdullah, H. (2010). Institutional quality as a determinant for FDI inflows: Evidence from ASEAN. World Journal Management, 2, 115–128 10.1.1.470.4575.
- Nwaogu, Uwaoma. (2012). Essays on Spatial Analysis of F ys on Spatial Analysis of Foreign Dir eign Direct Inv ect Investment and estment and Economic Growth Determinants in Developing Countries (Western Michigan University)https://scholarworks.wmich.edu/cgi/viewcontent.cgi?article=1033&context=dissertations .
- Paun, C., Mustetescu, R., & Munteanu, C. (2013). The monetary approach of the balance of payments: Empirical evidences from emerging markets. Economic Computation and Economic Cybernetics Studies and Research, 3 3 , 133–150 https://www.researchgate.net/publication/280064852_The_monetary_approach_of_the_balance_of_payments_Empirical_evidences_from_emerging_markets.
- Peng, G., Liu, F., Lu, W., Liao, K., Tang, C., & Zhu, L. (2018). A spatial-temporal analysis of financial literacy in United States of America. Finance Research Letters, 26, 56–62. https://doi.org/10.1016/j.frl.2017.12.003
- Regelink, M., & Paul Elhorst, J. (2015). The spatial econometrics of FDI and third country effects. Letters in Spatial and Resource Sciences, 8(1), 1–13. https://doi.org/10.1007/s12076-014-0125-z
- Thangavelu, S. M., & Narjoko, D. (2014). Human capital, FTAs and foreign direct investment flows into ASEAN. Journal of Asian Economics, 35, 65–76. https://doi.org/10.1016/j.asieco.2014.11.002
- Tille C and van Wincoop E. (2010). International capital flows. Journal of International Economics, 80(2), 157–175. 10.1016/j.jinteco.2009.11.003
- Uttama, N. P., & Peridy, N. (2009). The impact of regional integration and third‑country effects on FDI: Evidence from ASEAN. Asean Economic Bulletin, 26(3), 239–252. https://doi.org/10.1355/ae26-3a
- van der Ploeg, F., & Poelhekke, S. (2009). Volatility and the natural resource curse. Oxford Economic Papers, 61(4), 727–760. https://doi.org/10.1093/oep/gpp027
- Vo X Vinh. (2018). Determinants of capital flows to emerging economies - Evidence from Vietnam. Finance Research Letters, 27 23–27. 10.1016/j.frl.2018.02.031
- World Bank, 2019 World Development Indicators Accessed 2019 https://databank.worldbank.org/source/world-development-indicators