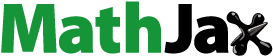
Abstract
Persistent energy consumption issues are ascribed to the failure of energy planners to understand the various macroeconomic factors that influence energy consumption. Therefore, we investigated the factors influencing energy consumption in six net African oil-exporting countries (AOECs) between 1980 and 2018. Our contribution to the literature is the use of estimators; cross-sectional autoregressive distributed lag (CS-ARDL) and cross-sectional distributed lag (CS-DL) modelling approaches, which take into account the time dynamics, the heterogeneity of different countries and cross-sectional dependence, to explore the relationship between energy consumption and its major determinants, such as openness, economic structure and per capita income. The results revealed that per capita income did not impact significantly on energy consumption in AOECs during the period under study, while trade openness had a positive and significant effect on it. The third variable of interest, namely economic structure, had a negative and significant effect on energy consumption. These results led to various recommendation for policymakers and future research in the concluding section.
PUBLIC INTEREST STATEMENT
The failure of the governments of African oil-exporting countries (AOECs) to improve energy consumption, which would lead to sustainable economic growth has been of major interest to scholars. Often, the inability of policymakers in these economies to obtain favourable results has been attributed to conflicting policy implementation and factors influencing energy consumption in the long run. To provide a better understanding of how energy consumption parameters drive sustainable economic growth of AOECs, the study investigated the factors determining energy consumption in these economies. We used both cross-sectional autoregressive distributed lag and cross-sectional distributed lag modelling approaches, which account for time dynamics, cross-sectional heterogeneity and cross-sectional dependence. The empirical findings showed that per capita income did not impact energy consumption significantly, while trade openness had a positive and significant effect. In addition, the study confirmed that economic structure tended to hinder energy consumption significantly. Thus, the study aimed to contribute to knowledge on the determinants of energy consumption in AOECs.
1. Introduction
The connection between energy consumption and economic growth has been well documented in the energy literature (Azam et al., Citation2015; Wang & Wang, Citation2020). The role of energy in propelling sustainable economic growth has been of great concern to energy economists and policymakers, especially in Africa where many countries are net African oil-exporting countries (AOECs; Bilgen, Citation2014; Dritsaki & Dritsaki, Citation2014; Fuinhas & Marques, Citation2012).
African countries have exhibited two tendencies in energy consumption and economic growth. Firstly, the region has the lowest energy consumption per capita, and the little energy it consumes is dominated by dirty fuel, which contributes to environmental deterioration (Kebede et al., Citation2010; Rennings et al., Citation2012). Secondly, Africa has the worst development indicators in the world, with high unemployment and poverty rates, poor access to education and health, and low life expectancy, for example, (Karekezi, Citation2002); (International Energy Agency (IEA), Citation2016).
A report from the World Bank shows that global poverty rate is on the decrease across various regions of the world, except in Africa where extreme poverty in sub-Saharan Africa (SSA), in particular, is on the increase (from 278 million in 1990 to 413 million in 2015). The poverty rate stood at 41% in 2015, compared to 13% in other regions. In addition, 27 out of 28 poorest countries in the World are from SSA (World Bank, Citation2018). This makes it difficult for the region to attain the poverty reduction target of the MDGs (Asongu et al., Citation2016; Asongu & Kodila-Tedika, Citation2015). Moreover, Shurig (Citation2015) maintains that only 5% of countries in SSA have access to electricity (IEA, Citation2016). This implies that per capita electricity consumption in the sub-region is one-sixth of the world’s average. In addition, the ineffective management of energy resources is another problem (Anyangwu, Citation2014). The governments of African countries subsidise fossil fuels, which are in high demand, at the expense of renewable energy. However, the increasing rate of fossil fuel consumption is one of the major causes of global warming due to environmental disturbance and contributes over 75% of carbon dioxide (C02) emissions (Huxster et al., Citation2015; Akpan and Akpan, Citation2012; Mundial, Citation2018). Nonetheless, fossil energy has improved humanity’s standard of living, which is reflected in the positive relationship between the total primary energy produced and the average gross national domestic product (GDP) per-capita.
However, owing to their greenhouse effect, fossil fuels need to be replaced by alternative energy sources (Smil, Citation2005). According to Waheed et al. (Citation2019), one aspect of energy consumption is concerned with economic growth, while the other relates to carbon emissions. Sustainable growth as well as averting environmental change need to be taken into cognisance while satisfying the growing energy demand. The energy produced from renewable sources can balance contradiction in the energy-mix market as well as guide the ecological environment (Ogbonnaya et al., Citation2019).
The use of energy covers diverse human activities, which include generating power, fueling machines and providing power to homes and industries (Haider & Adil, Citation2019). These activities are essential for the daily survival of human beings and the improvement of their standard of living. Physical labour or animals were used as power by people in the past. However, with increasing progress in technology, modern energyFootnote1 was substituted for traditional energy sources (Lean & Smyth, Citation2010; Stern, Citation2010). Modern energy constitutes an important input in the industrial process, mining, services, transportation and information sectors (Mallett, Citation2013). Hence, it is expected that, when industrial and agricultural activities increase, the demand for energy consumption will also increase. Increasing energy consumption, precisely industrial energy consumption, is a vital indicator of a country’s economic development level (Zhang et al., Citation2017). Therefore, low energy consumption and a poor energy mix are indicators of an underdeveloped economy (Karekezi & Kimani, Citation2002). Specifically, modern energy has been a fundamental part of economic growth for many developed economies since the great industrial upheaval.
As indicated above, there is a strong association between energy consumption and economic growth (Arabatzis & Malesios, Citation2011; Javid & Sharif, Citation2016; Koutroumanidis et al., Citation2009). Evidence from high-income countries reveals that high energy consumption correlates with high per capita income levels, whereas low energy consumption is associated with low-income countries (United States Energy Information Administration (US EIA), 2016). For instance, energy consumption in 2017 stood at 3132.2 million tonnes of oil equivalent (Mtoe) in China, 753.7 Mtoe in India and 295.9 Mtoe in South Korea, with growth rates of 6.76%, 7.17% and 3.06%, respectively. In the same year, the energy consumption of AOECs, such as Algeria, Angola and Nigeria was 51.8 Mtoe, 7.7 Mtoe and 28.2 Mtoe, respectively, with growth rates of 1.3%, −0.15% and 0.81%, respectively. This shows that affordable access to adequate and dependable energy supply is a fundamental factor in the attainment of the large productivity gains that guarantee sustainable economic growth.
Despite all the efforts being made by the AOECs to improve access to energy consumption and sustainable economic growth, it should, however, be noted that these have not been impressive in recent times (see, Clark et al., Citation2005; Karekezi & Kimani, Citation2002). The inability of the AOECs to improve both their energy consumption and income levels has been blamed on inconsistent policy implementation and policymakers’ failure to obtain adequate information on the factors influencing energy consumption.
The argument is that for countries that depend on crude oil exports for most of their foreign earnings (Davidson & Sokona, Citation2002; Wolde-Rufael, Citation2006), as a result of huge energy resources endowment (Kebede et al., Citation2010), the paradox of low energy consumption requires extensive investigation. This becomes more important when these countries also have poor developmental indicators and are affected by environmental issues resulting from the emissions of CO2. Given all these, it is, therefore, important to examine the determinants of energy consumption in this group of countries. This can be done by providing more precise estimates of major energy consumption parameters to understand how they drive sustainable economic growth. This approach is important for two reasons. Firstly, providing definite quantitative estimates for energy consumption parameters can help in policy making. Secondly, quantitative estimates will also help in forecasting future energy consumption.
Various studies have been conducted to identify factors influencing energy consumption. For instance, Kolawole et al. (Citation2017), point out that basic economic theory suggests that the demand of a good or service is usually dependent on factors like the price of the good, the income of the buyers, the price of a close substitute and other exogenous factors associated to the nature of the good. This was further buttressed by Xiong et al. (Citation2020) and US EIA (Citation2021) that prices of close substitutes, like natural gas and solar, are also important factors determining energy consumption. Similarly, Atakhanova and Howie (Citation2007) and Bohlmann and Inglesi-Lotz (Citation2021) conclude that the income of the consumer as well as energy price are factors influencing energy consumption. Other major variables influencing energy consumption, as pointed out by Samuel et al. (Citation2013) and Sineviciene et al. (Citation2017), include economic growth rate, population, foreign direct investment and industrial growth. Findings from these studies are mixed and have not reached a consensus. So, there is a need to carry out an empirical study on the topic since no study to the best of our knowledge has been conducted to investigate the major drivers of energy consumption in AOECs, using cross-sectional augmented autoregressive distributed lag (CS-ARDL) and cross-sectional distributed lag (CS-DL).
The focus of the study, therefore, was to identify drivers that help explain the influence of energy consumption in AOECs, using macroeconomic drivers such as per capita income, trade openness, economic structure and the reaction of the consumers to these drivers in the long run. Having enough understanding of their reaction to these drivers would serve as an important instrument for formulating an effective energy policy that will improve access to sustainable energy resources in AOECs.
Our novel contribution to the literature in AOECs, unlike related studies conducted by Samuel et al. (Citation2013) on the determinants of energy consumption and Kolawole et al. (Citation2017) for sub-Saharan Africa, is the use of recent panel data estimators, CS-ARDL and CS-DL estimating techniques that are robust to cross-sectional dependence and small sample size bias. Banerjee and Carrion‐i‐silvestre (Citation2015) maintain that the power of unit root and cointegration tests may be increased by combining the information from cross-section and time dimensions. This is especially beneficial when the time dimension is limited owing to the lack of accessibility to long series of consistent time series data, which is generally the case for African countries. Our methodologies controlled for time dynamics, cross-sectional heterogeneity and cross-sectional dependence because countries may share the same characteristics. We used the most significant determinants (per capita income, economic structure and openness) and constructed the unit root test based on the second-generation test that controlled for cross-sectional dependence. Although the degree of integration of these variables of interest was uncertain, we used the autoregressive distributed lag (ARDL) technique developed by Pesaran and Smith (Citation1995), as extended by Pesaran et al. (Citation1997), to evaluate the likelihood of a long-run cointegration relationship. Following the presence of cross-sectional dependence after conducting the Pesaran’s (Citation2004) test, versions of the dynamic common correlated effects (dynamic CCE) estimator built by Chudik and Pesaran (Citation2013), the CS-ARDL and the CS-DL estimating techniques, which are robust to small sample bias, were applied. We found that the three variables explained the variation of energy consumption in various ways. While trade openness had a positive and significant effect on energy consumption, the effect of economic structure was negative and significant. The results revealed that openness drove energy consumption in AOECs during the period under review. Conversely, economic structure did not drive energy consumption in the countries under study. Similarly, per capita income did not affect energy consumption. Most of these findings did not conform to both theoretical expectations and some previous empirical findings. These results, therefore, indicated that AOECs may behaved differently when it comes to what drives their energy consumption for a variety of reasons ranging from structural rigidity to their inability to transition to a more efficient and growth-enhancing energy regime.
The following section of this article reviews the relevant literature, whilst the third section presents the theoretical framework for the study, the sources of the data and the research methodology. The empirical results are presented and discussed in the fourth section, which is followed by the conclusion and recommendations for policy and research
2. Literature review
Varian’s (Citation1992) neoclassical consumption theory postulates that consumption is based on primary economic variables, influencing the demand for a commodity. According to this theory, consumption is linked to individual preferences and other factors affecting demand, such as the price of a commodity and income. Therefore, all these variables are incorporated in conventional energy demand models. Like other goods, energy is consumed primarily because of the satisfaction derived from it and its utility, for example, in production for industrialist and in operating domestic appliances for households. In addition, therefore, an increase in income would positively impact energy consumption, although the reverse happens when a consumer’s income falls. An increase in the price of energy would cause a consumer to consume less or seek an alternative source (see, Varian, Citation1992).
Several empirical studies have been conducted on the determinants of energy consumption (Samuel et al., Citation2013). For instance, Aziz et al. (Citation2013) investigated factors driving energy demand in 16 developing countries using panel data analysis. Findings from the study suggested that price and income were crucial factors determining energy consumption in these economies and economic structure and C02 emissions had a significant positive impact on energy use.
Azam et al. (Citation2015) employed ordinary least squares (OLS) to explore the determinants of energy consumption in Indonesia, Malaysia, and Thailand between 1980 and 2012. Their findings revealed that the inflow of foreign direct investment, economic growth, trade openness, and human capital development index had a positive and statistically significant effect on energy consumption. Urbanisation had a significant positive effect on energy consumption in Indonesia and Thailand, whilst the rate of population growth had positive and statistically significant effect on energy consumption in Malaysia.
Keho (Citation2016) investigated the drivers of energy consumption in 12 sub-Saharan African countries. Bounds test cointegration was employed to test the data set for individual countries from 1970 to 2011. Results from the study revealed evidence of cointegration with industrial output, imports, income, foreign direct investment, urbanization, population, and domestic credit to the private sector. It was further established that the share of the industrial sector in GDP, urbanization, population and per capita income had a long-run relationship with energy consumption. Keho (Citation2016) concluded that industrial output, economic/population growth were the major variables that influenced energy consumption in virtually all the countries of interest.
Using panel data analysis, Dalei (Citation2016) investigated the drivers of energy consumption in open economies, namely China, Japan, and India. The findings of the study revealed that trade openness, GDP, and carbon emissions influenced energy consumption in these economies during the period under study. Kolawole et al. (Citation2017) investigated factors that influenced energy demand in 16 countries in SSA from 1980 to 2014 using the panel cointegration technique. Their results confirmed income and urbanisation as major factors determining energy consumption.
Sarkodie and Adom (Citation2018) used nonlinear iterative partial least squares (NIPALS) to examine the drivers of aggregate energy consumption in Kenya. The findings revealed that price, population density, urbanisation and renewable energy from hydro sources played a vital role in promoting energy demand. However, climate change and higher income were expected to cause an increase in alternative energy consumption. Moreover, the growth in population rate was expected to increase the consumption of alternative energy, because of the fragility of the electricity infrastructure dependent on fossil fuels.
Zaharia et al. (Citation2019) used both bibliometric analysis and panel data techniques to analyse factors determining energy consumption in the EU-28 countries between 1995 and 2014. The results from the study showed that greenhouse gas emissions, GDP, population and labour growth had a positive correlation with both primary and final energy consumption. However, female population increase, healthcare expenditure and energy taxes had a negative impact.
Fernandes and Reddy (Citation2021) used the vector error correction model (VECM) to investigate the determinants of energy consumption in a sample of newly industrialised countries in Asia (China, India, Indonesia, Malaysia, Philippines and Thailand). The study found that industrialisation, the exchange rate, financial development and trade openness had a significant impact on energy consumption in China. In Thailand and India, only industrialisation had an impact, whilst GDP and trade openness had a significant impact on energy consumption in Indonesia and Malaysia, respectively.
Adetola and Sunday (Citation2021) investigated the determinants of energy consumption in Egypt using time series data from 1971 to 2017. The study adopted a wavelet coherent method for the analysis and the results revealed a positive connection between energy consumption and urbanisation at various scales and a bidirectional causal connection between the two variables. Additionally, the results revealed that imports were a significant determinant of energy consumption in diverse frequencies in Egypt. Moreover, evidence of bidirectional causality was found between economic growth and energy consumption, whilst gross capital formation was found to drive energy consumption in Egypt during the period under review. The researcher concluded that policymakers in Egypt should formulate policies to ensure sustainable energy consumption.
Canh et al. (Citation2021) integrated the influence of a shadow economy by using the stochastic impacts by regression on population, affluence and technology (STIRPAT) model for 115 countries between 1991 and 2014 to investigate the determinants of energy consumption. The study employed the system-GMM estimation technique to examine the determinants of energy consumption according to three aspects: level, intensity and renewable. The analysis of the impact of the shadow economy and the drivers of energy consumption contributed to the literature by showing that in the global sample, a higher informal sector caused a higher level, higher intensity and higher renewable energy consumption.
In studies in other fields, the CS-ARDL approach has been used to estimate long run relationships in the empirical application of macroeconomics models (Ditzen, Citation2019). Using the CS-ARDL approach, Mohaddes and Raissi (Citation2017) investigated the effect of commodity terms of trade (CTOT) volatility on economic growth in commodity-dependent countries and also assessed the role of sovereign wealth funds (SWFs) and quality of institutions in their long-term growth performance. The results showed that while CTOT volatility exerted a negative effect on economic growth, the average effect was dampened if a country had an SWF and better institutional quality. Raissi and Anderson (Citation2018) explored the long-term effect of incessant corporate debt accumulation on the productivity growth of Italian firms and examined if total factor productivity growth changed with the level of corporate indebtedness. The results revealed the significant negative impact of incessant corporate debt increase on total factor productivity growth and a weak indication of a threshold level of corporate debt, after which productivity expansion fell considerably. Ahmed (Citation2020) examined the effect of business and consumer sentiment on stock market performance within the contexts of both advanced and emerging markets. The study revealed that for developed markets, the business sentiment had a positive impact on stock returns, covering short- and long-term time horizons, while for emerging markets, the price effect of business sentiment was short-lived. However, consumer sentiment tended to influence both market types positively, although only in the short run. Moreover, the impact of sentiment appeared stronger in emerging markets than it did in developed countries.
The CS-ARDL model has been extensively used according to the literature because it is robust to omitted variable bias and the simultaneous determination of growth regressors. The validity of this method is the long-run connection amongst the variables of interest, the dynamic specification of the model, which sufficiently augmented for the regressors to become weakly exogenous and the resulting residual which is serially uncorrelated (Chudik & Pesaran, Citation2013). Moreover, CS-ARDL and CS-DL models account for cross heterogeneity, cross-sectional dependence arising from unobserved common factors, spillover effects dynamics and feedback effects. Therefore, they were used to investigate the factors influencing energy consumption, making the study novel, as we are not aware of any oil-related study that has employed these models to develop a CS-ARDL and CS-DL estimation for developing net oil-exporting countries in Africa. Therefore, this study contributes to knowledge by exploring CS-ARDL and CS-DL estimating techniques to investigate the relationship between energy consumption and its determinants in AOECs.
3. Methodology
3.1. Theoretical framework
Based on the theory of consumer behaviour and the studies conducted by Mudakkar et al. (Citation2013) and Tang and Tan (Citation2014), a framework was designed to investigate the determinants of energy consumption. Given that the theory of consumer behaviour assumes that all consumers are rational and will maximise utility/satisfaction with each unit of consumption subject to given budget constraints, the energy consumption function can be written as follows:
where represents energy consumption,
represents the level of income,
represents the price of energy,
represents the price of non-energy goods and
represents vector of factors influencing energy consumption. Assuming homogeneity, the specification of energy consumption can also be itemised as a function of relative price and real income of energy to non-energy goods as follows:
In the energy consumption framework in Equationequation (2)(2)
(2) , energy consumption is dependent on relative price, real income and other factors. According to the existing literature, other factors that affect energy consumption include economic structure, C02 emission, population size, employment and urbanisation (see, for instance, Azam et al., Citation2015; Aziz et al., Citation2013; Esen & Bayrak, Citation2017; Keho, Citation2016; Kolawole et al., Citation2017; Sarkodie & Adom, Citation2018). Therefore, the functional relationship between energy consumption and its determinants was modified as follows:
In Equationequation (3)(3)
(3) ,
denotes energy consumption,
denotes per capita income,
denotes trade openness and
denotes economic structure. The empirical model of energy consumption and its determinants could be written as follow:
where is the natural logarithm and the error term
is expected to be spherically distributed and white noise. The a priori expectations for the parameters in Equationequation 4
(4)
(4) are as follows:
.
3.2. Data and measurement of variables
The study investigated the factors influencing energy consumption in six net Africa oil-exporting countries; Angola, Algeria, Egypt, Gabon, Republic of the Congo and Nigeria (Sudan and Libya were left out because of the due non-availability of data). The choice of these countries was premised on their being exporters and producers of oil (OPEC, Citation2018). The study used annual data for the period between 1980 and 2018, the length of which allowed the recognition of changes in variables due to various energy-sector reforms to discover energy demand patterns (Kolawole et al., Citation2017; Shafiei et al., Citation2013). The dependent variable, energy consumption (EC), was proxied by total primary energy consumption in Mtoe. In line with Azam et al. (Citation2015), the trade indicator was proxied by trade openness (OPEN), which was the ratio of the total value of imports and exports of goods and services to GDP expressed as a percentage. In addition, in line with Kolawole et al. (Citation2017), economic structure (ES) was proxied by the value added of industrial output as a percentage of GDP. This comprised the value added of mining, manufacturing, construction, electricity, water and gas. Value added is the net output of a sector after adding up all outputs and subtracting intermediate inputs. This is calculated without making deductions for the depreciation of fabricated assets and the degradation of natural resources. In line with the empirical work of Kolawole et al. (Citation2017) and Keho (Citation2016), per-capita income (PERINC) was proxied by the GDP per capita of the selected AOECs in constant 2010 US$. This was measured as the ratio of GDP to the midyear population. Data on energy consumption were obtained from the USEIA (Citation2016) and data on the trade openness trade, economic structure and per capita income variables were sourced from the World Development Indicators (WDI; World Bank, Citation2016). In addition, all the variables were expressed in natural logarithms, except economic structure and trade openness, which were already in percentages.
3.2.1. Cross-sectional dependence test
Cross sectional dependence may occur because of the existence of common shocks and spatial spillover effects among cross sections (Damette & Seghir, Citation2013; De Hoyos & Sarafidis, Citation2006) and in panel data, may result in loss of efficiency and misleading statistics. According to Pesaran (Citation2006), errors in cross-sectional dependence in the panel model may lead to biased estimates.Footnote3 Consequently, it is important to test for error in cross-sectional dependence. To achieve this, we used the Pesaran’s (Citation2004) cross-sectional dependence (CD) tests as per the following specification:
where represents the time interval,
represents the number of cross sectionals, and
is the pairwise correlation coefficient between the cross sections. In addition, the asymptotic distribution of both CD tests is standard normal (
and
, and they have acceptable sample size properties. The null hypothesis for these tests was that there is cross-sectional independence in the panel model residuals.
3.2.2. Panel unit root test
The panel unit root test was employed in this study to test the stability of the variables (Wang & Su, Citation2019). Following the presence of cross-sectional dependence, the first-generation panel unit root tests like those conducted by Levin et al. (Citation2002), Im et al. (Citation2003), and Maddala and Wu (Citation1999) may be biased as a result of their harsh assumption of no cross-sectional dependence in the data (O’Connell, Citation1998; Westerlund, Citation2005). Therefore, we employed a second-generation panel unit root test, Pesaran’s (Citation2007) cross sectionally augmented Im Pesaran Shin (CIPS) test, which assumes that cross-sectional dependence is in the form of a single unobserved common factor. Pesaran’s (Citation2007) modified version of Im et al.’s (Citation2003) standard augmented dickey fuller (ADF) model, in conjunction with the cross-sectional averages of lagged levels and first differences of individual series, results in the cross-sectional augmented dickey-fuller (CADF) model, which is expressed as follows:
where and
As stated above, the CIPS test statistic is calculated as the average of the CADF test statistic of cross- sections. This is specified as follows:
Where denotes the CADF statistic for each
-th cross-section. Moreover, since it was likely that the dataset would suffer from cross-sectional dependence, the null hypothesis follows: All series are non-stationary. The alternative hypothesis was as follows: Some of the series are stationary.
3.2.3. Panel autoregressive distributed lag method
In line with Chudik and Pesaran (Citation2013), the study explored the panel autoregressive distributed lag (p. -ARDL) approach to determine, the presence of long and short-run relationships between energy consumption and its determinants in AOECs. The ARDL methodology was chosen because of its salient advantages over conventional short and long run techniques. The advantages are as follows: (i) The ARDL framework provides for the determination of both short- and long-run dynamic parameters (ii) It provides for diverse lagged variables, as they are allocated in the model (iii) The framework permits the mixture of both I(0) and I(I) data but not I(2) amongst others (Dritsakis, Citation2011; Katircioglu, Citation2009; Narayan, Citation2005; Rafindadi & Yosuf, Citation2013). We considered the following equation for the panel ARDL model:
where = 1, 2, …,
denotes the cross-sectional units,
1, 2, 3, … ., T denotes the periods,
represents the number of time lags,
denotes the lag of the dependent variable,
represents the lag of the independent variables in the equation,
represents the vector of the explanatory variables and
is the fixed effect. In line with Pesaran et al. (Citation1999) study, Equationequation (8)
(8)
(8) was explicitly rewritten as follows:
We rewrote Equationequation (9)(9)
(9) in error correction form to capture the highlight of the long-term relationship to accommodate the short-term adjustment. This was expressed as follows:
Where =
,
and
The term
denotes the error correction term, which is the long-run relationship between the dependent variables and the explanatory variables and
is the dependent variable.
is a
x 1 vector of independent variables,
is a vector that contains the long-run relationships. The parameter
is the error-correcting speed of the adjustment term. If
then we conclude no cointegration relationship existed between the variables.Footnote4
According to Pesaran and Smith (Citation1995), model (10) is expressed separately for each group and computed as an average of the different coefficients. The mean group (MG) estimator allows the intercept, all the coefficients and error variance to differ across groups. Pesaran et al. (Citation1999) propose another estimator called the pool mean group (PMG) estimator. This estimator allows the intercepts, short-run coefficients and error variances to change across groups but constrains long-run coefficients to be equal. Moreover, this estimator depends on a combination of pooling/averaging and employs a maximum likelihood method.
Chudik and Pesaran (Citation2013) note that the correlation of unobserved common factors with regressors will lead to the ARDL approach being inconsistent. To overcome this, we employed the CS-ARDL, which is an extension of Pesaran’s (Citation2006) model and built on Chudik and Pesaran’s (Citation2013) model. Chudik and Pesaran (Citation2013) explain that this estimator supports the ARDL regressions with cross-sectional averages of the regressors, the dependent variable and a sufficient number of lags. The specification of CS-ARDL is expressed as follows:
Where ,
and
represent the simple cross-sectional averages of
and
, with all other variables remaining as defined in equation (10).
Since the CS-ARDL may be subject to the small T bias, we introduced the cross-sectional distributed lag (CS-DL) estimator based on Chudik and Pesaran (Citation2013)Footnote5 model. According to Chudik and Pesaran (Citation2013), the estimator is robust to a number of issues, such as a unit root of regressors and its better small sample performance. However, the CS-DL should be used in combination with others since no feedback is expected from the independent variable (see; Chudik & Pesaran, Citation2013). Therefore, we specified CS-DL as follows:
4. Empirical results and discussion
4.1. Cross-sectional dependence test results
The Pesaran’s test results of the cross-sectional dependence test conducted on the regression model are shown in Table . The null hypothesis of no correlation of the residual was rejected. This indicated that cross-sectional dependence affected the countries under review, which justifies the use of the CS-ARDL estimator to correct this problem.
Table 1. Pesaran’s (Citation2004) CD test
4.2. Panel unit root test results
Cross section dependence in panel data time series constraints the consistency of first-generation panel unit root tests, which presume cross-section independence. Accordingly, we employed CIPS unit root test by Pesaran (Citation2007) to determine whether our variables of interest were stationary under the assumption of cross-sectional dependence. The null hypothesis of the CIPS test assumes that all series are non-stationary, the alternative hypothesis states that some series are stationary. It is therefore be noted that when CIPS statistic is larger than the critical value, the null hypothesis is rejected at the corresponding significance level. The results of the CIPS unit root test were provided in Table .
Table 2. CIPS Panel Unit Root Test
According to the results, most of the variables appeared to be integrated of order one, that is, I (1). Notably, the CIPS statistics were significant for EC at levels; I (0) with both the intercept and the intercept with trend, and for PERINC at levels I (0) with the intercept and trend only. Thus, the null hypothesis of non-stationary was rejected. Nevertheless, the rest of the variables were nonstationary and become stationary at first difference. Therefore, we inferred that the data were approximately stables. Given the results of the CIPS unit root test, which revealed that the variables were integrated in the order of zero and one, that is, I (0) and I (1). There was need to check for the existence of a long-run relationship between the variables. We applied Pesaran et al.’s (Citation1997, Citation1999)Footnote6 panel autoregressive distributed lag (P-ARDL) to explore the long-run cointegration. However, none of the variables of interest was I (2), which may have led to misleading estimations (see, Pesaran et al., Citation2001).
4.3. Panel autoregressive distributed lag results
The estimation results of the model (P-ARDL) for the long run and the speed of adjustment are presented in Table for both the MG and the PMG. Since one of the assumptions of the PMG is premised on long-run homogeneity, the MG was considered based on a rejection of poolability and the residual test. Therefore, in Model 2, the estimates indicated that in the long run/all the variables were statistically significant in explaining the variation in energy consumption, except economic structure. It is observed that OPEN and PERINC had a positive long-run impact on energy consumption, while ES has a negative insignificant impact on energy consumption in AOECs during the period under study.
Table 3.: MG and PMG estimates
The OPEN estimate indicated that a 10% increase in OPEN would lead to a 0.04% increase in energy consumption. This suggests that an increasing influx of trading opportunities could increase commercial activities and thus higher energy consumption. This was in line with the theory and empirical literature (Azam et al., Citation2015; Dalei, Citation2016).
Interestingly, the estimate indicated that a 10% increase in PERINC would lead to an 8.7% increase in energy consumption, which conformed with empirical studies and economic theory (Aziz et al., Citation2013; Keho, Citation2016; Kolawole et al., Citation2017). In AOECs, most energy users find it difficult to pay their bills, which leads to electricity disconnection. Therefore, an increase in income would increase their energy consumption.
The estimate indicated that ES did not influence energy consumption, which was in line with the findings of empirical studies (Kolawole et al., Citation2017). Zhang et al. (Citation2017) stress that increasing industrial energy consumption is a symbol of economic development. However, the study revealed that this was not the case in the AOECs under review, the reason for which could be inadequate energy infrastructure or household poverty despite these countries being endowed with various energy resources.
In the model 2, the speed of adjustment was negative and statistically significant at the 5% level, signifying that in the long run, equilibrium was achievable. This indicated that 13.6% of the deviation of the variables from long-run equilibrium was corrected annually following a shock. Hence, it would take approximately 4 years for EC to return to the equilibrium level. These results were in line with those of Kolawole et al.’s (Citation2017) study that revealed that a highly significant speed of adjustment indicates the presence of a stable long-run relationship.
Considering the MG estimates, only PERINC was statistically significant for all the specifications at a 10% level. The speed of adjustment was significant and correctly signed for Models 1 and 3, signifying a correction of close to 29.4% every year following equilibrium for Model 1 only.
As stated earlier, the existence of cross-sectional dependence may bias the MG and PMG estimates. Therefore, to overcome this problem, we estimated a CS-ARDL model up to three lags. The results are presented in Table . The coefficients of ES and OPEN differed. Evidently in Model 5, the coefficient of OPEN indicated a positive and statistically significant relationship with EC at a 10 percent level, which suggested that a 10% increase in OPEN would result in about a 0.02% increase in energy consumption. This result was similar to that of Fernandes and Reddy’s (Citation2021) study, which found that OPEN had a significant impact on EC in China and Malaysia. This suggested that an increase in trade openness (international trade) increases economic activities, which would lead to higher energy consumption in AOECs and other countries. This is consistent with arguments put forward by Cole (Citation2006) and Shahbaz et al. (Citation2014), but contrary to Sbia et al. (Citation2014) study.
Table 4.: CS-ARDL
The coefficient of ES indicated a negative and statistically relationship with EC at 10% level, which suggested that an increase in the contribution of the industrial sector to the GDP would not substantially affect energy consumption in AOECs. A plausible explanation might be that the industrial sector of these countries is dominated by resource-based industries, mining and oil/gas exploitation, which may not drive energy consumption the same way as manufacturing value-added. In addition, these countries are still dominated by the primary sector despite large oil and gas deposits. For example, in Nigeria, agriculture contributes 21.65% to the GDP, while the industrial sector and oil/gas contribute 9.91% and 8%, respectively. This suggested that the economies of AOECs as shown by the Nigeria example are still driven by primary sector with little value-added activities and therefore limits the energy consumption.
The estimate revealed PERINC did not have significant effect on energy consumption in all the countries under review, which is contrary to Keho’s (Citation2016), Kolawole et al.’s (Citation2017), and Bohlmann and Inglesi-Lotz’s (Citation2021) findings. A probable reason might be that growth in per capita income in the countries is driven by oil revenue and price and not by increase in production within these countries. This indicated that, as the foreign earnings of these countries on oil increase, there is tendency for the household to have more income to improve on their energy consumption. Nevertheless, the speed of adjustment for all the specified models recorded an interesting pattern; a correction of close to −1.04% occurred less than one year following disequilibrium.
The estimate of the CS-DL is presented in Table , which shows that there was no feedback from the control variable in the models, which did not make it better than the CS-ARDL. It is evident in Table that only PERINC was positive up to the third lag in all the specifications. However, the coefficient of ES maintained its negative effect on energy consumption. Only OPEN did not have any impact on energy consumption in all the models.
Table 5.: Cross-sectional DL
5. Conclusion and recommendations
The empirical evidence on the determinants of energy consumption seems to have provided diverse results driven by differences in dataset, methodology and proxy. Specifically, energy consumption seems to have been driven by different factors in both rich and poor economies. The study attempted to contribute to this debate by investigating the factors influencing energy consumption in AOECs during the period under review. This was to test whether factors driving energy consumption in these countries were sensitive to their status as net oil exporters. The study relied on the dataset of six samples of AOECs for the period 1980 to 2018. CS-ARDL and CS-DL modelling approaches were explored to consider the time dynamics, the heterogeneity and the possible problem of cross-sectional dependence that may occur, which most studies have not taken into cognisance.
The results revealed that trade openness, per capita income and economic structure influenced energy consumption in various ways. Specifically, trade openness had a positive and significant effect on energy consumption, and this may be because these countries rely on energy exports for most of their foreign earnings. The effect of the economic structure was negative and significant, suggesting that these countries have been unable to transform their economies despite huge oil earnings. Similarly, per capita income did not affect energy consumption, indicating that these economies have reached the stage where income levels do not drive energy consumption, which may be due to structural rigidity in the energy mix. Most of these findings did not conform to both theoretical expectations and previous empirical findings. These results, therefore, indicated that AOECs may behave differently when it comes to what drives their energy consumption for a variety of reasons ranging from structural rigidity to their inability to transition to a more efficient and growth-enhancing energy regime.
Based on the results of this study, some policy recommendations can be inferred. Firstly, since the structure of the economy, proxied by the share of the industrial sector, stunted energy consumption, policymakers should use oil earnings to grow manufacturing sectors in these countries. The governments of AOECs should encourage private investment from both internal and external sources, which can help to drive manufacturing value-added in these economies. Policymakers can kick start this process by providing an enabling environment to investors in industrial sectors to encourage investment and growth. Secondly, these countries should also accelerate the implementation of policies and create incentives that will stimulate the switch from fossil fuels to modern renewable energy. As stressed by Marinaș et al.’s (Citation2018) study, countries with stronger policy implementation, which encourages renewable energy consumption or sources, are the ones that enjoy higher growth rates of consumption of such energies. Thirdly, an important finding of the study was that per capita income did not have any impact on energy consumption, and this may be due to the low level of per capita income in the sampled countries and their energy consumption. Hence, these countries need to transform their economies structurally in a way that will make economic growth more inclusive, driven by domestic value-added and not exogenous income from primary products exports. A puzzling result from the study was the negative relationship between per capita income and energy consumption in AOECs. There is a need to provide more quantitative evidence of this relationship beyond offering plausible reasons. A better way to achieve this, is to use a new dataset on the energy mix to examine whether households transition from fossil fuel to renewable energy as per capita income increases. This may be grounds for future research.
Correction
This article has been corrected with minor changes. These changes do not impact the academic content of the article.
Disclosure statement
No potential conflict of interest was reported by the author(s).
Additional information
Funding
Notes on contributors
Akindele John Ogunsola
Akindele John Ogunsola is currently a PhD candidate in the School of Accounting, Economics and Finance (SAEF), College of Law and Management Studies, the University of KwaZulu-Natal, Durban, South Africa. In addition, he has served as a research assistant at the Macroeconomics Research Unit in the SAEF. He started his lecturing career in the Department of Economics, Faculty of the Social Sciences, Ekiti State University, Nigeria, as an assistant lecturer in 2016 and rose to the position of Lecturer II in 2019. He has published articles in well-recognised journals and presented his research at both local and international conferences.
Christian Kakese Tipoy
Christian Kakese Tipoy is a Senior Lecturer in the School of Accounting, Economics and Finance, College of Law and Management Studies at University of KwaZulu-Natal, South Africa. He holds a PhD degree from University of Pretoria, South Africa. He is a reviewer to many notable journals and an assessor to many universities around the world. He has also published several articles in many recognized journals.
Notes
1. In Africa, most of the renewable energy consumed is through traditional procedures which is being replaced by modern fossil fuel-based energy.
2. Note that several variables such as energy price, urbanisation, capital and labour were considered in our model. However, we retained the most significant variables in the model based on tests conducted. The results of other tests are available upon request.
3. This will happen if the unobserved common factors are undeniably correlated with the regressors
4. This could stand as a test for the presence of cointegration where a significant speed of adjustment contributes to cointegration between the sample variables of interest in the model.
5. Pros and cons of the ARDL, the CS-ARDL and the CS-DL can be retrieved from Chudik and Pesaran (Citation2013).
6. Not with standing the contradictory results on the order of integration, we ran the Westerlund’s (Citation2007) test. The result however showed that there were at least 2 cointegrating equations, indicating that there is long-run relationship between LNEC, LNPERINC, OPEN, and ES. Therefore, the null hypothesis of no cointegration was rejected. We will provide the results upon request.
References
- Adetola, O. J., & Sunday, A. T. (2021). Determinants Of energy consumption in Egypt: The Wavelet Coherence Approach. Studies in Business & Economics, 16(2).
- Ahmed, W. M. (2020). Stock market reactions to domestic sentiment: Panel CS-ARDL evidence. Research in International Business and Finance, 54, 101240. https://doi.org/10.1016/j.ribaf.2020.101240
- Akpan, G. E., & Akpan, U. F. (2012). Electricity consumption, carbon emissions and economic growth in Nigeria. International Journal of Energy Economics and Policy, 2(4), 292–17.
- Anyanwu, J. C. (2014). Marital status, household size and poverty in Nigeria: Evidence from the 2009/2010 survey data. African Development Review, 26(1), 118–137. https://doi.org/10.1111/1467-8268.12069
- Arabatzis, G., & Malesios, C. (2011). An econometric analysis of residential consumption of fuelwood in a mountainous prefecture of Northern Greece. Energy Policy, 39(12), 8088–8097. https://doi.org/10.1016/j.enpol.2011.10.003
- Asongu, S., El Montasser, G., & Toumi, H. (2016). Testing the relationships between energy consumption, CO 2 emissions, and economic growth in 24 African countries: A panel ARDL approach. Environmental Science and Pollution Research, 23(7), 6563–6573. https://doi.org/10.1007/s11356-015-5883-7
- Asongu, S., & Kodila-Tedika, O. (2015). On the empirics of institutions and quality of growth: Evidence for developing countries. SSRN Electronic Journal. African Governance and Development Institute WP/15/041. https://doi.org/10.2139/ssrn.2668581
- Atakhanova, Z., & Howie, P. (2007). Electricity demand in Kazakhstan. Energy Policy, 35(7), 3729–3743. https://doi.org/10.1016/j.enpol.2007.01.005
- Azam, M., Khan, A. Q., Zaman, K., & Ahmad, M. (2015). Factors determining energy consumption: Evidence from Indonesia, Malaysia and Thailand. Renewable and Sustainable Energy Reviews, 42, 1123–1131. https://doi.org/10.1016/j.rser.2014.10.061
- Aziz, A. A., Mustapha, N. H. N., & Ismail, R. (2013). Factors affecting energy demand in developing countries: A dynamic panel analysis. International Journal of Energy Economics and Policy, 3(4S), 1–6.
- Banerjee, A., & Carrion‐i‐silvestre, J. L. (2015). Cointegration in panel data with structural breaks and cross‐section dependence. Journal of Applied Econometrics, 30(1), 1–23. https://doi.org/10.1002/jae.2348
- Bank, W. (2016). World Development Indicators (WDI).
- Bilgen, S. (2014). Structure and environmental impact of global energy consumption. Renewable and Sustainable Energy Reviews, 38, 890–902. https://doi.org/10.1016/j.rser.2014.07.004
- Bohlmann, J. A., & Inglesi-Lotz, R. (2021). Examining the determinants of electricity demand by South African households per income level. Energy Policy, 148, 111901. https://doi.org/10.1016/j.enpol.2020.111901
- Canh, N. P., Schinckus, C., Dinh Thanh, S., & Hui Ling Chong, F. (2021). The determinants of the energy consumption: A shadow economy-based perspective. Energy, 225, 120210. https://doi.org/10.1016/j.energy.2021.120210
- Chudik, A., & Pesaran, M. H. (2013). Large panel data models with cross-sectional dependence: A survey. CAFE Research Paper, (13.15).
- Clark, A., Davis, M., Eberhard, A., Gratwick, K., & Wamukonya, N. (2005). Power sector reform in Africa: Assessing the impact on poor people. University of Cape Town Graduate School of Business/ESMAP.
- Cole, M. A. (2006). Does trade liberalization increase national energy use? Economics Letters, 92(1), 108–112. https://doi.org/10.1016/j.econlet.2006.01.018
- Dalei, N. N. (2016). Determinants of energy consumption in open economies. Contemporary Issues in Trade, Environment and Policy, 89–104.
- Damette, O., & Seghir, M. (2013). Energy as a driver of growth in oil exporting countries? Energy Economics, 37, 193–199. https://doi.org/10.1016/j.eneco.2012.12.011
- Davidson, O., & Sokona, Y. (2002). Think bigger act faster. EDRC/ENDA.
- De Hoyos, R. E., & Sarafidis, V. Testing for cross-sectional dependence in panel-data models. (2006). The Stata Journal: Promoting Communications on Statistics and Stata, 6(4), 482–496.3.3. Empirical approach. https://doi.org/10.1177/1536867X0600600403
- Ditzen, J. (2019). Estimating long run effects in models with cross-sectional dependence using xtdcce2. Centre for Energy Economics Research and Policy (CEERP) Working Paper No, 7.
- Dritsaki, C., & Dritsaki, M. (2014). Causal relationship between energy consumption, economic growth and CO2 emissions: A dynamic panel data approach. International Journal of Energy Economics and Policy, 4(2), 125.
- Dritsakis, N. (2011). Demand for money in Hungary: An ARDL approach. Review of Economics and Finance, 5.
- EIA, U.S. (2021). The international energy outlook, 2016.
- Esen, Ö., & Bayrak, M. (2017). Does more energy consumption support economic growth in net energy-importing countries? Journal of Economics, Finance and Administrative Science, 22(42), 75–98. https://doi.org/10.1108/JEFAS-01-2017-0015
- Fernandes, K., & Reddy, Y. V. (2021). Determinants of energy consumption in newly industrialised countries of Asia. International Journal of Energy Economics and Policy, 11(1), 93. https://doi.org/10.32479/ijeep.10725
- Fuinhas, J. A., & Marques, A. C. (2012). Energy consumption and economic growth nexus in Portugal, Italy, Greece, Spain and Turkey: An ARDL bounds test approach (1965–2009). Energy Economics, 34(2), 511–517. https://doi.org/10.1016/j.eneco.2011.10.003
- Haider, S., & Adil, M. H. (2019). Does financial development and trade openness enhance industrial energy consumption? A sustainable developmental perspective. Management of Environmental Quality: An International Journal, 30(6), 1297–1313. https://doi.org/10.1108/MEQ-03-2019-0060
- Huxster, J. K., Uribe-Zarain, X., & Kempton, W. (2015). Undergraduate understanding of climate change: The influences of college major and environmental group membership on survey knowledge scores. The Journal of Environmental Education, 46(3), 149–165. https://doi.org/10.1080/00958964.2015.1021661
- Im, K. S., Pesaran, M. H., & Shin, Y. (2003). Testing for unit roots in heterogeneous panels. Journal of Econometrics, 115(1), 53–74. https://doi.org/10.1016/S0304-4076(03)00092-7
- International Energy Agency. (2016). World energy statistics 2016. iea.
- Javid, M., & Sharif, F. (2016). Environmental Kuznets curve and financial development in Pakistan. Renewable and Sustainable Energy Reviews, 54, 406–414. https://doi.org/10.1016/j.rser.2015.10.019
- Karekezi, S. (2002). Poverty and energy in Africa—a brief review. Energy Policy, 30(11–12), 915–919. https://doi.org/10.1016/S0301-4215(02)00047-2
- Karekezi, S., & Kimani, J. (2002). Status of power sector reform in Africa: Impact on the poor. Energy Policy, 30(11–12), 923–945. https://doi.org/10.1016/S0301-4215(02)00048-4
- Katircioglu, S. T. (2009). Revisiting the tourism-led-growth hypothesis for Turkey using the bounds test and Johansen approach for cointegration. Tourism Management, 30(1), 17–20. https://doi.org/10.1016/j.tourman.2008.04.004
- Kebede, E., Kagochi, J., & Jolly, C. M. (2010). Energy consumption and economic development in Sub-Saharan Africa. Energy Economics, 32(3), 532–537. https://doi.org/10.1016/j.eneco.2010.02.003
- Keho, Y. (2016). What drives energy consumption in developing countries? The experience of selected African countries. Energy Policy, 91, 233–246. https://doi.org/10.1016/j.enpol.2016.01.010
- Kolawole, A., Adesola, S., & De Vita, G. (2017). Factors that drive energy use in Africa: Panel data evidence from selected Sub‐Saharan African countries. OPEC Energy Review, 41(4), 364–380. https://doi.org/10.1111/opec.12115
- Koutroumanidis, T., Ioannou, K., & Arabatzis, G. (2009). Predicting fuelwood prices in Greece with the use of ARIMA models, artificial neural networks and a hybrid ARIMA–ANN model. Energy Policy, 37(9), 3627–3634. https://doi.org/10.1016/j.enpol.2009.04.024
- Lean, H. H., & Smyth, R. (2010). CO2 emissions, electricity consumption and output in ASEAN. Applied Energy, 87(6), 1858–1864. https://doi.org/10.1016/j.apenergy.2010.02.003
- Levin, A., Lin, C. F., & Chu, C. S. J. (2002). Unit root tests in panel data: Asymptotic and finite-sample properties. Journal of Econometrics, 108(1), 1–24. https://doi.org/10.1016/S0304-4076(01)00098-7
- Maddala, G. S., & Wu, S. (1999). A comparative study of unit root tests with panel data and a new simple test. Oxford Bulletin of Economics and Statistics, 61(S1), 631–652. https://doi.org/10.1111/1468-0084.0610s1631
- Mallett, A. (2013). Technology cooperation for sustainable energy: A review of pathways. Wiley Interdisciplinary Reviews: Energy and Environment, 2(2), 234–250. https://doi.org/10.1002/wene.54
- Marinaș, M. C., Dinu, M., Socol, A. G., & Socol, C. (2018). Renewable energy consumption and economic growth. Causality Relationship in Central and Eastern European Countries. PloS One, 13(10), e0202951.
- Mohaddes, K., & Raissi, M. (2017). Do sovereign wealth funds dampen the negative effects of commodity price volatility? Journal of Commodity Markets, 8, 18–27. https://doi.org/10.1016/j.jcomm.2017.08.004
- Mudakkar, S. R., Zaman, K., Shakir, H., Arif, M., Naseem, I., & Naz, L. (2013). Determinants of energy consumption function in SAARC countries: Balancing the odds. Renewable and Sustainable Energy Reviews, 28, 566–574. https://doi.org/10.1016/j.rser.2013.08.006
- Mundial, B. (2018). Doing business 2018: Reforming to create jobs. A world bank group flagship report. The World Bank.
- Narayan, P. K. (2005). The saving and investment nexus for China: Evidence from cointegration tests. Applied Economics, 37(17), 1979–1990. https://doi.org/10.1080/00036840500278103
- O’Connell, P. G. (1998). The overvaluation of purchasing power parity. Journal of International Economics, 44(1), 1–19. https://doi.org/10.1016/S0022-1996(97)00017-2
- Ogbonnaya, C., Abeykoon, C., Damo, U. M., & Turan, A. (2019). The current and emerging renewable energy technologies for power generation in Nigeria: A review. Thermal Science and Engineering Progress, 13, 100390. https://doi.org/10.1016/j.tsep.2019.100390
- OPEC, (2018). Annual statistical bulletin. Organisation of Petroleum Exporting Countries (OPEC), Geneva, Switerland
- Pesaran, H. M. (2004). General diagnostic tests for cross-sectional dependence in panels. University of Cambridge, Working Papers in Economics, 435.
- Pesaran, M. H. (2006). Estimation and inference in large heterogeneous panels with a multifactor error structure. Econometrica, 74(4), 967–1012. https://doi.org/10.1111/j.1468-0262.2006.00692.x
- Pesaran, M. H. (2007). A simple panel unit root test in the presence of cross‐section dependence. Journal of Applied Econometrics, 22(2), 265–312. https://doi.org/10.1002/jae.951
- Pesaran, M. H., Shin, Y., & Smith, R. P. (1997). Estimating Long-run Relationships in Dynamic Heterogeneous Panels. DAE Working Papers Amalgamated Series, 9721.
- Pesaran, M. H., Shin, Y., & Smith, R. P. (1999). Pooled mean group estimation of dynamic heterogeneous panels. Journal of the American Statistical Association, 94(446), 621–634. https://doi.org/10.1080/01621459.1999.10474156
- Pesaran, M. H., Shin, Y., & Smith, R. J. (2001). Bounds testing approaches to the analysis of level relationships. Journal of Applied Econometrics, 16(3), 289–326. https://doi.org/10.1002/jae.616
- Pesaran, M. H., & Smith, R. (1995). Estimating long-run relationships from dynamic heterogeneous panels. Journal of Econometrics, 68(1), 79–113. https://doi.org/10.1016/0304-4076(94)01644-F
- Rafindadi, A. A., & Yosuf, Z. (2013). An application of panel ARDL in analysing the dynamics of financial development and economic growth in 38 sub-Saharan African continents. In Proceeding-Kuala Lumpur International Business, Economics and Law Conference Vol. 2. December 2 - 3, 2013. Hotel Putra, Kuala Lumpur, Malaysia
- Raissi, M. M., & Anderson, G. (2018). Corporate Indebtedness and Low Productivity Growth of Italian Firms. International Monetary Fund. No. 2018/033
- Rennings, K., Brohmann, B., Nentwich, J., Schleich, J., Traber, T., & Wüstenhagen, R. (Eds.). (2012). Sustainable energy consumption in residential buildings (Vol. 44). Springer Science & Business Media.
- Samuel, Y. A., Manu, O., & Wereko, T. B. (2013). Determinants of energy consumption: A review. International Journal of Management Sciences, 1(12), 482–487.
- Sarkodie, S. A., & Adom, P. K. (2018). Determinants of energy consumption in Kenya: A NIPALS approach. Energy, 159, 696–705. https://doi.org/10.1016/j.energy.2018.06.195
- Sbia, R., Shahbaz, M., & Hamdi, H. (2014). A contribution of foreign direct investment, clean energy, trade openness, carbon emissions and economic growth to energy demand in UAE. Economic Modelling, 36, 191–197. https://doi.org/10.1016/j.econmod.2013.09.047
- Shafiei, S., Salim, A. R., & Cabalu, H. (2013). The nexus between energy consumption and economic growth in OECD countries: A decomposition analysis. Unpublished results.
- Shahbaz, M., Nasreen, S., Ling, C. H., & Sbia, R. (2014). Causality between trade openness and energy consumption: What causes what in high, middle and low income countries. Energy Policy, 70, 126–143. https://doi.org/10.1016/j.enpol.2014.03.029
- Shurig, S. (2015). Who will fund the renewable solution to the energy crisis? The Guardian. Accessed 08 09 2015. http://www.theguardian.com/global-development-professionalsnetwork/2014/jun/05/renewable-energy-electricty-africa-policy
- Sineviciene, L., Sotnyk, I., & Kubatko, O. (2017). Determinants of energy efficiency and energy consumption of Eastern Europe post-communist economies. Energy & Environment, 28(8), 870–884. https://doi.org/10.1177/0958305X17734386
- Smil, V. (2005). Energy at the Crossroads. Retrieved April, 29, 2011. In Global Perspectives and Uncertainties (pp. 443). The MIT Press.
- Stern, D. I. (2010). The role of energy in economic growth. USAEE-IAEE Working Paper, (10–055).
- Tang, C. F., & Tan, B. W. (2014). The linkages among energy consumption, economic growth, relative price, foreign direct investment, and financial development in Malaysia. Quality & Quantity, 48(2), 781–797. https://doi.org/10.1007/s11135-012-9802-4
- USEIA. (2016). United States Energy Information Administration. United States Natural Gas Industrial Price, Annual.
- Varian, H. R. (1992). Microeconomic analysis (Vol. 3). Norton.
- Waheed, R., Sarwar, S., & Wei, C. (2019). The survey of economic growth, energy consumption and carbon emission. Energy Reports, 5, 1103–1115. https://doi.org/10.1016/j.egyr.2019.07.006
- Wang, Q., & Su, M. (2019). The effects of urbanization and industrialization on decoupling economic growth from carbon emission–a case study of China. Sustainable Cities and Society, 51, 101758. https://doi.org/10.1016/j.scs.2019.101758
- Wang, Q., & Wang, L. (2020). Renewable energy consumption and economic growth in OECD countries: A nonlinear panel data analysis. Energy, 207, 118200. https://doi.org/10.1016/j.energy.2020.118200
- Westerlund, J. (2005). Data dependent endogeneity correction in cointegrated panels. Oxford Bulletin of Economics and Statistics, 67(5), 691–705. https://doi.org/10.1111/j.1468-0084.2005.00137.x
- Westerlund, J. (2007). Testing for error correction in panel data. Oxford Bulletin of Economics and statistics, 69(6), 709–748.
- Wolde-Rufael, Y. (2006). Electricity consumption and economic growth: A time series experience for 17 African countries. Energy Policy, 34(10), 1106–1114. https://doi.org/10.1016/j.enpol.2004.10.008
- World Bank. (2018). The World Bank Economic Review. Washington, D.C: World Bank. World Bank
- Xiong, W., Yan, L., Wang, T., & Gao, Y. (2020). Substitution effect of natural gas and the energy consumption structure transition in China. Sustainability, 12(19), 7853. https://doi.org/10.3390/su12197853
- Zaharia, A., Diaconeasa, M. C., Brad, L., Lădaru, G. R., & Ioanăș, C. (2019). Factors influencing energy consumption in the context of sustainable development. Sustainability, 11(15), 4147. https://doi.org/10.3390/su11154147
- Zhang, C., Zhou, K., Yang, S., & Shao, Z. (2017). On electricity consumption and economic growth in China. Renewable and Sustainable Energy Reviews, 76, 353–368. https://doi.org/10.1016/j.rser.2017.03.071