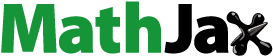
Abstract
The adoption of modern agricultural technologies remains to be a promising strategy to improve agricultural productivity, achieve food security and reduce poverty in Ethiopia. Despite the efforts to promote adoption in the country, the adoption rate has always been very low. So, it is essential to understand the determinants to the adoption of modern agricultural technologies. This study investigates the determinants of agricultural technology adoption decisions in the rural Amhara region of Ethiopia. The study is based on an Ethiopian socio-economic survey of 2015/16 and a sample of 656 farm households is considered. The paper uses a multinomial logit model to assess the factors affecting the adoption decision of agricultural technology. The result shows that farmers with more educational level, family size, off-farm participation, livestock, extension service, credit access, advisory service, and farmers closer to plot, all-weather road, zonal town, and farmers with lower remittance income are more likely to adopt new or improved agricultural technology. Accordingly, the study provides crucial policy implications regarding the technology adoption in the agricultural sector for all regions of Ethiopia.
PUBLIC INTEREST STATEMENT
In our country Ethiopia, where agriculture is at the heart of the economy, the adoption rate of agricultural technology has always been very low. Thus, this study tried to examine the determinants of multiple agricultural technology adoption so as to raise the productivity of the agricultural sector. The result reveals that educational level, family size, off-farm participation, livestock, extension contact, credit access, advisory service, and farmers closer to plot, all-weather road, zonal town, and farmers with lower remittance income are the determinants of multiple agricultural technology adoption in Amhara Region.
1. Introduction
In most developing countries, agriculture is the main stay of their economy. Agricultural development helps improve the welfare of rural households such as poverty reduction, achieve food security and environmental sustainability. The various manifestations of poverty are found disproportionately in rural areas because of low income, vulnerability to shocks, poor infrastructure facilities, political marginalization, and exposure to the degradation of natural resources. This implicates the need for improving agricultural productivity at farm level, and thereby improving farmer’s welfare (McCalla, Citation2001; Admassie & Ayele, Citation2010; Jayne et al., Citation2017). Furthermore, agriculture, with its high contribution to country’s GDP, exports, and employment, it is an essential motor of growth in most developing countries.
According to Wik et al. (Citation2008), growth originating in agriculture is about four times more effective in reducing poverty than other sectors. For this reason, policies that increase agricultural productivity can have a significant impact on poverty reduction. This is possible if and only if modern agricultural technologies are properly transferred and diffused so as to increase productivity. The green revolution introduced high yield varieties, fertilizers, pests, and others in developing countries, but the take up of these technologies in many developing countries has been uneven and have a low rate. In many areas traditional farming practices still dominate and the take up of the new technologies remains limited (De Janvry et al., Citation2017). This is true for Sub-Saharan African countries where the agricultural sector dominates and is characterized by low productivity due to the low rate of technology adoption (Asfaw et al., Citation2012; Feder & Savastano, Citation2017).
Agriculture is the main sector of the Ethiopian economy; it accounts for about 34.1% to the GDP, employs 79% of the population, accounts for 79% of foreign exchange earnings, it is the major sources of raw material and capital for investment and provides large market (Diriba, Citation2020). However, the share of the agriculture sector to GDP is decreasing overtime due to the sector is constrained by reliant on rainfall, traditional farming methods and minimal application of modern agricultural inputs (Belay & Mengiste, Citation2021). As a result, food production shortage, food insecurity and massive poverty are still the major development challenges in the country. For example, based on a $1 per day international poverty line 26.3% of the country’s population is estimated to be poor; and in $2 per day standard it increases to 80.7% (National Planning Commission, Citation2017).
Following this, the country has been implementing different extension approaches to promote the adoption of improved seed, fertilizer, pesticides, and improved agricultural practices (Tefera et al., Citation2016). Since land is scarce in the country; the feasible way to improve agricultural productivity in the country is through adoption of modern agricultural technology (Mohammed, Citation2014). Nevertheless, agricultural technology adoption remains very low in the country despite the fact that adoption has a direct impact on increasing yield and income generation as well as nutrition level. For instance, in 2014/15 cropping period, adoption rate of fertilizers, improved seed, pesticides and irrigation were 55.06%, 8.55%, 22.32% and 6.15%, respectively (Shita et al., Citation2018). Therefore, this point out that more works need to be done to make sure that farmers recognize agricultural technologies as vital contributor of agricultural productivity and confirm better adoption at the farm level.
In Ethiopia, different studies have been conducted on the determinants of adoption of agricultural technology; for example (Kassie et al., Citation2010; Admassie & Ayele, Citation2010; Mulugeta & Hundie, Citation2012; Asfaw et al., Citation2012; Hailu et al., Citation2014; Sebsibie et al., Citation2015; Amare, Citation2018; Wudu, Citation2017; Gebru, Citation2016; Worku, Citation2019; Natnael, Citation2019; Ayenew et al., Citation2020; Feyisa, Citation2020; Massresha et al., Citation2021; Tamirat & Abafita, Citation2021). They found that agricultural technology adoption decisions are influenced by factors of demographic, socioeconomic, institutional and plot characteristics. However, many of the aforementioned studies show the determinants of single agricultural technology adoption decisions. Hence, none of them showed farmers’ decision to adopt multiple agricultural technologies. In reality, the majority of the farmers are adopting a single and a combination of technologies. Thus, this paper investigates the determinants of adoption decisions of multiple agricultural technologies by considering adopters of at least one and more technologies in any of one of the crop land. Moreover, many of the studies conducted are using a few explanatory variables and covers a small sample and area coverage. However, this study includes several explanatory variables and covers a large sample and area than the aforementioned studies.
Furthermore, this study is conducted in Amhara regional sate of Ethiopia. In the region agriculture is the primary economic sector, in which nearly 84% of the population lives in rural areas. The most common agricultural activities practiced in the region are crop production, plantation, animal husbandry, forestry and logging, and fishing. To improve the livelihood of farming households, the application of modern agricultural technologies was taken as a measure throughout the region. Although there is a great focus on the application of agricultural technologies, the adoption rate of the region is quite low in the region, even as compared to Oromia and southern nation nationalities and peoples regions of Ethiopia and the use is still lower than the recommended rates (Tefera et al., Citation2016; Zegeye, Citation2021). Therefore, it is meaningful to investigate the factors of adopting agricultural technologies in the Amhara region. However, there are no enough studies conducted on the factors of adopting agricultural technologies in the region. Thus, this study investigates the determinants of adoption decisions of multiple agricultural technologies in Amhara Region, Ethiopia.
The rest of the paper is structured as follows: Section 2 provides methodology section that describes data and econometric analysis used in the study. Section 3 presents result and discussion of the study, and section 4 presents conclusion and recommendations of the study.
2. Methodology
2.1. Data description
The data for this study were obtained from the Ethiopian Socio-economic Survey (ESS) during 2015/16. The survey covers all the regions of Ethiopia; however, the data is argued to be representative for regional estimation in the most populous regions of Tigray, Amhara, Oromia, and Southern Nation and Nationality people’s regional state. The survey covered a wide range of topics such that it covers a range of topics including demography, education, health, labor, welfare, agriculture, food security, and shocks. The survey meets Ethiopia’s data demand gaps and is believed to be of high quality, and accessible to the public. The data cover rural areas and urban areas (small towns, medium and large towns); however, in this study households from urban areas were omitted for the reason of the non-applicability of agricultural activities. Then, this study considered merely rural farm households found in the Amhara regional state of Ethiopia. Consequently, the study considered 656 farm households drawn from rural Amhara regional state as a sample of this study.
2.2. Description and measurement of variables
Adoption in this study refers to a farm household, which used at least one technology (such as inorganic fertilizer (includes Urea and Dap), organic fertilizer (includes manure), herbicide and their combinations in any one of the crop fields. Those three technologies are selected based on the higher adoption rates in the region. Thus, the dependent variable is adoption of multiple agricultural technologies and is estimated using multinomial logit model. The description and measurement of variables used in the study are presented in Table as follows.
Table 1. Description of and Measurement of Variables used in the Study
2.3. The conceptual framework and estimation strategy
2.3.1. Modeling multiple technology adoption
The adoption of multiple agricultural technologies can be modeled in the setting of a framework. The adoption variable for this study is generated from the combinations of the organic fertilizer, inorganic fertilizer and herbicide technologies. The estimations of the adoption of alternative agricultural technology packages are estimated using Multinomial Logit model.
2.3.2. Multinomial adoption selection model
Following (Verbeek, Citation2004; Gillespie et al., Citation2014; Kassie et al., Citation2018) the choice of alternative agricultural technologies is made according to the expected benefits from the adoptions of specific choices given their limitations. The common starting point is a random utility framework, in which the utility of each alternative is a linear function of observed characteristics plus an additive error term. Economic theory dictates that farmers adopt a single or a combination of technologies that can maximize their utility. This implies adoption occurs if the utility of the chosen package is higher than the utility of the other alternatives. However, the utility that gained from adopting agricultural technology is not observed but only its choice of technology, one can assume a random utility model which states conditional probability choice given farmers choice. In most case, farmers are risk averse to adopt agricultural technology. This can be explained as; risk averse farmers tend to be more cautious individuals with preferences for less risky adoptions. But, a risk averter does not refuse to accept any risk at all. However, the risk averse farmer would seek to be compensated for the risk taken by receiving a higher return than would normally be obtained if there were no risk. Assuming that individual farmers are economically rational and maximize profits; a risk averse farmer probably compares all potential profits from alternative practices and then makes a decision about adoption. To formalize this, consider the following latent variable:
where: Aij* is a latent variable which describes the ith farmer’s behavior in adopting the alternative package of technology J (j = 1, 2, … … .m) with respect to another alternatives K. Z’s are a vector of observed independent variables (household characteristics, farm-level factors, institutional factors, biophysical factors and technology aspects) and are unobserved characteristics which are relevant to the farm household’s decision-maker but are unknown to the researcher.
The farm household i will choose a package of j-technologies with respect to adopting any other technologies of k if it provides greater expected utility than any other alternative k, k ≠ j, i.e. if =
(
*—
*) < 0.
It is assumed that the covariate vector of Zi is uncorrelated with the unobserved error term , i.e.
. Assuming that
are independent and Gumbel (identically) distributed (independence across utility functions and identical variance), that is under the independence of irrelevant alternatives (IIA) hypothesis; this model leads to the selection of a multinomial logit model.
2.3.3. Multinomial logit model (MNL)
Under adoption of multiple agricultural technologies, the number of alternatives that can be chosen is more than two; we can apply the multinomial discrete choice model to estimate simultaneously the effects of the explanatory variable on the adoption of different agricultural technologies. The variable Aij is a multiple-choice variable and can be consistently estimated using a limited dependent variable (Maddala, Citation1983). There are two mostly usable multinomial probabilistic choice models, namely multinomial logit (MNL) and probit (MNP). According to (Greene, Citation2003) there is a slight distinction between MNL and MNP models because of the need to evaluate multiple integrals of the normal distribution, the MNP model has found rather limited use in this setting and the estimated results are not sufficient to allow accurate and efficient evaluation for more than two variables in a sample of even moderate size, but the MNL model, in contrast, has been widely used in many fields. According to Kropko (Citation2008) and Dow and Endersby (Citation2004) technically, these models are very similar, they differ only in the distribution of errors terms. MNL has errors which are independent and identically distributed, while MNP has errors which are not necessarily independent, and distributed by a multivariate normal distribution. With multiple integrals, MNP fails to converge or provide a useful estimation at all. Meanwhile choice probabilities are relatively simple in MNL, even for a large number of choices. In this regard, this study applied the MNL model over other since the model is simple to calculate the choice probability and computers can maximize the resulting likelihood function even for a large number of choices. The results obtained from the model is more stable than others when IIA assumption fulfilled. Kropko (Citation2008) also shows the multinomial logit model nearly always provides more accurate and realistic results than others even if the Independent of Irrelevant Alternatives (IIA) assumption is severely violated. The model assumes that the possible distinct states are exhaustive in that they cover all possibilities.
In the model specification, the non-adoption is considered the base level and all the logit are made relative to the base category. When category k is taken as a base category, and let be the multinomial probability of an observation falling in the jth category, then the MNL model is specified as follows:
where, Yi is Adoption choice/decision, βi is the vector of parameters and Xi is a vector of all explanatory variables that can have influence on the adoption decisions of multiple agricultural technologies.
3. Results and discussion
3.1. Descriptive statistics
The eight possible agricultural technology packages are summarized in . Of the total 656 sampled farm households in the Amhara region, about 21.04% are non-adopters (F0M0H0), whereas 7.77%, 17.84% and 2.74% of them adopted only one technology (F1M0H0, F0M1H0, and F0M0H1) respectively, and 19.82%, 8.84% and 3.35% of them are adopted a combination of two technology packages (F1M1H0, F1M0H1, and F0M1H1) respectively, and lastly 18.6% of the farm households simultaneously adopted all of the three packages (F1M1H1). The result shows that package of F1M1H0 and F1M1H1, followed by F0M1H0 have the higher frequencies that may indicate that farmers expected utility derived from those could be higher than the rest of the technology packages ().
Table 2. Hypothesis explanation and expected sign of the variables used in the study
Table 3. Alternative Adoption of Agricultural Technology
Table 4. Description of Alternative Technology Packages
The descriptive statistics of explanatory variables for the eight combined alternative packages considered in this study are presented in Table . The explanatory variables’ mean value of non-adopters (F0M0H0) is used as a base category to compare with mean values of alternative adopters (F1M0H0, F0M1H0, F0M0H1, F1M1H0, F1M0H1, F0M1H1 and F1M1H1).
Table 5. Summary of Descriptive Statistics
The table revealed that, in the Amhara region, a set of household characteristics like sex (adopters are more of male headed), regarding education, adopters have high education level. Moreover, on average adopters have large family size than the non-adopters. On the other way, the mean age of the household head for adopters is lower than the non-adopters. Total farm size, remittance income, livestock wealth measured in TLU, and off-farm employment are used as a means to describe the economic status of the household. Average farm size for non-adopter is lower than adopters (except for herbicide adopters). The mean livestock wealth and the proportion of households with off-farm activity participation for adopters are higher than non-adopters. On average, remittance income of the household is larger for non-adopters (F0M0H0).
As the table shows, the mean distance from road, market and zonal town are larger for non-adopters. But adopters of manure, herbicide and a combination of the two packages, are higher than non-adopters indicating that as the distance increases farmers adopt the kind of technology that are easy to carry and nearby available technologies so as to reduce transportation costs.
Institutional factors such as extension service, advisory service, credit access, and tenure security are other factors that affect the adoption decision. The first two are related with farmers’ access to information on different packages and its profitability while access to credit indicates farmers’ ability to finance their purchase of modern technology under cash constraints. The result shows these supports are higher for adopters. This may indicate that the institutional support system has long been a major factor for modern agricultural technology adoption even if its support has remained low (Kassie et al. (Citation2010). Furthermore, adopters have significantly higher mean values in terms of microclimate indicators like plot potential wetness index, and lower mean values in terms of plot distance from homestead. Similarly, the proportion of non-fertile soil plot is significantly higher for non-adopters than adopters. To sum up, the result shows that the mean comparison test between adopters and non-adopters are significantly larger for adopters and the values are different across the different alternatives. provides hypothesis explanation and expected sign of the variables used in the study.
3.2. Econometric analysis
In econometric analysis, the study applies a method of analysis of maximum likelihood estimation technique for the purpose of estimating the multinomial logit functions. The result from the multinomial logit model is presented in Table . The base category is non-adoption (F0M0H0), where results of alternative packages are compared. The model fits the data reasonably well. Various post estimation tests were made to check the validity of the model. The Wald test is used to ensure that all regression coefficients are jointly equal to zero is rejected with [χ2(133) = (478.82); P >0.000]. The Hausman test result for test of IIA assumption confirms that all the alternative packages are distinguishable with respect to the variables in the model. VIF for continuous and correlation matrix for categorical variable also have been computed to test multicollinearity problem, and the result indicates that there is no serious multicollinearity problem across the explanatory variables. And finally, robust regression has used to control the problem of heteroscedasticity and non-normality.
Table 6. Maximum likelihood estimates for the multinomial logit model
3.3. Determinants of adoption of multiple agricultural technology
Based on this estimation, the result indicates that the sign of age of household head is negative and significant, indicating that young farmers in the Amhara region are more likely to adopt farm technology since young farmers may have formal education than the non-adopter, less risk averse, more willing, and have greater flexibility in accepting new ideas, other things being constant. This is consistent with (Admassie & Ayele, Citation2010; Ayenew et al., Citation2020). Similarly, education level of the head has positive sign and significant for F1M1H0, which is similar with the finding of (Asfaw et al., Citation2012; Kassie et al., Citation2010; Massresha et al., Citation2021). This is because farmers in the Amhara region with more educational years are more likely to adopt as they able to acquire, analyze and evaluate information on modern technology, market opportunity and its implied benefit.
The family size of farmers has a positive and significant effect on the adoption decision of manure and a combination of manure with herbicide packages. This implies the more family member of the household the more the adoption will be, because adoption of multiple farm technologies requires and attracts more labor force for agricultural activities (Kassie et al., Citation2010; Zegeye, Citation2021).
In the Amhara region, livestock asset measured by tropical livestock unit (TLU) significantly and positively affects the adoption decision of multiple technologies. This is because of farmers who possess a flock of livestock are more likely to adopt than the have-not as it helps to get improved technology (as income means and source of manure input), consistent with Mulugeta and Hundie (Citation2012) and Massresha et al. (Citation2021). Farm households who participate in off-farm activity are also more likely to adopt chemical fertilizer and herbicide packages. This is because of participating in off-farm activities can generate income and solve the problem that the farm household’s face while intending to purchase farm technologies, consistent with (Kassie et al., Citation2010; Mulugeta & Hundie, Citation2012; Ayenew et al., Citation2020).
The other important factor is farm remittance income, and the result shows that the impact of remittance income on the adoption decision is negative and significant. The descriptive analysis also supports this finding. From the descriptive analysis it was found that the remittance income in the Amhara region is higher for non-adopter households. One plausible reason for this is the household’s way of spending the money from remittance. Most often income from remittance will be used for daily consumption purpose than investing in agricultural development. This is supplemented by Tuladhar et al. (Citation2014) and Zegeye (Citation2021). The higher remittance inflows to households, and subsequently, the higher income buffer, might have increased the opportunity cost of engaging in agriculture, resulting in reliance on remittance income more than the income from the agriculture sector.
Distance to market, road and zonal town are significant proxy variables which capture the relationship between access to market information, access to input and farm households’ technology adoption. Distance to zonal town has a strong and negative effect on the adoption of package F1M0H0, F1M1H0, F1M0H1, and F1M1H1. Distance to all weather roads has also negative effect on the adoption of package F1M1H1. This result may verify that those framers in the Amhara region who live away from service centers such as urban centers, development agent, and market place are less likely to adopt farm technologies because of farmers could have less access to information on improved technologies and high production cost, and hence are unlikely to adopt new or improved technology; similar with (Admassie & Ayele, Citation2010; Hailu et al., Citation2014; Zegeye, Citation2021; Belay & Mengiste, Citation2021). However, distance to road and zonal town affects positively the adoption of package F0M1H0 and F0M0H1 respectively. This is because as the road distance is longer, farmers would adopt nearby available technologies like organic fertilizer and easy to carry type technologies like herbicide to minimize the production costs. This means that in the adoption decision, the farm household would be beneficial if their adoption is cost-effective (Mesele, Citation2019; Mohammed, Citation2014).
Institutional support factors such extension service, advisory service, credit access and tenure security are considered equally important to understand farm household’s technology adoption decision. That is, access to extension service and advisory service has a positive and significant relation on the adoption of alternative technologies. This is because the extension service provided by the local government helps the farmers to raise their awareness about the characterization and attributes of the technology, use and their impact. Extension gives detailed information, training and advisory services about the source, use and importance of the technologies to the farmers and engaging in input distribution. Advisory services, on the other hand, indicate that having access to regular and frequent advisory services by development agents, farm cooperatives and meetings plays a fundamental role in the dissemination and adoption of farm technology. Access to credit has a positive impact on the adoption decision of different packages. This is because of credit access solves income problems that household could face while they want to purchase agricultural technologies; and hence paves the way for timely application of modern farm inputs, and this is consistent with (Tefera et al., Citation2016; Sebsibie et al., Citation2015; Massresha et al., Citation2021; Zegeye, Citation2021; Belay & Mengiste, Citation2021).
The positive and significant effect of tenure security for a combination of chemical and manure fertilizer shows that when farmers are secured ownership right for their own land, the more likely in the adoption practice since they can make long-term investment, and the finding is in line with (Mohammed, Citation2014; Zegeye, Citation2021).
Plot characteristics and microclimate variables in the Amhara region are another set of factors considered in explaining household’s likelihood of technology adoption, such as soil quality, plot wetness and plot distance. Plot distance from the homestead is negatively affects the adoption of package F0M1H0, and F1M1H0. This is because of as plot is far away from the homestead, the less likely will be on time plot preparation, weed, harvest and input utilization and hence farm households are less likely to adopt, confirmed with Kassie et al. (Citation2010), Hailu et al. (Citation2014), and Zegeye (Citation2021). On the other hand, also, plotting potential wetness index has a positive impact on the probability of the adoption of full packages (F1M1H1). This is because of as the wetness of the plot increases (maintains vascular plant species richness, soil pH, groundwater level and soil moisture) the more likely the adoption of the agricultural technology. This is in line with the finding of (Sebsibie et al., Citation2015; Zegeye, Citation2021). Most of the explanatory variables like sex of the family head distance to market, farm size and soli quality, even if they are not statistically significant, they have the expected signs with the adoption decision.
4. Conclusion and policy recommendation
In Ethiopia, where agriculture is the mainstay of the economy, and where severe poverty is the main challenge, reducing poverty is the preliminary concern. Therefore, boosting production and productivity of agriculture through the use of improved agricultural technology is considered as a major solution. The country is underway of implementing agricultural technologies starting from the imperial regime up to now. Despite the efforts to promote adoption in most of the rural areas of Ethiopian farmers, the adoption rate in the country is very low and holds true for the Amhara region. Understanding the factors that influence or hinder adoption of agricultural technology is essential in planning and implementing yield and productivity enhancing technologies for meeting the challenges of poverty, food insecurity and food production in the country. Thus, the main objective of this study was to explain factors affecting the adoption of multiple farm technologies in rural Amhara region of Ethiopia. The study takes data from the Ethiopian socio-economic survey of collected in 2015/16. A total of 656 farm households were considered for a sample of the study. To assess the determinants of adoption the study employed multinomial logit model.
The result of the study revealed that farm household’s in the Amhara region decision to adopt improved or new farm technologies is manly influenced by factors of socioeconomic, institutional, access to information and distance factors, and plot characteristic. More specifically, the adoption of multiple agricultural technologies is positively and significantly affected by education level of the head, household size, off-farm activity, livestock wealth, farmers contact with extension and advisory service, farmers having credit access, farmers secured for their own land, and plot potential wetness index. However, the adoption of farm technologies is negatively and significantly affected by age of the family head, distance from zonal town, road and plot distance from the homestead, and farmers getting remittance income. However, the effect of distance from road and zonal town for the adoption of herbicide and manure technologies is positive, indicating as the farm lives far away from urban centers and development agents, farmers adopt nearby available technologies and easy to carry type technologies so as to reduce their cost of production.
Thus, in terms of policy implication, the federal and regional government of the country, agricultural development office, non-governmental organizations, and donor agencies should work in collaborations with the farm households. Specifically, policies for strengthening the access to information about the farm technologies by increasing the availability and quality of extension service, encouraging the participation of farmers in training centers and providing advisory service to increase the adoption by farm households. The influence of extension service, trainings and advisory services can counter balance the negative effect of lack of years of formal education on the overall decision to adopt farm technologies. Thus, polices for strengthening the extension service and advisory service delivery capabilities through technical support and short and long-term training programs are important in adoption decisions.
The study also found that distance of the household’s residence from the main road, market centers and zonal town is another important factor influencing the adoption of a technology. The closer the household is to market centers, zonal town and all weather road the better it would be to access information about technology and prices and hence is positively related to technology adoption. This implies that the access of these infrastructures increase the agriculture technology adoption decision by the farm households. Thus, the government and other stakeholders should support the access of these infrastructural services.
Access to credit is found to be a highly significant variable that positively determines the probability of adoption of alternative farm technology packages. This may call for the need and expansion of microfinance institutions in rural areas of the local government and Ethiopia where financial constraint is a major challenge for farmers while in adopting technology. This may reveal that adoptions of farm technologies are capital intensive: thus, credit should be accessible for the rural poor.
Lastly, the effect of remittance income on technology adoption is negative. Therefore, the study recommends the different stakeholders aimed at channeling remittances to finance agricultural capital and inputs that increase agricultural productivity is required. The government should have to make awareness to the recipients and to the sender to have a well-managed remittance use and to involve more effectively on the agricultural sector. This could help the government of Ethiopia to implement policies to enhance skills of migrants so that migrants remit more income to households, ultimately enabling them to acquire more productivity enhancing capital assets even after discounting for the high share of consumption expenditure.
Currently, the farm households in the Amhara region are acknowledged the role of agricultural technologies and their adoptions are constrained by factors of household characteristics, infrastructure, institutional and plot characteristics. Therefore, the government should ensure better targeting of agricultural technology adoption and appropriate rural institutions on rural farmers to increase farm technology adoption by the rural poor so as to understand farmers need as well as their ability to adopt technology in order to come up with technology that will suit them.
As the study used a cross-sectional data, it is limited to show the time effect of adopting agricultural technologies in the study area. Further research is recommended for investigating the determinants of agricultural technology adoption decisions by using panel data (with long-time dimension) to capture the real and long-lasting determinants of adoption decisions. The study gives only a focus on demand-side factors. Additionally, this study is also recommended for examining the determinants of adoption decisions of agricultural technologies including the supply-side related factors.
Acknowledgements
We would like to thank the editor and anonymous reviewers for the supportive comments and suggestions.
Disclosure statement
No potential conflict of interest was reported by the author(s).
Additional information
Funding
Notes on contributors
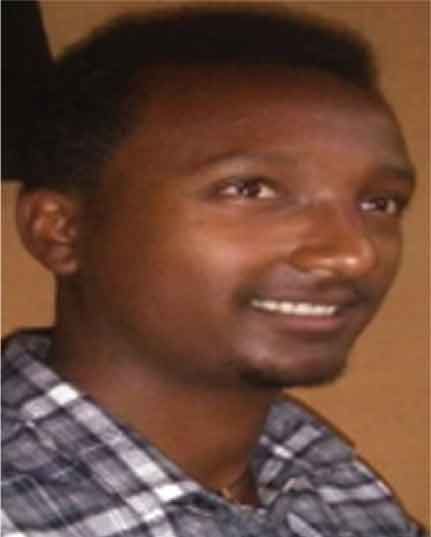
Mesele Belay Zegeye
Mesele Belay Zegeye, the author of this article is a lecturer at Debre Berhan University, Department of economics, Debre Berhan, Ethiopia. He earned his MSc degree in Development Economics and BA degree in Economics from Debre Berhan University. His research interest includes Effect, Impact assessment of policies, poverty, food security, technology adoption, and agricultural growth.
Notes
1. Adoption refers a farm household who used at least one technology in one of crop fields.
References
- Admassie, A., & Ayele, G. (2010). Adoption of improved technology in Ethiopia. Ethiopian Journal of Economics, 19(1), 155–23 doi:10.22004/ag.econ.258726.
- Amare, Y. (2018). Determinants of adoption of wheat row planting: the case of wogera district, North Gondar Zone, Ethiopia. IJIRMPS-International Journal of Innovative Research in Engineering & Multidisciplinary Physical Sciences, 9(250)().2
- Asfaw, S., Kassie, M., Simtowe, F., & Lipper, L. (2012). Poverty reduction effects of agricultural technology adoption: A micro-evidence from rural Tanzania. Journal of Development Studies, 48(9), 1288–1305. https://doi.org/10.1080/00220388.2012.671475
- Ayenew, W., Lakew, T., & Kristos, E. H. (2020). Agricultural technology adoption and its impact on smallholder farmers welfare in Ethiopia. African Journal of Agricultural Research, 15(3), 431–445. https://doi.org/10.5897/AJAR2019.14302
- Belay, M., & Mengiste, M. (2021). The ex‐ post impact of agricultural technology adoption on poverty: evidence from north shewa zone of Amhara region, Ethiopia. International Journal of Finance & Economics, 1–11 doi:10.1002/ijfe.2479.
- De Janvry, A., Macours, K., & Sadoulet, E. (2017). Learning for adopting: Technology adoption in developing country agriculture (Ferdi) p. 120 .
- Diriba, G. (2020). Agricultural and rural transformation in Ethiopia: obstacles. Triggers and Reform Considerations.
- Dow, J. K., & Endersby, J. W. (2004). Multinomial probit and multinomial logit: A comparison of choice models for voting research. Electoral Studies, 23(1), 107–122. https://doi.org/10.1016/S0261-3794(03)00040-4
- Feder, G., & Savastano, S. (2017). Modern agricultural technology adoption in sub-Saharan Africa: A four-country analysis. In Agriculture and rural development in a globalizing world (pp. 11–25). Routledge.
- Feyisa, B. W. (2020). Determinants of agricultural technology adoption in Ethiopia: A meta-analysis. Cogent Food & Agriculture, 6(1), 1855817. https://doi.org/10.1080/23311932.2020.1855817
- Gebru, A. (2016). The Determinants of Modern Agricultural Inputs Adoption and Their Productivity in Ethiopia (The Case of Amhara and Tigray Regions) (thesis, Addis Ababa University).
- Gillespie, J., Nehring, R., & Sitienei, I. (2014). The adoption of technologies, management practices, and production systems in US milk production. Agricultural and Food Economics, 2(1), 1–24. https://doi.org/10.1186/s40100-014-0017-y
- Greene, W. H. (2003). Econometric Analysis (5th ed.). Pearson Education Inc.
- Hailu, B. K., Abrha, B. K., & Weldegiorgis, K. A. (2014). Adoption and impact of agricultural technologies on farm income: evidence from Southern Tigray, Northern Ethiopia. International Journal of Food and Agricultural Economics (IJFAEC), 2(1128–2016–92058), 91–106 doi:10.22004/ag.econ.190816.
- Jayne, T. S., Yeboah, K., & Henry, C. (2017). The future of work in African agriculture trends and drivers of change. International Labour Office.
- Kassie, M., Marenya, P., Tessema, Y., Jaleta, M., Zeng, D., Erenstein, O., & Rahut, D. (2018). Measuring farm and market level economic impacts of improved maize production technologies in Ethiopia: Evidence from panel data. Journal of Agricultural Economics, 69(1), 76–95. https://doi.org/10.1111/1477-9552.12221
- Kassie, M., Shiferaw, B., & Muricho, G. (2010). . Environment for Development Discussion Paper-Resources for the Future (RFF) (Resources for the Future), (10–11).
- Kropko, J. (2008). Choosing between multinomial logit and multinomial probit models for analysis of unordered choice data ((Doctoral dissertation, The University of North Carolina at Chapel Hill).).
- Maddala. (1983). Limited dependent and qualitative variables in Econometrics. Cambridge University Press.
- Massresha, S. E., Lema, T. Z., Neway, M. M., & Degu, W. A. (2021). Perception and determinants of agricultural technology adoption in north shoa zone, Amhara regional state, Ethiopia. Cogent Economics & Finance, 9(1), 1956774. https://doi.org/10.1080/23322039.2021.1956774
- McCalla, A. F. (2001). Challenges to world agriculture in the 21st century. J. Agricultural Resource and Economics, 4(3 1–2).
- Mesele, B. Z. (2019). The Impact of Agricultural Technology Adoption on Poverty Reduction in Rural Ethiopia ( thesis). Debre Berhan University- Health Science National Academic Digital Repository of Ethiopia. https://doi.org/10.20372/nadre/6315.
- Mohammed, A. (2014). Adoption of multiple sustainable agricultural practices and its impact on household income: evidence from maize-legumes cropping system of Southern Ethiopia. International Journal of Agriculture 4 , 196–203.
- Mulugeta, T., & Hundie, B. (2012). Impacts of adoption of improved wheat technologies on households’ food consumption in southeastern Ethiopia (No. 1007–2016–79620).
- National Planning Commission. (2017). Ethiopia’s progress towards eradicating poverty. an interim report on 2015/16 poverty analysis study.
- Natnael, A. B. (2019). Impact of technology adoption on agricultural productivity and income: A case study of improved teff variety adoption in north eastern Ethiopia. Agricultural Research & Technology: Open Access Journal, 20(4), 556139. https://doi.org/10.19080/ARTOAJ.2019.20.556139
- Sahu, S. K., & Das, S. (2016). Impact of agricultural related technology adoption on poverty: A study of select households in rural India. In Technology (pp. 141–156). Springer.
- Sebsibie, S., Asmare, W., & Endalkachew, T. (2015). Agricultural technology adoption and rural poverty: A study on smallholders in Amhara regional state, Ethiopia. Ethiopian Journal of Economics, 23(683–2017–950), 117–156 doi:10.22004/ag.econ.259413.
- Shita, A., Kumar, N., & Singh, S. (2018). Agricultural technology adoption and its determinants in Ethiopia: A reviewed paper. Asia Pacific Journal of Research, 1(55), 99–104.
- Tamirat, N., & Abafita, J. (2021). Adoption of row planting technology and household welfare in southern Ethiopia, in case of wheat grower farmers in Duna district, Ethiopia. Asia-Pacific Journal of Science and Technology, 26(2).
- Tefera, T., Tesfay, G., Elias, E., Diro, M., & Koomen, I. (2016 ()). Drivers for adoption of agricultural technologies and practices in Ethiopia-A study report from 30 woredas in four regions. Capacity Building for Scaling Up of Evidence-based Best Practices in Agricultural Production in Ethiopia Project Report No. NS_DfA_2016_1CASCAPE.
- Tuladhar, R., Sapkota, C., & Adhikari, N. (2014). Effects of migration and remittance income on Nepal’s agriculture yield (Asian Development Bank).
- Verbeek, M. (2004). A guide to modern econometrics. ERIM (electronic) books and chapters. John Wiley&Sons.
- Wik, M., Pingali, P., & Brocai, S. (2008). Global agricultural performance: Past trends and future prospects (Washington, DC: World Bank. © World Bank.).
- Worku, A. A. (2019). Factors affecting diffusion and adoption of agricultural innovations among farmers in Ethiopia case study of Ormia regional state Westsern Sewa. International Journal of Agricultural Extension, 7(2), 137–147. https://doi.org/10.33687/ijae.007.02.2864
- Wudu, B. (2017). Determinants of adoption of improved wheat technology: in case of Gozzamen district, east Gojjam in Amhara regional state, Ethiopia (thesis, St. Mary’s University)
- Zegeye, M. B. (2021). Adoption and ex-post impact of agricultural technologies on rural poverty: evidence from Amhara region, Ethiopia. Cogent Economics & Finance, 9(1), 1969759. https://doi.org/10.1080/23322039.2021.1969759