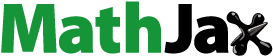
Abstract
This paper is a systematic attempt to establish the effect of anti-money laundering (AML) systems on FDI flows across the globe. Complex and related hypotheses are tested using data from 2012 to 2018 across 165 economies across different continents, income levels, and regulatory environments. First, the paper examines the effect of AML systems on FDI flows. Second, the paper examines the nonlinearities of the AML systems-FDI nexus. Third, the paper examines if host country peculiarities such as an offshore financial centre (OFCs) or originating from Africa alter this relationship. The paper employs the two-step system GMM and the dynamic panel threshold regression techniques to test the hypotheses of the study. Generally, the paper provides evidence that AML systems positively promote FDI inflows. However, the paper finds that AML structures dampen FDIs inflows for OFCs. Further, the paper finds that the influence of AML systems on FDIs is threshold-specific. Specifically, AML systems positively impact FDIs below the threshold for our full, developing, and African country samples. At the same time, the study finds a negative impact of AML structures on FDIs above the threshold value for our full sample and developing countries. However, for Africa, the study provides evidence of a positive impact of AML systems on FDIs across the different AML structures. Again, the study finds that AML systems negatively impact FDI across all AML structures for offshore financial centres. The findings of the study provide insights into the importance of AML systems in influencing MNEs’ FDI decisions to a country.
PUBLIC INTEREST STATEMENT
Money laundering is the process of disguising the origin of ill-gotten money to make it seem as though such funds were obtained from legitimate sources. Money laundering is said to undermine legitimate private-sector efforts, destabilize domestic markets, promote crime, corrupt the financial system, affect the reputation of countries etc. Therefore, anti-money laundering (AML) systems are the structures put in place to deal with the incidence of money laundering. We argue that anti-money laundering systems should influence the amount of foreign direct investment (FDI) into a country. However, AML systems might impede business processes and wealth creation. Further, AML compliance has become resource-intensive and expensive business for firms. Therefore, the study posits that FDI flows might be negatively impacted if AML systems/compliance become excessive. In this study, we extend the frontiers of knowledge by examining the existence of threshold effects in the AML systems-FDI flows nexus.
1. Introduction
Foreign Direct Investment (FDI) remains one of the most significant and most dependable capital inflows to developing countries. FDI is seen as an essential source of capital that complements domestic capital for development (UNCTAD, Citation2018). Consequently, FDI has become an essential alternative in the development finance process of nations (Global Development Report, Citation2005). FDI has remained a significant policy issue on the developmental agenda of especially developing countries given its tremendous contributions to economic growth via the introduction of new processes, managerial skills, technological transfers, and knowhow in the domestic market, international production networks, employee training, and international financial integration (Lee & Chang, Citation2009). Considering the importance of FDI to the development of nations, countries need renewed efforts to create the right atmosphere to attract the needed FDI.
Theoretical propositions, as well as empirical investigations, posit that host country characteristics largely influence FDI flows. According to the eclectic (OLI) paradigm of Dunning (Citation2009) on factors influencing FDIs, location variables such as the availability of natural resources, the quality of infrastructure, government restrictions in the form of legislation in the area of business, human resource capacity, and investment incentives are key variables influencing FDI decisions of Multinational Enterprises (MNEs). This suggests that governments creating the right atmosphere through deliberate policy direction can be an excellent incentive for MNEs’ FDI location decisions. Similarly, empiricists have shown that host country characteristics play a pivotal role in influencing FDI flows into countries (see, Agbloyor, Citation2019; Agbloyor et al., Citation2013; Al-khouri, Citation2015; Asiedu, Citation2006; Baah-Boateng, Citation2015; Felisoni de Angelo et al., Citation2010; Globerman & Shapiro, Citation2002; Saini & Singhania, Citation2018; Seetanah & Rojid, Citation2011; Wei, Citation2000).
Despite the preponderance of literature on the factors that influence FDI flows into a country, there appears to be conspicuously missing the effect of anti-money laundering (AML) systems on FDI flows. Money laundering has far-reaching consequences on the economies of nations around the globe. Money laundering impacts the economies of nations by undermining legitimate private-sector efforts, destabilizing domestic markets, distorting government budgets and government revenue through tax evasion, promoting crime, corrupting the financial system, undermining government institutions, fostering corruption that poses a national security risk to countries, among others (Baldwin, Citation2004; Loayza et al., Citation2019; McDowell & Novis, Citation2001; Van der Zahn et al., Citation2007). The United Nations estimates that the amount of money laundering occurring every year could range between 2 and 5 percent of the world’s gross domestic product- or somewhere between $1.6 trillion and $4 trillion (Weeks-Brown, Citation2018). This is an indication of the pervasiveness of money laundering across the globe.
One notable consequence of money laundering is its impact on the reputation of countries. Therefore, it has the possibility of affecting MNEs’ FDI location decisions. Although Dunning’s OLI paradigm posits that MNEs undertake FDI for resource, market, efficiency, and strategic asset-seeking motives (Dunning, Citation1980), in 2006, Dunning extended the OLI paradigm by acknowledging the critical role of institutional factors in influencing MNEs’ FDI decision (Dunning, Citation2006). Dunning (Citation2006) noted that due to globalization and the new paradigm of development, the content and quality of institutions are becoming essential components of both the competitive advantages of firms and the locational attractions of countries. Also, according to UNCTAD (Citation2018), companies increasingly look for investment locations offering the best conditions to rapidly deliver new and high-quality products. Aziz (Citation2018) posits that due to the high sunk cost associated with FDI, MNEs are reluctant to enter markets unless these markets have low levels of uncertainty and risk. Therefore, countries that plan to attract more foreign capital should provide an appropriate institutional environment for political stability, market efficiency, and property rights.
Again, countries cited for deficiencies in their AML framework or high money-laundering incidents are often blacklisted. This blacklisting indicates increased risk levels for international partners of such countries and it also increased transaction costs. Such blacklisting also slows down transactions as foreign firms are advised to adopt enhanced AML procedures in their dealings with such countries. Therefore, countries need to adopt a sound AML framework to attract more FDIs. Buckley et al. (Citation2010) noted that AML structures reduce uncertainty and risk associated with an investment as it promotes greater transparency in the business environment. Again, it creates competitive output markets, critical in MNEs’ FDI decisions. Nonetheless, Perez et al. (Citation2012) contend that a significant amount of FDI (i.e. 20%) is motivated by the desire to launder illicit funds. Therefore, implementing an effective AML framework may be a disincentive for such FDIs.
However, a review of extant literature suggests a dearth of literature on the influence of AML systems on FDI flows. In cross-country studies, (Mekpor et al., Citation2018) and Yepes (Citation2011) rather accessed the determinants of AML regulations/recommendations. Again, Adams and Opoku (Citation2015) accessed how regulations in the form of credit market regulations, business regulations, and labor market regulations influence FDI flows of 22 Sub-Saharan African countries. At the same time, Busse and Groizard (Citation2008) examined the effect of regulations in starting a business, labor market, contracts, creditor rights, and insolvency regulations on FDI flows. Agbloyor et al. (Citation2016) examined how institutional quality promotes the FDI-economic growth nexus under different environments. Agbloyor (Citation2019) examined the relationship between FDI, elections, and welfare. Particularly, Nugraha (Citation2013) examined the impact of corruption and money laundering on FDI for five selected ASEAN countries using 2000–2009. Nugraha (Citation2013) specifically assessed how the establishment of Financial Intelligence (FIUs) influences FDI flows to the five countries. Again, Perez et al. (Citation2012) examined the role of FDI in facilitating money laundering in transition economies.
This present study makes contributions to the existing body of knowledge. First, the study examines the impact of AML systems on FDI flows using data from 165 countries globally and a more comprehensive measure of AML systems. The study uses the Basel AML Index, which assesses the AML risks worldwide based on the quality of AML framework of countries. The assessment is also based on control of bribery and corruption, financial transparency and standards, accountability and transparency of the public sector, and the legal and political risk of countries. Second, the study examines the influence of AML systems on FDIs for developed, developing, and African countries and offshore financial centres (OFCs). This is against the background that the impact of AML systems on FDIs may differ across different economies due to the differences in the institutional contexts of these economies. The study pays particular attention to African countries and OFCs. The African continent is perceived as risky due to uncertainty with government policy, low quality of infrastructure, and political instability (Asiedu, Citation2002). In addition, Africa is noted for a high incidence of money laundering. It is noted that the African continent that produces just 3 percent of the world GDP accounts for between 10 to 20 percent of the total illicit cross-border flows (AAPPG, Citation2006). Additionally, according to the Basel AML Report 2021, Africa has the highest overall risk score of all regions (Basel Institute on Governance, Citation2021). Further, OFCs are characterized by low taxation regimes and financial secrecy laws (Hines, Citation2010) and are attractive destinations of FDIs. However, in a counter-argument, Antoine (Citation1999) posits that money laundering and bank secrecy are inextricably linked, and combating financial crime can only be achieved by undermining confidentiality laws. The core of AML systems is transparency, and therefore we expect that implementing AML systems in OFCs should discourage FDIs.
Third, the study examines the existence of threshold effects in the AML systems-FDI flows nexus. Although the importance of AML systems cannot be underestimated, Geiger and Wuensch (Citation2007) argued that AML systems might present some unintended consequences. They noted that the expected benefits of AML systems might not be realized as AML systems impede wealth creation by increasing financial and non-financial costs of transactions. Further, Mccarthy et al. (Citation2015) opine that AML compliance has become resource-intensive and expensive business for firms. For instance, a survey report by LexisNexis Risk Solutions (Citation2021) estimates that AML compliance costs US financial firms to the tune of $35.2 billion, $39.8 billion for the UK, $57.1 billion for Germany, $24.8 billion for France, $20.0 billion for Italy, while the global AML compliance cost is estimated at $213.9 billion. These AML compliance costs often result in increased transaction costs for firms. The report further noted that financial crime compliance operations hurt productivity and customer acquisition efforts. Therefore, the study posits that FDI flows might be negatively impacted if AML systems/compliance become excessive. The study employs the two-step systems GMM and the dynamic panel threshold approaches to examine the impact of AML systems on FDIs across developed, developing, and African countries as well as OFCs. Finally, the study explores the threshold effects of AML systems-FDIs nexus. The rest of this paper is organized as follows: Section 2 reviews literature relevant to the study. Section 3 details the methodology employed for the study. Section 4 discusses the empirical results, and Section 5 concludes the study and offers policy recommendations.
2. Literature review
This section reviews relevant literature on FDI and anti-money laundering systems.
2.1. The link between anti-money laundering systems and FDI flows
“Money laundering is the process of disguising the origin of ill-gotten money to make it seem as though such funds were obtained from legitimate sources. Simply put, money laundering is the process of washing “dirty money” to make it look “clean”“ (Mekpor et al., Citation2018, p. 442). The phenomenon of money laundering is said to have gradually grown with organized crime, which has had wide-ranging and devastating effects on the world’s economies (Raweh et al., Citation2017). Money laundering promotes crimes such as bribery, drug trafficking, human trafficking, gambling, embezzlement, fraud, illegal arms sales, insider trading, prostitution rings, smuggling, terrorism, which affects a country’s reputation and, therefore, its ability to attract FDIs (Baldwin, Citation2004). In addition, crime increases a country’s risk profiles and makes it unattractive to foreign investors. Again, the cost of doing business in countries exposed to high crime incidents is high because investors would have to spend more to secure their assets, making the country unattractive to foreign investment. Therefore, an effective AML system puts countries in a better position to attract the needed FDIs. Further, corruption has been identified as one of the significant disincentives to FDI flows. It makes it difficult for people to transact business effectively and it increases the cost of doing business (Wei, Citation2000). However, Gounev and Bezlov (Citation2010) noted that money laundering fosters corrupt activities as it allows money launderers to enjoy proceeds from their corrupt activities without fear of being exposed. Therefore, combating money laundering will help reduce corrupt practices since it denies corrupt officials the opportunity to conceal the origin of their ill-gotten funds for future consumption. Again, embedded in every anti-money laundering framework is the principle of transparency which is a major corruption-fighting tool. Against this backdrop, countries with effective anti-money laundering systems are expected to control corruption better and attract FDIs.
It is widely accepted that a well-functioning financial system should help attract more FDI (Global Financial Stability Report, Citation2007). Therefore, a developed financial market is an incentive for foreign direct investment. However, Van der Zahn et al. (Citation2007) noted that money laundering corrupts the financial market and erodes the confidence and trust of customers in the financial system. Again, money laundering has the propensity to destabilize financial institutions and the financial system as a whole (Mekpor et al., Citation2018). Therefore, it is expected that an effective anti-money laundering system should promote the development of the financial market, hence making the country a destination for foreign direct investments. Furthermore, an effective AML framework is expected to provide a competitive output market and a more leveled playing field for all firms because front companies that benefit from illicit proceeds can subsidize their products and services at levels far below market rates. Money laundering makes it difficult, if not impossible, for the legitimate firms to compete favourably with these front companies that benefit from illicit proceeds from money laundering (McDowell & Novis, Citation2001) which is a major disincentive for market-seeking FDIs. Therefore, the study hypothesizes that effective AML systems should promote FDI flows to countries.
Although the study argues that implementing AML structures in countries should promote FDIs, AML systems might have some unintended consequences if they are too excessive. For instance, Geiger and Wuensch (Citation2007) noted that the costs and benefits of AML measures are hardly quantifiable and therefore cast doubt on the measurement of the effectiveness of AML systems. They further concluded that the costs of money laundering prevention are far more than the benefits that accrue to the economy. Also, according to Mccarthy et al. (Citation2015), AML compliance has become a resource-intensive and expensive business for firms and could be a disincentive for FDI if it becomes too excessive. This position was re-echoed by Tsingou (Citation2010), who observed that developing countries consider strict AML rules to be damaging to their development strategy due to the cost of implementation and compliance. The study further indicated that a sizeable part of the population might be excluded from the formal banking systems due to the documentary requirements of AML rules. Bester et al. (Citation2008) indicated that the implementation of AML controls hurts the access and usage of financial services. Therefore, the study again opines a negative impact of AML systems on FDIs when AML structures become excessive.
However, the effectiveness of AML systems in promoting FDIs may be influenced by the institutional or business environment of a country. An effective AML regime thrives heavily on the principle of transparency therefore, countries with regulatory regimes and systems that promote transparency are able to implement effective AML frameworks. For instance, offshore financial centres (OFCs) and developing countries may not have structures that promote the effective implementation of AML regimes. According to Campbell (Citation2018), money laundering thrives in jurisdictions where structures are set up to hide the nature and ownership of funds. Warf (Citation2002) revealed that the success of OFCs is the degree of confidentiality that investors feel they can obtain. OFCs are noted for lax regulations, tax havens, legally enforced banking secrecy, non-disclosure of corporate ownership, non-disclosure of who benefits from offshore trusts and foundations, and an absence of effective information exchange with the authorities of third party countries (Christensen, Citation2012; Gilmour, Citation2020). These confidentiality laws frustrate the implementation of effective AML systems in OFCs and make OFCs high-risk money laundering jurisdictions. However, according to Christensen (Citation2012), OFCs are attractive destinations for FDIs as about one-third of foreign direct investment is routed through secrecy jurisdictions or OFCs. Therefore, the study argues that implementing AML systems in OFCs may discourage FDIs as AML provisions may breach the principle of confidentiality in OFCs.
2.2. Determinants of FDI flows
The OLI paradigm of Dunning (Citation1980) suggests that several factors influence the FDI decisions of MNEs and these factors largely explain the inflows of FDI into a country. Following the Dunning (Citation1980) OLI paradigm, countries that present attractive market opportunities are potential destinations for MNEs’ FDI decisions. In the case of the market seeking FDI, market size becomes an important determinant of MNEs’ FDI decisions. A larger market size enables MNEs to exploit their ownership advantages and benefit from economies of scale (Cuyvers et al., Citation2011). Asiedu (Citation2002) posits that the impact of trade openness on FDI depends on the type of investment. A less open economy may impose tariffs or impose certain restrictions on trade. When an investment is market-seeking, trade restriction (less opened economy) is expected to impact FDI positively. In contrast, MNEs engaged in export-oriented investment may prefer to locate in a more open economy (see, Adams & Opoku, Citation2015; Agbloyor et al., Citation2013; Asiedu, Citation2002; Bokpin et al., Citation2017). Financial market development plays a key role in influencing FDI into an economy. When foreign firms enter a domestic market, they may need to open bank accounts with the local banks and interface with other financial institutions. These foreign enterprises are usually big account holders who require quality services comparable to international banks (Agbloyor et al., Citation2013). A developed financial market can meet the needs of foreign enterprises and, therefore, influence FDI flows. Dutta and Roy (Citation2011) found that financial development leads to greater FDI inflows but to a certain level of financial development.
Furthermore, investors prefer to invest in more stable economies, and therefore, countries with stable inflation can attract more FDIs. The literature has widely established a negative relationship between FDI and inflation (Agbloyor, Citation2019; Agbloyor et al., Citation2013; Asiedu, Citation2006). According to Dunning (Citation2009), in the 1970s, natural resource endowment was a crucial location incentive for multinational enterprises. The availability of local inputs at lower costs is a critical ingredient in the effective operations of firms. Therefore countries that abound in natural resources are better destinations for FDIs (Asiedu, Citation2006). Again, institutional quality promotes freedom of expression of the citizenry, the protection of property rights, democratic governance, a stable political environment and minimal government interference in the operations of businesses. Countries with better and stronger institutions should be better placed to attract foreign investors (Agbloyor et al., Citation2013). Further, the availability of a skilled labour force is a major determinant of MNEs’ location decisions. MNEs are noted for superior technologies, and the host country’s workforce’s ability to use these technologies is an essential driver of MNEs’ location decisions. Kahouli and Maktouf (Citation2015) find quality human capital to promote FDI significantly. Finally, infrastructure development in providing good roads, electricity, internet, and telephony services provide the basis for businesses’ development. Infrastructural development offers an attractive environment for FDIs. Liu et al. (Citation2020) found that infrastructure positively affects FDI attractiveness.
3. Methodology
This section describes the various methods and procedures the study adopts in data analysis. To investigate the effect of AML systems on FDI, the study utilises panel data covering the period 2012–2018 in 165 countries. The Basel AML Index is only available from 2012 and for the countries in the sample. Panel data is used because it allows us to explore the cross-sectional and time-series variation of the data. Panel data control for omitted variables and also helps to account for both long-run and short-run effects (Wooldridge, Citation2012)
3.1. Empirical model
In this study, a dynamic panel data model on the impact of AML systems on FDIs is estimated as follows;
where represents foreign direct investment of country i in year t and
represents the lag of foreign direct investment.
represents the AML index. Again,
represents human capital development while
represents financial development.
represents trade openness and
represents the size of the economy. Further,
represents natural resource endowment while
represents the quality of institutions.
represents the level of inflation of a country and
represents the infrastructural development of a country. The
terms represent the coefficients of the respective variables.
and
represent individual country effects.
3.2. Variable description and measurements
The study measures FDI as the natural logarithm of net FDI inflows. The data is sourced from World Development Indicators (WDI) by the World Bank. The AML index is measured using the Basel Anti-money Laundering Index published by the Basel Institute on Governance. The Basel AML Index is an independent annual ranking that assesses the risk of money laundering and terrorist financing (ML/TF) worldwide. The index assesses the effectiveness of AML systems and structures to counter money laundering and terrorist financing in countries. The index covers five main domains weighted as follows; quality of anti-money laundering/Countering Financing of Terrorism framework (65%); bribery and corruption (10%); financial transparency and standards (15%); public transparency and accountability (5%); and legal and political risks (5%). The index ranges from 0 to 10, where 0 indicates the lowest and 10 indicates the highest. For easy interpretation, we rescale the index following Agoba et al. (Citation2019) and Ofoeda et al. (Citation2020), so lower scores now correspond to less effective AML systems, and higher scores correspond to more effective AML systems. We use the formula −1*(AMLR-10), where AMLR is the AML Index. We expect countries with an effective AML framework to be better positioned to attract more FDIs.
In line with the literature, the study controls for human capital development, trade openness, market size, natural resource endowment, institutional quality, inflation, and infrastructural development. The study uses the IMF composite financial development index to proxy the extent of countries’ financial development (Svirydzenka, Citation2016). It is expected that countries with a well-functioning financial sector will be in a better position to attract FDIs. The study measures trade openness as the ratio of export plus imports to GDP. According to Asiedu (Citation2002), trade openness is a crucial driver of FDI flows. Therefore, the study hypothesizes a positive relationship between trade openness and FDI flows. The data is sourced from WDI. Again, the study expects a positive relationship between FDI and market size. The natural logarithm of GDP per capita income from the WDI series to proxy market size is used. Countries with higher income levels or larger ones are likely to attract more FDIs because of their ability to consume more. This is evident especially in market-seeking FDIs (Agbloyor, Citation2019). Again, inflation to proxy macroeconomic performance is used. Inflation is measured using the consumer price index. The study expects a negative relationship between inflation and FDI. This is because higher inflation discourages foreign investors from investing in an economy. Agbloyor (Citation2019) and Asiedu (Citation2006) found a negative relationship between inflation and FDI.
Further, natural resource endowment is expected to have either a negative or positive relationship with FDI. Therefore, the study measures natural resource endowment using natural resource rents. A positive relationship indicates that natural resources attract FDI flows into a country (Agbloyor, Citation2019; Asiedu, Citation2006), while a negative relationship suggests the natural resource curse (Okafor, Citation2015). In addition, it is generally believed that there is a strong relationship between FDI inflow and the human capital development of the host country (Zaman et al., Citation2012). The study uses econdary school enrollment as a percentage of all eligible children to measure human capital development. Again, infrastructure development should provide an attractive business environment for FDIs. Infrastructure development using telephone plus mobile subscriptions per 100 people is measured. Data on inflation (consumer price index), secondary school enrollment, infrastructure development (telephone plus mobile subscriptions per 100 people), and natural resource rents are sourced from the WDI series. Further, the study hypothesizes a positive relationship between institutional quality and FDI, suggesting that institutions promote FDI flows. The study uses the civil liberties score obtained from the Freedom House database. The civil liberties score ranges from 7 to 1, with 7 representing the least rating and 1 the highest. Therefore, following Agoba et al. (Citation2019), the study rescales the original score to range from 0 to 6 where lower scores now represent weak institutions and higher scores representing high-quality institutions. To do this, the study uses the formula −1*(CLS-7), where CLS is the civil liberty score as given by Freedom House. In , we present the measurements as well as the sources of data for all variables used in the study
Table 1. Variable descriptions and data sources
3.3. Estimation technique
3.3.1. GMM estimation
The study employs the two-step systems generalized method of moments (SGMM) estimator in estimating the hypothesized relationships. The two classes of GMM estimators as developed by Arellano and Bover (Citation1995), Blundell and Bond (Citation1998), Arellano and Bond (Citation1991), and Holtz-Eakin et al. (Citation2011) are the difference GMM estimator and the SGMM estimator. The SGMM overcomes the problems associated with using the difference GMM, which is biased because it uses lagged levels of explanatory variables as instruments. Additionally, the difference GMM estimator eliminates the country-specific effect. Further, the SGMM adds a level equation to the difference equation to deal with the issue of weak instruments (Roodman, Citation2009). There are two variants of the SGMM: one-step and two-step SGMM. The two-step SGMM introduced by Blundell and Bond (Citation1998) was designed to deal with higher persistent data. The study adopts the two-step estimator with Windmeijer (Citation2005) corrected standard errors as it is asymptotically more efficient than the one-step estimator. Again, the study uses the two-step SGMM because it is particularly relevant for studies with short time periods than the number of countries, which happens to be the case with our dataset. Further, the two-step SGMM approach allows us to treat FDI as a dynamic process where previous FDI flows influence current FDI flows. Finally, the GMM approach allows us to control for endogeneity which often plagues panel data. To ensure the consistency of estimates, the study employs the Sargan/Hansen test of overidentification restrictions and the Arellano and Bond test for second-order serial correlation in the error term. According to Roodman (Citation2009), the Sargan/Hansen tests measure the validity of instruments by analyzing sample analogues of the moment conditions used in the estimation. Finally, the error term may be serially correlated in the first order. However, the second-order must not be serially correlated because that could be a sign of misspecification of the model.
3.4. Dynamic panel threshold regression
The study further argued that the impact of AML systems on FDI flows might differ across the different levels of AML systems. The study posits that the AML systems have a positive effect on FDIs. However, excessive AML structures may discourage FDI flows. This is because the cost of AML compliance has become resource-intensive, which may increase the transaction costs of businesses and may increase the cost of doing business in a country. This increases the country’s production cost and makes the country an unattractive destination for FDIs. Again, more stringent AML systems mean more AML compliance requirements which slow down the pace of transactions which is critical in MNEs’ decisions for FDIs. Traditionally, the threshold effect is assessed by introducing a quadratic term in the model (see, Aibai et al., Citation2019; Ibrahim & Alagidede, Citation2018; Taghizadeh-Hesary et al., Citation2019). However, this approach cannot identify the exact point where the relationship changes direction. Also, it may be prone to multicollinearity problems and may not be able to address structural breaks in the data (Huang et al., Citation2018).
Therefore, in exploring the nonlinear behavior of the AML frameworks-FDI nexus, the study adopts the dynamic panel threshold regression of Seo and Shin (Citation2016) and Seo et al. (Citation2019), which had been applied in recent literature (Bolarinwa et al., Citation2021; Botev et al., Citation2019; Luan et al., Citation2019). This approach is built on the principles of GMM. Again, this approach extends the cross-sectional threshold model of Caner and Hansen (Citation2004) and the dynamic panel threshold estimation approach by Kremer et al. (Citation2013). Caner and Hansen’s (Citation2004) model deals with endogenous regressors for cross-sectional data, while Kremer et al. (Citation2013) address endogeneity for dynamic panel data regressors. However, Seo et al. (Citation2019) address endogeneity with the regressors and the threshold variable, and it also accounts for the lagged explained variables. Again, it addresses the inherent problems of endogeneity and simultaneity which cannot be ruled out in the AML systems-FDI connection. Again, this approach reduces sampling errors and also allows the regressors and threshold variable to be endogenous simultaneously (Zhang et al., Citation2019). Further, this estimation technique can trace the threshold for policy decisions. Also, the threshold regression model can be described as follows:
where subscripts i and t refer to country and time, respectively. represents foreign direct investment while
denote the lag of foreign direct investment. Again,
denote AML Index whereas
represents the country-specific fixed effects. Further,
is a zero mean, finite variance, i.i.d. disturbance. We denote our control variables hypothesized to affect FDI by a vector
. Again,
is the regime-switching or threshold variable that is used in splitting our data into two sample groups while
is the threshold value. Furthermore,
and
are the coefficients of the lag of FDI and AML Index below the threshold value
, whereas
are the coefficients of the lag FDI and AML Index above the threshold value.
As noted earlier, in examining the threshold effects, the conventional technique is to impose the functional or standard form of the threshold on the model, that is, by either introducing the quadratic term or the cubic term. As a result, the threshold level is determined exogenously. However, the panel threshold regression technique as proposed by Seo et al. (Citation2019) and Seo and Shin (Citation2016) does not impose the functional form of the threshold on the data. Thus, it allows the thresholds to be determined endogenously. It identifies the threshold in the data set and splits the sample based on the established thresholds. The study uses 2000 bootstrap replications, a 15% trimming percentage, and 100 grid numbers to test the existence of thresholds. The bootstrap method allows for the construction of a confidence interval and assessment of the statistical significance of the thresholds identified. Seo et al. (Citation2019) and Seo and Shin (Citation2016) proposed the first difference transformation that relaxes the exogeneity assumption of regressors and the threshold variable to estimate the coefficients. It further guarantees that the estimators follow a normal distribution asymptotically, which validates the use of the Wald test for standard.
4. Empirical results
This section presents the summary statistics, the correlation among the variables used in the study, and the regression results. The descriptive statistics are presented in Table . The study reports the mean log of net FDI inflows of 20.82, 22.37, 20.36, 19.97, and 20.77 for the full sample, developed, developing, African countries, and OFCs, respectively. AML index reports a mean of 4.17 for our full sample, 5.41 for developed countries, 3.80 for developing countries, 3.37 for African countries, and 4.53 for OFCs. The Basel AML index ranges from 0–10, where 0 indicates the highest level of effectiveness of AML systems (lowest risk level) and 10 indicates the lowest level of effectiveness of AML structures (highest risk level). However, the rescaled AML index indicates the highest level of effectiveness of AML structures for a score of 10 and the lowest level of effectiveness for a score of 0. Therefore, a mean of 4.17 shows a below-average performance of AML systems globally. This is an indication of relatively weak AML frameworks worldwide. This is corroborated by the findings of the 2021 report of the Basel Institute on Governance which indicates that the effectiveness of AML structures of countries is low (Basel Institute on Governance, Citation2021). The report also indicates 17 percent of countries scored zero for the effectiveness of their preventive measures.
Table 2. Descriptive statistics
Human development recorded a mean of 87.16 percent for our full sample, 111.29 percent for developed countries, 79.94 percent for developing countries, 62.54 percent for African countries, and 98.23 percent for OFCs. This shows a high level of human capital development worldwide. However, comparatively, Africa has the lowest level of human capital development. Further, for financial development, the study reports an average of 0.29, 0.41, 0.25, 0.25, and 0.27 for full, developed, developing, Africa, and OFCs samples, respectively. The findings suggest a less developed financial sector globally. However, developed countries and OFCs have more advanced financial systems. Again, the results show that globally, countries are open to trade. The study reports an average of 92.16 percent, 97.67 percent, 90.51 per cent, 92.74 percent, 98.83 percent for trade openness for our full, developed, developing, Africa and OFCs samples, respectively. Comparatively, OFCs are more open to international trade than any other part of the world.
Further, the natural logarithm of GDP per capita income reports 8.75 for our full sample, 10.32 for developed countries, 8.28 for developing countries, 7.48 for African countries, and 9.59 for OFCs. Natural resource rents as a percentage of GDP report a mean of 6.37 percent for the full sample, 1.16 per cent for developed countries, 7.93 percent for developing countries, 9.89 percent for African countries, and 1.32 percent for OFCs. This indicates a less reliance of countries on natural resources globally. However, Africa compares higher to other parts of the world regarding natural resource rents to GDP.
Again, the findings show a mean of 3.78, 4.67, 3.51, 3.51, 4.54 for our full, developed, developing, Africa, and OFCs samples, respectively, for civil liberties index (institutional quality). The consumer price index (inflation) reports a mean of 127.58 for our full sample, 109.5 for developed countries, 132.99 for developing countries, 143.43 for African countries, and 111.27 for OFCs. Infrastructure (number of telephone and mobile lines per 100 people) shows an average of 128.80 for the full sample, 158.19 for developed countries, 132.99 for developing countries, 143.43 for African countries, and 150.17 for OFCs. Again, the study presents the correlation matrix that shows how the variables are correlated in Table . The correlation matrix also helps to ascertain the presence of multicollinearity in our data. The findings of our study show that there is no presence of multicollinearity in our data. The study shows a positive association between AML structures and FDIs. Market size, trade openness, financial development, and human capital development are positively associated with FDIs.
Table 3. Correlation results
Further, the study examines the impact of AML systems on FDI flows across different economies of the world. The study examines how AML systems influence FDIs to developed, developing, African countries and offshore financial centres. The study uses the United Nations classifications to categorize countries into developed and developing countries, and the IMF classification to identify 25 countries in the sample as Offshore Financial Centres. The study again examines how AML systems impact FDI flows across the levels of AML structures of countries. This is based on the fact that the impact of AML systems on FDIs may not be constant. That is, AML structures may have a detrimental impact on FDI flows when it becomes excessive. Table presents the two-step SGMM regression results for the full sample, developed, developing, Africa, and OFCs in models 1, 2, 3, 4 and 5, respectively.
Table 4. Two-Step systems GMM regression results on the effect of AML systems on FDI flows
The study reports that AML structures positively impact FDI flows for our full, developing, and African country samples in models 1, 3, and 4, respectively. This indicates that more effective AML systems stimulate FDI flows across the globe. Foreign investors are interested in investment locations that offer the best conditions. It is a widely accepted fact that the legal framework largely influences the economic fortunes of a country. Hence, FDI is attracted to countries with a quality legal framework because of superior economic performance. Countries with a high incidence of money laundering are characterized by policy mistakes, volatility in exchange and interest rates, high inflation rates, etc., which is a disincentive for FDI flows. Therefore, an effective AML structure is expected to promote a stable macroeconomic environment, hence, attract FDIs.
Again, money laundering has significant implications for the reputation of countries as countries cited for deficiencies in their AML frameworks are often blacklisted. This reputational damage results in increased transaction costs as well as increased risk for investors. Therefore, sound AML systems reduce uncertainty and risk associated with an investment as it promotes greater transparency in the business environment. Additionally, the high incidence of money laundering distorts the financial systems of countries. After investing in a country, foreign investors may want to raise finance from domestic financial markets to complement their resources. Also, according to Adams and Opoku (Citation2015), effective regulatory regimes lead to more competitive output markets. It is expected that countries with effective AML regimes will have a well-functioning financial market and, therefore, can attract more FDIs. The result of the study is consistent with Nugraha (Citation2013) findings, which found that the establishment of Financial Intelligence Units positively affects FDI flows to five ASEAN countries.
Furthermore, the results clearly show that developing and African countries benefit more from implementing sound AML structures. Developing and African countries are noted for weak institutions, less developed financial markets, heightened uncertainties and risks associated with an investment, and weak AML regulatory frameworks. Therefore, an attempt to promote sound AML frameworks is expected to engender a more than proportionate response in FDI flows. The study suggests that if developing and African countries implement effective AML systems, they are better placed to attract FDIs. For instance, the African continent is highly endowed with natural resources, which would have been a major incentive for FDIs. However, the continent is also plagued with a high incidence of money laundering and its attendant problems. Therefore, Africa implementing effective AML structures make the continent an attractive destination for FDIs.
Nevertheless, in model 5, the study found AML structures negatively impact FDIs flows to OFCs. The results of our study show that AML systems rather discourage FDI flows to OFCs. OFCs thrive heavily on confidentiality laws and strict financial secrecy. Most MNCs and foreign investors found OFCs attractive for investment because of the confidentiality laws they implement. Christensen (Citation2006) noted;
The “secrecy space” creates an effective barrier to investigation of the activities in the OFCs by external authorities and facilitates the laundering of proceeds from a wide range of criminal and unethical practices, including fraud, embezzlement, and theft, bribery, narco-trafficking, illegal arms trafficking, counterfeiting, insider trading, false trade invoicing, transfer mispricing and tax dodging. (p. 2)
However, an effective AML framework requires transparency which flies directly in the face of the confidentiality laws implemented by OFCs. Therefore, implementing effective AML systems in OFCs instead discourage FDIs. This is consistent with the findings of Perez et al. (Citation2012), who reported that over 20% of FDI to money-laundering countries in their sample were made to facilitate illicit money flows.
The study includes some control variables. In model 1, the study found a positive relationship between human capital development and FDI. This suggests that countries with more developed human capital attract more FDIs. This result is similar to Kahouli and Maktouf (Citation2015). Again, financial development had a positive and significant relationship with FDI in models 1, 3, and 4. The results suggest that well-developed financial markets stimulate FDI flows. Foreign firms in a domestic economy use domestic financial markets by opening bank accounts. Therefore, they expect a well-functioning financial market that can provide them with quality internationally comparable services. This finding is confirmed by Agbloyor et al. (Citation2013). Further, the study found trade openness to positively impact FDIs in models 1, 2, 4, and 5. The results suggest that countries that are open to international trade attract more FDIs. This is corroborated by the findings of Agbloyor et al. (Citation2013). Institutional quality enters models 1, 2, 3, and 4 positively with FDI flows. The results suggest that countries with stronger institutions receive more FDIs. This is because stronger institutions promote freedom of expression of the citizenry, the protection of property rights, democratic governance, stable political environment, which are important in creating the right conditions for FDI. This finding is similar to Agbloyor et al. (Citation2013). Again, market size reported a positive coefficient from models 1, 2, 3 and 5. This indicates that countries with larger market sizes attract more FDIs. A larger market size enables MNEs to exploit their ownership advantages and benefit from economies of scale. This is supported by Asiedu (Citation2002). Inflation which is a proxy for macroeconomic stability reports a negative coefficient with FDIs in model 3. This indicates that higher inflation rates discourage FDI flows to countries. Finally, infrastructure development enters models 1, 2, 4 and 5 positively. This suggests that countries with more developed infrastructure are attractive destinations for FDIs.
4.1. Panel threshold regression
Given that in this study, the aim is to establish the nonlinearity in the AML systems-FDIs nexus, as a first step, the study determines whether the relationship is monotonic. The study hypothesizes that there is a nonlinear relationship between AML systems and FDIs. That is, the impact of AML systems on FDI differ across the different levels of AML structures. Accordingly, the study tests the null hypothesis of linearity were against the alternative hypothesis of a threshold model, that is,
. The study uses 2000 bootstrap replications, a 15% trimming percentage, and 100 grid numbers to ascertain the hypothesized relationships. Also, the study tests the existence of a threshold for our full, developed, developing, Africa and OFCs samples. In Table , the study presents the results on the test of the existence of threshold as depicted by the p-values of the linearity test as well threshold regression. As shown in Table , the bootstrap p-values (linearity test p-values) for the test of the existence of threshold effect for our full, developed, developing, Africa and OFCs samples indicate p-values less than 0.05. This suggests the presence of a threshold effect in the AML systems-FDIs connection. The findings, therefore, suggest that the impact of AML systems on FDI flows is determined by the level of effectiveness of AML systems of the country. Consequently, the study divides the sample into two regimes where one regime is above the threshold value, and the other is below the threshold value.
Table 5. Dynamic panel threshold regression results on the effect of AML systems on FDI flows
Having established the existence of threshold effect in the relationship between AML systems and FDIs, the study proceed with the panel threshold regression. As presented in Table , the results of the study show threshold values of 3.381, 4.783, 3.141, 2.899, and 4.033 for our full, developed, developing, Africa, and OFCs samples, respectively. From the results of the study, it was found that AML systems positively impact FDIs for our full and developing country samples. However, the study reports a positive significant coefficient (−0.869) below the threshold and significant negative coefficient (0.708) above the threshold for our full sample and positive significant coefficient (3.223) below the threshold, and a significant negative coefficient (−3.632) above the threshold for developing country sample. The findings show that although AML systems positively impact FDI, the impact is only experienced below the threshold value. The results indicate that the positive impact of AML systems on FDIs wanes away and becomes negative when AML systems become excessive or are beyond the threshold value. This suggests that excessive AML structures might discourage FDIs. AML compliance has become a resource-intensive and expensive business for firms. AML compliance costs result in increased transaction costs for firms, making firms highly uncompetitive. Consequently, increased AML structures may discourage FDI flows. This finding relates to Ofoeda et al. (Citation2020), who report that AML systems negatively impact financial sector development beyond the threshold value.
Further, the study found that AML systems have negative coefficients across all levels of AML structures for developed countries. Nonetheless, these relationships are not significant. The results show FDIs in developed countries are not driven by AML structures. Additionally, the study provides evidence of a positive impact of AML systems on FDIs across all AML systems for African countries. The study further shows a positive coefficient (0.634) below the threshold and (1.213) above the threshold value. Although this study shows a positive impact of AML systems on FDIs across all AML structures, the effect is more pronounced at higher levels of AML structures. This indicates that for African countries to attract more FDIs, they need to tighten their AML systems. This is because Africa is perceived to have a high money laundering risk which discourages foreign investors from investing in Africa. According to the Basel Institute on Governance Report on money laundering across the globe, Africa is considered the region with the highest overall money laundering risk (Basel Institute on Governance, Citation2021). Therefore, implementing effective AML systems will encourage more foreign direct investment into Africa.
In contrast, the study found that AML systems negatively impact FDI across all AML systems for offshore financial centres. The study reports a negative coefficient of (−3.066) below the threshold and (−7.160) above the threshold value. The findings show AML structures engender a more than proportionate negative response in FDIs. However, this negative response is more pronounced when AML structures are above the threshold value. As indicated earlier, OFCs thrive on the principle of confidentiality and are found as attractive destinations for investors because of the laxity of their laws and the fact that they serve as tax havens. However, with the implementation of AML structures which requires transparency, most investors shy away from these OFCs.
5. Conclusion and policy recommendations
Countries around the world have sought to increase FDI in recent years by implementing policies that are friendly to attracting FDI. FDI remains one of the largest and most dependable sources of capital inflows to developing countries and is seen as an essential source of capital that complements domestic capital for development. Nonetheless, MNEs increasingly look for investment locations that offer the best conditions to rapidly deliver new and high-quality products. In recent times, money laundering has become topical in the development agenda of most economies across the globe due to its devastating consequences on the global financial system and the economies of nations. The incidence of money laundering affects a country’s reputation and consequently its attractiveness for MNEs’ FDI location decisions. Therefore, effective AML regulation is expected to provide the right environment to attract FDIs. However, AML structures may have some unintended consequences. This paper examines the relationship between AML systems and FDI for developed, developing, African countries and offshore financial centres. The study again examines the existence of threshold effects in the AML systems-FDI connection. Also, the study examines the impact of AML systems on FDIs above and below the threshold value and this is done for developed, developing, African countries and OFCs. The study relied on data from 165 countries across different economies over the period 2012–2018. The study employs the two-step system GMM and the dynamic panel threshold regression of Seo and Shin (Citation2016) and Seo et al. (Citation2019) in estimating the data.
Generally, the results suggest that AML structures significantly explain FDI flows. However, the study found that AML systems somewhat do not impact FDIs for developed countries. This study further reports that effective AML structures stimulate FDIs in developing and African countries. In sharp contrast, the study found that AML systems rather discourage FDIs in OFCs. Further, it was found that the influence of AML systems on FDIs is threshold-specific for our full, developed, developing, Africa and OFCs samples. Specifically, AML systems positively impact FDIs below the threshold for our full, developing, and African country samples. However, this impact becomes negative above the threshold for our full and developing country samples. This is because the implementation and compliance of AML systems are resource-intensive, and therefore, excessive AML systems may somewhat discourage FDIs. However, for developed countries, the relationships above or below the threshold values are not significant. Again, the study provides evidence of a positive impact of AML systems on FDIs across all levels of AML structures for African countries. Although the study shows a positive impact of AML systems on FDIs across all levels of AML systems, the impact is more pronounced at higher levels of AML systems. Finally, the study found that AML systems negatively impact FDI across all levels of AML structures for offshore financial centres. OFCs thrive on the principle of confidentiality and are found as attractive destinations for investors. Therefore, the implementation of AML structures may discourage most investors from investing in OFCs.
The findings of the study impose some policy implications. First, the domestic business and institutional environment of countries must be critically studied in the design of FDI policies. This is because the impact of AML systems on FDIs differ across the different structures and economies of the world. More specifically, the study provides evidence that AML systems influence MNEs’ FDI decisions to developing and African countries. Therefore, developing and African countries can attract FDIs by implementing AML systems/structures. Policymakers and regulators of developing and African countries should strengthen AML frameworks by implementing policies that improve financial transparency and standards, enhance public sector transparency and accountability, reduce legal and political risk and fight bribery and corruption if they are to attract more FDIs to promote growth. However, the findings of the study show that FDIs to OFCs are frustrated by AML structures. Therefore, policymakers and regulators of OFCs must develop AML structures that respond to their specific needs. Second, the study provides evidence that the impact of AML systems on FDIs is nonlinear and therefore threshold-specific. Specifically, AML systems positively impact FDIs below the threshold values of 3.381 for our full, 3.141 for developing, and 2.899 for African country samples. However, the impact is negative beyond the threshold values. This suggests that excessive AML structures have a detrimental effect on FDIs. Therefore, regulators should develop methodologies for integrating AML oversight into their existing supervisory regimes to make AML implementation and compliance cost-effective. This study only focused on inward FDIs. However, the motive to conceal proceeds from illicit activities may motivate outward FDIs. Therefore, assessing how FDI outflows are motivated by AML systems would be enriching. However, this work provides an essential step in evaluating the effect of AML systems on inward FDIs.
Disclosure statement
No potential conflict of interest was reported by the author(s).
Data availability statement
The data that support the findings of this study are openly available in World Development Indicators at http://datatopics.worldbank.org/world-development-indicators/ and the Basel Institute of Governance at https://www.baselgovernance.org/basel-aml-index/ public-ranking
Additional information
Funding
Notes on contributors
Isaac Ofoeda
Isaac Ofoeda is a Lecturer in the Department of Accounting at the University of Professional Studies, Accra (UPSA). He holds a PhD in Finance from the University of Ghana Business School and also a member of the Institute of Chartered Accountants, Ghana (ICAG). He currently coordinates the MPhil Finance and Msc Insurance Risk Management Programmes at the School of Graduate Studies. He was the Research Officer for the Department of Accounting. His research focuses on anti-money laundering regulations, financial inclusion, financial sector development, corporate governance, financial markets and institutions regulations and foreign direct investment.
References
- AAPPG. (2006). The other side of the coin: The UK and corruption in Africa, A Report by the Africa All Party Parliamentary Group, March.
- Adams, S., & Opoku, E. E. O. (2015). Foreign direct investment, Regulations and growth in sub-Saharan Africa. Economic Analysis and Policy, 47 Sep , 48–27. https://doi.org/10.1016/j.eap.2015.07.001
- Agbloyor, E. K. (2019). Foreign direct investment, political business cycles and welfare in Africa. Journal of International Development, 31(April), 345–373. https://doi.org/10.1002/jid.3408
- Agbloyor, E. K., Abor, J., Adjasi, C. K. D., & Yawson, A. (2013). Exploring the causality links between financial markets and foreign direct investment in Africa. Research in International Business and Finance, 28(1), 118–134. https://doi.org/10.1016/j.ribaf.2012.11.001
- Agbloyor, E. K., Gyeke-dako, A., Kuipo, R., & Abor, J. Y. (2016). Foreign direct investment and economic growth in SSA : The role of institutions. Thunderbird International Business Review 58 5 , 1–19. https://doi.org/10.1002/tie
- Agoba, A. M., Abor, J. Y., Osei, K., Sa-Aadu, J., Amoah, B., & Dzeha, G. C. O. (2019). Central bank Independence, elections and fiscal policy in Africa: Examining the moderating role of political institutions. International Journal of Emerging Markets, 14(5), 809–830. https://doi.org/10.1108/IJOEM-08-2018-0423
- Aibai, A., Huang, X., Luo, Y., & Peng, Y. (2019). Foreign direct investment, institutional quality, and financial development along the belt and road : An empirical investigation. Emerging Markets Finance and Trade 55 14 , 1–20. https://doi.org/10.1080/1540496X.2018.1559139
- Al-khouri, R. (2015). Determinants of foreign direct and indirect investment in the MENA region. The Multinational Business Review, 23(2), 148–166. https://doi.org/10.1108/MBR-07-2014-0034
- Antoine, R. M. B. (1999). The protection of offshore confidentiality: Policy implications and legal trends. Journal of Financial Crime, 7(1), 9–25. https://doi.org/10.1108/eb025916
- Arellano, M., & Bond, S. (1991). Some tests of specification for panel data:Monte Carlo evidence and an application to employment equations. Review of Economic Studies, 58(2), 277–297. https://doi.org/10.2307/2297968
- Arellano, M., & Bover, O. (1995). Another look at the instrumental variable estimation of error-components models. Journal of Econometrics, 68(1), 29–51. https://doi.org/10.1016/0304-4076(94)01642-D
- Asiedu, E. (2002). On the determinants of foreign direct investment to developing countries: Is Africa different ? World Development, 30(1), 207–119. https://doi.org/10.1016/S0305-750X(01)00100-0
- Asiedu, E. (2006). Foreign direct investment in Africa: The role of natural resources, market size, government policy, institutions and political instability. World Economy, 29(1), 63–77. https://doi.org/10.1111/j.1467-9701.2006.00758.x
- Aziz, O. G. (2018). Institutional quality and FDI inflows in Arab economies. Finance Research Letters, 25(August 2016), 111–123. https://doi.org/10.1016/j.frl.2017.10.026
- Baah-Boateng, W. (2015). Unemployment in Ghana: A cross-sectional analysis from demand and supply perspectives. African Journal of Economic and Management Studies, 6(4), 402–415. https://doi.org/10.1108/AJEMS-11-2014-0089
- Baldwin, F. N. (2004). The financing of terror in the age of the internet: Wilful blindness, greed or a political statement ? Journal of Money Laundering and Control, 8(2), 127–158. https://doi.org/10.1108/13685200510621154
- Basel Institute on Governance. (2021). Basel AML Index: 10th Public Edition - Ranking money laundering and terrorist financing risks around the world. https://baselgovernance.org/sites/default/files/2021-09/Basel_AML_Index_2021_10th-Edition.pdf
- Bester, H., Chamberlain, D., De Koker, L., Hougaard, C., Short, R., Smith, A., & Walker, R. (2008). Implementing FATF standards in developing countries and financial inclusion: Findings and guidelines. In Washington, DC: FIRST Initiative, The World Bank. (Issue February). https://www.findevgateway.org/sites/default/files/publications/files/mfg-en-paper-implementing-fatf-standards-in-developing-countries-and-financial-inclusion-findings-and-guidelines-feb-2008.pdf
- Blundell, R., & Bond, S. (1998). Initial conditions and moment restrictions in dynamic panel data models. Journal of Econometrics, 87(1), 115–143. https://doi.org/10.1016/S0304-4076(98)00009-8
- Bokpin, G. A., Mensah, L., & Asamoah, M. E. (2017). Legal source, institutional quality and FDI flows in Africa. International Journal of Law and Management, 59(5), 687–698. https://doi.org/10.1108/IJLMA-03-2016-0028
- Bolarinwa, S. T., Olayeni, R. O., & Vo, X. V. (2021). Is there a nonlinear relationship between nonperforming loans and bank profitability? Evidence from dynamic panel threshold. Managerial and Decision Economics, 42(3), 649–661. https://doi.org/10.1002/mde.3262
- Botev, J., Égert, B., & Jawadi, F. (2019). The nonlinear relationship between economic growth and financial development: Evidence from developing, emerging and advanced economies. International Economics, 160(December 2017), 3–13. https://doi.org/10.1016/j.inteco.2019.06.004
- Buckley, P. J., Clegg, J. L., Cross, A. R., & Voss, H. (2010 What can emerging markets learn from the outward direct investment policies of advanced countries?). In Foreign Direct Investments from Emerging Markets, Moran, (New York: Palgrave Macmillan), 243–276. https://doi.org/10.1057/9780230112025_13
- Busse, M., & Groizard, J. L. (2008). Foreign direct investment, regulations and growth. The World Economy, 31(7), 861–886. https://doi.org/10.1111/j.1467-9701.2008.01106.x
- Campbell, L. (2018). Dirty cash (money talks): 4AMLD and the money laundering regulations 2017. Criminal Law Review, 2 2018 , 102–122 chrome-extension://efaidnbmnnnibpcajpcglclefindmkaj/viewer.html?pdfurl=https%3A%2F%2Fdro.dur.ac.uk%2F22985%2F1%2F22985.pdf%3FDDD19%2Brxcg23%2Bd700tmt&clen=428586&chunk=true.
- Caner, M., & Hansen, B. E. (2004). Variable instrumental estimation of a threshold. Econometric Theory, 20(5), 813–843. https://doi.org/10.1017/S026646660420501
- Christensen, J. (2006). Follow the money: How Tax havens facilitate dirty money flows and distorts global markets. Being a Paper Presented at the Economic Geography Research Group Geographies of Corruption, RGS-IBG Conference Kensington Gore, London, 32( 2), 68–69. https://doi.org/10.1080/00987913.2006.10765032
- Christensen, J. (2012). The hidden trillions: Secrecy, corruption, and the offshore interface. Crime, Law and Social Change, 57(3), 325–343. https://doi.org/10.1007/s10611-011-9347-9
- Cuyvers, L., Soeng, R., Plasmans, J., & Van Den Bulcke, D. (2011). Determinants of foreign direct investment in Cambodia. Journal of Asian Economics, 22(3), 222–234. https://doi.org/10.1016/j.asieco.2011.02.002
- Dunning, J. H. (1980). Toward an eclectic theory of international production: Some empirical tests. Journal of International Business Studies, 11(1), 9–31. https://doi.org/10.1057/palgrave.jibs.8490593
- Dunning, J. H. (2006). Towards a new paradigm of development: Implications for the determinants of international business. Transnational Corporations, 15(1), 175–230. https://doi.org/10.4337/9781788118606.00015
- Dunning, J. H. (2009). Location and the multinational enterprise: John Dunning’s thoughts on receiving the Journal of International Business Studies 2008 Decade Award. Journal of International Business Studies, 40(1), 20–34. https://doi.org/10.1057/jibs.2008.75
- Dutta, N., & Roy, S. (2011). Foreign direct investment, financial development and political risks. The Journal of Developing Areas, 44(2), 303–327. https://doi.org/10.1353/jda.0.0106
- Felisoni de Angelo, C., Eunni, R. V., & Manoel Martins Dias Fouto, N. (2010). Determinants of FDI in emerging markets: Evidence from Brazil. International Journal of Commerce and Management, 20(3), 203–216. https://doi.org/10.1108/10569211011076901
- Geiger, H., & Wuensch, O. (2007). The fight against money laundering An economic analysis of a cost-benefit. Journal of Money Laundering Control, 10(1), 91–105. https://doi.org/10.1108/13685200710721881
- Gilmour, P. M. (2020). Lifting the veil on beneficial ownership: Challenges of implementing the UK’s registers of beneficial owners. Journal of Money Laundering Control, 23(4), 717–734. https://doi.org/10.1108/JMLC-02-2020-0014
- Global Development Report. (2005). . Global development finance: Mobilizing finance and managing vulnerability (World Bank Publications), . https://doi.org/10.5860/choice.42sup-0471
- Global Financial Stability Report. (2007). In Financial Market Turbulence: Causes, Consequences, and Policies (Issue October). https://www.imf.org/External/Pubs/FT/GFSR/2007/02/pdf/text.pdf
- Globerman, S., & Shapiro, D. (2002). Global foreign direct investment flows: The role of governance infrastructure. World Development, 30(11), 1899–1919. https://doi.org/10.1016/S0305-750X(02)00110-9
- Gounev, P., & Bezlov, T. (2010). . Examining the links between organised crime and corruption (Center for the Study of Democracy, Sofia). https://ec.europa.eu/home-affairs/sites/homeaffairs/files/doc_centre/crime/docs/study_on_links_between_organised_crime_and_corruption_en.pdf
- Hines, J. R. (2010). Treasure Islands. Journal of Economic Perspectives, 24(4), 103–126. https://doi.org/10.1257/jep.24.4.103
- Holtz-Eakin, D., Newey, W., & Rosen, H. S. (2011). Estimating vector autoregressions with panel data. Econometrica, 56(6), 1371–1395. https://doi.org/10.2307/1913103
- Huang, J., Liu, Q., Cai, X., Hao, Y., & Lei, H. (2018). The effect of technological factors on China’s carbon intensity: New evidence from a panel threshold model. Energy Policy, 115(August 2017), 32–42. https://doi.org/10.1016/j.enpol.2017.12.008
- Ibrahim, M., & Alagidede, P. (2018). Effect of financial development on economic growth in sub-Saharan Africa. Journal of Policy Modeling, 40(6), 1104–1125. https://doi.org/10.1016/j.jpolmod.2018.08.001
- Kahouli, B., & Maktouf, S. (2015). The determinants of FDI and the impact of the economic crisis on the implementation of RTAs: A static and dynamic gravity model. International Business Review, 24(3), 518–529. https://doi.org/10.1016/j.ibusrev.2014.10.009
- Kremer, S., Bick, A., & Nautz, D. (2013). Inflation and growth: New evidence from a dynamic panel threshold analysis. Empirical Economics, 44(2), 861–878. https://doi.org/10.1007/s00181-012-0553-9
- Lee, C. C., & Chang, C. P. (2009). FDI, financial development, and economic growth: International evidence. Journal of Applied Economics, 12(2), 249–271. https://doi.org/10.1016/S1514-0326(09)60015-5
- LexisNexis Risk Solutions. (2021). True Cost of Financial Crime Compliance Study (Issue October). https://risk.lexisnexis.com/insights-resources/research/true-cost-of-financial-crime-compliance-study-global-report
- Liu, H., Aminul, M., Asif, M., Ph, K., & Finance, D. (2020). Does financial deepening attract foreign direct investment ? Fresh evidence from panel threshold analysis. Research in International Business and Finance, 53 Oct , 101198. https://doi.org/10.1016/j.ribaf.2020.101198
- Loayza, N., Villa, E., & Misas, M. (2019). Illicit activity and money laundering from an economic growth perspective: A model and an application to Colombia. Journal of Economic Behavior and Organization, 159 March , 442–487. https://doi.org/10.1016/j.jebo.2017.10.002
- Luan, B., Huang, J., & Zou, H. (2019). Domestic R & D, technology acquisition, technology assimilation and China’s industrial carbon intensity : Evidence from a dynamic panel threshold model. Science of the Total Environment, 693 Nov , 133436. https://doi.org/10.1016/j.scitotenv.2019.07.242
- Mccarthy, K. J., Van Santen, P., & Fiedler, I. (2015). Modeling the money launderer: Microtheoretical arguments on anti-money laundering policy. International Review of Law & Economics, 43(October 2005), 148–155. https://doi.org/10.1016/j.irle.2014.04.006
- Mcdonell, R. (1998, May, 7–8). Money laundering methodologies and international and regional counter-measures. Paper Presented at the Conference Gambling, Technology and Society: Regulatory Challenges for the 21st Century, Convened by the Australian Institute of Criminology in Conjunction with the Australian Institute for Gambling Research and Held in Sydney (Sydney).
- McDowell, J., & Novis, G. (2001). The consequences of money laundering. Economic Focus, 6(2), 6–8 https://www.ojp.gov/ncjrs/virtual-library/abstracts/consequences-money-laundering-and-financial-crime.
- Mekpor, E. S., Aboagye, A., & Welbeck, J. (2018). The determinants of anti-money laundering compliance among the financial action task force (FATF) member states. Journal of Financial Regulation and Compliance, 26(3), 442–459. https://doi.org/10.1108/JFRC-11-2017-0103
- Nugraha, Y. W. I. (2013). The impact of corruption and money laundering on foreign direct investment in ASEAN. Jurnal Ekonomi Kuantitatif Terapan, 6(2), 106–111.
- Ofoeda, I., Agbloyor, E. K., Abor, J. Y., & Osei, K. A. (2020, April). Anti‐money laundering regulations and financial sector development. International Journal of Finance & Economics Dec , 1–20. https://doi.org/10.1002/ijfe.2360
- Okafor, G. (2015). Locational determinants of US outward FDI Into Sub-Saharan Africa. The Journal of Developing Areas, 49(1), 187–205. https://doi.org/10.1353/jda.2015.0000
- Perez, M. F., Brada, J. C., & Drabek, Z. (2012). Illicit money flows as motives for FDI. Journal of Comparative Economics, 40(1), 108–126. https://doi.org/10.1016/j.jce.2011.03.007
- Raweh, B. A., Erbao, C., & Shihadeh, F. (2017). Review the literature and theories on anti-money. Asian Development Policy Review, 5(3), 140–147. https://doi.org/10.18488/journal.107.2017.53.140.147
- Roodman, D. (2009). How to do xtabond2: An introduction to difference and system GMM in Stata. Stata Journal, 9(1), 86–136. https://doi.org/10.1177/1536867x0900900106
- Saini, N., & Singhania, M. (2018). Determinants of FDI in developed and developing countries: A quantitative analysis using GMM. Journal of Economic Studies, 45(2), 348–382. https://doi.org/10.1108/JES-07-2016-0138
- Seetanah, B., & Rojid, S. (2011). The determinants of FDI in Mauritius: A dynamic time series investigation. African Journal of Economic and Management Studies, 2(1), 24–41. https://doi.org/10.1108/20400701111110759
- Seo, M. H., Kim, S., & Kim, Y. J. (2019). Estimation of dynamic panel threshold model using Stata. Stata Journal, 19(3), 685–697. https://doi.org/10.1177/1536867X19874243
- Seo, M. H., & Shin, Y. (2016). Dynamic panels with threshold effect and endogeneity. Journal of Econometrics, 195(2), 169–186. https://doi.org/10.1016/j.jeconom.2016.03.005
- Svirydzenka, K. (2016). . Introducing a new broad-based index of financial development.I (IMF Working Paper, International Monetary Fund https://www.imf.org/external/pubs/ft/wp/2016/wp1605.pdf) doi:.
- Taghizadeh-Hesary, F., Phi, N. T. M., Hong, H. H. T., & Chu, V. T. (2019). Does financial integration matter for financial development? Evidence from the East Asian and Pacific Region. Journal of Economic Integration, 34(4), 591–618. https://doi.org/10.11130/jei.2019.34.4.591
- Tsingou, E. (2010). Global financial governance and the developing anti-money laundering regime: What lessons for international political economy. International Politics, 47(6), 617–637. https://doi.org/10.1057/ip.2010.32
- UNCTAD. (2018). World investment report; Foreign direct investment inflows in Malaysia. www.unctad.org/diae
- Van der Zahn, M., Makarenko, M. I., Tower, G., Kostyuk, A. N., Barako, D., Chervoniaschaya, Y., Brown, A. M., & Kostyuk, H. (2007). The anti-money laundering activities of the central banks of Australia and Ukraine. Journal of Money Laundering Control, 10(1), 116–133. https://doi.org/10.1108/13685200710721908
- Warf, B. (2002). Tailored for Panama: Offshore banking at the crossroads of the Americas. Geografiska Annaler, Series B: Human Geography, 84(1), 33–47. https://doi.org/10.1111/j.0435-3684.2002.00112.x
- Weeks-Brown, R. (2018). Straight Talk: Cleaning Up. FInance & Development, 55 004 . https://doi.org/10.7591/cornell/9780801450723.003.0007
- Wei, S. J. (2000). How taxing is corruption on international investors. Review of Economics and Statistics, 82(1), 1–11. https://doi.org/10.1162/003465300558533
- Windmeijer, F. (2005). A finite sample correction for the variance of linear efficient two-step GMM estimators. Journal of Econometrics, 126(1), 25–51. https://doi.org/10.1016/j.jeconom.2004.02.005
- Wooldridge, J. (2012). . In Introductory econometrics: Amodern approach (5th (South-Western: Cengage Learning) edn). https://doi.org/10.4324/9781351140768-8
- Yepes, V. C. 2011 Compliance with the AML/CFT international standard: Lessons from a cross-country analysis. (). . IMF Working Paper, WP/11/177 (International Monetary Fund).
- Zaman, K., Shah, I. A., Mushtaq Khan, M., & Ahmad, M. (2012). Macroeconomic factors determining FDI impact on Pakistan’s growth. South Asian Journal of Global Business Research, 1(1), 79–95. https://doi.org/10.1108/20454451211207598
- Zhang, J., Zhao, Z., & Jian, W. (2019). Do cash flow imbalances facilitate leverage adjustments of Chinese listed firms ? Evidence from a dynamic panel threshold model. Economic Modelling 89 Jul. https://doi.org/10.1016/j.econmod.2019.10.016