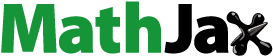
Abstract
We employ a Boxplot method for detecting and analyzing outlying daily returns of 14 international stock market indices sampled from around the world. The main objective of the paper is to provide an extensive analysis of the main characteristics, features and effects of the detected outlier returns. The results show that from about 4–10% of observations constitute outlying returns with an average of 6%. Conservatively, about 1.4% of return series are extreme outliers. Negative outliers are found more frequent, influential, severe and transmissible. The bulk of detected outliers are found to be in the magnitude of three standard deviations. Also, outliers tend to cluster together, both within individual return series over time and across stock markets. We find a sequential pattern in outlier occurrence within individual return series, and a concurrent pattern across stock markets. Moreover, adjusting for outlying returns leads to a decrease in standard deviation, negative skewness and kurtosis by about 18%, 74% and 69% on average, respectively. We do not find consistent evidence that advanced and well-developed stock markets have less frequent and/or sever outliers. Overall, the results and analysis of the paper provide important considerations about international stock market returns which are relevant to stock investment, portfolio and risk management. The results show that the best (worst) outlying returns which represent about only 1% of the return observations have an enormous effect on the stock return performance and realization.
Public interest statement
Stock markets are among the main destinations for investment and portfolio management. Aberrant stock market fluctuations which result in outlying returns constitute very important issues to be examined and analyzed carefully. Having a better understanding of the features and effects of stock outlying returns is relevant to stock investment, portfolio and risk management as well as diversification decisions. For 14 international stock markets sampled from around the world, the paper provides an extensive analysis of the features, characteristics and effects of the detected outlying returns, including frequency, signs, magnitude, severity and influence. We also examine and analyze the potential patterns of outlying returns within individual return series as well as across the stock markets of our sample. The paper also examines the effect of outlying returns on some stock return statistics. Importantly, the paper examines the impact of outlying returns on the performance and realization of returns in stock markets.
1. Introduction
The existence of outlying and aberrant values can lead to serious difficulties in statistical analysis of data set under consideration. Outliers in a data set can result in erroneous statistical inferences, unreliable hypothesis testing and inaccurate results and conclusions. Moreover, detected outliers can have information content which can help in decision making process in many settings and applications. Therefore, detecting, analyzing and accounting for outliers have gained considerable attention in many disciplines and applications. One of these disciplines is financial and economic time series.
It is well documented that most financial and economic time series have heavy-tailed distributions. One agreed reason for this phenomenon which typically introduced in the literature is the existence of outlying observations. The existence of aberrant observations in financial and economic time series has substantial effects on financial and economic modelling in many areas. Consequently, outliers are increasingly being an important subject of research for various fields of finance and economics. This includes macroeconomics (e.g., Balke & Fomby, Citation1994; Shen et al., Citation2018; Tolvi, Citation2001), Metal and oil industry (e.g., Behmiri & Manera, Citation2015; Ju et al., Citation2016), foreign exchange markets (e.g., Franses et al., Citation2004; Villavicencio, Citation2008), commodity and electricity industry (e.g., Afanasyev & Fedorova, Citation2019; Stanley, Citation2003; Thomas et al., Citation2011) and stock markets (e.g., Ané et al., Citation2008; Schluter & Trede, Citation2008; Charles, Citation2008; Grané & Veiga, Citation2010; Haggard et al., Citation2015; Loperfido, Citation2020 among others). Motivated by the importance of investigating outliers in stock markets, in this paper we examine and analyze outliers in returns of an extended sample of various stock markets from around the world.
The importance of detecting and analyzing anomalies in stock market movements stems from their implications and relevance to many financial activities and applications, including volatility modelling and forecasting (e.g., Ané et al., Citation2008; Charles, Citation2008; Franses & Ghijsels, Citation1999; Schluter & Trede, Citation2008), risk measurement (e.g., Berkowitz & O’Brien, Citation2002) and asset pricing (e.g., Aronne et al., Citation2020). Moreover, detecting and analyzing outliers per se can convey important information on market dynamics and behaviour which, in turn, can results in more reliable decisions regarding risk and portfolio management, market timing (e.g., Estrada, Citation2008, Citation2009), and security selection and asset allocation (e.g., Bauer et al., Citation2004; Welsch & Zhou, Citation2007).
Different models and algorithms have been introduced in the literature for detecting and handling outliers in stock market movements for different purposes. The bulk of those methods and approaches is based on GARCH and ARMA models and their variants (see, e.g., Ané et al., Citation2008; Charles, Citation2008; Franses & Ghijsels, Citation1999; Schluter & Trede, Citation2008). In addition, Wavelet models, density-based methods, fractal theory, and recently, kurtosis-based projection pursuit have also been employed (e.g., Grané & Veiga, Citation2010; Loperfido, Citation2020; Sun et al., Citation2008; Zhao et al., Citation2012). Despite the contributions of these models and approaches, they are greatly sensitive to the data distributional assumptions, the model parameter estimates and the presumed data generating processes.
Differently, in this paper, we employ Boxplot method for identifying and examining possible outliers in global stock market fluctuations. In addition to the straightforwardness of the Boxplot method, it has a great advantage of not having strict assumptions regarding the data generating processes and the underlying probability distributions of the data, making it more flexible to be used and implemented in practical settings (Shevlyakov et al., Citation2013). In other words, the Boxplot method does not involve processing data series in a specific form, but rather it maintains the original data and detect outliers depending on the features and properties within the data series itself. Another advantage of the Boxplot method is that it is independent of the extremely aberrant observations when computing a measure of data dispersion. Rather, the inner and outer outlier fences are determined based on data quartiles so that they are not distorted by a few extreme values (Schwertman et al., Citation2004).
By outlying financial returns, such as the daily stock returns, we mean the high and low returns, which result from extreme price variations, that are extremes relative to the rest of the daily returns. In this sense, investigating outliers in the context of financial returns is important because of their effects on the behaviour of return performance and realization from financial investments. Whether returns are achieved through normal and smooth price changes or through a few numbers of extreme price changes over the holding period of investments are essential considerations for investors in financial instruments. In other words, the potential effects of outlying returns on the performance of investment and portfolio returns can impact the investment and portfolio choices of the different types of investors.
The focus of the paper is on the information content of stock outlying returns. Specifically, our purpose is to provide a detailed analysis of the detected outliers’ characteristics and features. To this end, we adopt Boxplot method for identifying and scrutinizing potential outliers in daily returns of 14 stock markets. Our market sample encompasses the major stock indices of USA, UK, Germany, France, Japan, Russia, China, Brazil, South Africa, India, Turkey, Hong Kong, Saudi Arabia and South Korea. Our sample period extends from November 1997 to April 2020. After constructing outlier fences within the Boxplot settings, we analyze detected outliers in terms of frequency, sign, magnitude, severity and effects. We also examine the influence of return outliers on return series divided into good and bad days. In addition, we look at the distribution of detected outliers over years and examine whether they exhibit any recognizable patterns within and across stock market indices. Moreover, we investigate the sequential pattern of outliers occurrence within return series for every stock market, and the concurrent pattern of outliers occurrence across stock markets. We also conduct an exercise to adjust for outliers and examine their effects on the distribution of return series. In addition, we investigate the effects of outlying returns on the performance and realization of stock market returns.
The analysis and results of the paper are relevant in the contexts of stock investment and portfolio management. Considering frequency, classification, magnitude, effects and patterns of outlying returns in stock markets can lead to better portfolio management and selection so that asset allocation decisions can be made with more efficiency and effectiveness. This is because outlier returns are found to have considerable impact on the long-run performance of stock portfolios (e.g., Estrada, Citation2008, Citation2009). For example, we find that the sum of positive outlying returns accounts for up to about 33% of the sum of all positive returns over the sample period, and the sum of negative outliers accounts for up to about 51% of the sum of negative returns. In addition, we find that the best (worst) outlying returns which represent about only 1% of return observations have a massive impact on stock return performance and realization. Moreover, it is essential to consider outlying returns in the framework of mean-variance optimization analysis. This is because few coincidental outliers can noticeably affect correlations between stock returns (Kim et al., Citation2015). This in turn can affect the diversification decisions of portfolio managers. For example, our analysis of the concurrent pattern of outliers across stock markets shows that considerable number of outliers occur simultaneously in stock markets. China and Turkey are found to have the smallest numbers of concurrent outliers with other stock markets, implying that they can provide greater diversification benefits. Outliers’ analysis is also important and relevance for market risk management. The variance of returns that is the mostly used measure of investment risk may be no longer valid as an accurate risk measure in the presence of influential outlying returns. In addition, some models of measuring market risk such as value at risk (VaR), extreme value theory (EVT) and expected shortfall can be substantially affected without properly handling outliers. So, understanding the features, characteristics, pattens and effects of outlying returns would help in developing more reliable market risk models. For example, our analysis shows that outliers have considerable impacts on the higher moments of the return distribution. Policy makers may also need to pay attention to outlier occurrence in stock markets. One of the most important impacts of outliers is the increased volatility of stock markets. Higher stock market volatility can lead to more expensive funds for firms considering having financing from stock markets. This will affect the investment decisions of the firms. Such an increased cost of financing can then impair financial development and economic growth. Therefore, a better understanding of the dynamics of stock markets and the effects of outliers by policy makers might lead to structural and regulatory changes that help in maintaining the smooth functioning and stability of financial systems. Overall, the paper contributes to the literature in two ways. First, the paper provides an extensive analysis of outliers in returns of a large sample of global stock market indices. Our market sample comprises 14 stock indices from different regions with different degrees of development. Second, the paper introduces the Boxplot method as a useful, practical and effective tool for identifying and screening outliers in stock market returns.
The rest of the paper is organized as follows: in Section 2, we review some outlier literature; in Section 3, we explain the Boxplot methodology and present our data set; in Section 4, we present our analysis, results and discussion; and in Section 5, we conclude the paper.
2. Literature review
Outliers and their effects are investigated in literature from different points of view and for different purposes. Some early studies investigate outliers in macroeconomic time series and examine their effect on non-linearity which usually found in macroeconomic variables. It is found that outliers are existent in a large set of macroeconomic variables, and that controlling for outliers can eliminate those non-linearities (Balke & Fomby, Citation1994; Tolvi, Citation2001). González-Sánchez (Citation2021) examined the relationship between time scaling property and outliers in daily returns of many stocks, exchange rates and commodities. He found that time scaling is a consequence of the outliers’ existence. Aronne et al. (Citation2020) proposed Weighted Forward Search (FSW) method to study the effect of outliers on asset pricing data. When using monthly data, they found that the existence of outliers in portfolios can lead to the marginal rejection of the Fama-French three-factor model. As distributions of many variables in finance and economics are heavy-tailed such as stock market returns, Raggad and Boutahar (Citation2012) examined the behaviour of tail in returns of international stock market indices. They found that tail behaviour of stock returns is instable, but rather it is subject to some structural breaks which found to coincide with major financial and economic events and crises. Schluter and Trede (Citation2008) developed a procedure for identifying multiple outliers when having heavy-tailed distributions. They applied their procedure on Dow Jones Industrial Average return data and found that Black Monday market crash is not unusual structural event and so it is not an outlier.
Some authors focus on the effect of outliers on volatility modelling and forecasting. Behmiri and Manera (Citation2015) employed GARCH-type models and examine the effect of outliers on volatility persistence in ten precious and industrial metals. They fpund that adjusting for outliers can improve volatility modelling. Charles (Citation2008) applied GHARCH models for detecting and correcting outlying returns in 17 French stocks and CAC40 index. He showed that parameter estimates of volatility dynamics equation are biased with the existence of return outliers. He also found that volatility forecasting getting better when accounting for detected return outliers. Grané and Veiga (Citation2010) proposed a wavelet-based approach for detecting and correcting outliers that can be used in several volatility models. They found their approach effective when applied on three stock market indices of S&P500, Dow Jones and FTSE100. Ané et al. (Citation2008) introduced AR(1)-GRACH(1,1)-based algorithm for outliers identification. They applied their approach on five Asian stock markets using weekly frequency return data. They showed that their approach of identifying and correcting outliers improves forecasting accuracy compared to the classical GARCH models. Charles and Darné (Citation2006) examined outliers in 10 international stock market indices to investigate the effect of the terrorist attacks in USA on 11 September 2001. They found that international stock markets exhibit temporary and permanent large shocks in response to the event and showed that controlling for such events can improve modelling volatility in stock market prices.
Focusing on the information content of outliers, Ferdousi and Maeda (Citation2006) examined outliers in stock market data using Peer Group Analysis (PGA) for detecting stock fraud. In the same context, Li et al. (Citation2016) used Boxplot method to examine its ability for detecting insider trading in Chinese stock market as outlier observations. From another perspective, Welsch and Zhou (Citation2007) employed various statistical methods, which aim at reducing the effect of outliers and increasing the stability of portfolio performance. Estrada (Citation2009) examined and quantify the effect of outliers on the long-run performance of the Dow Jones Industrial Average index over 107 years. He found that outlying returns have considerable influence on the long-term performance of stock portfolios. In this paper, we analyze outlying returns of several international stock market indices. By this, we aim to enrich the literature with an extensive analysis of outliers in stock returns and provide more insights into their aspects and effects.
3. Methodology and data
3.1. Methodology
There are many definitions of outliers. We adopt the definition of outliers as they are the aberrant observations in the data series that are numerically away from the rest of the observations. We employ Boxplot method (Tukey, Citation1977) for detecting and analyzing the potential outliers in daily returns of 14 stock market indices. The Boxplot method of identifying outlaying observations is based on selected order-statistics. Specifically, the method is built upon identifying the sample quartiles (hinges) of the data set and then constructing outlier fences (a lower fence and an upper fence). Observations of the data set which lie outside (on both directions) of the constructed fences are considered possible outliers. The lower and upper fences are constructed as follows:
where and
are the first and third sample quartiles,
is the sample interquartile range i.e.,
and
is a constant.
Depending on the value of fence constant , the Boxplot method enable us to construct two sets of fences: inner fences (
) and outer fences (
) each with a lower fence (
,
) and an upper fence (
,
). The standard values of
for inner fences and outer fences are 1.5 and 3, respectively. So, we have the following sets of fences:
,
for inner fences. (3)
,
for outer fences. (4)
Detected outlaying observations can then be classified based on the inner and outer fences. Specifically, an observation is regarded mild outlier if
whereas an observation is regarded extreme outlier if
3.2. Data
We apply the Boxplot method with the purpose of identifying and analyzing abnormal fluctuations of 14 stock market indices on daily basis. Our data set comprises 14 stock market indices sampled from around the world. We collect the daily closing prices for each index from various sources. Namely, Investing.com, Yahoo finance and Wall Street journal. Our data set encompasses the stock market indices of USA (S&P500), U.K. (FTSE AllShare), Germany (DAX), France (CAC 40), Japan (Nikkei 225), Russia (RTSI), China (shanghai composite), Brazil (IBOVESPA), South Africa (SA40), India (Nifty 50), Turkey (BIST 100), Hong Kong (HIS), Saudi Arabia (Tadawul All Share) and South Korea (KOSPI). In our choice of this sample of stock markets, we consider the geographical distribution. The stock markets in our sample are meant to be from different regions around the world. In addition, we consider a sample of stock markets that are varying in size and advancement. The sample comprises stock markets of developed, less developed and developing countries. USA, UK, France, Germany and Japan are amongst the world leading developed markets. As a representative of the large less developed and developing markets, we include BRICS countries (Brazil, Russia, India, China and South Africa). As medium size developing markets, we include Turkey and Hong Kong. Moreover, as a representative of Middle Eastern countries we include Saudi Arabia, and from South-East Asian countries we include South Korea. The sample period extends from November 1997 to April 2020.
We calculate daily returns for every stock market index from the daily closing prices in the form of log-difference. That is, where
is the observed closing price at time
. Table reports descriptive statistics of daily returns. Despite the high frequency data of daily returns, normality test can be rejected for all indices. Except for Brazil and Hong Kong which have significant positive skewness at conventional significance levels, the rest of indices have significant negative skewness except for Turkey.Footnote1 All indices have large significant positive kurtosis with some variations. Brazil has the largest kurtosis of 17.21, while China has the lowest kurtosis of 7.94. Leptokurtocity in return of stock indices is a stylized fact. One reason for this is the existence of outlying and abnormal fluctuations in return series.
Table 1. Descriptive statistics
Table presents the inner and outer fences for each stock index. Lower fences range from −4.289% (Turkey) to −1.801% (Saudi Arabia) for inner fences, and from −7.555% (Turkey) to −3.218% (Saudi Arabia) for outer fences. The average of lower-inner fences is −2.955%, and the average of lower-outer fences is −5.201%. Upper fences range from 1.977% (Saudi Arabia) to 4.420%(Turkey) for inner fences, and from 3.394% (Saudi Arabia) to 7.686% (Turkey) for outer fences. The average of upper-inner fences is 3.060%, and the average of upper-outer fences is 5.315%. On average, less developed and developing stock markets tend to have greater lower and upper fences in absolute values compared to more developed markets. For example, Russia, Brazil, Turkey and Hong Kong have greater fences compared to USA, UK, France and Germany. Figure depicts the series of index price along with daily returns for every market. In addition, we outline the inner (solid horizontal lines) and outer (dashed horizontal lines) fences. By and large, it can be noticed that most of the detected outliers are concentrated in the early years of the sample period, in the period 2007–2009 and at the very end of the sample period for most of the indices. Despite the figure is useful in terms that it gives a general view of the frequency, magnitude, sign and timing of return outliers across all indices, it is far from being satisfactory. Thus, the following sections provide results, analysis and discussion for these aspects of the detected potential outliers in more details.
Table 2. Outlier fences
Figure 1. Index price and return series. Notes: the figure depicts time series of stock index prices and stock index daily returns. Right scale of index price is in thousands. Left scale is for index return. Solid horizontal lines represent outlier inner fences. Dashed horizontal lines represent outlier outer fences.
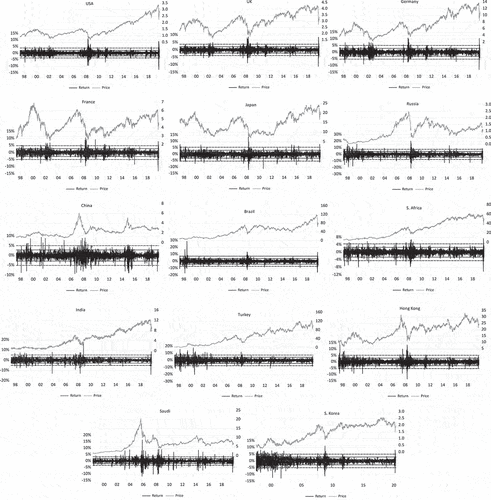
4. Empirical results and analysis
Throughout our analysis and discussion, we present the results for two sets of outliers. Namely, all outliers and extreme outliers. By all outliers we mean mild outliers plus extreme outliers. The reason for separating extreme outliers from all outliers is to show the results from a more conservative point of view. This is because adopting outer fences of the Boxplot method is deemed a conservative approach for identifying outlying observations (Schwertman et al., Citation2004).
4.1. Number and sign of outliers
Table presents an overview on the number of potential outliers, the percentage of outliers to the number of observations and the percentages of positive and negative outliers for each stock market index. For all markets, the average number of all outliers is 345, while the average number of extreme outliers is 77. The number of all outliers (extreme outliers) ranges from as low as 210 (41) to as high as 575 (207). Saudi Arabia has the largest number of outliers (575, 207), followed by South Korea (484, 114), USA (409, 93), Russia (372, 85) and China (350, 77). Brazil, Japan and South Africa are among the markets which have the smallest number of outliers. On average, all outliers represent 6.1% of the daily return observations. This percentage ranges from as high as 10.2% (Saudi Arabia) followed by 8.6% (South Korea) and 7.2% (USA), to as low as 3.8% (Brazil) followed by 4.3% (Japan). Extreme outliers represent 1.4% of the number of observations on average. The percentage of extreme outliers to the number of observations ranges from as high as 3.7% (Saudi Arabia) followed by 2.0% (South Korea) and 1.6% (USA), to as low as 0.7% (Brazil) followed by 0.8% (Japan and South Africa). We note that the percentages of outliers to the number of observations are also the largest for Saudi Arabia, South Korea, USA, Russia and China, while they are the smallest for Brazil, Japan and South Africa.
Table 3. Number and classification of outliers
Remarkably, we note that negative outliers are more frequent than positive outliers for all markets. On average, negative outliers represent 57% in the case of all outliers with little variation across the markets. In the case of extreme outliers, the variation of the percentage of negative outliers is larger with an average of 59%. The percentage ranges from 52% (USA and Hong Kong) to 66% (UK, China and Saudi Arabia).
We can put these findings into perspective by comparing them with those of Ané et al. (Citation2008), who analyze outliers in weekly return series of five Asian stock markets by employing AR(1)-GARCH(1,1) model over the period January 1990 to December 2005. They find that outlaying returns represent as high as 3.73% (Korea) and as low as 1.32% (Taiwan) of observations with an average of 2.38%. For three out of the five markets, they find that negative outliers account for 52.5% of return outliers on average. Also, González-Sánchez (Citation2021) investigateed outliers in daily returns of numerous stocks, exchange rates and commodities over the period of January 2014 to December 2018. He found that the percentage of the number of outliers to the number of observations ranges from 1% to 6%.
In order to shed more light on the frequency of extreme outliers relative to all outliers, in Table we present the percentage of extreme outliers to all outliers, the percentage of extreme positive outliers to all positive outliers and the percentage of extreme negative outliers to all negative outliers for every stock market. Roughly, about one-fifth of detected outliers are extremes. Specifically, Extreme outliers represent as high as 36% (Saudi Arabia) and as low as 17% (UK and France) of all outliers with an average of 22%. Extreme positive outliers represent as high as 29% (Saudi Arabia) followed by 26% (USA), and as low as 14% (UK) of all positive outliers with an average of 20%. Extreme negative outliers represent as high as 42% (Saudi Arabia) followed by 26% (China), and as low as 17% (France, South Africa and Hong Kong) of all negative outliers with an average of 22%.
4.2. Magnitude and severity of outliers
Preliminary, for having a general view of the size of the abnormal daily returns in stock market indices we first report the averages of four sets of outliers in the upper part of Table . Namely, all positive outliers, all negative outliers, extreme positive outliers and extreme negative outliers. The average of all positive outliers ranges from as low as 3.11% (UK) to as high as 6.58% (Russia), while the average of all negative outliers ranges from −3.18% (UK) to −6.72% (Russia). The average of extreme positive outliers ranges from as low as 5.22% (USA) to as high as 11.25% (Brazil), while the average of extreme negative outliers ranges from −5.12% (Saudi Arabia) to −10.71% (Russia). Russia, Brazil and Turkey have the greatest averages, while UK, USA, South Africa and Saudi Arabia have the lowest ones. Note that this is consistent with their outlier fences.
Table 4. Magnitude of outliers
In the second part of Table , we present the sums of the four sets of outliers as defined above. The sum of all positive outliers ranges from as low as 406% (South Africa) to as high as 1,047% (Russia) with an average of 660%, while the sum of all negative outliers ranges from −562% (South Africa) to −1,431% (Russia) with an average of −856%. The sum of extreme positive outliers ranges from as low as 108% (UK) to as high as 367% (Saudi Arabia) with an average of 218%, while the sum of extreme negative outliers ranges from −157% (South Africa) to −701% (Saudi Arabia) with an average of −310%. We note that the sum of negative outliers is greater than the sum of positive outliers for all indices (except for Hong Kong in the case of extreme outliers), resulting in negative sum of outliers in total. This reflects the higher frequency of negative outliers and indicates that negative outliers might have larger magnitudes. In addition, about one-third of the sums of all positive and negative outliers comes from extreme outliers on average.
Importantly, in the third part of Table , we present the results of investigating the magnitude and severity of outliers in terms of unconditional standard deviation (). It can be noticed that the vast majority of daily return outliers are concentrated in the magnitudes of
,
,
and
for all indices. The number of outliers of these magnitudes accounts for 95.6% of the total number of outliers for all indices (4,825). Interestingly, we note that the greatest numbers of outliers are in the magnitude of
for all indices except for Saudi Arabia where the greatest number of outliers is in the magnitude of
. Apart from Saudi Arabia, the number of outliers in the magnitude of
accounts for 58.3% of the total number of outliers. This percentage is followed by 16.7% for
, 14.6% for
, and 6.2% for
. Individually, the percentage of outliers in the magnitude of
to the number of outliers for each index ranges from as low as 43% (USA) to as high as 70.5% (South Africa) with an average of 59.6%. We also note that USA, UK, France, Brazil, India and Saudi Arabia have at least one outlier of magnitude
or greater, while only China has no outliers of magnitude greater than
.
In the last part of Table , we present the largest positive and negative outliers in terms of unconditional standard deviation for every stock market index. We note that USA, UK, France and India have the greatest negative outliers relative to the other markets, while Brazil, India and Saudi Arabia have the greatest positive outliers. Note that these markets are among the markets that have the largest kurtosis. Interestingly, despite having well developed stock markets, USA and UK are amongst the markets that have more severe return outliers. Remarkably, for 9 out of the 14 markets the largest negative outliers in absolute values are greater than the largest positive outliers, implying that the largest negative outliers are more severe than the largest positive outliers for most of stock markets in our sample. In addition, this finding along with the previous finding that negative outliers are more frequent can explain the significant negative skewness for most return series as noticed in Table .
4.3. Outliers and return series
We can have more insights into the frequency, size and effect of the abnormal index price movements by investigating their numbers and sums relative to the return series classified into positive and negative returns. To make sense of our analysis, in the first and second parts of Table , we first provide an overview of the performance of each stock market in terms of the sum of return series, and in terms of the number of good days and bad days relative to the total number of return observations. The upper part of Table shows that the sum ranges from as low as 20.20% (Japan) to as high as 357.03% (Turkey) with an average of 133.87%. We note that over our sample period Turkey, India, South Africa and Brazil have the largest sums, while Japan, UK, France and Hong Kong have the lowest ones. The second part of Table shows that the number of days of positive return is greater than the number of days of negative return for all markets. On average, the number of positive returns represents 52.7%, while the number of negative returns represents 47.1% of the total number of return observations.
Table 5. Outliers and return series
In the third part of Table , we provide the percentages of the number of positive and negative outliers to the number of positive and negative returns. The number of all positive outliers represents as low as 3.3% (Brazil) and as high as 8.2% (Saudi Arabia) of the number of positive returns with an average of 5.1%, while the number of extreme positive outliers represents as low as of 0.6% (Japan and Brazil) to as high as 2.6% (Saudi Arabia) with an average of 1.1%. On the other hand, the number of all negative outliers represents as low as 4.3% (Brazil) and as high as 14.0% (Saudi Arabia) of the number of negative returns with an average of 7.5%, while the number of extreme negative outliers represent as low as 0.9% (Japan and Brazil) and as high as 5.8% (Saudi Arabia) with an average of 1.8%. We note that for all indices negative outliers are more frequent with respect to negative returns compared to positive outliers with respect to positive returns.
In the last part of Table , we present the percentages of the sums of positive and negative outliers to the sums of positive and negative returns. On average, all positive outliers account for 21.2% of the sum of positive returns while all negative outliers account for 29.0% of the sum of negative returns. The percentages of the sum of all positive outliers to the sum of positive returns are the largest for Saudi Arabia, South Korea and USA (33.3%, 29.0% and 24.8%, respectively), while they are the smallest for Japan, Brazil and South Africa (14.7%, 15.1% and 15.5%, respectively). Similarly, the percentages of the sum of all negative outliers to the sum of negative returns are the largest for Saudi Arabia, South Korea and USA (51.6%, 37.7% and 33.6%, respectively), while they are the smallest for Brazil, Japan and South Africa (19.0%, 21.3% and 23.4%, respectively). For extreme outliers, extreme positive outliers account for 7.0% of the sum of positive returns, while extreme negative outliers accounts for 10.6% of the sum of negative returns on average. The percentages of the sum of extreme positive outliers to the sum of positive returns are the largest for Saudi Arabia, South Korea and USA (15.2%, 10.6% and 9.9%, respectively), while they are the smallest for Japan, South Africa and Brazil (4.3%, 4.4% and 4.8%, respectively). The percentages of the sum of extreme negative outliers to the sum of negative returns are the largest for Saudi Arabia, South Africa and Russia (31.0%, 12.8% and 12.6%, respectively), while they are the smallest for Japan, Brazil and South Africa (6.2% and 6.5%, respectively). We note that for all indices the percentages of the sum of negative outliers to the sum of negative returns are higher than the percentages of the sum of positive outliers to the sum of positive returns. This implies that for all stock markets of our sample negative outliers are more influential and severe compared to positive outliers.
4.4. Distribution of outliers over years
The time of occurrence of extreme return fluctuations and whether they exhibit identifiable patterns within and/or across stock markets are important aspects to be considered and investigated when studying and analyzing stock returns. This is especially relevant when analyzing stock markets so that decisions of investment diversification and asset allocation can be made with greater efficiency and effectiveness. With this in mind and from a conservative point of view, in Table we present the number of extreme outliers in each year for every stock market.
Table 6. Distribution of outliers by year: extreme outliers
One important pattern which can be identified is that in some specific time periods outliers tend to occur in almost all markets, implying outlier clusters across stock markets. Clearly, four clusters of outliers (including both positive and negative) across all markets can be noticed in periods of the late 1990s, early 2000s, 2008–2009 and early 2020. In these time periods, almost all markets exhibit noticeable number of extreme returns. Global events occurred in these time periods which can possibly explain the aforementioned outlier clusters include Asian and Russian crises (late 1999s), Dot-Com bubble and crash (early 2000s), global financial crisis (2008–2009) and the first wave of Coronavirus (early 2020). Excluding Saudi Arabia that has the most turbulent stock market in terms of the number of outliers over the whole sample period, we note that the number of outliers in 1998–1999, 2001–2002, 2008–2009 and early 2020 accounts for 74.1% of the total number of outliers (873). The year 2008 has the largest number of outliers (240), which account for 27.5% of the total number of outliers, followed by 1998 with 126 outliers and a percentage of 14.4%, 2020 with 79 outliers and a percentage of 9.0% and 2002 with 57 outliers and a percentage of 6.5%.
Looking at outliers for every market individually, another important pattern can be identified. We note that all markets exhibit consecutive years of outliers, implying outlier clusters within return series. At least two to three outlier clusters can be identified for each series. For example, most outliers in USA return series are concentrated in two periods with consecutive years of outliers, namely 2000–2003 with 16 outliers which account for 17.2% of the USA total number of outliers, and 2008–2011 with 52 outliers which account for 55.9% of the total number of outliers. UK, Germany and France return series have two coinciding outlier clusters in periods of 2001–2003 and 2008–2011. On average, the first and second clusters account for 30% and 48% of their total number of outliers, respectively. India return series has two outlier clusters in periods of 1998–2001 and 2006–2009. The first cluster accounts for about 30% while the second cluster accounts for 48.5% of its total number of outliers. China return series has three longer outlier clusters in periods of 1998–2003, 2005–2010 and 2013–2016. Russia, Saudi Arabia and Turkey have the longest consecutive years of turbulence. Russia return series has 8 consecutive years of outliers, 1997–2004, which account for 55.3% of its total number of outliers. Turkey has 7 consecutive years of outliers, 1997–2003, which accounts for 88.2% of its total number of outliers.
4.5. Outlier groupings within and across return series
In this section, we look more closely and further investigate the sequential pattern of outliers occurrence for each stock market return series and the concurrent pattern of outliers occurrence across stock markets. The analysis of sequential pattern is based on all detected outliers, while the analysis of concurrent pattern is based on extreme outliers. Table presents the numbers and percentages of outliers that occur sequentially within a brief time period of seven days regardless of the sign of the outliers. Specifically, column “” presents the numbers and percentages of outliers which are followed by another outlier consecutively in the next day. Column “
” presents the numbers and percentages of outliers which are followed by another outlier after two days, and so forth. We note that on average 17% of the outliers are followed by another outlier in the next day. This percentage is as large as 25% for Saudi Arabia and as law as 11% for France and Japan. For USA, Russia, Brazil, India, Turkey and Saudi Arabia, about one-fifth of the outliers are followed by another outlier in the next day. The numbers and percentages of the sequential outliers decrease as we move to the longer timeframes. However, for almost all stock markets more than one-third of the outliers are followed by other outliers within a time period of 3 days in total. Remarkably, we note that on average 62% of the outliers are followed by other outliers within a time period of 7 days in total.
Table 7. Sequential outliers
Table presents the number of outliers that are concurrent between every two stock markets. Given the different time zones and trading hours for the stock markets of our sample, we define concurrent outliers as the ones that occur at the same date or with a difference of one day. We note that considerable number of the outliers are concurrent across stock markets. Most of the stock markets have large numbers of the concurrent outliers with the USA. For example, UK, Russia, Brazil, South Africa, India and Saudi Arabia have the largest number of the concurrent outliers with USA. The largest number of the concurrent outliers is between USA and UK. Germany and France also have relatively large numbers of concurrent outliers with USA. We also note large numbers of concurrent outliers within European stock markets especially between Germany and France. Apart from UAS, we note that Japan has the largest number of the concurrent outliers with UK, and Turkey has the largest number of the concurrent outliers with Russia. Interestingly, China and Turkey have small numbers of concurrent outliers with other markets, implying that they can be good destinations for portfolio diversification. Moreover, we divide the concurrent outliers into negative concurrent outliers and positive concurrent outliers. A noteworthy pattern revealed from this division is that the number of the negative concurrent outliers is greater than the positive concurrent outliers for almost all market pairs.
Table 8. Concurrent outliers
The analysis in this section reveals two important features of outlying returns in stock markets. The first feature is that outliers tend to occur in a sequential pattern. A considerable number of outliers tend to be followed by other outliers within a very short time period of 7 days. From as low as 50% (Japan) to as high as 71% (Saudi Arabia and South Korea) of the outliers are followed by other outliers within this window of time in total. For a shorter window of time, form as low as 25% (Japan) to as high as 47% (Saudi Arabia) of the outliers are followed by other outliers within a timeframe of 3 days in total. The second feature is that outliers tend to occur simultaneously across stock markets. Our analysis shows that substantial numbers of the outliers are concurrent for most of market pairs. This pattern of outliers occurrence shed more light and provide further insights onto the transmission and spillover effects amongst global stock markets. Our analysis indicates that the transmission and spillover effect from USA to the other markets is the greatest. Most of the markets have large numbers of the concurrent outliers with USA. Also, our analysis shows that negative fluctuations are more transmissible across stock markets than positive fluctuations. This reflects the phenomenon that global stock markets tend to be more connected and correlated during the times of downturns compared to the upturn ones (see e.g., Longin & Solnik, Citation2001).
4.6. Outliers and return distribution
Here we conduct an exercise for handling and adjusting for outliers in the framework of Boxplot method for examining the effect of outliers on return series unconditional standard deviation, skewness and kurtosis. Our exercise involves replacing outlier values with fence values.Footnote2 We apply this methodology for correcting all outliers and for correcting extreme outliers only. Specifically, in the case of all outliers we replace all outliers below the inner lower fence () with the value of inner lower fence and all outliers above the inner upper fence (
) with the value of inner upper fence. In the case of extreme outliers, we replace extreme outliers below the outer lower fence (
) with the value of outer lower fence and extreme outliers above the outer upper fence (
) with the value of outer upper fence.
In Table , we report the percentage change in unconditional standard deviation, skewness and kurtosis after adjusting for outliers in return series relative to those of original series as presented in Table . We also present J–B normality test for adjusted series along with its p-value. We note that on average the standard deviation decreased by about 18% in the case of all outliers and by about 7% in the case of extreme outliers. Saudi Arabia, USA, Russia and South Korea have the largest reductions in standard deviation, while Japan and South Africa have the smallest reductions.
Table 9. Effect of outliers on some statistics
Large kurtosis and negative skewness in original return series decreased considerably after adjusting for outliers. On average, skewness decreased by about 74% in the case of all outliers.Footnote3 In the case of extreme outliers, skewness became more negative for Germany and Turkey, otherwise the skewness decreased by about 49 % on average. In the case of all outliers, Saudi Arabia and China have the largest decrease in their negative skewness by about 93% and 89%, respectively, while Germany has the smallest decrease by a percentage of 43.7%. For kurtosis, it decreased by about 69% on average in the case of all outliers and by about 51% in the case of extreme outliers. In both cases of all outliers and extreme outliers, Saudi Arabia and Brazil have the greatest decrease in kurtosis, while China has the smallest decrease by percentages of 60.7% and 36.9%. J–B normality test can be rejected at conventional significance levels for all adjusted return series except for China and Saudi Arabia and only in the case of all outliers. The rejection of normality test can be attributed to significant negative skewness found in return series even after adjusting for outliers. A possible reason for this is that some large negative daily returns are more frequent and larger in magnitude than their positive returns counterpart. This is inconsistent with Ané et al. (Citation2008),where they find J–B normality test cannot be rejected in adjusted (for outliers) return series of their sample of five Asian stock indices.
4.7. Outliers and return performance and realization
One of the most important implications of outlying returns for stock portfolio and risk management is the effect of outlying returns on the behaviour of return realization over the investment holding periods. In this section we investigate the impact of the best (positive) and worst (negative) outlying returns on the return performance and realization for every stock market over the sample period. The results of this exercise are presented in Table . Panel A presents the terminal value of passively investing 100 units of local currency over the whole sample period for every stock market. Panel B shows the effect of excluding the best (positive) 10, 20 and 50 outlying returns on the terminal value of the 100-unit investment, along with the percentage change in the terminal value in each case compared to the terminal value of the passive investment. Panel C shows the effect of excluding the worst (negative) 10, 20 and 50 outlying returns on the terminal value of the 100-unit investment, along with the percentage change in the terminal value for each case compared to the terminal value of the passive investment.
Table 10. Outliers and return realization
First of all, Panel A shows that in the cases of UK, France, Japan and Russia the terminal value of the passive investment is less than the initial investment over our sample period. In contrast, Turkey, South Africa and India have the greatest terminal values. Moving to Panel B we note that compared to the passive investment the terminal value on average decreases by about 58% and 77% when excluding the best (positive) 10 and 20 outlying returns, respectively. In the case of excluding the best 10 outlying returns we note that Russia, Brazil and Turkey have the greatest reductions (73% and 72%, respectively), while UK and South Africa have the smallest reductions (47% and 44%, respectively). In the case of excluding the best 20 outlying returns, the reduction ranging from as low as 65% (UK) to as high as 89% (Russia and Turkey). Remarkably, excluding the best 50 outlying returns, which represent only about 1% of the number of return observations, results in a decrease in the terminal value by about 94% on average compared to the passive investment. The reduction in the terminal value ranging from as low as 87% (UK) to as high as 99% (Russia and Turkey). In the case of Russia, we note that the initial amount invested is almost totally lost. When excluding the best 50 outlying returns, we note that for 8 out of the 14 stock markets (France, Japan, Russia, China, Brazil, Turkey, Hong Kong and South Korea) the terminal value decreases to less than 10% of the initial amount invested.
Moving to the worst (negative) outlying returns, in Panel C we note that compared to the passive investment the terminal values on average increases by about 193% and 569% when excluding the worst (negative) 10 and 20 outlying returns, respectively. In the case of excluding the worst 10 outlying returns we note that UK, South Africa and Germany have the smallest increases (107%, 121% and 133%, respectively), while Russia, Turkey and Brazil have the greatest increases (449%, 334% and 289%, respectively). In the case of excluding the worst 20 outlying returns, the increase ranging from as low as 244% (UK) to as high as 1,824% (Russia). Considerably, excluding the worst 50 outlying returns, which represent only about 1% of the number of return observations, results in an increase in the terminal value by about 5,576% on average compared to the passive investment. The increase in the terminal value ranging from as low as 1,052% (UK) to as high as 32,723% (Russia).
The relevant implication of this exercise for stock portfolio and risk management is that the performance and realization of stock returns are heavily dependent on the outlying fluctuations rather than on normal ones. in other words, the results of this exercise show that outlying returns have considerable effects on stock return performance. In addition, the results show that a small number of returns, about 1% of the total number of return observations, has an enormous impact on stock market returns. This, in turn, makes stock market timing decisions are not an easy task for stock portfolio management. In addition, these results show the importance of diversification decisions in stock portfolio risk management; diversifying stock portfolios with other asset classes which have offset effects to the outlying stock market movements is extremely important especially in turbulent time periods.
4.8. Outliers at lower frequency data
As different market participants and practitioners are interested in examining stock markets at different and lower frequencies, in this section we investigate outliers in weekly and monthly returns. Table presents a basic summery of the weekly outlying returns. The number of all weekly outliers ranges from as low as 30 (South Africa) to as high as 72 (Saudi Arabia), with an average of 49. The percentage of outliers to the number of weekly return observations ranges from as low as 3% (Japan, Brazil, South Africa and Hong Kong) to as high as 6% (Russia, Saudi Arabia and South Korea), with an average of 4% across the stock markets. Negative outliers are more frequent than positive outliers for all markets. On average, negative outliers represent 65%, while positive outliers represent 35%. For the extreme weekly outlying returns, the number of extreme outliers ranges from as low as 4 (Japan and Hong Kong) to as high as 18 (Saudi Arabia), with an average of 9. The percentage of the extreme outliers to the number of observations ranges from as low as 0.3% (Japan) to as high as 1.6% (Saudi Arabia), with an average of about 1%. Classifying extreme outliers into negative and positive outliers, we note again that extreme negative outliers are more frequent than positive ones for all markets, except for Hong Kong and South Korea, with a percentage of 72% on average.
Table 11. Weekly outliers
Table presents a basic summery of the monthly outlying returns. The number of all monthly outliers ranges from as low as 6 (Japan) to as high as 21 (Saudi Arabia and South Korea), with an average of 12 across the stock markets. The percentage of the outliers to the number of monthly return observations ranges from as low as 2% (Japan) to as high as 8% (Saudi Arabia and South Korea), with an average of 4%. Negative outliers are more frequent than positive outliers for all stock markets except for China and Turkey. On average, the negative outliers represent 67%, while the positive outliers represent 33%. For extreme monthly outlying returns, the number of extreme outliers ranges from as low as zero (China) to as high as 4 (Saudi Arabia), with an average of 2. We note that China does not have extreme outliers at monthly frequency. On average, the extreme monthly outliers represent about 1% of the monthly return observations across the stock markets of our sample. Interestingly, all extreme monthly outliers are negative for all markets except for Turkey.
Table 12. Monthly outliers
5. Conclusions
In this paper, we employ the Boxplot method for detecting and examining the possible outliers in daily returns of 14 international stock market indices over the period November 1997 to April 2020. The market sample includes the major indices of USA, UK, Germany, France, Japan, Russia, China, Brazil, South Africa, India, Turkey, Hong Kong, Saudi Arabia and South Korea. We analyze the detected outliers in many aspects, including frequency, sign, magnitude, effect and distribution within and across return series. We also conduct an exercise to adjust for detected outlying returns and examine their impact on return series distribution. We analyze two sets of outliers; all outliers which include mild and extreme outliers, and from a conservative point of view the second set includes only extreme outliers.
The results show that outlying returns represent about 6% of the number of return observations on average. Conservatively, 1.4% of return observations are extreme outliers which means that there is about one extreme outlying return every three months on average. Also, we find that for all indices negative outliers are more frequent than positive outliers with a percentage of 57% of the number of all outliers on average. We then examine the magnitude and severity of outliers in terms of unconditional standard deviation, . The results show that the bulk of detected outliers are in the magnitude of 3
. We find that USA, UK, France, Brazil, India and Saudi Arabia are amongst the markets that have at least one outlier of magnitude
or greater, while only China has no outliers of magnitude greater than
. Moreover, the analysis shows that the largest negative outliers are more severe than the largest positive outliers for most of stock markets in our sample. Interestingly, we do not find consistent evidence that frequency, magnitude and severity of outliers are greater in less developed and developing stock markets than in well developed markets. Inspecting the number of outliers over years reveals two important patterns. First, we find that return outliers tend to cluster within a return series over time. Second, return outliers tend to cluster together across stock market indices. Moreover, our analysis shows that outliers tend to occur in a sequential patter within the individual return series, and concurrently across stock markets. Up to 71% of outliers are followed by other outliers within a time period of 7 days. Most of the stock markets have larger numbers of concurrent outliers with USA. The number of negative concurrent outliers are found greater than the number of positive concurrent outliers. Adjusting for all outliers in return series results in a decrease in standard deviation by about 18%, 74% in negative skewness and 69% in kurtosis on average relative to original series. However, we find that adjusted return series failed to pass J–B normality test for almost all markets. Our analysis also show that outlying returns have an enormous impact on stock return performance and realization. Overall, the paper provides an in-depth analysis of the outlying daily returns of an extensive sample of international stock markets over an extended sample period. In addition, the paper introduces the Boxplot method as a handy, practical and effective tool for detecting possible outliers in stock market returns.
Acknowledgment
We would like to thank the editor for the helpful guidance throughout the process. Also, we would like to thank the anonymous referees for their constructive and useful comments and suggestions which improve the quality and contribution of the paper.
Correction
This article has been corrected with minor changes. These changes do not impact the academic content of the article.
Disclosure statement
No potential conflict of interest was reported by the author(s).
Additional information
Funding
Notes on contributors
Ali A. Shehadeh
Ali A. Shehadeh is an assistant professor at the Department of Finance, Faculty of Business, the University of Jordan/Aqaba. His research interests include international finance, financial markets, stock markets, corporate finance, financial analysis and financial economics. Among others, he has publications in International Review of Financial Analysis and Czech Journal of Economics and Finance.
Sadam M. Alwadi
Sadam M. Al-Wadi is an associate professor at the Department of Finance, Faculty of Business, the University of Jordan/Aqaba. His research focuses on modelling and forecasting financial time series and forecasting stock market decomposition.
Mohammad I. Almaharmeh
Mohammad I. Almaharmeh is an associate professor at the Department of Accounting, Faculty of Business, the University of Jordan/Aqaba. His research interests include earnings management, stock price informativeness, stock price synchronicity, IFRS, analyst coverage, and market-based research.
Notes
1. A concern about the classical Boxplot method in detecting outliers is the asymmetry of data distribution. However, Walker et al. (Citation2018) find that the classical Boxplot method perform well compared to other modified alternatives when sample sizes are large even with the existence of asymmetry in data distribution.
2. Although outliers can be replaced with zero, mean or median as usually suggested in the literature, we believe that our exercise is more realistic.
3. We exclude Brazil and Hong Kong as skewness in their original return series was positive but turned to negative in adjusted series.
References
- Afanasyev, D. O., & Fedorova, E. A. (2019). On the impact of outlier filtering on the electricity price forecasting accuracy. Applied Energy, 236, February, 196–27. https://doi.org/10.1016/j.apenergy.2018.11.076
- Ané, T., Ureche-Rangau, L., Gambet, J. B., & Bouverot, J. (2008). Robust outlier detection for Asia–Pacific stock index returns. Journal of International Financial Markets, Institutions and Money, 18(4), 326–343. https://doi.org/10.1016/j.intfin.2007.03.001
- Aronne, A., Grossi, L., & Bressan, A. A. (2020). Identifying outliers in asset pricing data with a new weighted forward search estimator. In Revista Contabilidade & Finanças, 31(84), 458–472. https://doi.org/10.1590/1808-057x201909620
- Balke, N. S., & Fomby, T. B. (1994). Large shocks, small shocks, and economic fluctuations: Outliers in macroeconomic time series. Journal of Applied Econometrics, 9(2), 181–200. https://doi.org/10.1002/jae.3950090205
- Bauer, R., Haerden, R., & Molenaar, R. (2004). Asset allocation in stable and unstable times. The Journal of Investing, 13(3), 72–80. https://doi.org/10.3905/joi.2004.434554
- Behmiri, N. B., & Manera, M. (2015). The role of outliers and oil price shocks on volatility of metal prices. Resources Policy, 46 December, 139–150. https://doi.org/10.1016/j.resourpol.2015.09.004
- Berkowitz, J., & O’Brien, J. (2002). How accurate are value‐at‐risk models at commercial banks? The Journal of Finance, 57(3), 1093–1111. https://doi.org/10.1111/1540-6261.00455
- Charles, A. (2008). Forecasting volatility with outliers in GARCH models. Journal of Forecasting, 27(7), 551–565. https://doi.org/10.1002/for.1065
- Charles, A., & Darné, O. (2006). Large shocks and the September 11th terrorist attacks on international stock markets. Economic Modelling, 23(4), 683–698. https://doi.org/10.1016/j.econmod.2006.03.008
- Estrada, J. (2008). Black swans and market timing: How not to generate alpha. The Journal of Investing, 17(3), 20–34. https://doi.org/10.3905/joi.2008.710917
- Estrada, J. (2009). Black swans, market timing and the Dow. Applied Economics Letters, 16(11), 1117–1121. https://doi.org/10.1080/17446540802360074
- Ferdousi, Z., & Maeda, A., 2006, April. Unsupervised outlier detection in time series data. In 22nd International Conference on Data Engineering Workshops (ICDEW’06), USA. x121–x121. IEEE.
- Franses, P. H., Dijk, D. V., & Lucas, A. (2004). Short patches of outliers, ARCH and volatility modelling. Applied Financial Economics, 14(4), 221–231. https://doi.org/10.1080/0960310042000201174
- Franses, P. H., & Ghijsels, H. (1999). Additive outliers, GARCH and forecasting volatility. International Journal of Forecasting, 15(1), 1–9. https://doi.org/10.1016/S0169-2070(98)00053-3
- González-Sánchez, M. (2021). Is there a relationship between the time scaling property of asset returns and the outliers? Evidence from international financial markets. Finance Research Letters, 38 January, 101510. https://doi.org/10.1016/j.frl.2020.101510
- Grané, A., & Veiga, H. (2010). Wavelet-based detection of outliers in financial time series. Computational Statistics & Data Analysis, 54(11), 2580–2593. https://doi.org/10.1016/j.csda.2009.12.010
- Haggard, K. S., Jones, J. S., & Witte, H. D. (2015). Black cats or black swans? Outliers, seasonality in return distribution properties, and the Halloween effect . In Managerial Finance, 41(7), 642–657. https://doi.org/10.1108/MF-07-2014-0190
- Ju, K., Su, B., Zhou, D., Wu, J., & Liu, L. (2016). Macroeconomic performance of oil price shocks: Outlier evidence from nineteen major oil-related countries/regions. Energy Economics, 60 November, 325–332. https://doi.org/10.1016/j.eneco.2016.10.009
- Kim, Y., Kim, T. H., & Ergün, T. (2015). The instability of the Pearson correlation coefficient in the presence of coincidental outliers. Finance Research Letters, 13 May, 243–257. https://doi.org/10.1016/j.frl.2014.12.005
- Li, A., Feng, M., Li, Y., & Liu, Z. (2016). Application of outlier mining in insider identification based on boxplot method. Procedia Computer Science, 91, 245–251. https://doi.org/10.1016/j.procs.2016.07.069
- Longin, F., & Solnik, B. (2001). Extreme correlation of international equity markets. The Journal of Finance, 56(2), 649–676. https://doi.org/10.1111/0022-1082.00340
- Loperfido, N. (2020). Kurtosis-based projection pursuit for outlier detection in financial time series. The European Journal of Finance, 26(2–3), 142–164. https://doi.org/10.1080/1351847X.2019.1647864
- Raggad, B., & Boutahar, M. (2012). Structural change in tail behaviour and the recent financial crises. International Journal of Monetary Economics and Finance, 5(3), 277–298. https://doi.org/10.1504/IJMEF.2012.049060
- Schluter, C., & Trede, M. (2008). Identifying multiple outliers in heavy-tailed distributions with an application to market crashes. Journal of Empirical Finance, 15(4), 700–713. https://doi.org/10.1016/j.jempfin.2007.10.003
- Schwertman, N. C., Owens, M. A., & Adnan, R. (2004). A simple more general boxplot method for identifying outliers. Computational Statistics & Data Analysis, 47(1), 165–174. https://doi.org/10.1016/j.csda.2003.10.012
- Shen, C. H., Fan, X., Huang, D., Zhu, H., & Wu, M. W. (2018). Financial development and economic growth: Do outliers matter? Emerging Markets Finance and Trade, 54(13), 2925–2947. https://doi.org/10.1080/1540496X.2018.1440547
- Shevlyakov, G., Andrea, K., Choudur, L., Smirnov, P., Ulanov, A., & Vassilieva, N., 2013, May. Robust versions of the Tukey boxplot with their application to detection of outliers. In 2013 IEEE International Conference on Acoustics, Speech and Signal Processing, Canada. 6506–6510. IEEE.
- Stanley, H. E. (2003). Statistical physics and economic fluctuations: Do outliers exist? Physica A: Statistical Mechanics and Its Applications, 318(1–2), 279–292. https://doi.org/10.1016/S0378-4371(02)01825-3
- Sun, J. H., Feng, Y. J., & Hu, J. (2008). Outlier pattern mining of stock time series with fractal theory. Operations Research and Management Science, 27(5), 135–140.
- Thomas, S., Ramiah, V., Mitchell, H., & Heaney, R. (2011). Seasonal factors and outlier effects in rate of return on electricity spot prices in Australia’s National Electricity Market. Applied Economics, 43(3), 355–369. https://doi.org/10.1080/00036840802570421
- Tolvi, J. (2001). Outliers in eleven finnish macroeconomic time series. Finnish Economic Papers, 14(1), 14–32.
- Tukey, J. W. (1977). Exploratory Data Analysis, 2, 131–160.
- Villavicencio, A. L. (2008). Nonlinearities or outliers in real exchange rates? Economic Modelling, 25(4), 714–730. https://doi.org/10.1016/j.econmod.2007.11.001
- Walker, M. L., Dovoedo, Y. H., Chakraborti, S., & Hilton, C. W. (2018). An improved boxplot for univariate data. The American Statistician, 72(4), 348–353. https://doi.org/10.1080/00031305.2018.1448891
- Welsch, R. E., & Zhou, X. (2007). Application of robust statistics to asset allocation models. REVSTAT–Statistical Journal, 5(1), 97–114.
- Zhao, Q. J., Gan, J., & Che, W. G., 2012, July. Remarks on outliers in time series of stock prices based on density. In Proceedings of the 10th World Congress on Intelligent Control and Automation, China. 4523–4526. IEEE.