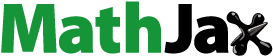
Abstract
The paper seeks to analyze residential energy use patterns and the price and energy expenditure responsiveness of household demand for residential energy in Ghana, using GLSS 7 data, the multivariate probit model, and the Quadratic Almost Ideal Demand System (QUAIDS) model. The study focuses on four main fuels; firewood, charcoal, and others (FCO), LPG, electricity, and kerosene. The results show that the demand for all household energy fuels in Ghana was significantly influenced by price, income, and social demographic factors. Household demand for electricity and LPG fuels are income elastic with values greater than 1, while FCO and kerosene fuels are income inelastic. The own-price elasticity also shows modern fuel (LPG and electricity) as the most responsive fuel to a price increase, even though all energy fuels are price inelastic and negative. The complementary cross-price elasticity of all fuels estimated must be carefully interpreted in the context of the cultural factors, socioeconomic factors, and the unique purposes of each fuel. After a robustness check was done in correcting for bias arising from zero energy expenditure, the study finds that the own-price elasticity for modern energy fuel (LGP and ECG) reduced slightly, even though the demand for all energy fuel remains negative and price inelastic. The study includes implications for policies geared towards reducing the price of residential energy fuels and policies to ensure clean and efficient residential energy fuels are readily accessible for consumption.
PUBLIC INTEREST STATEMENT
Most national discourse on residential energy consumption in Ghana focuses on the devastating impact of traditional energy fuel consumption such as wood fuels on forest depletion, health, and economic growth, calling for the adoption of renewable or modern energy. Largely, Ghana is energy poor posing critical challenges to the economy. Transitioning to modern energy consumption could take a long time in Ghana. However, effectively addressing issues of energy poverty in Ghana can be viewed in two folds; the affordability and availability of energy fuel to meet the energy needs of the households. Similarly, a household’s ability to purchase or adopt efficient energy fuel depends on the elasticity of demand for that fuel. It is given this, that the study seeks to discuss the sensitivity of household demand for residential fuel types to changes in prices and household income to guide government design policies to promote the use of affordable clean modern fuels in Ghana.
1. Introduction
The choice of residential energy type does not only satisfy the fundamental needs of a household in providing warmth, light, and cooking sources but also has economic and health implications. In the literature, the consumption of renewable energy and its positive impact on economic growth and production has been largely discussed (see, Ivanovski et al., Citation2021; Nathaniel et al. Citation2020; Tiwari et al., Citation2021). For developing countries, the transition to renewable energy may take some time. The prevalence of energy poverty and its impact on health and growth in developing countries can be viewed in two folds; the affordability and availability of energy fuel to meet the energy needs of households or the economy (see, Adjei-Mantey & Takeuchi, Citation2021; Awaworyi Churchill & Smyth, Citation2021; Llorca et al., Citation2020; Oliveras et al., Citation2020).
In Ghana, energy poverty poses a serious challenge. Despite the longstanding efforts to address energy poverty, the share of energy-poor households in Ghana is estimated at 82.5 percent. (Adusah-Poku & Takeuchi, Citation2019). Wood fuels remain the predominant residential energy fuel, accounting for over 39% of the total primary energy supply and about 39% of the final energy demand (Ministry of Energy, Ghana). These wood fuels are obtained from natural forests and are the principal cause of the depletion of Ghanaian forests and the release of toxic health emissions into the air (United Nations Environmental Program [UNEP]—Risoe Center,2013). According to the World Bank, in 2016, close to 21 percent of households in Ghana do not have access to electricity with the majority located in the rural areas. The most important fuels used by households for cooking are charcoal, 34.1 percent followed by wood, 33.3 percent, and Gas, 24.5 percent (GLSS 7 report). Other sources of fuel used for cooking are less than 1 percent of the total population.
The high rate of energy-poor households in Ghana (82.5%) raises concerns. As the call for the consumption of renewable energy rises, the ability of a household to purchase or adopt efficient energy fuel depends on the elasticity of demand for that fuel. This leads to the fundamental research question; how does a unit change in the cost of energy fuel type and household income influence household demand for residential fuel type in Ghana? Similarly, do social-economic factors such as the location of the household and the sex of the household head influence residential energy expenditure patterns in Ghana? The objective of the study is to understand the sensitivity of household demand for fuel types to changes in prices and household energy budget (income) to guide government design policies to promote the use of affordable efficient modern fuels in Ghana.
The author is not aware of any studies on the exact demand elasticities of demand for energy fuel in Ghana. Most studies on household energy consumption patterns in Ghana merely focused on determinants of household energy access and energy poverty (see Abdul-Wakeel Karakara & Dasmani, Citation2019; Faisal et al., Citation2013; Adusah-Poku & Takeuchi, Citation2019; Adusah‐Poku et al., Citation2021; Awan et al Citation2014; Kwakwa et al., Citation2013). These studies at best estimated probabilistic elasticity which does not reflect the exact demand elasticities. This study, however, seeks to fill the lacuna. The combined use of the Quadratic Almost Ideal Demand System (QUAIDS) and the Multivariate Probit model was adopted to ensure a robustness check in estimating the exact price and expenditure elasticities of demand for residential energy in Ghana. The expenditure of households in Ghana is non-linear and the QUIAIDs model has the unparalleled capability of incorporating non-linear effects and interactions of price and expenditures on the demand specification over other competing flexible demand systems.
The main conclusions of the study are that; modern energy is income elastic but income inelastic to demand traditional energy. Also, the elasticity of demand for all residential energy fuels is both own price and cross-price inelastic, even though households have a higher price elasticity of demand for modern energy than traditional energy fuels in Ghana. However, when the study controls for bias arising from zero expenditure from the data using the predicted budget shares from the MVP analysis (that is, assuming all households use a positive amount of each energy type and had access to all fuels), there is evidence that all fuels have negative and significant inelastic own and cross-price elasticities. Also, there was a reduction in the own-price elasticity of demand for modern fuels (i.e. electricity and LPG) and an increase in the price elasticity for traditional fuels. This is significant for policy guidance on cost and access to energy types in Ghana. The study finds that urban households and male-headed households have an increased likelihood of consuming modern energy, whereas older and larger-sized households are less likely to consume modern energy fuels.
The remaining section of the paper is organized as follows: The next section examines the theoretical and empirical literature on household energy consumption. Section 3 then discusses the methodology used for the estimationand an overview of the study data. The penultimate section summarizes the empirical results before the paper concludes in the last section, with some policy implications about to residential energy demand in Ghana.
2. Literature review
2.1. Theoretical framework
There is a plethora of models developed to provide comprehensive explanations on the pattern of household energy consumption. A product of early models such as the behavioral model is the energy ladder hypothesis argued by Heltberg (Citation2003). The hypothesis suggests that household energy expenditure is in three progressive stages. The first stage is mostly characterized by the dependence on cheap and locally available non-modern energy but is not efficient at the macro level. The second stage is the transit from biomass to fuels such as coal, kerosene, and charcoal. The final stage is where the household transits to use modern energy for cooking and lighting, cooling, heating, etc. The energy ladder model, however, has been disparaged as being deficient in espousing the actual energy consumption patterns because transitioning process is difficult as it depends largely on social and cultural issues. Vasseur et al. (Citation2019) argued that the energy consumption pattern of households is influenced by a complex interaction between contextual, economic, and social influences. This interaction has been structured into internal context—personal factors, internal context—attitudinal factors, and external factors suggesting a multilevel factor that influences household energy consumption patterns. The energy mix framework suggests that a household will only share its disposable income among a good or combination of goods that yield the maximum utility. That means households are inclined to first decide how much of their income will be spent on energy and in addition then decide the amount and quantity to be spent on the different fuel types. . Considering household energy expenditure, households allocate their income among different forms of energy: woodfuels, kerosene, Liquefied petroleum gas (LPG), and electricity. For a developing country like Ghana where there are income constraints, the energy mix model forms the conceptual framework for analyzing the demand for residential energy in Ghana.
2.2. Empirical literature review
There is no significant debate in the literature on the significance of factors either considered alone or some combinations of determinants within the same category that can be related to household energy use. These studies vary from an economic perspective (monetary factors such as household income and price of energy fuel) to perspectives related to household personal characteristics (i.e. household size, sex of household head, rural/urban location of household, etc.).
The role of income on energy demand can be viewed in two folds. Some argue that wealthier households tend to purchase luxury appliances that may not be energy efficient while poorer households consume less efficient energy appliances because of a lack of money (see, Lenzen et al., Citation2006). Other studies, however, conclude that households with a higher income can afford to consume more modern and efficient energy, while poor households cannot demand modern energy, and are also consequently forced to conserve (see, Faisal et al., Citation2013; Mensah et al., Citation2016; Tchereni, Citation2013).
Traditionally, it is assumed that households that have more members are more likely to demand more energy fuel than households with fewer members (see, Leahy & Lyons, Citation2010). However, it is also possible that the per capita amount and cost of energy usage decrease as the household size rises which could trigger the demand for modern energy (see. Twerefou & Abeney, Citation2020). In the case of Ghana, Mensah and Adu (Citation2013) found an inverse relationship between the size of the household and its corresponding demand for clean modern energy (LPG and Electricity), whereas a positive relation was established for household demand for traditional energy fuels as household size increases.
In the literature, the general understanding is that rural households are more inclined to demand more wood fuels than urban households who on the other hand, are more likely to demand modern fuel. This is because urban households are more likely to have increased incomes and access to purchase clean modern fuel equipment than rural households (Olabisi et al., Citation2019). Awan et al. (Citation2014) perfectly summarize that rural households are energy poorer than their urban counterparts. This finding is supported by the study done by Twerefou and Abeney (Citation2020) for households in Ghana
Studies have also shown that building characteristic influences household choice and the total amount of energy fuel consumed (see, Jones et al., Citation2016). For instance, the nature of the building and the corresponding fitted equipment can influence the choice of fuel use. Modern houses with modern equipment are more common in urban areas and are likely to rely more on the consumption of modern fuels. . In Finland, Heinonen and Junnila (Citation2014) found that on a household level, while detached housing types are more energy-intensive, followed by row/terrace building types and apartments, detached houses are more energy efficient. While it is important to recognize the influence of building type on energy use, it is also reasonable to suggest that household behavior especially, the energy-saving efforts of the members can make a difference in household energy expenditure. As such, the role of building type on household energy use may exhibit an insignificant correlation (Thøgersen & Grønhøj, Citation2010; Wright, Citation2008).
Demographic trends show that male-headed households demand more modern energy fuels than female-headed householdsthat use more non-modern energy or a mixture of both modern and traditional energy (Mekonnen & Köhlin, Citation2008; Ogwumike et al., Citation2014). In the case of Ghana, Karakara and Dasmani (Citation2019) found that female-headed households have a reduced probability of using electricity by 0.6%, LPG/natural gas by 3.9%, kerosene by 0.07% to cook, and an increased probability of using charcoal by 4.4%, and wood by 0.06% to cook.
Estimations of the exact demand elasticities for the choice and demand for residential energy fuels in Ghana are rather limited. Notwithstanding, studies from other countries suggest an inverse relationship between the price of residential energy fuel and its corresponding quantity demand (Olabisi et al., Citation2019). For instance, Jordan, Al-Bajjali, and Shamayleh (Citation2018) found that residential demand for electricity in Singapore was price inelastic. Démurger and Fournier (Citation2007) postulated that firewood and coal are substitutes. In the case of Ghana, Twerefou and Abeney (Citation2020) estimated the probabilistic elasticity of demand for electricity in Ghana. They found that the probabilistic price and income elasticity of demand for electricity in Ghana were −0.738 and 0.112, respectively. Similarly, urban households have a higher probabilistic income elasticity of electricity demand (0.1292) than their rural counterparts.
3. Data and methodology
3.1. Data
This paper relies on data from the Ghana Living Standard Survey (GLSS) round 7, which covered twelve (12) months from 22 October 2016 to 17 October 2017. Out of the 15,000 households selected, 14,009 representing 93.3 percent of households responded. The GLSS 7 collects expenditure information on all four residential energy fuels. The data also capture household demographics such as rural/urban location of household, sex of household head, household size, and the age of household head.
For this study, the analysis focuses on a dataset comprising 10,931 observations as valid for the estimation. The study controlls for selected socioeconomic factors that influence household choice and demand for fuel. Table provides summary statistics of the data used. From the table, urban households constitute the majority of respondents (51.82 percent) compared to 48.18 percent of rural households. The respondents are mainly non-poor (81.27 percent) because the majority of the respondents are engaged in some level of employment (80.24 percent). Notwithstanding, rural households on average have lower incomes and larger household sizes. Urban households tend to have younger household heads and they make the highest energy expenditures. Even though 67 percent of the households have male heads, female-headed households are more common in urban than in rural areas, and they represent almost one-third of households in rural areas.
Table 1. Summary characteristics of householdsFootnote1
3.2. Methodology
3.2.1. Quadratic almost ideal system
The study adopts the Quadratic Almost Ideal Demand System (QUAIDS) developed by Banks et al. (Citation1997). The model estimates the budget share of various commodities and shows they are linearly related to the logarithm of real total expenditure and the logarithms of relative prices. It is an extension of the Almost Ideal Demand System proposed by Deaton and Muellbauer (Citation1980).
The choice of the Quadratic Almost Ideal Demand System (QUAIDS) for the study is based on the attributes the model has over other major models such as the Almost Ideal Demand System (AIDS) model (Akbay et al., Citation2007), Rotterdam model (Barnett & Seck, Citation2008), and Translog models (Holt & Goodwin, Citation2009). One advantage of the QUAIDS model is that the homogeneity and symmetry restrictions in the form of a linear approach are easily imposed and tested. QUAIDS model also has a functional form that is consistent with household budget data in the sense that household expenditure in Ghana is non-linear and only the QUAIDS model (of the previously mentioned models) provides for non-linearity. The model satisfies the axioms of choice exactly. The model is also able to estimate elasticities that are consistent with consumer theory. Moreover, the model can aggregate perfectly over consumers without invoking parallel linear Engel curves (Aepli, Citation2014). By introducing the quadratic income term, the QUAIDS model gains more flexibility, which positively influences the quality of the estimated model outcomes. Demographic variables can be specified in QUAIDS and are incorporated using Ray’s (Citation1983) method. Finally, the model can easily be used to estimate own price elasticity and cross-price elasticity between two or more goods as well as the compensated and uncompensated elasticities (Aepli, Citation2014). The AIDS, Rotterdam, and Translog models may also have some of these properties at their respective model levels but then neither of them satisfies all the properties compared to QUAIDS.
To begin with, we consider a consumer’s demand for categories of residential energy; FCO (firewood, charcoal, and others), LPG (gas), electricity, and kerosene. For which the consumer spends m amount on residential energy. We derive the indirect utility function of the QUAIDS model as;
Where In a(p) is the transcendental logarithm function
In this function, pi is the price of good i for i = 1, …,4
And b(p) is the Cobb–Douglas price aggregator
And
For the model to adhere to consumer demand theory, adding up, homogeneity, and Slutsky symmetry conditions requires that
The consumer demand theory conditions in Equationeqn (3)(3)
(3) are imposed during estimation and ensure that Equationeqn (2)
(2)
(2) defines a(p) as a linearly homogeneous function of the individual prices.
Let qi denote the quantity of good i consumed by a household and define the expenditure share for good i as wi = piqi/m. Applying Roy’s identity to Equationeqn(1(1)
(1) ), we obtain the expenditure share equation for good i as;
i = 1,2,3 and 4
Setting λi = 0, reduces the QUAIDS model to the original AIDS model.
For purposes of the research reported in this paper, a set of demographic variables is added for each household using Ray’s (Citation1983) method based on an expenditure (cost) function of the form;
Where z represents a vector of s household characteristics (in this case; location of household, sex of household head, and age of household head), eR(p,u) is the expenditure function of a reference household, and m0 (p,z,u) scales the expenditure function to account for household characteristics and can be decomposed as;
where mo measures the increase in a household’s expenditures as a function of z and ø controls for changes in relative prices and the actual goods consumed. Further, mo (z) is parameterized as
where ƿ is a vector of parameters to be estimated. ø(p,z,u) is parameterised as
where ƞj represents the jth column of s x k parameter matrix ƞ. The QUAIDS expenditure share for the purpose of this estimation takes the form
Where
Where all the demand restrictions of Equationeqn (3)(3)
(3) hold, total residential energy expending sums to 1
To obtain the parameters in QUAIDS, the expenditure share equations are estimated together and the Iterated Feasible Generalized Nonlinear Least-Squares was used based on the Seemingly Unrelated Regression (SUR) method.Footnote2 Following the adding up restriction imposed on the QUAIDS model, and to avoid a singular covariance matrix, the expenditure shares of one of the equations is dropped and the nonlinear maximum likelihood method is applied to the remaining expenditure share equations. The maximum likelihood estimates are invariant to the choice of equation deleted. According to Poi (Citation2012), the iterated feasible generalized nonlinear least-squares estimator is equivalent to the multivariate normal maximum-likelihood estimator for this class of problems. The parameter α0 is defined as the expenditure outlay required for a minimal standard of living when prices are unity. Following Banks et al. (Citation1997), the value of α0 is set at a value lower than the lowest log expenditure value.
As shown by Banks et al. (Citation1997), uncompensated price and energy fuel expenditure elasticities are presented as follows;
Uncompensated Price Elasticity of good i owing to a unit change in the price of good j incorporating household demographic is given as;
The income elasticity of demand for good i is given as;
3.2.1.1. QUAIDS variables and assumptions
Based on EquationEquation (9)(9)
(9) , the study estimates four (4) residential energy fuels consumed by households in Ghana with corresponding budget shares given as; FCO (w1), LPG (w2), electricity (w3), and kerosene (w4). This is calculated by dividing a corresponding energy fuel by total household energy expenditure. The corresponding prices are p1 to p4. The QUAIDS assumes fixed prices for each individual consumer. Therefore, the study assumes energy fuel prices to be fixed and are calculated by dividing household energy expenditure for a type of energy fuel by total household size. The demographic variables (z) captured in EquationEq. (9)
(9)
(9) are the sex of the household head (1 if Male, 2 if Female), location (1 if urban, 0 if rural), and age of the household head. There may be other demographic factors that influence household choice and pattern of energy use, but we are warned not to throw in too many demographics when estimating a detailed demand system like QUAIDS. This is mainly because the number of coefficients to be estimated increases significantly with each additional variable.
3.2.2. Multivariate probit model (MVP)
The study adopted the MVP for two reasons. Firstly, the MVP model is estimated by maximum likelihood and produces probabilistic estimates. The results predict how different demographic factors affect the household probability of adopting a particular fuel type, as other scholars have done (e.g Olabisi et al., Citation2019). To achieve this, the first partial derivative of each explanatory variable was estimated at means to produce the marginal effect of each independent variable on the four major fuel types; FCO, LPG, electricity, and kerosene. Even though the interest of this study is not in the probabilistic estimates, it will provide the socioeconomic direction of the relationship between the explanatory and dependent variables.
Secondly, since the data used are at the household level, it is expected that some households may make zero expenditure on some fuel types. This may create biased results (Denton and Mountain, Citation2016). Given this, Shonkwiler and Yen’s (Citation1999) two-step estimation technique was adopted as done in other studies (see, Olabisi et al., Citation2019). Firstly, the probit model is estimated to drive the predicted cumulative distribution and probability distribution functions for each household demand for each energy fuel. The predicted distribution is then used to modify Equationequation (9)(9)
(9) serving as budget shares instead as;
where cdfi and pdfi are the cumulative and probability distribution functions, respectively, and wi* is the new budget share.
4. Results and discussions
4.1. Descriptive statistics
4.1.1. Socioeconomic factors that influence residential energy fuel choice
Households use energy for either cooking or lighting. For this study, the use of fuel for cooking comprises all fuel used to produce any form of domestic meal. Similarly, the use of fuel for lighting purposes includes lighting, cooling, and powering any domestic gadget other than for cooking. From Table , firewood remains the most consumed energy fuel for cooking, with 37 percent of households using it, followed by charcoal (34.41 percent). Specifically, poor, very poor, and rural households tend to have more access to wood for cooking. This is likely because wood is cheap and is easily produced by them. The use of charcoal for cooking, however, is predominantly common among urban households possibly because of affluence and availability of equipment that uses charcoal among urban households than rural households.
Table 2. Access to energy for cooking (%)
From Table , electricity remains the principal source of energy consumed by households for lighting (88 percent). This is a sharp contrast from household energy consumption in 2013 where Kerosene (49.3 percent) and electricity (49.2 percent) were the most consumed energy for households for lighting purposes (GLSS data, 2013). This probably is evidence of the success of the National Electrification Project (NEP) in connecting homes to the national grid(Ministry of Energy, 2010). Also, the government’s commitment to resolving the severe electricity supply challenges popularly called “dumsor” experienced from 2012 to 2014, has led to increased access to electricity to homes in Ghana for lighting. Specifically, close to 94 percent of urban households rely on electricity for lighting while almost 70 percent of poor and very poor households rely on electricity for lighting. The data shows that female-headed households rely less on modern energy fuels for lighting than male-headed household heads except for gas. It is also clear that households that do not make any expenditure on electricity, depend on other means of fuel for mere lighting and are not a substitute to power other electronic home equipment.
Table 3. Household access to fuel for lighting (%)
4.1.2. Average cost of household energy fuel
Table provides the average cost of each residential energy fuel in Ghana using the data as it is. The average cost is calculated by dividing total fuel expenditure by the number of household members. The results show that the average cost of household total energy in Ghana is GH 211.82. Out of this, modern energy cost represents 76 percent of the total fuel cost. Residential energy cost is higher in urban areas than in rural areas possibly because they have fewer household members and also because they have a corresponding increasing demand for it. On the contrary, the cost of traditional energy is cheaper in rural areas because these materials are abundant in cheaper quantities and they are produced by themselves.
Table 4. Summary statistics of the average price of energy by location of household
4.1.3. Expenditure shares of household energy fuel
From Table , households spend more than 50 percent of their household energy expenditure on modern energy. Specifically, urban households make the highest modern energy expenditure, representing more than 60 percent of their total household energy expenditure. It is also common to see male-headed households make more modern energy expenditure than female-headed households. Probably because urban households have access to modern energy and also because of affluence. Rural, poor and very poor households make more expenditure share on traditional fuel and kerosene than urban households.
Table 5. Summary statistics of household energy expenditure shares by selected demographics
4.2. Multivariate probit results
The multivariate probit result explains the probabilistic relationship between the explanatory and explained variables. In this case, the explained variable is a dummy for residential expenditure for the four major fuels; FCO, LPG, electricity, and kerosene. The explanatory variables include a dummy variable for the sex of household head, age of household head, household size, and a dummy for the geographical location of the household. The results from Table are not far from expectations. Larger households are less likely to use modern fuels, whereas urban households are less likely to use kerosene. Older household heads have an increased likelihood of using modern fuels, while male-headed households are more likely to use modern energy and have a decreased likelihood of using FCO and kerosene.
Table 6. Multivariate probit estimate
The multivariate probit estimate only tells us the direction of the relationship between the independent and dependent variables but not the exact probability estimates. To achieve this, the study estimates the marginal effect of each explanatory variable holding other explanatory variables at means (Table ). The estimated coefficients of other demographic variables are consistent with previous studies using similar estimation methods in the context of energy use (e.g., Ouedraogo, Citation2006; Twerefou & Abeney, Citation2020).
Table 7. Marginal effects of coefficients in multivariate probit model
Considering the influence of the geographical location of the household on the choice of fuel, urban households have a reduction in the probability of purchasing kerosene by 1.3 percent and an increased probability of purchasing FCO (particularly charcoal) by 9 percent, LPG gas by 21.5 percent, and electricity by 9.1 percent. This may be influenced by the availability of equipment that uses charcoal, LPG, and ECG, or the affluence of urban areas. Raising the age of household head by one decreases the probability of using both LPG and electricity by 0.2 percent and increases the probability of using FCO by 0.2 percent. Household size is estimated to increase the likelihood of using all energy fuels except kerosene. Meanwhile, male household heads have decreased the likelihood of using FCO and kerosene by 14.3 percent and 1.8 percent, respectively.
Notwithstanding, this paper is more interested in estimating the exact demand elasticities for these four major energy fuels in Ghana, unlike other studies that only focused on determinants of residential energy. This satisfies the difference between this study and others.
4.3. QUAIDS results
To achieve our study objectives on the sensitivity of household demand for fuel type based on changes in the price of fuel and household fuel expenditure, the expenditure and uncompensated price elasticities are estimated using the QUAIDS model. The results of the parameters estimated are summarized in Table in Appendix A. All the intercepts (alpha coefficients) are significant. Also, the significance of the linear and quadratic expenditures means the consumption pattern of residential energy is sensitive to household energy expenditures. At any given income and price, all the demographic variables (location of household, sex of household head, and age of household head) have a significant influence on total household energy demand. The study, notwithstanding, is more interested in the price and expenditure elasticity estimates instead of parameters per se. To achieve this, the expenditure and price elasticities were estimated for each household and then summarized in tabular form. The results assume zero expenditures on a particular type of energy as part of the normal behavior of households. In explaining the expenditure and price elasticities (or coefficients), one must consider the pattern of energy use of these fuels.
4.3.1. Expenditure elasticity of household energy demand
From Table the expenditure and Marshallian (uncompensated) price elasticity results for the QUAIDS estimation are all significant at 1 percent. From the table, demand for FCO and kerosene are slightly income inelastic, while LPG(gas) and electricity are income elastic. The estimated income elasticities for FCO and kerosene show that even though FCO and kerosene usage have negative health and economic consequences, they are relatively cheaper and serve as basic necessary energy fuels households consume, hence their share of household energy expenditure is very low. The results are consistent with the literature as other studies that made the same observations (see, Faisal et al., Citation2013; Olabisi et al., Citation2019; Ouedraogo, Citation2006). On the other hand, clean modern energy (LPG and electricity) are considered efficient and healthy energy sources but are also expensive and mostly considered luxury goods. Thus, their demand is highly responsive to household income (budget). This is also likely a clearer indication of households in Ghana gradually appreciating the good benefits of modern energy and willing to shift away from demand for traditional fuels to modern energy consumption. The increasing use of equipment that consumes clean modern energy fuels also makes modern energy fuels more income elastic than traditional fuels.
Table 8. Elasticity estimate for residential energy demand (All households)
4.3.2. Price elasticities of demand for residential energy fuels
From Table , the uncompensated own-price elasticities for all energy fuels are inelastic and significant at 1 percent. This explains the necessity of these fuel types for household needs.
Notwithstanding, households in Ghana are slightly more price-sensitive to demand modern fuels than traditional dirty fuels. This is much expected, likely because traditional fuels are relatively cheaper and are often consumed largely for cooking purposes as basic necessity fuels, as such, households are less price-sensitive to its demand. Another possible reason is that some households are likely to produce FCO fules by themeselvesF instead of purchasing them, while the partial use of cheap kerosene by households who mostly use it for lighting explains the observed price inelastic demand for traditional/dirty fuels. On the other hand, modern fuels, despite their distinct demand, necessity cum health, and economic benefits, are relatively expensive comparatively and are susceptible to other alternatives; which are the cheap traditional fuels amidst limited budget. For instance, not all households are connected to the national grid for electricity or have stable power, as such these households (especially rural households) are likely to use alternative electricity. Imported LPG fuel is also relatively expensive with quite a population not having equipment that uses LPG at home, despite the LPG promotion initiated by the government through the Ministry of Energy. As such, any further increase in their prices will decrease their energy consumption pattern by a slightly higher percentage comparatively, as observed. The results are consistent with other studies that found the demand for residential energy to be negative and inelastic due to cultural preferences (types of foods cooked) or socioeconomic factors (see, Olabisi et al., Citation2019; Wiedinmyer et al., Citation2017).
Considering cross-elasticity estimates, the study finds that all energy fuels are slightly cross-price inelastic. However, it is imperative to interpret these cross-price elasticity coefficients in the context of the cultural factors (e.g., the type of food prepared), socioeconomic factors, and the unique purposes of each fuel in household energy consumption patterns. Most households in Ghana use different energy fuels with related purposes simultaneously for different use on different occasions based on economic and cultural reasons (Christine Wiedinmyer et al., Citation2017). For instance, it is implausible to find households in Ghana to use different fuels simultaneously by considering the time length in using the fuel for a particular purpose, the sturdy and stability of the energy fuel equipment required, and the fuel efficiency based on availability and cost. It is also plausible to find households in Ghana use specific energy fuel for specific use based on cultural values. It is common for households in Ghana to use traditional fuel for certain stable foods (like Kenkey, smoking of fish/meat, fufu, Banku, and Tuo-Zaafi) because of the longer length of cooking while using LPG for cooking meals such as rice. For this reason, groups of energy fuels with the same primary purpose were observed to be weak complements or near substitute goods to each other and not necessarily because one fuel cannot be consumed without the other. According to a Ministry of Energy (MOE) report on “Ghana Country Action Plan for Clean Cooking”, out of the 35 percent of households who depend on charcoal for cooking, the majority of these people also have in addition, access to LPG (gas) and use it when they need it. According to Wiedinmyer et al. (Citation2017), 15 percent of urban households use charcoal, wood, and LPG to cook the same meal compared to 1 percent of rural households who use these three energy fuels to cook.
If as anecdotes suggest, kerosene is used mainly for lighting, while FCO and LPG are used for cooking, then a change in the price of kerosene has very little to insignificant influence on household demand for FCO and LPG because households consume kerosene largely for lighting. As observed, a 1 percent increase in the cost of kerosene decreases the household quantity demanded for FCO by 0.06 percent and LPG by 0.09 percent. The insignificant impact on electricity (−0.02) however, shows that electricity is most preferred by households in Ghana for lighting.
4.3.3. Uncompensated price elasticity of demand for residential energy fuels by rural and urban households
Next is to compute the difference in demand for residential energy based on selected demographics. To estimate the difference in demand for energy, separate estimations are done for both rural and urban households. The results are summarized and presented in Table .
Table 9. Own price elasticity (uncompensated) of energy demand estimate based on selected demographics
From the table, rural households’ demand for all energy fuels is less price inelastic than urban households except for LPG (gas). This is likely because of the necessity of these fuels among urban households than among rural households. For instance, charcoal is more common in urban areas and has a smaller share in household energy expenditure probably because of affluence. Rural households, on the other hand, have lower costs of producing and transporting FCO than urban households, as such are less price inelastic to its demand.
Urban households are more likely to be connected to the national grid for cheaper and more stable electricity than rural households who may resort to alternative sources of light. Urban households in Ghana are also more likely to have equipment that uses electricity (e.g air conditioners, home theater systems, refrigerators, lights, television sets, etc.) than they are in rural households. The nature of modern houses built in urban households also contributes significantly to the increasing demand for electricity. Kerosene as an imported product is more accessible and affordable to urban households, though they are less likely to use it, than they are to rural households, owing to limited equipment, transportation, and distribution challenges. This explains the estimated lower price elasticity of demand for electricity, FCO, and kerosene among urban households than in rural households.
Notwithstanding, the price sensitivity of demand for LPG energy among rural households is lower than they are in urban households. Perhaps rural households enjoy government LPG subsidies more than urban households. Examples of such subsidies include the Unified Petroleum Price Fund (UPPF), the National energy policy, and the free distribution of CLPG cylinders and stoves initiative in rural areas all under the LPG promotion campaign.
4.3.4. Uncompensated price elasticity of energy demand based on sex and age of household head
Next, the study computes the demographic differences in uncompensated price elasticity of demand for energy fuels based on sex and age of the household head. To estimate this, we ran separate estimations for male households, female households, young household heads (< average age of household head), and older household heads (≥ average age of household head). The results are presented in Table . The results show that the price elasticity of demand for energy among both male and female household heads has a similar trend (i.e. inelastic) However, male-headed households are less price responsive to demand all fuels than female household heads except for electricity (which shows an insignificant difference). This is likely because male household heads are more engaged in active paid jobs that correspond with increased incomes and reduced poverty to afford these fuels than female household heads (see, Table ; Baah-Boateng, Citation2009; Twerefou et al., Citation2014). Similarly, male-headed households are more common in urban areas (see, Table ), have more access to these fuels, and own more equipment that uses modern fuels (e.g LPG). Younger household heads (lower than average age) are less price responsive to demand all energy fuels compared to older household heads except for electricity. This is likely because younger household heads are more common in urban areas and are more likely to be engaged in some active paid employment with increased income than older household heads (Baah-Boateng, Citation2009). They are also more inclined to use modern technological equipment that requires the use of modern energy (Osabuohien & Karakara, Citation2018).
4.4. Robustness check
Next, we compute for robustness check to correct for bias that may occur owing to zero observations in the data. Table shows the uncompensated price elasticities after correcting for bias cases of zero total residential energy expenditures. Simply put, the study considers the putative scenario where all fuel categories were equally available to all households. From the result, all energy fuels are price inelastic after correcting for zero expenditure. Also, the own-price elasticity for modern energy fuel (LGP and ECG) reduced slightly after the robustness check. With this empirical model, there is a statistically significant complementary effect between all energy groups, suggesting that households use multiple energy fuels with related purposes simultaneously for different purposes on different occasions based on economic and cultural reasons. The results may provide an opportunity for policies to encourage the availability of different energy fuels. That means the results could necessitate the call to induce the supply of energy services (especially modern fuel) to more communities through subsidies or waivers so
Table 10. Uncompensated elasticities with zero-expenditure adjustments
5. Conclusion and policy implications
The study estimates the price and expenditure responsiveness of household demand for four major residential energy consumed in Ghana; wood, charcoal and others (FCO), LPG (gas), electricity, and kerosene which are mostly consumed for cooking, lighting, heating, and other domestic purposes. The study further examines a sub-heterogeneous demand analysis by estimating the demand elasticities based on the location of the household and the sex of the household head.
The main findings of the study suggest that wood fuels remain the dominant energy fuel consumed by households for cooking (79 percent) while electricity is the dominant energy consumed for lighting (73.59 percent). The QUAIDS simulation shows a positive income effect and a negative price effect on household energy demand. Specifically, the paper finds that household demand for modern energy is income elastic (luxury goods), while demand for traditional/dirty fuel is income inelastic. Also, the price elasticity of demand for all residential energy is inelastic, even though households are more price sensitive to demand modern energy than traditional energy. Considering demographic factors, the study also estimates that urban households are more likely to use all energy fuels except kerosene, while larger-sized households are less likely to use LPG, electricity, and kerosene. Also, urban households are more price-insensitive to energyemand except for LPG. Male household heads are more price inelastic to demand all energy fuels except for electricity. In correcting for bias arising from zero expenditure, the study finds that the own-price elasticity for modern energy fuel (LGP and ECG) reduced slightly after the robustness check, even though the demand for all energy fuels remains negative and inelastic.
In policy recommendation, there should be a national initiative to widen and speed up the LPG promotion campaign, especially among rural and poor households. This initiative should be a private sector-led project with government support. Government support can take the format of subsidy, fiscal support, and provision of infrastructure (e.g facilitate land acquisition, road network, energy to plant sites, etc.) to private sector businesses who establish LPG sale points, manufacture LPG cylinders and ensure distribution of LPG to households especially in the hinterlands. Government should also consider the installation of free LPG plants for major public institutions like schools, hospitals, etc.
It is important government speed up the extension of the electrification project to the rural areas. Government may by way of policy introduce a “one household, one free meter” policy to motivate and speed up household use of electricity for domestic purposes. Government should also consider decreasing the electricity utility tariffs in Ghana especially among rural households through subsidies and also explore other sustainable renewable energy to provide a constant supply of electricity at a lower cost. This will discourage the use of alternative traditional energy that not only negatively affects the health of individuals but also pose a threat to the environment and agricultural productivity.
Future studies should consider estimating the demand elasticities of residential energy, based on separate use of each fuel type and the equipment used for each fuel.
Disclosure statement
No potential conflict of interest was reported by the author(s).
Additional information
Funding
Notes on contributors

Divine Kwaku Kutortse
Divine Kutortse is currently the Programme Officer at the National AfCFTA Coordination office of the Ministry of Trade and Industry, where he is responsible for Trade and Development Finance and Trade in service. He is also the scheduled office for the Market Expansion Project of the National AfCFTA Coordination Office. He holds a Master of Philosophy in Economics from the University of Ghana. He also holds a Bachelor of Arts in Economics from the University of Ghana. His areas of interest include; monetary economics, poverty reduction, energy economics, education economics, Development Economics, Trade policy and Finance, Small and Medium Enterprises Development, and Small Business Financing. His hobbies include reading novels, playing football, and swimming.
Notes
1. ** Poverty line description according to GLSS7 report = Very poor- ≤ GH 792.05 ($179.46); Poor- > GH 792.05 < GH 1314 and Non poor—≥ GH 1314 ($297.72) NB: 1 $ = GH 4.4134.
2. The study used STATA 15 by applying STATA’s nlsur command with the ifgnls option.
References
- Abdul-Wakeel Karakara, A., & Dasmani, I. (2019). An econometric analysis of domestic fuel consumption in Ghana: Implications for poverty reduction. Cogent Social Sciences, 5(1), 1697499. https://doi.org/10.1080/23311886.2019.1697499
- Adjei-Mantey, K., & Takeuchi, K. (2021). The effect of in utero exposure to household air pollution on child health: Evidence from Ghana. Health Policy OPEN, 2, 100029. https://doi.org/10.1016/j.hpopen.2020.100029
- Adusah-Poku, F., & Takeuchi, K. (2019). Energy poverty in Ghana: Any progress so far? Renewable and Sustainable Energy Reviews, 112, 853–22. https://doi.org/10.1016/j.rser.2019.06.038
- Adusah‐poku, F., Adjei‐mantey, K., & Kwakwa, P. A. (2021). Are energy‐poor households also poor? Evidence from Ghana. Poverty & Public Policy, 13(1), 32–58. https://doi.org/10.1002/pop4.301
- Aepli, M. (2014) Analysis of Final Demand for Food and Beverages in Switzerland, Unpublished Ph.D. Thesis, ETH Zurich Switzerland. DOI https://doi.org/10.3929/ethz-a-010251132
- Akbay, C., Boz, I., & Chern, W. S. (2007). Household food consumption in Turkey. European Review of Agricultural Economics, 34(2), 209–231. https://doi.org/10.1093/erae/jbm011
- Al-Bajjali, S. K., & Shamayleh, A. Y. (2018). Estimating the determinants of electricity consumption in Jordan. Energy, 147, 1311–1320. https://doi.org/10.1016/j.energy.2018.01.010
- Awan, R. U., Abbas, A., & Sher, F. (2014). An Investigation of Multidimensional Energy Poverty in Pakistan: A Province Level Analysis. International Journal of Energy Economics and Policy, 4(1), 65–75. https://www.econjournals.com/index.php/ijeep/article/view/645
- Baah-Boateng, W. (2009). Gender perspective of labour market discrimination in Ghana [Doctoral dissertation], University of Ghana.
- Banks, J., Blundell, R., & Lewbel, A. (1997). Quadratic Engel curves and consumer demand. Review of Economics and Statistics, 79(4), 527–539. https://doi.org/10.1162/003465397557015
- Barnett, W. A., & Seck, O. (2008). Rotterdam model versus almost ideal demand system: Will the best specification please stand up? Journal of Applied Econometrics, 23(6), 795–824. https://doi.org/10.1002/jae.1009
- Churchill, S. A., & Smyth, R. (2021). Energy poverty and health: Panel data evidence from Australia. Energy Economics, 97, 105219. https://doi.org/10.1016/j.eneco.2021.105219
- Deaton, A., & Muellbauer, J. (1980). An almost ideal demand system. The American Economic Review, 70(3), 312–326. https://www.jstor.org/stable/1805222
- Démurger, S., & Fournier, M. (2007). Rural poverty and fuelwood consumption: Evidence from Labagoumen Township (China). SSRN 553761.
- Denton, F. T., & Mountain, D. C. (2016). Biases in consumer elasticities based on micro and aggregate data: An integrated framework and empirical evaluation. Empirical Economics, 50(2), 531–560. https://doi.org/10.1007/s00181-015-0939-6
- Faisal, W. A., Brew-Hammond, A., & Osei, E. E. (2013). Relationships between energy use and income levels for households in Ghana. European Scientific Journal, 9(6), 233–245. https://eujournal.org/index.php/esj/article/view/1146
- Heinonen, J., & Junnila, S. (2014). Residential energy consumption patterns and the overall housing energy requirements of urban and rural households in Finland. Energy and Buildings, 76, 295–303. https://doi.org/10.1016/j.enbuild.2014.02.079
- Heltberg, R. (2003). Household fuel and energy use in developing countries: A multi-country study. The World Bank, 1–87. https://esmap.org/sites/default/files/esmap-files/Report_FuelUseMulticountryStudy_05.pdf
- Holt, M. T., & Goodwin, B. K. (2009). The almost ideal and translog demand systems. Quantifying consumer preferences.
- Ivanovski, K., Hailemariam, A., & Smyth, R. (2021). The effect of renewable and non-renewable energy consumption on economic growth: Non-parametric evidence. Journal of Cleaner Production, 286, 124956. https://doi.org/10.1016/j.jclepro.2020.124956
- Jones, R. V., Fuertes, A., Boomsma, C., & Pahl, S. (2016). Space heating preferences in UK social housing: A socio-technical household survey combined with building audits. Energy and Buildings, 127, 382–398. https://doi.org/10.1016/j.enbuild.2016.06.006
- Kwakwa, P. A., Wiafe, E. D., & Alhassan, H. (2013). Households energy choice in Ghana. Journal of Empirical Economics, 1(3), 96–103. https://www.researchgate.net/publication/270452842_Households_Energy_Choice_in_Ghana
- Leahy, E., & Lyons, S. (2010). Energy use and appliance ownership in Ireland. Energy Policy, 38(8), 4265–4279. https://doi.org/10.1016/j.enpol.2010.03.056
- Lenzen, M., Wier, M., Cohen, C., Hayami, H., Pachauri, S., & Schaeffer, R. (2006). A comparative multivariate analysis of household energy requirements in Australia, Brazil, Denmark, India and Japan. Energy, 31(2–3), 181–207. https://doi.org/10.1016/j.energy.2005.01.009
- Llorca, M., Rodriguez-Alvarez, A., & Jamasb, T. (2020). Objective vs. subjective fuel poverty and self-assessed health. Energy Economics, 87, 104736. https://doi.org/10.1016/j.eneco.2020.104736
- Mekonnen, A., & Köhlin, G. (2008). Biomass fuel consumption and dung use as manure: Evidence from rural households in the Amhara region of Ethiopia. Environment for Development Discussion Paper-Resources for the Future (RFF), (08–17).
- Mensah, J. T., & Adu, G. (2013). An empirical analysis of household energy choice in Ghana. Swedish University of Agricultural Sciences, Department of Economics. Working Paper Series 2013: Uppsala.
- Mensah, J. T., Marbuah, G., & Amoah, A. (2016). Energy demand in Ghana: A disaggregated analysis. Renewable and Sustainable Energy Reviews, 53, 924–935. https://doi.org/10.1016/j.rser.2015.09.035
- Ministry of Energy – Ghana. (2010). National electrification scheme (NES) master plan review (2011–2020). http://www.mida.gov.gh/pages/view/NES_Master_Plan_Review_Executive_Summary_Main_Report.pdf/111
- Nathaniel, S., & Khan, S. A. R. (2020). The nexus between urbanization, renewable energy, trade, and ecological footprint in ASEAN countries. Journal of Cleaner Production, 272, 122709. https://doi.org/10.1016/j.jclepro.2020.122709
- Ogwumike, F. O., Ozughalu, U. M., & Abiona, G. A. (2014). Household energy use and determinants: Evidence from Nigeria. International Journal of Energy Economics and Policy, 4(2), 248–262. https://www.econjournals.com/index.php/ijeep/article/view/708
- Olabisi, M., Tschirley, D. L., Nyange, D., & Awokuse, T. (2019). Energy demand substitution from biomass to imported kerosene: Evidence from Tanzania. Energy Policy, 130, 243–252. https://doi.org/10.1016/j.enpol.2019.03.060
- Oliveras, L., Artazcoz, L., Borrell, C., Palència, L., López, M. J., Gotsens, M., Marí-Dell’Olmo, M., & Marí-Dell’Olmo, M. (2020). The association of energy poverty with health, health care utilisation and medication use in Southern Europe. SSM-population Health, 12, 100665. https://doi.org/10.1016/j.ssmph.2020.100665
- Osabuohien, E. S., & Karakara, A. A. (2018). ICT usage, mobile money and financial access of women in Ghana. Africagrowth Agenda, 15(1), 14–18 https://journals.co.za/doi/10.10520/EJC-dc947bee3.
- Ouedraogo, B. (2006). Household energy preferences for cooking in urban Ouagadougou, Burkina Faso. Energy Policy, 34(18), 3787–3795. https://doi.org/10.1016/j.enpol.2005.09.006
- Poi, B. P. (2012). Easy demand-system estimation with quaids. The Stata Journal, 12(3), 433–446. https://doi.org/10.1177/1536867X1201200306
- Ray, R. (1983). Measuring the costs of children: An alternative approach. Journal of Public Economics, 22(1), 89–102. https://doi.org/10.1016/0047-2727(83)90058-0
- Shonkwiler, J. S., & Yen, S. T. (1999). Two‐step estimation of a censored system of equations. American Journal of Agricultural Economics, 81(4), 972–982. https://doi.org/10.2307/1244339
- Tchereni, B. H. M. (2013). An economic investigation into fuel-wood demand behaviour in South Lunzu Township in Malawi. Developing Country Studies, 3(4), 153–159 https://www.iiste.org/Journals/index.php/DCS/article/view/5412/5530.
- Thøgersen, J., & Grønhøj, A. (2010). Electricity saving in households—A social cognitive approach. Energy Policy, 38(12), 7732–7743. https://doi.org/10.1016/j.enpol.2010.08.025
- Tiwari, A. K., Eapen, L. M., & Nair, S. R. (2021). Electricity consumption and economic growth at the state and sectoral level in India: Evidence using heterogeneous panel data methods. Energy Economics, 94, 105064. https://doi.org/10.1016/j.eneco.2020.105064
- Twerefou, D. K., Senadza, B., & Owusu-Afriyie, J. (2014). Determinants of poverty among male-headed and female-headed households in Ghana. Ghanaian Journal of Economics, 2(1), 77–96. https://journals.co.za/doi/10.10520/EJC169145
- Twerefou, D. K., & Abeney, J. O. (2020). Efficiency of household electricity consumption in Ghana. Energy Policy, 144, 111661. https://doi.org/10.1016/j.enpol.2020.111661
- Vasseur, V., Marique, A. F., & Udalov, V. (2019). A conceptual framework to understand households’ energy consumption. Energies, 12(22), 4250. https://doi.org/10.3390/en12224250
- Wiedinmyer, C., Dickinson, K., Piedrahita, R., Kanyomse, E., Coffey, E., Hannigan, M., Oduro, A., & Oduro, A. (2017). Rural–urban differences in cooking practices and exposures in Northern Ghana. Environmental Research Letters, 12(6), 065009. https://doi.org/10.1088/1748-9326/aa7036
- Wright, A. (2008). What is the relationship between built form and energy use in dwellings? Energy Policy, 36(12), 4544–4547. https://doi.org/10.1016/j.enpol.2008.09.014
Appendix A
Table A11. QUAIDS estimated results