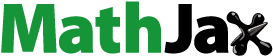
Abstract
The aim of the study was to determine extent of earning manipulations and possible fraudulent activities in banking sector. The study involved banks in East Africa where the financial statements of 34 banks in Kenya, 30 banks in Tanzania and 20 banks in Uganda were analysed. The study used Beneish model to classify banks as manipulators and non-manipulators. Later, probit regression model was applied based on the results of Beneish model to reclassify the banks as fraudulent or otherwise. The study revealed that 79.4% of Kenyan, 83.3% of Tanzanian and 70% of Ugandan banks could be non-manipulators. Further analysis showed that 79.4%, 76.7% and 80% of Kenyan, Tanzanian and Ugandan banks, respectively, were possibly not engaged in fraudulent activities. The study concluded that some banks could have been involved in earning management and fraud. The study contributes earning management literature by providing evidence that earning management is rife in banking sectors in less-developed countries though banks have strict regulations. The study further contributes to mechanisms of reclassification of institutions as either fraudulent or otherwise. The study recommends that financial statements prepared must be given professional autonomy. Further, it was recommended that the auditors should emphasise computations of ratios by financial statement prepared in order to give a clear, true and fair view of financial position of organizations. The study findings can be used by the management, regulators and government bodies in less developed and developing countries to develop policies that would seal the loopholes that fraudsters use.
1. Introduction
Earning management or earning manipulation may be a legal or illegal way or action that the management take to either increase the earnings or reduce the amount of losses to be reported (Ismail et al., Citation2015). The methods used to manipulate earnings may involve actual transactions or management of accruals. Accrual management arises due to flexibility of accounting standards where managers are allowed to select accounting policies and methods that they apply in estimations and judgment. On the other hand, measurements and recognition concepts are applied in actual transactions, which are sometimes not prudently applied (Pereira & Alves, Citation2017).
Manipulation of earnings has been classified as either white, black or grey. Earning manipulation, that is classified as white, is the manipulation that is meant to improve transparency in financial reporting, grey earning manipulation simply means the use of creative accounting in reporting for opportunistic purposes or for enhancing efficiency in financial reporting whereas black classification means that earning management was out rightly meant to misrepresent facts in order to defraud the organization (Roy & Debnath, Citation2015). Earning manipulation also referred to as fraudulent accounting may involve reclassification of expenses as non-recurrent and aggressively use of discretionary accruals (Shahzad, Citation2016). Financial statements of the business enterprises are the main sources of information and therefore the main focus in corporate reporting should be the quality of earnings reflected in financial statements. Despite the importance of financial statements as a source of information, people who prepare the financial statements have freedom of selecting the applicable accounting rules and estimations in order to make financial reports informative (H. R. Talab et al., Citation2018).
Earning management may arise when the managers intervene or directs the way the process of financial statement reporting is undertaken. This intervention may be to influence the people preparing the financial statement to create a perfect picture of the performance of the business to the stakeholders and also influence those contracts that mostly depend on the financial health of businesses that is reflected through financial statement (Haque et al., Citation2016). The directives from the managers on how to prepare the financial statements may be detrimental to the survival of the organization as most of them are not professional accountants or financial analysts. Pressure from different quarters may force managers to practice earning management. The shareholders of the business, the market, urgency and importance of raising finances to finance a given project or activity may increase the pressure on the management to manipulate financial statements (Drábková, Citation2015). The motivations for manipulation of earnings discussed may give the management the intended results in a short while, though the consequences of their actions may be dire at the end.
The management may be motivated to manipulate the financial statements to ensure that the set financial targets are met as reflected in financial statements as opposed to the real situation. Managers are likely to manipulate financial statements by managing the abnormal accruals (Abbadi, Hijazi & Al-Rahahleh, Citation2016). Further motivations of earning management may include initial public offer of the stocks of the company, incentive to change the chief executive officer and motivation of hefty bonuses (Muda et al., Citation2018). Although the managers may succeed in hoodwinking the shareholders and investors that the financial health of the company is sound, the company may find itself in financial distress if the practice continues.
Earning management may also be due to cost of capital, though this motivation is not important as compared to motivation based on the management’s earnings. The managers may not have incentive to use equity for earning management as the owners of the business decide dividends to be paid. Consequently, managers use debt as motivation for earning management because if the company reports higher earnings in financial statements, it makes the business more attractive to lenders (Nikoomaram et al., Citation2016). Earning management may be used by managers as a tool to avoid taxes, to evade political costs and in some cases to improve the worthiness through stock prices in the eyes of lenders (Shanwari & Shah, Citation2015). Taxes paid to government reduce the amounts distributed as dividends to shareholders as well as increased bonuses for managers based on reported profits. Therefore, avoidance of taxes may be beneficial to the management (increased bonuses), shareholders (increased dividends) and improved stock prices benefit the entire organization by providing more capital for expansion. However, the benefits may not last for long especially when earning manipulation was in contravention of the laws.
Agency problems lead to earnings management due to inconsistency between the stakes of the business owners and business management’s interest. Management has an advantage of having more information than other stakeholders, which leads to information asymmetry (Sari & Sari, Citation2019). Due to information asymmetry, managers make opportunistic decisions as the other stakeholders do not have sufficient information that they can use to question the management’s actions (Haque et al., Citation2016). As per agency theory, information asymmetry is one of the reasons why management make opportunistic decisions, however, this may not be entirely true as some decisions may require quick deliberations thus no need to involve other stakeholders and in other cases, some stakeholders may not fully understand some information. Therefore, need or importance for full disclosure of information is defeated.
Large- and medium-sized firms are likely to practice earning management to report better results. Among the large- and medium-sized firms, it was estimated that between 30% and 40% of the firms engage in earning management in order to report positive earnings, while other firms estimated to be between 8% and 12% practice earning management to report higher earnings than really earned incomes (Ali et al., Citation2015). Earning management identification is important as it enables the users of financial statements examine the economic performance of the business, be able to forecast the profitability of the company as well as be able to correctly find out the real value of the firm. Earning management can be determined by the use of M-score (Mahama, Citation2015). The fraudsters manipulate the financial statements by overstating elements like assets, sale and profits and on the other hand by reducing the amount of liabilities and losses reported. These form of fraud have increased in number (Mehta & Bhavani, Citation2017).
Numerous studies about earning management have been undertaken. Paolone and Magazzino (Citation2014) undertook study on earning management in Italy. In their research, they analysed food, textile, automotive clothing as well as metallurgic industries. Omar et al. (Citation2014) researched on financial statement fraud in Megan Media Holdings Berhad a company established in Malaysia to produce components of electronics, plastic injections and auto parts. It later expanded operations to include manufacture of digital versatile discs. Mahama (Citation2015) undertook a research on corporate fraud and financial statement manipulation in Enron a USA company which collapsed. Mehta and Bhavani (Citation2017) studied the financial statements of Toshiba a Japanese company to determine whether there was evidence of earnings manipulation.
The studies reviewed were either from a single country or based on the information from a single organization and none focused on banking sector. The studies also focused on developed economies or developing but not less developed countries. Hardly any research focused on less developed countries. The aim of this study was to determine extent of earning manipulations and possible fraudulent activities in banking sector. The study focused on less developed East African banking sectors that have not received enough attention from the scholars as witnessed in the background studies undertaken by various researchers. This study bridged that gap by focusing on banking sector in three East African countries of Kenya, Tanzania and Uganda. These countries formed the original East African Community block and have treaties on economies, politics and monetary policies.
The study is unique in that there is no study that has combined and compared the extent of earning management and fraud in East African banking sectors. The study has provided additional aspect of not only demonstrating the existence of earning management (which can be legal or illegal), but also re-categorising the act of earning management as fraudulent or otherwise. Beneish model is applied in determination the existence of earning management only. Earning management has been categorised as white (to improve transparency), grey (use creative accounting for opportunistic purposes) and black (misrepresentation of facts). Under Beneish model, there are no distinctions in aforementioned classes of earning management. This study has gone beyond demonstration of earning management presence to re-evaluation of earning management acts and categorising the acts as fraudulent or otherwise. The additional analysis and classification of earning management is new phenomenon in banks and more so in developing countries or emerging economies to the best of my knowledge. This study has therefore added to the literature of earning management and fraud by providing additional mechanism of categorising earning management as either fraudulent or non-fraudulent. The study adds to the literature of earning management in different ways which include: provision of evidence through empirical study that earning management and manipulation of financial statements is also persistent in less developed countries and more so in East Africa. The study has provided evidence that even in banks where there are strict regulations earning management is rife. The study contributes to mechanisms used to classify firms as either manipulators or non-manipulators of financial statements. Finally, the study has extended the application of Beneish model in determination of earning management in banks. The rest of the paper is organized into the following sections: background of banking sector in East Africa is presented in section 2, literature review is presented in section 3, methodology applied in the study is presented in section 4, tests of assumptions have been presented in section 5, data analysis and findings are presented in section 6 and finally discussions, conclusions and recommendations are presented in section 7.
2. Background of banking sector in East Africa
Kenya, Tanzania and Uganda formed East Africa Community (EAC) in 2000. Other countries that include Burundi and Rwanda were admitted to EAC in 2007, whereas the last entrant to EAC, South Sudan was admitted to the community in 2016. The EAC ratified a monetary union in 2015 and it is expected to culminate in single currency in 2024. Under this monetary union treaty, the EAC established protocols to supervise banks among other things (Dridi & Nguyen, Citation2018). Liberalization of finance led to growth of banking sector in East Africa (Mathuva & Nyangu, Citation2021a). Monetary affairs committee in EAC developed protocols to enhance harmonization of legal, supervisory and practices of central banks in East Africa (European Investment Bank European Investment Bank, Citation2016).
Kenyan banking sector is largest in EAC (European Investment Bank, Citation2016). Kenya has 42 licensed commercial banks of which 13 are foreign. Banking sector in Kenya is dominated by Tier 1 banks that control 75% of the entire sector (Mathuva & Nyangu, Citation2021a). Kenyan banking sector experienced the worst banking crisis between 1984 and 1999 where 24 banks collapsed. Between 2014 and 2016, three commercial banks collapsed. The failures of these banks were mainly non-performing loans, weaknesses in corporate governance and unregulated advances of loans, which were fraudulent (Mathuva & Nyangu, Citation2021a).
Tanzania has 37 registered commercial banks (Makanile & Pastory, Citation2022). In recent past, Tanzania banking sector has not experienced major crisis. However, in 1990, the government-owned banks were found to be insolvent and they were controlling 95% banking sector’s assets. This loss was equated to 10% on the country’s gross domestic product. Later in 1994, the banking sector in Tanzania had non-performing loans of between 60% and 80% (Mathuva & Nyangu, Citation2021a).
In Uganda, the number of registered banks has increased to 25 in 2020 as compared to 1999 where there were 20 registered banks. The banking sector in Uganda is considered as one of least developed in sub-Saharan Africa mainly due to government domination and control, inefficiency and minimum competition (Katusiime, Citation2021). Fifty per cent of banks in Uganda were insolvent by 1994. Between 1998 and 1999, Ugandan-banking sector experienced a crisis where four banks that had a control of 12.1% of the entire banking sector’s deposit collapsed. The collapse was mainly due to corruption and insider lending (Mathuva & Nyangu, Citation2021a).
3. Literature review
The study is guided by the agency theory. The theory was coined by Jensen and Meckling in 1976 and it stated that a party referred to as principal contracts another party referred to as agent to transact business on his/her behalf. In this theory, it is observed that the agent has more information and the principal is always disadvantaged information wise (Najah & Omar, Citation2018). In agency relationship, the contract between the agent and the principal mostly sets objectives based on the interests of the principal; however, the agent in this the management of the business will pursue mostly their own goals at the detriment of the principal (Nwofia, Citation2018). The managers have been described as self-serving where they try to achieve their personal goals as opposed to the shareholders’ interest which if not checked may affect owners’ wealth maximization negatively (Huang et al., Citation2016). The agency theory interprets information asymmetry as withholding of information by the management at the detriment of other stakeholders for opportunistic purposes. This assertion may not be entirely true, as managers may not disclose the information due to technicality of the issue at hand, sensitivity of issue to be addressed and also little value that the disclosure will have to the stakeholders. Agency theory puts the management at the centre of decision-making on behave of other stakeholders, in earning management, the managers direct the financial statement preparers on what to do in order to report favourable results. Therefore, agency theory was essential in this study.
The study of earning management or manipulation has been extensive in various countries and economic sectors. Paolone and Magazzino (Citation2014) studied earning management among various main sectors in Italy which included food, textile, automotive clothing as well as metallurgic industries. In the study, 562 firms from textile sector were sampled, 779 firms from food industry, 344 firms from clothing industry were covered, 424 firms in automotive industry and 489 firms from metallurgic industry. The study utilized financial statements of the sampled companies from 2005 to 2012. The study applied Beneish model and the results showed that 51.4% of the firms used in the study had higher probability of earning manipulation. Mahama (Citation2015) undertook a research on detection of corporate fraud and financial statement manipulation in Enron an American company. The researcher analysed financial statements of Enron from 1996 to 2000. The results indicated that Enron manipulated earnings in 1998 based on Beneish model findings. Mehta and Bhavani (Citation2017) studied the financial statements of Toshiba a Japanese company. The study evaluated financial statements for seven years from 2008 to 2014 to determine whether there was evidence of earnings manipulation; however, using Beneish model they concluded that the company had not participated in earnings manipulation for seven years that were analysed in this study.
A research was conducted in Megan Media Holdings Berhad a Malaysian company by Omar et al. (Citation2014), the researchers analysed the financial statements for three consecutive years from 2005 through 2007. The results from the study by Omar et al. (Citation2014) on financial statement fraud using Beneish model found that the company manipulated the accounts. M-Score model is used to determine whether an organization has engaged in earning manipulation the variables are derived from financial statement of the organization and are used establish the extent of earning manipulation (Omar et al., Citation2014).
The studies reviewed have indicated the presence and absence of financial statement manipulations in various sectors and countries. However, these studies did not provide details on financial consequences, legality or illegality of such actions. The studies did not indicate the financial health of the companies involved in earning management, though Enron collapsed in 2001, the analysis by Mahama (Citation2015) showed that Enron was involved in financial statement manipulation in 1998. Consequently, more research is required to combine several models and analysis tools to project the financial distress and eventuality of company failure.
3.1. Beneish model
Beneish m-score model applies ratios to test earning manipulation. It has been advocated for as a tool for determining whether there is earnings manipulation on financial statements (Mavengere, Citation2015). There are two versions of Beneish model; an 8-variable model and 5-variable model. The five-variable model is appropriate in the study as three ratios in 8-variable model were found to be meaningless (Paolone & Magazzino, Citation2014). Paolone and Magazzino (Citation2014) stated that Sales, General and Administrative Expenses Index, Leverage Index and Total Accruals to Total Assets were found to be meaningless or insignificant. The Beneish 5-variable model is demonstrated as:
M = −6.065 + 0.823*DRSI+ 0.906*GMI + 0.593*AQI + 0.717*SGI + 0.107*DEPI
Days’ Sales in Receivable Index (DRSI), is a ratio used to compare the receivables from core business (sales receivables) of the current period with receivables of the previous period with an aim of exposing any inflation of revenues (Mavengere, Citation2015). Any value greater than one of this index shows increase in the sales receivables; however, any unprecedented increase of this index may indicate inflation of revenues (Mahama, Citation2015). Gross Margin Index (GMI), this index compares the margins of the current period with that of the previous period. Any deterioration in this index creates doubts for the future prospect of the organization and may lead to manipulation of earnings (Paolone & Magazzino, Citation2014). Asset Quality Index (AQI), this index compares the non-current assets and total assets, though the property, plant and equipment are not included in non-current assets while computing this ratio (Mehta & Bhavani, Citation2017). Increase in this ratio may indicate capitalization and deferment of costs (Ramírez-Orellana et al., Citation2017). Sales Growth Index (SGI), this ratio compares the sales of current period with the sales of previous period. Any value greater than one indicates there is growth in sales which may be interpreted as manipulation of earnings (Mahama, Citation2015). The pressure mounted on managers to meet specific growth goals may lead to managers manipulating the financial statements to show growth (Ramírez-Orellana et al., Citation2017). Depreciation Index (DEPI), this ratio compares the depreciation of the current period with that of the previous period. The firm may adopt methods of depreciation that would reduce the depreciation thus increasing the profits. The firms may also adjust the economic life of the assets upwards to reduce the amount of depreciation expense (Mavengere, Citation2015). The increase in income due to depreciation reduction may be as a result of earning management (Mehta & Bhavani, Citation2017).
Beneish model mainly is applied to determine the extent of earning management but cannot be used to predict bankruptcy of the firm (Mahama, Citation2015). This assertion pinpoints the weakness of Beneish model, though the model indicate the presence of earning management, the stakeholders cannot evaluate the continuity of their business by using this model. Therefore, it is prudent to combine Beneish model with other models and analytical tools to establish whether financial manipulation detected may lead to collapse or failure of the firm. This combination of models and other analytical tools will provide the stakeholders with clear picture of financial health and perpetual succession of the firm. Roy and Debnath (Citation2015) categorised earning management as white (to improve transparency), grey (use creative accounting for opportunistic purposes) and black (misrepresentation of facts), Beneish model cannot categorise the actions of manipulators on whether they are legal or illegal. Therefore, it is necessary to have more mechanisms to foretell whether the actions are legal or otherwise.
4. Methodology
The study used content analysis research method to analyse financial statements of banks in East Africa. Content analysis entails either qualitative or quantitative analysis of verbal, visual or written documentation (Koufogiannakis et al., Citation2004). The content analysis was used because the study required audited financial statements for ratios computation. The disclosures in banking sector are reliable through audited statements as stipulated by Lotto (Citation2019). Content analysis is free from bias, therefore, required to analyse documented evidence (in this case audited financial statements) objectively rather than seeking answers from individuals who may not be truthful. Census was applied for analysis in this study, as all banks in the three East African countries were the target population. The East African banks were used as they have financial treaties or monetary union that makes them have almost similar laws and banks regulations. Kenya, Tanzania and Uganda have the most developed banking systems in Eastern Africa and their laws facilitate transparency in publication of financial statements. Therefore, banks in these three countries were most suitable for the research. Kenya has 42 registered banks; Tanzania has 37 banks and Uganda has 25 banks. The study however, analysed financial statements of 34 banks in Kenya, 30 banks in Tanzania and 20 banks in Uganda for a period of five years between 2016 and 2020. This period was suitable as it preceded immediate collapse of three Kenyan banks between 2014 and 2016. The period was selected, as financial statements for those periods were readily available. The omitted banks either were in process of merger or acquisition, the data sought was not available at the time of research and other banks operated in all the three countries and therefore group’s financial statements were analysed. The study first applied Beneish M-score model to categorize the banks as likely manipulators or non-manipulators. Beneish model used was the 5-variable model rather than 8-variable model. The five-variable model was selected because some three variables from the original eight-variable model namely; Sales, General and Administrative Expenses Index, Leverage Index and Total Accruals to Total Assets were found to be meaningless or insignificant (Paolone & Magazzino, Citation2014). Maccarthy (Citation2017) stated that Beneish model alone cannot be used check earning manipulation completely. It is advisable to use other analytical tool to strengthen the findings of the study (Omar et al., Citation2014). Determination of fraudulent and or non-fraudulent firm is a dichotomous variable. Ordinary least method cannot be applied in such kind of study (Shanwari & Shah, Citation2015). The study further applied probit regression model to establish whether the banks engaged in fraudulent activities. The model is used to generate new m-score based on the average derived from non-manipulators as classified using Beneish model. The Beneish model used in the study is as follows:
M = −6.065 + 0.823*DRSI+ 0.906*GMI + 0.593*AQI + 0.717*SGI + 0.107*DEPI
Where;
The Probit regression model used in the study was:
Where 1 represents fraud
4.1. Test of regression assumptions
4.1.1. Normality test
Shapiro-Wilk (SW) test is used to test normality of data if the number of items ranges between three and five thousand (3 ≤ n ≤ 5000) (Razali & Wah, Citation2011). Razali and Wah (Citation2011) stated that the value of statistics (W) is between one and zero and that the value of one indicates normality of data. shows the results of normality test using Shapiro-Wilk test.
Table 1. Normality test
The statistics from the test are shown in Table and are as follows; DSRI had a value of statistic of 0.915 and p value of 0.125, GMI had a W of 0.956 and p value of 0.147, AQI had a W of 0.886 and p value of 0.478, SGI had a W of 0.881 and p value of 0.233, finally DEPI had W of 0.886 and p value of 0.123. The null hypothesis of Shapiro-Wilk test states that, data is from normally distributed population. The p value from the test for all parameters are greater than 0.05, and therefore it was concluded that the data was normally distributed.
4.1.2. Test of heteroscedasticity
Breusch Pagan and Koenker test was carried out to confirm existence of non-constant variances of errors in the data (Osoro et al., Citation2015). The variances of errors are assumed to be constant across observations. The results of Test of Heteroscedasticity are presented in Table
Table 2. Test results of heteroscedasticity
shows the results of heteroscedasticity the p value of Breusch Pagan test was 0.197 and that of Koenker test was 0.205. The p values are greater than 0.05 therefore, the null hypothesis that there is no heteroscedasticity was not rejected and it was concluded that there was no heteroscedasticity.
4.1.3. Test of multicollinearity
Existence of multicollinearity among independent variable will lead to wrong regression results (Palaniappan, Citation2017). The variables were tested for multicollinearity using tolerance and variance inflation factor (VIF). Khalaf and Iguernane (Citation2016) indicated that any value of VIF beyond 10 indicates presence of multicollinearity. The results of multicollinearity test are presented in Table
Table 3. Test of multicollinearity results
The results of multicollinearity test using tolerance and VIF are shown in . A VIF for DSRI was 1.222, GMI had VIF of 1.436, AQI had VIF of 1.135 and SGI and DEPI had VIFs of 1.220 and 1.430 respectively. The values of VIF for all parameters were below 10, which indicated that there was no multicollinearity. The value of tolerance for DSRI, GMI, AQI, SGI and DEPI were 0.818, 0.696, 0.881, 0.819 and 0.699 respectively. The tolerance values for all parameters were above threshold of 0.10 which ruled out presence of multicollinearity. It was therefore prudent to conclude that there was no multicollinearity between the independent variables.
4.1.4. Test of autocorrelation
Durbin-Watson test was applied to check presence of autocorrelation. Gan and Ahmad (Citation2011) stated that any value of between 1.5 and 2.5 indicates independence of error terms. The results on autocorrelation are provided in
Table 4. Autocorrelation test results
The value of Durbin-Watson test in was 2.246. The value Durbin-Watson test obtained is less than 2.5 and more than 1.5 which indicates that there was no autocorrelation between variables. Therefore, it was concluded that the error terms were independent.
5. Data analysis and findings
This section presents the findings from data analysed using both Beneish model and probit regression model. The study utilised available audited financial statements of banks in East Africa for the years 2016 through 2020. The findings were analysed country by country. Beneish model is first used to classify the banks as manipulators of non-manipulators. The probit regression model was later applied for further analysis based on the averages derived from non-manipulators to find out the banks that were involved in fraudulent activities as suggested by Feruleva and Maria (Citation2017).
Beneish model provides certain thresholds for each ratio. DSRI has a threshold of 1.031 or less for a firm to be regarded as non-manipulator and anything beyond but a ratio of 1.465 and beyond, the firm is categorised as manipulator of financial statements. For GMI, a ratio of 1.041 or less may mean that the firm is not involved in manipulation of financial statements, whereas a ratio of f 1.193 or more indicates presence of manipulation. A ratio of one or more in AQI, pinpoints possibility of manipulation through expense or intangible assets capitalization. In interpretation of SGI, a ratio of 1.134 or less leads to categorising a firm as non-manipulator, but any value equal or more than 1.607 means that the firm is a manipulator. Manipulation of DEPI is represented by a value of 1.077 or more, whereas non-manipulation is represented by a value of 1.001 or less (Akra & Chaya, Citation2020).
5.1. Analysis of banks using Beneish model
In analysis of banks using Beneish model, value of 1 represents manipulators while 0 represents non-manipulators for data in .
Table 5. Kenyan banks classification
Table 6. Classification of Tanzanian banks
Table 7. Classification of Ugandan banks
5.2. Analysis of Kenyan banks
Results in show classification of Kenyan banks as manipulators and non-manipulators based on Beneish model.
The results presented in shows that 17 Kenyan banks representing 50% did not manipulate DSRI, Four banks representing 12% of analysed banks were found to have manipulated DSRI whereas 13 banks representing 38% were on borderline as per threshold presented by Akra and Chaya (Citation2020). The results further indicate that 18 (53%) of the Kenyan banks did not manipulate GMI, however the remaining 16 banks were either in borderline (8 banks) or manipulated (8 banks) the GMI. Akra and Chaya (Citation2020), indicated that any value of AQI equal or exceeding one is an indication of manipulation, based on this assertion, 15 banks were found to be non-manipulator and 19 banks were categorised as manipulators of AQI. The analysis further revealed that 29 banks were not involved in manipulation of SGI, four banks were in borderline while 1 bank manipulated SGI. The final analysis was on DEPI, the threshold provided Akra and Chaya (Citation2020) showed that 47% (16 banks) were non-manipulators of DEPI, 35% (12 banks) were involved in manipulation of DEPI and 6 banks translating to 18% of analysed banks were on borderline.
Under Beneish five-variable model, any M-score that exceeds −2.76 indicates possibility of earning manipulations (Feruleva & Maria, Citation2017). The results show that twenty-seven banks translating to 79.4% on the banks analysed are likely non-manipulators based on the Beneish Five-variable model. This means that approximately 20.6% of the banks analysed likely engaged in earning manipulations.
5.3. Analysis of Tanzanian banks
The analysis and classification of Tanzanian banks as manipulators and non-manipulators based on Beneish model are presented in .
The results presented in shows that 18 banks in Tanzania representing 60% did not manipulate DSRI, three banks representing 10% of analysed banks were found to have manipulated DSRI whereas nine banks representing 30% were on borderline as per threshold presented by Akra and Chaya (Citation2020). Further, it was found that 19 (63%) of the Tanzanian banks did not manipulate GMI, whereas five banks manipulated the GMI and six banks were in borderline representing 17% and 20% respectively. Akra and Chaya (Citation2020), indicated that any value of AQI equal or exceeding one is an indication of manipulation, based on this assertion, 13 banks (43%) were found to be non-manipulator and 17 banks (57%) were categorised as manipulators of AQI. The analysis further revealed that 27 banks in Tanzania representing 90% did not manipulate SGI, whereas 10% (4 banks) of banks in Tanzania were in borderline. Lastly, in analysing DEPI using threshold provided Akra and Chaya (Citation2020) it was found that 12 banks (40%) were not involved in manipulation of DEPI, 16 banks representing 53 % of banks analysed were involved in manipulation of DEPI and 2 banks translating to 7% of analysed banks were on borderline.
The findings of Beneish model analysis on Tanzanian banks shows that five banks out of thirty banks analysed translating to 16.7% were likely manipulators. This means that approximately 83.3% of Tanzanian banks are likely manipulators. The results of the Beneish model show that more Kenyan banks are likely manipulators as compared to Tanzanian banks; this may be due to strict banking regulations and supervision in Tanzania as compared to supervision in Kenya.
5.4. Analysis of Ugandan banks
Analysis results for specific ratios and classification of Ugandan banks are shown in . The results show that five of banks analysed representing 25% did not manipulate DSRI, thirteen banks representing 65% of analysed banks were on borderline whereas two banks representing 10% were found to have manipulated DSRI as per threshold presented by Akra and Chaya (Citation2020). The results further indicate that 11 (55%) of the Ugandan banks did not manipulate GMI, whereas, 6 banks representing 30% manipulated the GMI and the remaining three banks (15%) in borderline. Akra and Chaya (Citation2020), indicated that any value of AQI equal or exceeding one is an indication of manipulation, based on this assertion, 10 banks were found to be non-manipulator and 10 banks were categorised as manipulators of AQI. The analysis further revealed that 14 banks (70%) were not involved in manipulation of SGI, five banks (25%) were in borderline while 1 bank (5%) manipulated SGI. The final analysis was on DEPI, the threshold provided Akra and Chaya (Citation2020) showed that 75% (15 banks) were non-manipulators of DEPI, 10% (2 banks) were on borderline and three banks translating to 15% of analysed banks were involved in manipulation of DEPI.
Results of banks analysis in Uganda is presented in Table . The results show that approximately 30% of banks analysed engage are likely manipulators. This means that 70% of the banks may not have engaged in earning manipulation in Uganda. The Ugandan banks are shown to be worse in manipulation of earnings based on Beneish five-variable model. This may be because of either lax in supervisory responsibilities of Central bank or deliberate actions of the preparers of financial statements.
5.5. Analysis using probit regression model
The financial statements were further analysed to reclassify the banks as either fraudulent or non-fraudulent. The probit regression model was used for this purpose. The averages of non-manipulators based on Beneish model were used as bench mark variables to develop a new M-score that was used to categorise the banks as fraudulent or not as suggested by Feruleva and Maria (Citation2017). The findings using probit regression model for each country were presented separately as the averages applied are for specific banks from different nations.
5.6. Probit regression results
The average M-score for all non-manipulators from Beneish model was obtained and used as benchmark variable to categorise the banks as fraudulent or non-fraudulent. The probit regression model was applied for the purpose of generating new m-score that was used to determine whether the banks were involved in fraudulent activities as applied in the study by Feruleva and Maria (Citation2017). The benchmark values were derived from all perceived non-manipulators based on Beneish model. represents the averages of all non-manipulators for all applied ratios.
Table 8. Non-Manipulators averages based on Beneish M-score
Results in show the averages of the banks that were categorised as non-manipulators. The averages for DSRI was 1.0964, the average for GMI was 0.8093, the averages for AQI, SGI and DEPI were 0.9579, 1.0009 and 1.0571 respectively. These averages were used to derive a new m-score to recategorise the firms as fraudulent or non-fraudulent.
The probit regression coefficients and the likely hood ratio are presented in Table .
Table 9. Probit regression coefficient
The results in show coefficients obtained from probit regression for different parameters as follows; 16.536 for DSRI, 16.972 for GMI, 12.848 for AQI, 12.363 for SGI and −0.007 for DEPI. The scale of 1 from the indicate that manipulators were taken as response thus non-manipulators were taken as reference category. The results further show a likelihood chi-square value of 87.289 and p value of 0.0001 which shows that the model fits well. The new coefficients obtained from probit regression analysis are substituted in the Beneish model to obtain the revised m-score. The revised m-score model is given as..
Revised M-Score = −67.954 + 16.536 DSRI + 16.972GMI + 12.848 AQI + 12.363 SGI—0.007 DEPI
Using the revised m-score model, the new m-score model was applied to determine whether the banks used in the study actually involved in fraudulent activities. The revised m-score computed (−11.4147) as shown in the model given was applied in categorising banks as fraudulent or non-fraudulent.
Revised M-Score = −67.954 + 16.536 (1.0964) + 16.972 (0.8093) + 12.848 (0.9579) + 12.363 (1.0009)—0.007 (1.0571) = −11.4147
shows ratios averages of all non-manipulators (benchmark ratios), revised ratios (parameters) and new m-score used for classifying banks as fraudulent or non-fraudulent
Table 10. Benchmark and revised variables
The results in show the averages of non-manipulators’ variables, the revised variables from probit analysis and the revised m-score. The revised m-score of −11.4147 was used to categorise the banks as fraudulent or non-fraudulent.
5.7. Classification of banks as fraudulent or non-fraudulent
The classifications of banks to determine whether they were fraudulent or otherwise were presented per country. The fraudulent banks were coded as 1 and non-fraudulent were coded as 0 for data in .
Table 11. Probit regression revised M-score results of Kenyan banks
Table 12. Probit regression revised M-score results of Tanzanian banks
Table 13. Probit regression revised M-score results of Ugandan banks
shows the results of Kenyan banks classified as fraudulent or non-fraudulent based on new m-score derived from probit analysis.
The results show that seven banks out of the total thirty-four banks analysed translating to 20.6% could have engaged in fraudulent activities. This is exactly the same percentage that was categorised as manipulators in Beneish model. The remaining 79.4% were categorised as non-fraudulent.
shows the results of Tanzanian banks classified as fraudulent or non-fraudulent based on new m-score derived from probit analysis.
Probit model analysis for Tanzanian banks showed that seven banks out thirty banks analysed could be potentially fraudulent. This number translates to 23.3% this is slightly above the results of Beneish model which categorised 16.7% as potential manipulators. shows categorization of Tanzanian banks.
shows the results of Ugandan banks classified as fraudulent or non-fraudulent based on new m-score derived from probit analysis.
The results on analysed Ugandan banks presented in shows that only four out of twenty banks analysed are categorised as potential fraudulent. This translates to 20%, which is less than the predicted number based on Beneish model that translated to 30% potential manipulators.
The results of analysis for all banks in East Africa showed that there were fraudulent and non-fraudulent activities. The results are concurrent with the study undertaken by H. Talab et al. (Citation2017) titled “Role of Beneish m-score model in detecting of earnings management practices: Empirical study in listed banks of Iraqi stock exchange” in this study it was revealed that 15 banks constituting 62.5% of banks analysed manipulated their profits whereas the 34.8% were not involved in profit management. Sujeewa and Kawshalya (Citation2020) undertook study on corporate financial statements in Sri Lanka for a period between 2013 and 2019, in their study they deduced that some of listed companies were involved in financial statement manipulations. The results indicated that 79%, 89%, 69%, 76%, 80%, 82% and 81% of all companies analysed were involved in financial statement manipulations for the years 2013, 2014, 2015, 2016, 2017, 2018 and 2019 respectively. The findings were similar to the results of this study as not all companies were found to be fraudulent. The study was contradicting the findings of Gyawali (Citation2021) who explored application of Beneish model on selected private banks in Nepal. The study concluded that Beneish model could not detect financial fraud in Nepalese private banks.
6. Discussion, conclusion and recommendations
The aim of the study was to determine extent of earning manipulations and possible fraudulent activities in banking sector. The study was based on banks in East Africa region. The study found that some banks were involved in earning management and further analysis using probit regression indicated that some banks may be involved in fraudulent activities. The study results were similar to the findings by Feruleva and Maria (Citation2017) who found that some firms in Russia were engaged in earning management and fraud. Approximately 51.4% of Italian companies were found to have been involved in possible earning management based on Beneish model (Paolone & Magazzino, Citation2014). H. R. Talab et al. (Citation2018) studied earning management in banks listed in Iraq stock exchanged. The study applied Beneish model and it was found that the 65.2% of the banks analysed were involved in earning management. The results however were different from the study undertaken by Mehta and Bhavani (Citation2017) who analysed financial statements for evidence of earnings manipulation, however using Beneish model they concluded that the company had not participated in earnings manipulation for seven years that were analysed in this study.
Based on study findings, some banks have engaged in both earning management and fraud. Therefore, it was concluded that banking sector in East Africa has not fully eliminated earning management and fraud. This study recommends a policy development that will ensure that the management is separated from those who prepare financial statements. The personnel who prepare financial statements should be given professional autonomy to be able to decide treatments of certain financial statements items especially the accruals that mostly get misused for earning management purposes. Further the study recommends that the auditors should ensure that ratios are computed during preparation of financial statements so as to detect any manipulation early enough.
The study contributes to the literature of earning management by proving evidence through empirical study that earning management and manipulation of financial statements is also persistent in less developed countries and more so in East Africa. The study has provided evidence that even banks where there are strict regulations practice earning management. The study further contributes to mechanisms used to classify firms as either manipulators or non-manipulators of financial statements. Lastly, the study has extended the application of Beneish model in determination of earning management in banks. This study will be helpful to the management of banking sector especially in developing and less developed countries as it has pinpointed the possibility of earning management and fraud in financial statements in rather professionally prepared financial statements. Bank regulators and government agencies in these countries could also utilize the findings of the study to develop policies that would tighten the loopholes that might be utilized to perpetrate these fraudulent acts.
Declaration statement
The authors have no conflict of interest or financial interest.
Disclosure statement
The research was not supported by any agency or organization.
Additional information
Funding
References
- Abbadi, S., Hijazi, Q., & Al-Rahahleh, A. (2016). Corporate Governance quality and earnings management: Evidence from Jordan. Australasian Accounting, Business and Finance Journal, 10(2), 54–20. https://doi.org/10.14453/aabfj.v10i2.4
- Akra, R. M., & Chaya, J. K. (2020). Testing the effectiveness of altman and beneish models in detecting financial fraud and financial manipulation: Case study kuwaiti stock market. International Journal of Business and Management, 15(10), 70–81. https://doi.org/10.5539/ijbm.v15n10p70
- Ali, U., Noor, M., Khurshid, M. K., & Mahmood, A. (2015). Impact of firm size on earnings management: A study of textile sector of Pakistan. SSRN Electronic Journal, 7(28), 47–56. https://doi.org/10.2139/ssrn.2698317
- Drábková, Z. (2015). Analysis of possibilities of detecting the manipulation of financial statements in terms of the IFRS and Czech accounting standards. Acta Universitatis Agriculturae et Silviculturae Mendelianae Brunensis, 63(6), 1859–1866 DOI:10.11118/201563061859.
- Dridi, J., & Nguyen, D. N. (2018). Assessing inflation convergence in the East African community. Journal of International Development, 31(2), 119–136. https://doi.org/10.1002/jid.3396
- European Investment Bank. (2016). Banking in sub-saharan Africa recent trends and digital financial inclusion (Luxembourg: Euopean Investment Bank).
- Feruleva, N., & Maria, S. (2017). Detecting financial statements fraud: The evidence from Russia ( pp. 32–35). Journal of Corporate Finance Research.
- Gan, S. O., & Ahmad, S. (2011). Multiple Linear Regression to Forecast Balance of Trade. Journal of Fundamental Sciences, 7 (2), 150–155 : http://jfs.ibnusina.utm.my/
- Gyawali, S. (2021). Does model reflect on reality? Exploring beneish m score on selected private commercial banks in Nepal. The Journal of Nepalese Business Studies, 14(1), 18–28. https://doi.org/10.3126/jnbs.v14i1.41485
- Haque, A., Mughal, A., & Zahid, Z. (2016). Earning management and the role of accounting conservatism at firm level. International Journal of Economics and Finance, 8(2), 197. https://doi.org/10.5539/ijef.v8n2p197
- Huang, W., Boateng, A., & Newman, A. (2016). Capital structure of Chinese listed SMEs: An agency theory perspective. Small Business Economics, 47(2), 535–550. https://doi.org/10.1007/s11187-016-9729-6
- Ismail, N. I., Zakaria, N. B., & Sata, F. H. A. (2015, April). Auditors roles towards the practice of earnings manipulation among the Malaysian public firms. Procedia Economics and Finance, 28, 145–150. https://doi.org/10.1016/S2212-5671(15)01093-X
- Katusiime, L. (2021). COVID 19 and bank profitability in low income countries: The case of Uganda. Journal of Risk and Financial Management, 14(12), 588. https://org/10.3390/jrfm14120588
- Khalaf, G., & Iguernane, M. (2016). Multicollinearity and a ridge parameter estimation approach. Journal of Modern Applied Statistical Methods, 15(2), 400–410. https://doi.org/10.22237/jmasm/1478002980
- Koufogiannakis, D., Slater, L., & Crumley, E. (2004). A content analysis of librarianship research. Journal of Information Science, 30(3), 227–239. https://doi.org/10.1177/0165551504044668
- Lotto, J. (2019). Evaluation of factors influencing bank operating efficiency in Tanzanian banking sector. Cogent Economics & Finance, 7(1), 1–14, 1664192 https://doi.org/10.1080/23322039.2019.1664192.
- Maccarthy, J. (2017). Using Altman Z-score and Beneish M-score models to detect financial fraud and corporate failure : A case study of Enron corporation. International Journal of Finance and Accounting, 6(6), 159–166. https://doi.org/10.5923/j.ijfa.20170606.01
- Mahama, M. (2015). Detecting corporate fraud and financial distress using the Atman and Beneish models. International Journal of Economics, Commerce and Management, 3(1), 1–18 http://ijecm.co.uk/wp-content/uploads/2015/01/3159.
- Makanile, D., & Pastory, D. (2022). Determinants of lending behaviour of commercial banks in Tanzania. International Journal of Research in Business and Social Science, 11(2), 260–269 https://doi.org/10.20525/ijrbs.v11i2.1638.
- Mathuva, D. M., & Nyangu, M. N. (2021a). Revisiting earnings quality and bank efficiency among East African developing economies: Do systemic banking and financial crises matter? Journal of Applied Accounting Research, ahead-of-print No. ahead-of- print. https://doi.org/10.1108/JAAR-10-2020-0219.
- Mathuva, D. M., & Nyangu, M. N. (2021b). Does banking regulatory regime affect the quality of bank earnings in the East African region? Journal of Accounting in Emerging Economies, 12(4), 2042-1168. . https://doi.org/10.1108/JAEE-12-2020-0325
- Mavengere, K. (2015 Predicting corporate bankruptcy and earnings manipulation using the Altman Z-score and Beneish M score. The case ofZ manufacturing firm in Zimbabwe.International Journal of Management Sciences and Business Research4 (10) , pp. 8–14 ).
- Mehta, A., & Bhavani, G. (2017). Application of forensic tools to detect fraud: the case of toshiba. Journal of Forensic and Investigative Accounting, 9(1), 1188–1197. https://doi.org/10.1111/j.1469-7610.2010.02280.x
- Muda, I., Maulana, W., Siregar, H. S., & Indra, N. (2018). The analysis of effects of good corporate governance on earnings management in Indonesia with panel data approach. Iranian Economic Review, 22(2), 599–625 doi:10.22059/IER.2018.66169.
- Najah, E. A., & Omar, T. (2018). The contribution of internal audit to the improvement of internal control system. European Scientific Journal, ESJ, 14(7), 200. https://doi.org/10.19044/esj.2018.v14n7p200
- Nikoomaram, H., Arabahmadi, F., & Arabahmadi, A. (2016). The relationship between earning management and capital structure International Journal of Finance and Managerial Accounting . 1(1), 51–56 https://ijfma.srbiau.ac.ir/article_9386.
- Nwofia, J. E. (2018). Contracting out services in the nigerian local government: implications for internal revenue generation. International Journal of Social Science Studies, 6(7), 71. https://doi.org/10.11114/ijsss.v6i7.3411
- Omar, N., Koya, R. K., Sanusi, Z. M., & Shafie, N. A. (2014). Financial statement fraud: A case examination using beneish model and ratio analysis. International Journal of Trade, Economics and Finance, 5(2), 184–186. https://doi.org/10.7763/IJTEF.2014.V5.367
- Osoro, A., Muturi, W. M., & Ngugi, P. K. (2015). Econometric data analysis affecting performance of supply chain systems in the petroleum industries in Kenya the. International Journal of Business & Management, 3(9), 98–114 https://www.iiste.org/Journals/index.php/IEL/article/view/25579.
- Palaniappan, G. (2017). Determinants of corporate financial performance relating to board characteristics of corporate governance in Indian manufacturing sector: An empirical study. European Journal of Management and Business Economics, 26(1), 67–85. https://doi.org/10.1108/EJMBE-07-2017-005
- Paolone, F., & Magazzino, C. (2014). Earnings manipulation among the main industrial sectors. Evidence from Italy. Economia Aziendale Online, 5(4), 253–261. https://doi.org/10.4485/ea203-5498.005.0022
- Pereira, A., & Alves, M. D. C. G. (2017). La manipulación contable y el Reglamento Europeo 1606/2002: Evidencias de empresas portuguesas no financieras que cotizan en Euronext. Revista de Contabilidad, 20(2), 107–117. https://doi.org/10.1016/j.rcsar.2017
- Ramírez-Orellana, A., Martínez-Romero, M. J., & Mariño-Garrido, T. (2017). Measuring fraud and earnings management by a case of study: Evidence from an international family business. European Journal of Family Business, 7(1–2), 41–53. https://doi.org/10.1016/j.ejfb.2017.10.001
- Razali, N. M., & Wah, Y. B. (2011). Power comparisons of Shapiro-Wilk, Kolmogorov- Smirnov, Lilliefors and Anderson-Darling tests. Journal of Statistical Modeling and Analytics, 2(1), 21–33 https://www.researchgate.net/publication/267205556_Power_Comparisons_of_Shapiro-Wilk_Kolmogorov-Smirnov_Lilliefors_and_Anderson-Darling_Tests.
- Roy, C., & Debnath, P. (2015). Earnings management practices in financial reporting of public enterprises in India: An empirical test with M-score. SSRN Electronic Journal. https://doi.org/10.2139/ssrn.2551713
- Sari, R. P., & Sari, R. P. (2019). Study of earning management and audit quality in Indonesia. Journal of Economics, Business, and Government Challenges, 1(2), 79–88. https://doi.org/10.33005/ebgc.v1i2.23
- Shahzad, A. (2016). Detecting earning management and earning manipulation in BRIC countries; a panel data analysis for post global financial crisis period. International Journal of Accounting Research, 4(1), 1–10. https://doi.org/10.4172/2472-114X.1000134
- Shanwari, I., & Shah, S. M. A. (2015). Detecting earning management: deferred Taxes vs Accruals: A Pakistani perspective. Journal of Accounting and Finance in Emerging Economies, 1(2), 111. https://doi.org/10.26710/jafee.v1i2.68
- Sujeewa, G. M. M., & Kawshalya, M. D. P. (2020). Detecting red flags of corporate financial statement frauds using Beneish M score model in Sri Lanka. International Journal of Accounting & Business Finance, 6(2), 71–81. https://doi.org/10.4038/ijabf.v6i2.72
- Talab, H., Hammood, H., & Ali, S. I. (2017). Role of Beneish m-score model in detecting of earnings management practices: Empirical study in listed banks of iraqi stock exchange. International Journal of Applied Business and Economic Research, 15(23), 287–302 www.serialsjournals.com.
- Talab, H. R., Mohammed, Y. N., & Flayyih, H. H. (2018). Measurement of earning management practices of listed companies in iraqi stock exchange: A case of kothari model analysis. Third international conference on: “social and natural resources-global challenge 2018,” 278–286. http://weekly.cnbnews.com/news/article.html?no=124000