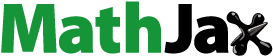
Abstract
The current investigation explained the dissemination of long-term share yields grounded on the historic record of share marketplace outcome in an extensive cross section of 39 developing and developed nations in time span from 1841 to 2021. Our wide-ranging data set sample mitigates apprehensions over survivor and informal data biases that outbreak other work in this range. A bootstrap simulation analysis suggests considerable ambiguity about long-horizon share market effects, and we approximate a 12% probability that a diversified stockholder with a 30-year venture time horizon will lose comparative to inflation. The findings oppose the predictable guidance that shares are safe investment avenue over long holding time periods.
1. Introduction
Capitalising in corporate shares has proven to be a lucrative choice for enduring shareholders. The historic share premium in the United States of America is relatively huge (Mehra & Prescott, Citation1985). According to conformist, perception shares are secure over longer holding times, and long-term damage comprehensions in the United States of America have been occasional or non-existent (Siegel, Citation2014). A study also approximated a small likelihood of damage and a high likelihood of extensive improvement for shareholders with prospects of 20 or 30 years. Based on these data, communal asset information is that shareholders with long prospects should capitalise heavily in shares. An apprehended loss over an extended speculation time period, though, is predominantly detrimental to a shareholder since it uses much of its feasible redeemable time period without creating prosperity.
While the United States historical data are supportive, there is motive to be careful while evaluating shortcomings of hazard. In this regard, frequently used illustration from the Centre for the Research in Safety Prices extends fewer than 100 years, which suggests inadequate arithmetic data around what occurs over 30-year prospects. Encompassing the United States of America, illustration backward only develops apprehensions nearby survivor bias (Brown et al., Citation1995). In addition to it, confirmation of unforeseen rise in share estimation proposes that the past outcome from the United States may be hopeful comparative to other prospects (Avdis & Wachter, Citation2017). At the close of 1989, Japanese share market was the major globally in terms of collective marketplace capitalization. Moreover, from 1990 to 2021, a differentiated speculation in Japan shares generates yields of −9% in nominal expressions and −21% in real expressions. Japanese practise is not exceptional, and numerous industrialised republics have understood poorer performance or even whole stock marketplace catastrophe (see, e.g., Jorion & Goetzmann, Citation1999). Given the unadorned significances of long-lasting poor outcome, we pursue measureable suggestion on downside risk.
The present study investigated the dispersal of long-horizon stock marketplace proceeds. Our examination sums across an extensive data set of developing markets with the aims of exploiting information about developing economies’ earnings and decreasing probable preconceptions. We employed the historic record of share marketplace outcome to assess the dissemination of purchase and hold returns for an agreed venture prospect. For the purpose of the examination, we employed a bootstrap simulation method that re-samples from historic data set to yield inducements of cumulative purchase and hold returns. We employed an extensive sample of 39 developing countries over extended historic time periods that collectively cover nearly 32,000 months (i.e., nearly 2700 years) of data about stock returns inaugurating in 1841 and extending to 2021. This data set offered us with a considerably wider opinion of hazard over long time spans in comparison to US-centric scheme.
In the present study, the development process of a sample alleviates two possible preconceptions that mark implications about predictable outcome and hazards. First, Dimson et al. (Citation2002) defined an informal data bias, where constant share return information set from fruitful marketplace is more enthusiastically accessible. Our sample set achieves considerably better reporting of developing economies’ time periods in contrast to prior studies to curtail this predisposition. Second, Brown et al. (Citation1995) deliberated survivor prejudice, in which training on ultimate marketplace consequence data series with no disturbances produces an upward bias in presentation relative to ex ante prospects. We contest survivor predisposition with our arrangement of developing economies and our action of return information set. Our developing economies’ arrangement is grounded on data that were accessible at the period and does not state on ultimate consequences. Earlier than 1948, nations come in our developing sample when their agricultural labour shares decline below 50%, drawing on proof about labor designs from the economics literature (Kuznets, Citation1973). The United States, e.g., come into this foundation in 1890. After 1948, we use association in the Organisation for Economic Co-operation and Development (OECD) and its European predecessor, the Organisation for European Economic Co-operation (OEEC).
Inside the developing economy share marketplace, we cautiously manage with market distractions and catastrophes. The share interactions in numerous republics provisionally locked during our sample, and the share marketplace enduringly vanished in Czechoslovakia. In this research, the return data set replicates stockholder understandings to reduce survivor preconception.
The present study produced bootstrap disseminations of collective yields and prosperity at time period stretching from 1 month to 30 years. Our immobile block bootstrap scheme permits for topographies of earnings that are significant for acquire and hold shareholders, with mean return and instability determination, and then also transmits the benefit that we endure moderately uncertain around the fundamental arrangement of the return information set. Both nominal and real yields were studied, but the current study emphasized on real yields because they well imitate the investor understanding, assuming a limited time period of increased inflation in the data set.
The wealth altitudes reveal the consequence of capitalizing $1 with three decades of acquisition and hold strategy. We notice that this examination utilizes real yields calculated in local money like the industrialised nation dispersal is enlightening about a home-based country share marketplace speculation in any developing country. We signify wealth in dollars solitary for the suitability of stipulating a money when we deliberate wealth intensities both wealth dispersals. The predictable wealth intensities are also comparable at $7.37 for developing nations and $8.92 for the United States. The disseminations fluctuate meaningfully, nevertheless, when we associate the left-hand extremities. Steady with the outcomes in previous works, the dispersal grounded exclusively on US information which specifies a small 1.2% likelihood of harm in procurement power over a three-decade prospect. The complete model dispersal reveals a much-advanced likelihood of loss at 12.1%. Furthermore, the 1st and 5th percentiles for developing nation’s long-term prosperity are only representing that tragic share market presentation cannot be governed out for long-term share marketplace stockholders.
Suggestions from the developing nations agree a substantial threat of loss for lasting shareholders. The dispersal suggests that the −21.5% real yield understanding in Japan over the historical three decades is not remarkably rare. In fact, this opinion lies in the 9th percentile of the wealth distribution. While this information proposes that Japanese shareholders were unsuccessful, their preparation seems to be a deliberation of the considerable risk exposures of long-term equity depositors. A depositor who acquires about the delivery of three decades of earnings using only the United States experience, in comparison, would allocate a likelihood of just 0.50% that a return as exciting as the Japanese yield realization could occur. The wealth of similar instances recommends that the US dissemination is excessively hopeful with esteem to loss prospects.
Our outcomes of increased harm likelihoods for industrialised country marketplaces comparative to the US involvement are not determined by early phases, small nations, or small stock markets. Specifically, loss prospects remain elevated as we differ the preliminary year of the illustration between 1841 and 2000 and eliminate nations with comparatively small populaces and due to inferior ratios of marketplace capitalization to GDP. Our consequences are also vigorous to another bootstrap expectations and to calculating real returns in USD in spite of local money. In all stipulations, our examination proposes that stockholders are wide open to considerable risk of damage once we excuse for data from the larger illustration of industrialized countries.
To examine the economic inferences of findings, we employed a modest collection optimal submission. The stockholders enhance predictable usefulness by assigning transversely shares and a risk-free advantage. We bargain that shareholders using the emerging country sample are significantly less hostile while capitalizing in shares. Stockholders depending on the developing economy dispersal would prefer to pay carefully augmented cost of up to 1.0% per annum to continue their optimum weightiness rather than embrace the comparatively higher weight in shares obscure by the United States analysis. The current study adds to an enormous works in asset valuing that appraises experimental wonders among global strength marketplaces and overlarge data set times. The utmost persuasive effort in this research emphasizes financial queries for the purpose of which outdated US statistics set miscarry to produce conclusive responses. In numerous circumstances, these researches are inspired by arithmetic apprehensions like survivor and informal information preferences (Rietz, Citation1988) and small supremacy of econometric investigations to classify noteworthy associations over small period series. For instance, Barro (Citation2006), Barro and Ursúa (Citation2008, Citation2012, Citation2017), Barro and Jin (Citation2011), and Nakamura et al. (Citation2013) inspect the asset appraising inferences of occasional macro-economic measures utilizing data set of consumption and GDP catastrophes in a comprehensive cross segment of republics. Lundblad (Citation2007) reflects the certainty of the marketplace hazard premium with lagged expected share marketplace instability by means of a very long period sequence, and Goetzmann and Jorion (Citation1995) and Golez and Koudijs (Citation2018) implement an analogous methodology in their research on dividend output probability. The writings on the cross segment of share yields contain frequent instances of studies that prolong the current confirmation based on US data set to world-wide share arcades (e.g., Asness et al., Citation2013; Fama & French, Citation1998) and to extended data times. (e.g., Linnainmaa & Roberts, Citation2018; McLean & Pontiff, Citation2015; Schwert, Citation2003). A great capacity of previous work also inspects regular share marketplace yields and shareholder premiums across nations (e.g., Dimson et al., Citation2002; Jorion & Goetzmann, Citation1999). Our themes—the dispersal of returns and the probability of loss over long prospects—are compatible for an expanded sample investigation. The present work in this capacity (Fama & French, Citation2018a, Citation2018b) is grounded on the past US involvement and offers a convenient first phase, but the outcomes suffer from the survivor and informal data set. Our larger panel of past returns improves these apprehensions and provides an additional consistent description of tail results, which are of specific interest for enduring stakeholders. Additionally, the dispersal of long time period returns in developing marketplace is a theme in prerequisite of measureable indication.
2. Literature review
The study defined as primary research for stock investment in 39 countries (developing and developed nations) from pre-World War I & II and after it. According to Mayer (Citation1988), even massive stock markets are minor sources of corporate capital. According to Joseph E. Stiglitz (Citation1985) and J. E. Stiglitz (Citation1994), stock market liquidity will not boost incentives for acquiring information about firms or practising corporate governance. Devereux and Smith (Citation1994) also point out that increasing risk sharing via internationally networked stock markets might depress savings rates and stymie economic expansion.
Finally, Andrei Shleifer and Summers (Citation1988) and Randal et al. (Citation1990a, Citation1990b) argue that expanding the stock market might hinder economic development by making inefficient corporate takeovers easier. According to Greenwood and Smith (Citation1997), huge stock markets are facilitating investments in highly productive technology fields. They further argued that facility providers are providing these services at a lesser cost. Because huge stock market enterprises have a large number of consumers, their returns are comparable to or even higher than those who invest small amounts of money in the stock market. It is also true that money investors want control in some way, but in order to keep them in the stock market, numerous stock exchanges provide investors with liquid equity markets (Naheed et al., Citation2021).
Their assets become considerably safer and less susceptible here, as well as more appealing. Simply put, investors may sell their shares whenever they choose. Stock markets, according to Cottle et al. (Citation1962), are critical because they can offer a continuous and liquid market for the exchange of outstanding and issued securities. Stock retuning, according to Tobin (Citation1969), is a key connection between the actual and financial elements of economic growth. The stock market, according to Romer (Citation1993), Boyle and Peterson (Citation1995), Malliaropulos (Citation1996), and Chami et al. (Citation2006), is a critical channel for monetary policy transmission that influences economic activity. They show that the influence of inflation rates on family asset holdings can be a route for this mechanism through stock markets. Levine (Citation1991), Bencivenga and Smith (Citation1991), Diamond (Citation1996), and Fulghieri and Rovelli (Citation1998) made the most significant contributions to the theoretical literature on stock market development and economic growth, arguing that the stock market is necessary for economic growth and that stock market liquidity is necessary for economic growth. In a somewhat different vein, Levine (Citation1996) performed an empirical analysis on 38 instances from industrialised and developing nations and concluded that increased liquidity in stock markets might impact economic activity. There is a substantial amount of research on the relationship between stock market development and economic growth (Jawad et al., Citation2021).
There is a substantial amount of research on the relationship between stock market development and economic growth. The majority of research show a positive correlation between the two factors (see, for instance, Akinlo & Akinlo, Citation2009; Beck & Levine, Citation2004; Chen et al., Citation1986; Fama, Citation1990, Citation1981; Geske & Roll, Citation1983; Harris, Citation1997; Levine & Zervos, Citation1998; Mukherjee & Naka, Citation1995). The results of Sabra and Eltalla’s study on the effects of stock investment on economic growth are particularly positive—that is, increases in domestic investment have aided the country’s economic growth. On the other hand, several additional research have shown a negative association between the factors (see, for example, Bhide, Citation1993; J. E. Stiglitz, Citation1994; Garcia & Lin, Citation1999; Hassapis & Kalyvitis, Citation2002; Joseph E. Stiglitz, Citation1985; A. Shleifer & Vishny, Citation1986). Other research has found a bidirectional relationship between stock market development and economic growth (Deb & Mukherjee, Citation2008; El-Wassal, Citation2005; Enisan & Olufisayo, Citation2009; Nishat & Saghir, Citation1991; Shahbaz et al., Citation2008). SMD has a deleterious effect on EG, according to Pan and Mishra (Citation2017).
The purpose of this paper is to examine the causal link between stock market development and economic growth in developing and developed economies. The objective in our investigation is pertinent for a widespread variety of hypothetical subjects, including optimum assortment excellent and prosperity administration, prime consumption and investment behaviour in lifespan prototypes, and the valuation of macro-economic prototypes of asset values. Furthermore, the objective also has strategy connotations for the scheme of annuity structures (see, e.g., Poterba et al., Citation2007; Shiller, Citation2003, Citation2006) and the structure of target-date mutual reserves (see, e.g., Pástor & Stambaugh, Citation2012; Viceira, Citation2008).
3. Data and methodology
The primary data of the study are related to the country-level stock market index returns. We make these return series by utilizing the GF Database from the Global Financial Data, which is also known as GFD. The aim is to deliver the returns for the widest accessible index of the stock market in every country as far as simple period and stock inclusion both to best address an investor’s performance. The underlying sample approach represents an investor’s viewpoint that invests in her homeland’s stock market over a predetermined horizon and lives in a developed country.
We examine both nominal and real stock market indexes on returns in this end in advanced countries. Returns reflect the viewpoint of a native investor who can avoid extra risk of foreign exchange that is dominant in local currency. A viewpoint of global USD investors considers another sample formation. In this situation, we measure real and adjust global stock market index return performance in USD to change the exchange rate over an investment period. We also observe data from advanced countries. The return of these periods is instructive but expanded investments of certain countries and horizons that have been hard to succeed for the foreign investors.
In a broader sense, the GF Database gives data directly from authentic source documents or that gives the data of various indexes which are calculated and created by the stock exchange (the Tokyo Stock Price Index from the Tokyo Stock Exchange) or by known index given (the S&P 500 Index). The state-level index data reflects the investor experience in a way that accounts for the changes in capital (exchange offers, dividends of cash, managers, subscription rights, stock dividends and splits) in consensus with industry standards. The unique characteristic to make a sample approach, which we particularly consider a stock market return, is comprehensive dividends. We emphasize on the performance of cumulative stock over a long period, with an effect of a dividend income that is essential for precisely evaluating the investment results. However, the dividend income impact on a return mean and variance for a single month is not that much and such impact rises with the holding period. Exclusion of dividends would drive a descending dividend yield and shows that biased performance appraisal significantly influences the performance to a long horizon (see, e.g., Dimson et al., Citation2002). Moreover, the dividends permit us to measure the risk over a long period so the uncertainty related to dividend income provides a wide return ratio of variance when the investment period rises (Avramov et al., Citation2018). Our collective return distribution expects that the dividends are again invested, which is usually considered a default choice to save for vehicles in a long term for 401 (k) accounts.
We take precautions to avoid unnecessary problems while developing our sample of two potential biases. The first bias data explained by Dimson et al. (Citation2002) described the studies’ enriched material to observe return data which are freely accessible and approachable, such as the data for the UK or US. Data, which are accessible, are an endogenous outcome so the issue with these returns is not illustrative for the larger sample. We include many countries with larger historical periods and make samples of advanced market returns which can alleviate the easy data bias. Secondly, Brown et al. (Citation1995) explained survivor bias that describes conditions applying to the outcomes of the stock market when developing the sample. While overcoming the problem, first we classify a period when countries formed metrics that were easily accessible to investors instead of the situation where economies are formally established. We try to observe investor’s historical experiences of stock markets which had substantial disturbance or the country’s market that eventually died.
The theory of endogenous growth links the price level to the marginal product of capital and therefore the rate of economic growth. That is, changes in the price level can impact economic growth. Obversely, policies that raise growth (for instance, the expansionary fiscal and monetary policies) have the potential to raise prices. It may be fair to state that the long-run relation between stock investment and output growth is backward-bending (Billmeier and Massa, 2007; Vaona, Citation2012).
4. Final data and return
Global Financial Data are the fundamental source for our study. The database consists of macroeconomic data and historical period data for the stock market with developed economies. The relevant data permit a big sample of developed countries in terms of their historical stock return, which can be compared with past studies in the distribution of long period stock return. From the database, we make five variables to construct the returns; these variables are (a) Price indexes that evaluate capital gains, (b) The total return indexes which depict both dividends and price changes, (c) Exchange rates of local currency in relation to the United States of America currency, (d) Price indexes of consumers, and (e) The dividend yields at index level. The characteristics to segregate developed and developing economies are (a) income per capita, (b) current account, and (c) unemployment rate.
We choose an individual index with the consideration of the time span of historical period and also breadth of market spread for a given country that consists of multiple price indexes or total return indexes from the database. The indexes are broadly available in the Internet Appendix. We use price index or total return blend and dividend yield index. The nominal return is reflected in the monthly percentage change in a total return index.
We unite data from dividend yields and price indexes to construct returns, which are inclusive of dividends, for a period of zero total return data. The described dividend yields depict dividends that paid on the indexes over the preceding 12 months.
By merging the capital gain that deduced from the dividend payments and price index, the nominal return for each month can be measured. Namely, gross nominal return for the month of December is (i.e., τ = 0)
As shown, return in the equation that outlines the real investment performance of an investor in a country “i”. From the outlook of an investor of global USD, we also review the outcomes of real investment of a person who spends in a native market that talks about the beginning and closing period between local currency and the US dollars.
5. Categorization of economies
The category was made for a country by using knowledge that is probably available to investors about the financial and economic market development in real time. Our definition of economic development centred on economic prosperity including technological boom and industrialization advancement in pre-1948 time and to be officially part of any economic institution in post-1948 time. Broadly, such a country needs a strong economy with the presence of a stock market for sample formation. The model contains 39 countries with 40 developed horizons of stock market return. Developing and developed countries are shown in , as our analysis for each country’s sample depends on the data availability, start & end dates, and development.
Table 1. Observation from developed and developing economies
6. Financial data properties
Our developed country sample shows many challenges during the formation of return series. Market disturbance produced by wars, revolutions, and economic crises affects the availability of data (Dimson et al., Citation2002; Brown et al., Citation1995). The dotted black bars show a developed period with unenviable data, whereas these dark blue lines show data coverage. we have not had unintended spaces in the mid or closure of developed country horizon. In case of data accessibility issue with on and off wars and economic crisis, we observe that this feature in our sample is significant to reducing the data. However, the early historical stock return records of few countries are inaccessible, which we discuss in detail below.
Historical return data of month wise are available in our sample. Five scenarios make exceptions. We briefly instigate the classifications and then explain the approaches to each scenario. We also give complete information on such data items in a section of Internet Appendix. Firstly, in our sample, many countries restricted trading or closed the stock exchanges for an extended time. Secondly, our country’s stock exchange opens with small gaps in dividend yield data or in price that often occur in the middle part of our sample; thus, these challenges emerge during prime historical occasions. Thirdly, from the view of investors, Germany outlines the return observations in a period of hyperinflation that are not significant. Fourthly, the closing prices do not depict the truth of investor outcomes, and Czechoslovakian stock exchange was audibly discontinued. Lastly, the historical data of some countries are unavailable in earlier periods.
describes our developed sample for the countries with periods of heavily restricted trading or stock exchange closures. We report beginning to end dates of the period for each event, number of months spent disruption in the horizon, real return (evaluated in local and US currency) that was noticed when exchange is returned to normal business, and nominal return. The groups in break the occurrence of events into WWI (Group A), WWII (Group B), Political Instability in a country (Group C), on and off Economic crises (Group D), and lastly Labour Protests (Group E).
Table 2. Returns Data
In the history of the United States of America, there is only one prominent disruption we have seen that affected access to month-wise details during the time of WWI. From July 31 to December 1914, NYSE was not fully functional. The New Street black market appeared within the eight working days of NYSE cessation to ease stock business (Silber, Citation2005). GF Database holds return information from the New Street Exchange at that time of New York Stock Exchange cessation (also black-market information of some other countries). Silber (Citation2005) explained in his report that the New Street market spread was significantly less related to NYSE. However, most of the investors had no peaceful entrance to their black market. Majority of people on 30 July 1914 carried stocks that would have preserved their situations till the trade restart in the month of December.
During WWII, the German market also gave the example of market disturbance. As Germany gained victories, the Stock prices rose, but with the defeat at Stalingrad, in January 1943, Germany stuck its stock prices. Even though the exchange market was open, trading was on a limited scale. After the Allied force invasion, the stock market was eventually closed. In July 1948, when the prices again permitted to float, the stock market dropped.
We treat each event as a single return observation to cover multiple time horizons in to get our empirical analysis. Heavily restricted trading or exchange cessation classifies every event. Our treatment depicts that the majority of the investors would have not been able to trade rather than to stand for the time of realization for a long time to carry returns.
We utilize dividend yield knowledge to make a systematic bias and to avoid dropping such periods for such countries in existing horizons to build hypotheses related to dividends in the time of lost horizons. The gentle returns over lost months due to such missing returns seem exclusively related to data in these cases. While making these changes, this permits us to undisrupted return range for every progressed country horizon.
Model includes an exception to practicing of measuring the nominal returns that demonstrates in local currency. With the start of WWI in 1914, Germany surrendered as a gold standard and moved “Gold stamp” to “Paper stamp” being the main currency.
We lack adequate records on dividends and prices in each country with a missing horizon so that we are not able to find out monthly returns inclusive of dividends. It is crucial to study potential effects as missing returns in some developed periods show the spaces in our sample. Brown et al. (Citation1995) and Dimson et al. (Citation2002) elaborated that countries’ return data are more analytically accessible through improved financial results. We initially observe missing data of 14 countries and conclude that they incline to have a bit greater end-of-sample development, as evaluated through real per capita GDP, than with countries having a complete record sample. An outline shows that most of the countries of missing data are not so big such as Singapore, Iceland and Luxembourg. As compared to countries of missing data according to the 2021 populace, countries with complete range are around three times bigger on normal. However, the exact effect of missing returns on our outcomes is unknown, and also size-related under-sampling looks less disruptive than performance-related understanding. With the comparison of Fama and French (Citation2018a) study, they cover 1.7% of US return data by using 642 months (about 53 years) of complete development period. Comprehensively, our big sample consists of valuable knowledge almost long-period returns.
7. Data attributes
Our return sample summary statistics is shown in . The statistics in Group A is dependent on nominal return, that of Group B is dependent on real return, and that of in Group C is dependent on real USD returns. In , the number of observations and number of months may vary for assumed country due to multiple month periods. We mention the geometric mean (R¯g) and arithmetic mean (R¯a) for higher-order return moments along with returns. In the sample, both geometric means and arithmetic means report the number of months somewhat to number of readings. The upper moment treats every reading uniformly.
Table 3. Summary Data
Furthermore, we express the minimum and maximum return observations as well. Finally, every group has a “complete sample” case that is pooled across all developed periods.
We notice that (a) cross-country comparisons are somehow difficult in a setting due to difference of sample periods and (b) newly developed countries have small samples that do not represent long-term expectations.
The nominal returns in Group A () frequently show extreme behaviour. The arithmetic (geometric) mean return is a bit higher than the US average monthly pooled sample. However, the skewness and kurtosis of the complete model show that the statistics comprises outliers. In , the returns of Japan and Austria are listed as their markets were closed during WWII, and both countries accompanied by negative real returns. The worst nominal returns in the sample turn out in Portugal return during Carnation Revolution that started in 1974, Germany in the consequences of WWII, Iceland with a return in October 2008, and Czechoslovakia with our estimated return that accompanies the official discontinuation and the seizure of assets of its stock market.
The real return in Group B () gives further insight into the investor’s knowledge related to the nominal returns in Group A. The arithmetic (geometric) mean of the pooled model of real return in such a way equity returns considerably exceed inflation on average. The US is not an outlier related to other countries but has an approximately higher average performance with a complete sample. The observation is more alike with the results of Dimson et al. (Citation2002) instead of Jorion and Goetzmann’s (Citation1999) findings who say that the high equity premium in the US “appears to be the exception rather than the rule”.
As compared to nominal returns, the real returns have slightly lower skewness and kurtosis and lower standard deviation. Furthermore, the minimum and maximum real returns in Group B frequently vary significantly from nominal return counterparts in Group A. High currency devaluation in Japan contributed to inflation with the best nominal return reading corresponding to its worst real return at the end of WWII.
The real US dollar returns in Group C () show the real investment performance from spending in the native market in the US currency. The findings are widely similar according to economic views, which are presented in Group B. The pooled model of real US dollar returns express a bit greater arithmetic mean, comparatively to real local currency return and an identical geometric mean. For each individual country, the standard deviation of real US dollar return is greater relative to the standard deviation of real native currency returns. This finding is anticipated; thus, the real US dollar return includes foreign exchange hazard.
The consideration for inflation is essential to better understand the long-term stock return, which shows in a summary statistic in . Investors no doubt do care about their purchasing power of savings. Our observed inquiry depends on real US dollar returns and real returns as given large inflation effects in our sample. We show findings depending upon the nominal returns in the Internet Appendix.
8. Dynamic model specification
The prime investigation relates to the distribution of buy-and-hold in the stock market return at long term. Broadly speaking, this part presents the bootstrap simulation method that estimates such distributions and draws 32,930 observations with a horizon of H month to make a series of returns.
We measure wealth level returns and cumulative returns that are given in return sequence; we repeat this process of these variables of interest to make bootstrap distributions. The method permits us to observe the range of dependencies that also affect investors of stock market return to account time-series properties. The most significant fact for long term which is often referred to as mean reversion in the literature is negative serial correlation return for investors (Barberis, Citation2000; Campbell & Viceira, Citation2002; Poterba & Summers, Citation1988; Siegel, Citation2014). The mean reversion shows in subsequent years for the feature of returns to a high positive return in the present month that influence to be comparatively low returns. The expected return can vary with time and thus this pattern shows a variation in the observation (Campbell & Shiller, Citation1988). However, negative magnitude shows the small value of correlation but overall gives a large economic impact. Mean reversion makes more foundation of an argument that young people should spend more in stock relative to aged people. Thus, market returns for the long term are more secure.
The extended features of market returns perform for smaller horizons to produce fatter tails and also to increase variance, whereas the mean reversion feature keeps the variance less and acts over a long time. Short-term autocorrection in nominal returns affects cumulative returns over a short horizon that is a bit riskier, which leads to become positive (Lo & MacKinlay, Citation1988). Short period auto-correction in real returns may contribute to measured persistence inflation. Risk in short time cumulative returns does have a large effect in the return variance constantly (Engle, Citation1982). The range of dependencies can influence buy-and-hold investors, but these patterns may not make a broader list.
To consider the range of dependencies in a stock return, we followed the block bootstrap approach. The advantage of using bootstrap method is that it does not need any specific range of dependencies of desired various and market return; on the other hand, alternative approaches have long-term risk to understand vector autoregression (Avramov et al., Citation2018; Pástor & Stambaugh, Citation2012). Return blocks depicted the formation of market return. Many finance-related literature including Brown and Warner (Citation1980, Citation1985), Sullivan et al. (Citation1999), Kosowski et al. (Citation2006), Patton and Timmermann (Citation2010), and Martin and Wagner (Citation2019) follow the method of block bootstrap to consider the range of dependencies in a market return.
The foundation of the model depends on the studies of Politis and Romano (Citation1994) to use stationary bootstrap. Average block length controls the parameter and picks the size of blocks randomly. The stationary bootstrap determines a block, which starts from the end range, also that could not fill details, and also wraps again to starting the range to fill in a block and to avoid undersampling from any piece of sample. We make return series, which fill the 360 months of stock returns; thus, the 30-year horizon is our longest period. The method to producing bootstrap market return m shows as follows:
With a parameter of probability, random block size “b” is made from a geometric distribution that equals an inverse of a block length of expected mean.
In a sample, the return block draw begins with arbitrarily selected readings from 31,465 observations. We give this observation within a country as Ri,t, where i represents countries and t represents readings. If a country “i” sample holds return observations Ri,t from Ri,t + b-1 which comprise individual-month market returns, stock return block also drawn as Bb = {Ri,t, Ri,t + 1, …, Ri,t + b − 1}. Expectations happen If the sample of a country ends before observation t + b-1 or when series of country “i” in stock returns involved in multiple months analysis.
b used as a block size for number of months instead of number of observations. From , when single observation of Ri,t from Ri,t + b-1 is multiple month market return investigation, number of months covered by the {Ri,t, Ri,t + 1, …, Ri,t + b − 1}, which is greater than b. By observing a lowest no. b* so as b s = * 0 Ni,t + s ≥ b in this case, where Ni,t + s reflects number of months covered at Ri,t + s (such that, Ni,t + s = 1 despite many observations & Ni,t + s = 5, from closure of WW1 of five months). Bb = {Ri,t, Ri,t + 1, …, Ri,t + b*} reflects return block draw. Bb could be covered more time as compared to b months when Ri,t + b* reflects as various month observation.
When we examine the last period of a country i as Ri, T & T s = 0 t Ni,t + s < b, then {Ri,t, Ri,t + 1, …, Ri,T} could not be sufficient for filling up a block Bb. From the 40 developed periods, we draw random country j in this case which is discussed in Section 2.2, and for the block, use the starting of its sample. The bootstrap assured the beginning from the country j’s sample. Suppose the sample of country “j” has sufficient details for filling up a block (such as, j span details in a country minimum b—T s = −0 t Ni,t + s months), block as Bb = {Ri,t, Ri,t + 1, …, Ri,T, R j,1, R j,2, …, R j,b*}, where b* shows as a lowest no. so T s = −0 t Ni,t + s + b s = * 1 Nj,s ≥ b. In a country j, assume that the details do not cover sufficient months, then we again do the same procedure until the block is filled to make a country randomly. To avert any serial dependencies across periods, we utilize data from random countries where nothing actually exists. If we simply heap alphabetically the country’s data, such as the Economic uncertainty (2008) unstable Iceland financially resulted the war with India at the period of 1936 to 1938.
3) By adding Bb to bootstrap stock return vector R(m), then go back to stage 1 and again do the same procedure till R(m) holds at minimum 360 return examinations.
Finally, bootstrap in repetition m as R(m) = {R1(m), R2(m), …, R360 (m)}.
For every horizon H, we stated the bootstrap return vector R(m), while noticing the lowest number H* so that H s = * 1 Ns(m)≥H, where Ns(m) reflects number of months covered as Rs(m). Returns might cover more to H months by assuming last observation RH(m*) is a multiple month observation. This scenario is a situation of a person who comes to the closing period at a horizon where he might be waiting to open the stock market again before selling his shares and the exchange is closed.
Furthermore, bootstrap captures short-term effects such as constant volatility and positive auto-correction. However, small blocks lead to loss of long-term mean rotation. From the data by using the large blocks of returns, observations permit our cumulative returns to be reflected in long-run serial correlation. We describe these effects in the Internet Appendix by utilizing the readings of return besides various block size distributions. Over a long period, given the priority of mean reversion for cumulative buy-and-hold returns, we select the length of a base case that finds 120 months of an average block size.
9. Distribution of stock market return
We show findings in this section of long-run stock market return from bootstrap simulations. Our fundamental case of empirical design centers in that depends on developed stock markets to remodel from complete models of the returns. Findings of substitute model have shown in Section 4.2. We evaluate the quantitative effect of survivor biases and model data by analysing the base case findings from models conditioned on eventual economic conclusions. We measured the effect from countries comparatively having not too much population, the effect of excluding data at model horizon, or the countries having not large economies.
10. Initial point model
Real distribution of payoffs over 1,000,000 bootstrap readings is summed up in . Simulation depends on a complete model, as presented in Section 3, of developed country returns over the horizon from January 1841 to 2021. We show findings for the period of 1 month, 1 year, 5 years, 10 years, 20 years and lastly 30 years. Every simulation time engages resampling with replacement utilizing the method of stationary block bootstrap from a pooled sample at monthly returns, where the length of every block has a mean of 120 months with geometric distribution.
Table 4. Bootstrap Data
Group A shows mean and standard deviation of real payoffs for every period in . The volatility of the payoff and average payoff present pronounced period effects as expected. The skewness of unreported payoffs with periods also increases. Whereas the average payoffs significantly increased the median payoffs in long periods, the corresponding median values are close to average 1 month and 1 year payoffs. Noticing the right tail features and at 5-year period of each distribution, depicting an extreme positive cumulative return at the high probability. Generally, the financial experts claim the power of compound interest that also shows upside stock potential of these payoffs over long holding periods.
The most significant characteristic of the findings in Group A () shows ambiguity in real investment results of long periods that are faced by investors. This alteration has considerable implications in investment results for asset allocation decision, savings, and consumption in long-run investors. Depending on previous literature that the US has the strong aggregate mean revision in its financial stock (Poterba & Summers, Citation1988; Siegel, Citation2014), it shows that the outcomes in Group A () seem that the financial market is secure for young people. The first percentile of real payoff produces $0.14 to disastrous stock, which results in 30-year investors. Furthermore, the tenth percentile, which is $0.85, produces great loss in the economy.
Over a given period, investors feel the risk of loss in investing in the financial market. The percentage of simulation runs shows in the last column of in which the terminal payoff is less than $1.00. Based on the study by Fama and French (Citation2018a), they evaluated similar facts on the US stock distribution of nominal payoff. They conclude that at a period beyond 20 years, the probability to lose money taken by the stock market is negligible.
At a short period, the probability of experiencing a loss is large as presented in Group A in . However, from the stock market, investments in 20 and 30 years of probabilities are still facing fall. These outcomes differ with those of Fama and French’s (Citation2018a) study and reverse at long periods that the stocks are safe investment. Our conclusions represent the significance of protecting biases, which are associated with simple and survival details in classifying a risk, which people face in the long run. Though most developed markets in the world are for long periods, investors experience substantial risk of loss.
Gaining market payoff exposure in Group A () does not add any impact on fees, cost and profit percentage. Practically, for long-run investors, the cumulative outcome of fees is a significant consideration. To present this impact, we review the perspective of the present-day investors who can use the bootstrap method, which helps to estimate stock market funds.
The findings advise that the loss probabilities and mean investment results could charge differently in vehicle investment at less-fee vs. more-fee. We also classify in the Internet Appendix at various investment periods that bootstrap as compound return. Fama and French (Citation2018a) focus beyond 10 years from the closure of US to normal periods for the distribution of continuously compounded return in a way that the distribution of this is considered as lognormal. From the perspective of the developed country model, we study this challenge and find that in long periods of return the distribution is not normal. Cumulative log returns show excess kurtosis and negative skewness even at a 30-year period. These outcomes review for the long-run investors that associated downside risk and the pronounced left tail distribution.
Group B () again does examine Group A of returns in US dollar payoffs for the comparison. That method views the perspective of an international investor in respect of nominal return, which is adjusted for the local currency for both exchange rate changes in relation to US inflation and US dollar. Each result from Group A depends on local money returns that are pursued to carry Group B.
At each, same as in Group A and B, a higher standard deviation produces the exchange rate risk considered in Group B of the payoff distribution. This growing dispersion accompanies more extreme tail findings, such as the 30-year real US dollar payoffs of 5th and 95th percentiles.
Our findings for international investors who have the exposure in currency rates are more hypothetically strong. This section of the study highlights the real local money returns to preserve the gap. In the Internet Appendix, we show the corresponding findings for US dollar returns.
11. Contingency models
From the year 1841 to 2021, the base case study gathers the details of 39 developed countries’ stock market. We take a comparison in this part to the base case findings with a research method that depends on substitute models. These substitute models have been divided into two classes. First, we build a model that is easy data biased and subject to survival to show the quantitative worth of our model formation process. We take many precautions as mentioned in Part 2 in making our base model to prevent the economy interruptions and conditioning on market results. These design features add the evaluations of multiple month return readings to cater investment performance impact of economic disturbance, the ex-ante recognition of industrialized countries, and incorporation of terminal return of Czechoslovakia. In contrast, past studies mostly focus on investment results in terms of easy and survival data accessibility by using biased samples. For example, there is huge literature that estimates mean real returns, risk premiums or mean nominal return by using the data from individual country stock markets. Dimson et al. (Citation2002) noted that several studies utilize information of models that focus on recent horizons as an outcome that prioritizes researchers for the details, which are not fabricated by any wars, trading halts, hyperinflation and many other impactful events.
Secondly, we build models that measure outcomes of screens. Over a long model horizon in particular, cross-sectional information of countries used for the base case. This shows that the outcomes are managed from horizon information for the countries with the same stock market or data that are not so relevant for today’s investor’s view. Our base case outcomes highlight contrast with those from samples that focus on large-scale populations, most recent data and the equity in the market of capitalized economy.
12. Financial asset distribution inferences
The distribution of accumulated wealth from investment in the financial market shows great risk of loss that highlighted in Section 4. To understand the economic implication of this result, we consider the problem of choosing a portfolio with one risky asset and a risk-free asset. An investor of a industrialised country assumes that the domestic stock index is a risky asset. Investors use information from our large model of financially stable countries to shape the expectations for a long period about financial performance by using the US distribution sample.
We are introducing two substitute features for the risk-free asset. (1) A risk-free inflation-protected asset and (2) cash. Inflation-protected risk-free assets are risk-free in real terms, while cash is only nominal risk-free.
Inflation-protected risk-free asset retains its true principal balance but does not accurately interest, so an investment of $1.00 will retain that value for each equity period as shown in Unit 4. Cash is nominal risk-free but carries an inflation risk. Since inflation is usually positive, a cash position is likely to depreciate in real value over time.
The task of choosing a portfolio of two assets is simply intended to isolate the influence of investor opinion on the efficient allocation of domestic stock markets. In fact, investors have an approach to various asset categories. Home bias in stock holdings is reported in previous studies (French & Poterba, Citation1991), but investors have access to various global stock markets. Investors also have access to government and corporate bonds. They sometimes suffer large losses associated with major stock market crashes (Barro, Citation2006). We choose the risk-free substitutes in our model to avoid risk default and access to information of the government finances of developed countries in this document.
We examine asset allocation for an investor with exponential utility versus ultimate wealth . The investor wants to increase the expected return by referring it to the financial market and risk-free assets, where “a” presents as risk aversion coefficient, “w” represents as weight in stick and WH(w) presents real wealth at H-month with weight “w” and starting investment of US $1.00. The folder is maintained monthly between the free-risk asset and stock market to obtain weight w and 1- w, accordingly. We statistically resolve the optimal weighting by computing the expected return for bootstrap distribution WH (w). If cash is a risk-free asset, then we use the corresponding real inflation for each observation of stock performance in the bootstrap product to find out the actual value of money. Risk aversion parameter fixes to three; however, the findings are consistent with the replacement values. In the Internet Appendix, complete information on how to calculate accumulated wealth and the outcomes of substitute investment models with annuity structure is accessible .
Table 5. Substitute Data
Table 6. Bootstrap Data with bisaness
highlights optimal stock weights for investors of the developed countries and US models. To gain the economic significance in optimal weighting between developed countries and the US, the money that any developed country’s investor is willing to pay in order to accept optimal policies and their preferences instead of US investor policies.
Table 7. Bootstrap Data with more sample
presents investors of the progressed country model that invest less in equities than investors in the US sample. For example, over the 30-year period, an investor of an economically strong country with access to inflation-protected risk-free assets would choose an optimal weight of 43% in stocks relative to US investor weight of 80%. An investor from a stable market can pay a fee up to 0.50% yearly to keep the weight down. In all cases, an investor based on the model of an industrialized country will take lower optimal weight compared to the investor of the US model and the fee ranges as 0.10% to 1.10% yearly. Intuitively, depending on a broad analysis of stable countries, higher equity risk leads to more conservative equity investment, and the difference with the US inference is economically high.
presents an investor with access to cash who invests comparatively more in stock than an investor with access to risk-free, inflation-protected assets. For example, a 30-year-old investor in a developed country chooses 99% weight for cash stock instead of 43% for stocks when there is a risk-free and inflation-protected asset. Although cash has no minimal risk, inflation exposes cash to risk and actual expected loss. To separate the impact of expected real losses, we consider an investor with access to the stock market and an asset of $1.00 investment that provides real payoff of $.35. This investor has an optimal equity weight of 82%, so the difference is 43% allocation with inflation-protected risk-free assets and 99% with cash. The abiding contrast is ascribable due to inflationary risk of cash and the probability that stock safeguard against inflation.
The overall findings with nominal and real risk-free assets show that financial markets are beneficial to save real purchasing power; for example, an investor without evaluating the real risk-free assets in real terms would optimally select high weight in the finance market in spite of its significant risk.
The findings show that asset allocation should take into account a large model of industrialized countries, and also, a higher sense of risk drives lower stock allocation than in the US sample; however, the contrasts are economically significant. The approach of substitute assets that safeguard investors against inflation including principal loss is a key aspect in evaluating the desirability of stocks.
13. Conclusion
We use the long-horizon period and a broad cross-sectional approach of 39 financial market countries from 1841 to 2021 to characterise the distribution of stationary block bootstrap procedure for stock market investors. Furthermore, we expand the time-series and cross-sectional period of the sample of returns and payoffs that reflected in previous literature (e.g., Fama & French, Citation2018a, Citation2018b). This method permits to overcome the availability of data (Dimson et al., Citation2002) and biasness of attributable survival (Brown et al., Citation1995).
The analysis yields three main conclusions. Firstly, diversified equity investments in long-period outcomes are extremely uncertain. Depending on the historical stock market performance data, the fifth percentile real payoff in developed countries is evaluated with regard to 30-year investment $0.47 in a local currency of $1.00 investment, while 95th percentile presents as $23.30. This indicates a conventional view of rotation in equity returns that constructs investing equity comparatively safer in a long period. Secondly, in a 30-year period, catastrophic investment outcomes are common. Thirdly, it is observed that the US historical data for its financial market performance are notably different as compared to the findings that we experienced across many developed markets based on their historical records. Estimates that depend on the US details propose that in real investment there is a rare chance for losses in the long period. The difference in findings shows the implication of economic significance for choice of optimal portfolio, safeguard against survivor and simple data biases to evaluate the payoff distributions.
This study contributes largely to existing studies that try to reduce investor fear with the consideration of the US stock market with various countries’ historical market records. Most of the studies emphasize on estimating equity premiums and average returns (e.g., Dimson et al., Citation2002; Jordà et al., Citation2019). These findings yield meaningful understanding, such as to the manager who needs inputs to calculate the capital cost. In a long period, these insights play an important role in an investor’s expected performance. However, we also consider the magnitude of extreme tail events, higher-order moments, and likelihood by given the single-draw nature of long-term returns for retirement savers. The outcomes of work show that investors should be more careful while considering the risk of loss before equity, and the findings serve as a guide to evaluating such potential results.
Ethical approval
This article does not contain any studies with human participants perform by any of the authors.
Acknowledgements
The corresponding author appreciated the efforts of the research team to this task, which will help out the policy maker for better implementation of policies. The author also wants to appreciate the direction of Anarkulova, Aizhan., Cederburg, Scott. And O’Doherty, and Michael S. for their paper titled “Stocks for the long run? Evidence from a broad sample of developed markets published Journal of Financial Economics”.
Data availability statement
The data will be available on request. The data were collected from Stock Markets and Accounting Research (CSMAR) database, which is the most reliable data source for corporate governance variables.
Disclosure statement
No potential conflict of interest was reported by the authors.
Additional information
Funding
Notes on contributors
Muhammad Jawad
Muhammad Jawad is working as an Assistant Professor in Department of Commerce, Fatima Jinnah Women University. Previously, he performed his duties as Post-Doctoral Research Fellow in the University of York, UK, for two years. He worked as an Associate Dean at University of Lahore. He did his Ph.D. in Leadership and Management Studies from the National Defence University, Pakistan. He has 35 national and international publications in world-renowned journals. He is an associate editor and editorial member of several ESCI and SSCI journals. He represents his university in national and international conferences & workshops.
Munazza Naz
Munazza Naz is working as an Assistant Professor in the Department of Mathematical Sciences, Fatima Jinnah Women University, Pakistan. Previously, he performed his duties as Post-Doctoral researcher in the University of York, UK, for two years. He did his Ph.D. in Mathematical Sciences from Quid-i-Azam University, Pakistan. He has 32 national and international publications in world-renowned journals.
Sohail Rizwan
Sohail Rizwan is serving as an Assistant Professor in Fatima Jinnah Women University, Rawalpindi, Pakistan. He earned Ph.D. majoring in Finance in 2019. His teaching and research portfolio covers working in institutes within Pakistan and abroad. The research domains of interest include corporate finance, corporate frauds, digital finance, entrepreneurial finance, banking, forensic accounting, corporate governance and corporate social responsibility.
Zaib Maroof
Zaib Maroof is a young and dedicated Research Associate working with SP Jain School of Global Management Sydney, Australia. He is a recipient of three Gold Medals and numerous awards and honors including Young Researcher Award 2018 & Young Researcher Award 2019. He has successfully published numerous research papers in well-reputed journals, published a book chapter in Springer Nature, participated in International Research Conferences, successfully delivered research trainings and worked on numerous funded research projects. He is also an active member of Australian and New Zealand Academy of Management (ANZAM). Apart from this, he also has a proven track record of success and experience in different teaching and administrative roles during the last eight years in Higher Education Sector.
Afkar Majeed
Afkar Majeed is currently pursuing his Ph.D. from Azman Hashim International Business School, Universiti Teknologi Malaysia. He is currently working as a Lecturer in Fatima Jinnah Women University, Rawalpindi, Pakistan. He also has many national and international publications.
References
- Akinlo, A. E., & Akinlo, O. O. (2009). Stock market development and economic growth: Evidence from seven sub-Sahara African countries. Journal of Economics and Business, 61(2), 162–26. https://doi.org/10.1016/j.jeconbus.2008.05.001
- Asness, C. S., Moskowitz, T. J., & Pedersen, L. H. (2013). Value and momentum everywhere. The Journal of Finance, 68(3), 929–985. https://doi.org/10.1111/jofi.12021
- Avdis, E., & Wachter, J. A. (2017). Maximum likelihood estimation of the equity premium. Journal of Financial Economics, 125(3), 589–609. https://doi.org/10.1016/j.jfineco.2017.06.003
- Avramov, D., Cederburg, S., & Lucivjanská, ˇ. K. (2018). Are stocks riskier over the long run? Taking cues from economic theory. The Review of Financial Studies, 31(2), 556–594. https://doi.org/10.1093/rfs/hhx079
- Barberis, N. C. (2000). Investing for the long run when returns are predictable. The Journal of Finance, 55(1), 225–264. https://doi.org/10.1111/0022-1082.00205
- Barro, R. J. (2006). Rare disasters and asset markets in the twentieth century. Quarterly Journal of Economics, 121(3), 823–866. https://doi.org/10.1162/qjec.121.3.823
- Barro, R. J., & Ursúa, J. (2008). Consumption disasters in the twentieth century. American Economic Review, 98(2), 58–63. https://doi.org/10.1257/aer.98.2.58
- Barro, R. J., & Jin, T. (2011). On the size distribution of macroeconomic disasters. Econometrica, 79(5), 1567–1589. https://doi.org/10.3982/ECTA8827
- Barro, R. J., & Ursúa, J. (2012). Rare macroeconomic disasters. Annual Review of Economics, 4(1), 83–109. https://doi.org/10.1146/annurev-economics-080511-110932
- Barro, R. J., & Ursúa, J. (2017). Stock-market crashes and depressions. Research in Economics, 71(3), 384–398. https://doi.org/10.1016/j.rie.2017.04.001
- Beck, T., & Levine, R. (2004). Stock markets, banks, and growth: Panel evidence. Journal of Banking and Finance, 28(3), 423–442. https://doi.org/10.1016/S0378-4266(02)00408-9
- Bencivenga, V. R., & Smith, B. D. (1991). Financial Intermediation and endogenous growth. The Review of Economic Studies, 58(2), 195–209. https://doi.org/10.2307/2297964
- Bhide, A. (1993). The hidden costs of stock market liquidity. Journal of Financial Economics, 34(1), 31–51. https://doi.org/10.1016/0304-405X(93)90039-E
- Boyle, G., & Peterson, J. (1995). Monterey policy, aggregate uncertainty, and stock market. Journal of Money, Credit, and Banking, 27(1), 570–582. https://doi.org/10.2307/2077884
- Brown, S. J., & Warner, J. B. (1980). Measuring security price performance. Journal of Financial Economics, 8(3), 205–258. https://doi.org/10.1016/0304-405X(80)90002-1
- Brown, S. J., & Warner, J. B. (1985). Using daily stock returns: The case of event studies. Journal of Financial Economics, 14(1), 3–31. https://doi.org/10.1016/0304-405X(85)90042-X
- Brown, S. J., Goetzmann, W. N., & Ross, S. A. (1995). Survival. The Journal of Finance, 50(3), 853–873. https://doi.org/10.1111/j.1540-6261.1995.tb04039.x
- Campbell, J. Y., & Shiller, R. J. (1988). The dividend-price ratio and expectations of future dividends and discount factors. Review of Financial Studies, 1(3), 195–228. https://doi.org/10.1093/rfs/1.3.195
- Campbell, J. Y., & Viceira, L. M. (2002). Strategic asset allocation: Portfolio choice for long-term investors. Oxford University Press.
- Chami, R., Cosimano, T. F., & Gapen, M. (2006). Beware of emigrants bearing gifts: Optimal Fiscal and Monetary policy in the presence of remittances. IMF Working Papers 06/61 Washington: International Monetary Fund.
- Chen, N., Roll, R., & Ross, S. (1986). Economic forces and the stock market. The Journal of Business, 59(3), 383–403. https://doi.org/10.1086/296344
- Cottle, S., Dodd, D. L., & Graham, B. (1962). Security Analysis. New York: McGraw.
- Deb, S. G., & Mukherjee, J. (2008). Does stock market development cause economic growth? Time series analysis for Indian economy. International Research Journal of Finance and Economics, 21(3), 142–149. https://doi.org/10.1016/S2212-5671(12)00177-3
- Devereux, M. B., & Smith, G. W. (1994). International Risk Sharing and Economic Growth. International Economic Review, 35, 535–550.
- Diamond, D. W. (1996). Financial intermediation as delegated monitoring: A simple example. Federal Reserve Bank of Richmond Economic Quarterly, 82(3) Summer, 82–83. https://www.richmondfed.org/-/media/RichmondFedOrg/publications/research/economic_quarterly/1996/summer/pdf/diamond.pdf
- Dimson, E., Marsh, P. R., & Staunton, M. (2002). Triumph of the optimists: 101 years of global investment returns. Princeton University Press.
- El-Wassal, K. A. (2005). Stock market growth: An analysis of cointegration and causality. Economic Issues, 10(1), 37–58. https://www.usc.es/economet/reviews/aeid13116.pdf
- Engle, R. F. (1982). Autoregressive conditional heteroscedasticity with estimates of the variance of United Kingdom inflation. Econometrica, 50(4), 987–1007. https://doi.org/10.2307/1912773
- Enisan, A. A., & Olufisayo, A. O. (2009). Stock market development and economic growth: Evidence from seven sub-Sahara African countries. Journal of Economics and Business, 61(2), 162–171. https://doi.org/10.1016/j.jeconbus.2008.05.001
- Fama, E. F. (1981). Stock returns, real activity, inflation and money. American Economic Review, 71(4), 545–565. http://www.jstor.org/stable/1806180
- Fama, E. F. (1990). Stock returns, expected returns and real activity. Journal of Finance, 45(4), 1089–1108. https://doi.org/10.1111/j.1540-6261.1990.tb02428.x
- Fama, E. F., & French, K. R. (1998). Value versus growth: The international evidence. The Journal of Finance, 53(6), 1975–1999. https://doi.org/10.1111/0022-1082.00080
- Fama, E. F., & French, K. R. (2018a). Long-horizon returns. The Review of Asset Pricing Studies, 8(2), 232–252. https://doi.org/10.1093/rapstu/ray001
- Fama, E. F., & French, K. R. (2018b). Volatility lessons. Financial Analysts Journal, 74(3), 42–53. https://doi.org/10.2469/faj.v74.n3.6
- French, K. R., & Poterba, J. M. (1991). Investor diversification and international equity markets. American Economic Review, 81, 222–226. https://www.nber.org/system/files/working_papers/w3609/w3609.pdf
- Fulghieri, P., & Rovelli, R. (1998). Capital markets, financial intermediaries and liquidity supply. Journal of Banking and Finance, 22(9), 1157–1180. https://doi.org/10.1016/S0378-4266(98)00053-3
- Garcia, F. V., & Lin, L. (1999). Macroeconomic determinants of stock market development. Journal of Applied Economics, 2(1), 29–59. https://doi.org/10.1080/15140326.1999.12040532
- Geske, R., & Roll, R. (1983). The Fiscal and Monetary linkage between stock market returns and inflation. Journal of Finance, 38(1), 7–33. https://doi.org/10.1111/j.1540-6261.1983.tb03623.x
- Goetzmann, W. N., & Jorion, P. (1995). A longer look at dividend yields. The Journal of Business, 68(4), 483–508. https://doi.org/10.1086/296674
- Golez, B., & Koudijs, P. (2018). Four centuries of return predictability. Journal of Financial Economics, 127(2), 248–263. https://doi.org/10.1016/j.jfineco.2017.12.007
- Greenwood, J., & Smith, B. (1997). Financial markets in development and the development of financial markets. Journal of Economic Dynamics & Control. https://doi.org/10.1016/0165-1889(95)00928-0
- Harris, R. (1997). Stock markets and development: A re-assessment. European Economic Review, 41(1), 139–146. https://doi.org/10.1016/S0014-2921(96)00021-9
- Hassapis, C., & Kalyvitis, S. (2002). Investigating the links between growth and stock price changes with empirical evidence from the G7 economies. Quarterly Review of Economics and Finance, 42(3), 543–575. https://doi.org/10.1016/S1062-9769(01)00111-9
- Jawad, M., Naz, M., & Shamsi, M. A. (2021). Impact of noninterest income on bank risk-taking and bank lending spread. Review of Pacific Basin Financial Markets and Policies, 24(4), 2150029. https://doi.org/10.1142/S0219091521500296
- Jordà, Ò., Knoll, K., Kuvshinov, D., Schularick, M., & Taylor, A. M. (2019). The rate of return on everything, 1870–2015. The Quarterly Journal of Economics, 134(3), 1225–1298. https://doi.org/10.1093/qje/qjz012
- Jorion, P., & Goetzmann, W. N. (1999). Global stock markets in the twentieth century. The Journal of Finance, 54(3), 953–980. https://doi.org/10.1111/0022-1082.00133
- Kosowski, R., Timmermann, A., Wermers, R., & White, H. (2006). Can mutual fund “stars” really pick stocks? New evidence from a bootstrap analysis. The Journal of Finance, 61(6), 2551–2595. https://doi.org/10.1111/j.1540-6261.2006.01015.x
- Kuznets, S. (1973). Modern economic growth: Findings and reflections. American Economic Review, 63(3), 247–258. http://links.jstor.org/sici?sici=0002-8282%2819730O%3B2-I&origin=repec
- Levine, R. (1991). Stock market, growth, and tax policy. Journal of Finance, 46(4), 1445–1465. https://doi.org/10.1111/j.1540-6261.1991.tb04625.x
- Levine, R. (1996). Stock markets: A spur to economic growth. Article Based on 12 Papers Presented at a World Bank conference, “Stock Markets, Corporate Finance, and Economic Growth” World Bank, 1995, Washington DC, World Bank.
- Levine, R., & Zervos, S. (1998). Stock markets, banks and economic growth. American Economic Review, 88(3), 537–558. http://www.jstor.org/stable/116848
- Linnainmaa, J. T., & Roberts, M. R. (2018). The history of the cross-section of stock returns. The Review of Financial Studies, 31(7), 2606–2649. https://doi.org/10.1093/rfs/hhy030
- Lo, A. W., & MacKinlay, A. C. (1988). Stock market prices do not follow random walks: Evidence from a simple specification test. Review of Financial Studies, 1(1), 41–66. https://doi.org/10.1093/rfs/1.1.41
- Lundblad, C. (2007). The risk return tradeoff in the long run: 1836–2003. Journal of Financial Economics, 85(1), 123–150. https://doi.org/10.1016/j.jfineco.2006.06.003
- Malliaropulos, D. (1996). Money, long-run super neutrality, and real equity prices. Discussion paper, (7), Brunel University: UK.
- Martin, I. W. R., & Wagner, C. (2019). What is the expected return on a stock? The Journal of Finance, 74(4), 1887–1929. https://doi.org/10.1111/jofi.12778
- Mayer, C. (1988). New issues in corporate finance. European Economic Review, 32(5), 1167–1188. https://doi.org/10.1016/0014-2921(88)90077-3
- McLean, R. D., & Pontiff, J. (2015). Does academic research destroy stock return predictability? The Journal of Finance, 71(1), 5–32. https://doi.org/10.1111/jofi.12365
- Mehra, R., & Prescott, E. C. (1985). The equity premium: A puzzle. Journal of Monetary Economics, 15(2), 145–161. https://doi.org/10.1016/0304-3932(85)90061-3
- Mitchell, M. L., & Stafford, E. Managerial decisions and long-term stock price performance (October 1999). CRSP Working Paper No. 453, SSRN: https://ssrn.com/abstract=94137;http://dx.doi.org/10.2139/ssrn.94137.
- Mukherjee, T. K., & Naka, A. (1995). Dynamic relations between macroeconomic variables and the Japanese stock market: An application of a vector error correction model. Journal of Financial Research, 63(2), 223–237. https://doi.org/10.1111/j.1475-6803.1995.tb00563.x
- Naheed, R., Jawad, M., Naz, M., Sarwar, B., & Naheed, R. (2021). Managerial ability and investment decisions: Evidence from Chinese market. Managerial and Decision Economics, 42(4), 985–997. https://doi.org/10.1002/mde.3287
- Nakamura, E., Steinsson, J., Barro, R., & Ursúa, J. (2013). Crises and recoveries in an empirical model of consumption disasters. American Economic Journal, 5(3), 35–74.
- Nishat, M., & Saghir, M. (1991). The stock market and Pakistan economy. Savings and Development, 15(2), 131–145. http://www.jstor.org/stable/25830261
- Pan, L., & Mishra, V. (2017). Stock market development and economic growth: Empirical. https://doi.org/10.1016/j.econmod.2017.07.005
- Pástor, Ľ., & Stambaugh, R. F. (2012). Are stocks really less volatile in the long run? The Journal of Finance, 67(2), 431–477. https://doi.org/10.1111/j.1540-6261.2012.01722.x
- Patton, A. J., & Timmermann, A. (2010). Monotonicity in asset returns: New tests with applications to the term structure, the CAPM, and portfolio sorts. Journal of Financial Economics, 98(3), 605–625. https://doi.org/10.1016/j.jfineco.2010.06.006
- Politis, D. N., & Romano, J. P. (1994). The stationary bootstrap. Journal of the American Statistical Association, 89(428), 1303–1313. https://doi.org/10.1080/01621459.1994.10476870
- Poterba, J. M., & Summers, L. H. (1988). Mean reversion in stock prices: Evidence and implications. Journal of Financial Economics, 22(1), 27–59. https://doi.org/10.1016/0304-405X(88)90021-9
- Poterba, J., Rauh, J., Venti, S., & Wise, D. (2007). Defined contribution plans, defined benefit plans, and the accumulation of retirement wealth. Journal of Public Economics, 91(10), 2062–2086. https://doi.org/10.1016/j.jpubeco.2007.08.004
- Randal, M., Shleifer, A., & Vishny, R. W. (1990a). Do managerial objectives drive bad acquisitions? Journal of Finance, 45(1, March), 31–48. https://doi.org/10.1111/j.1540-6261.1990.tb05079.x
- Randal, M., Shleifer, A., & Vishny, R. W. (1990b). The stock market and investment: Is the market a sideshow? Brookings Papers on Economic Activity, 2, 157–215.
- Rietz, T. A. (1988). The equity premium: A solution. Journal of Monetary Economics, 22(1), 117–131. https://doi.org/10.1016/0304-3932(88)90172-9
- Romer, D. (1993). Credit channel or credit action? An interpretation of the post-war transmission mechanism. In J. Wyoming (Ed.), Changing capital markets: Implication for monetary policy, 1–66. Federal Reserve Bank of Kansas City.
- Schwert, G. W. (2003). Anomalies and market efficiency. In G. M. Constantinides, M. Harris, & R. M. Stulz (Eds.), Handbook of the Economics of Finance (pp. 939–974). Elsevier.
- Shahbaz, M., Ahmed, N., & Ali, L. (2008). Stock Market Development and Economic Growth: ARDL Causality in Pakistan. International Research Journal of Finance and Economics, 14, 1450–2887.
- Shiller, R. J. (2003). Social security and individual accounts as elements of overall risk-sharing. American Economic Review, 93(2), 343–347. https://doi.org/10.1257/000282803321947326
- Shiller, R. J. (2006). Life-cycle personal accounts proposal for social security: An evaluation of President Bush’s proposal. Journal of Policy Modeling, 28(4), 427–444. https://doi.org/10.1016/j.jpolmod.2005.10.010
- Shleifer, A., & Vishny, R. W. (1986). Large shareholders and corporate control. Journal of Political Economy, 96(3), 461–488. https://doi.org/10.1086/261385
- Shleifer, A., & Summers, L. (1988). Breach of trust in hostile takeovers. In A. Auerbach (Ed.), Corporate takeovers: Causes and consequences. Chicago: University of Chicago Pres.
- Siegel, J. (2014). Stocks for the long run. McGraw-Hill.
- Silber, W. L. (2005). What happened to liquidity when World War I shut the NYSE? Journal of Financial Economics, 78(3), 685–701. https://doi.org/10.1016/j.jfineco.2005.02.005
- Stiglitz, J. E. (1985, May). Credit markets and the control of capital. Journal of Money, Credit, and Banking, 17(2), 133–152. https://doi.org/10.2307/1992329.
- Stiglitz, J. E. (1994). The role of the state in financial markets. In Proceedings of the World Bank Annual Conference on Development Economics 1993, Washington, D.C.: World Bank.
- Sullivan, R., Timmermann, A., & White, H. (1999). Data-snooping, technical trading rule performance, and the bootstrap. The Journal of Finance, 54(5), 1647–1691. https://doi.org/10.1111/0022-1082.00163
- Tobin, J. (1969). A general equilibrium approach to monetary theory. Journal of Money, Credit, and Banking, 1(1), 15–29. https://doi.org/10.2307/1991374
- Vaona, A. (2012a). Inflation and Growth in the Long-Run: A New-Keynesian Theory and Further Semiparametric Evidence. Macroeconomic Dynamics, 16, 94–132.
- Viceira, L. M. (2008). Life-cycle funds. In A. Lusardi (Ed.), Overcoming the saving slump: How to increase the effectiveness of financial education and saving programs (pp. 140–177). Chicago Press.