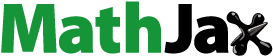
Abstract
Livelihood diversification is important for urban development to end poverty and food insecurity problems. In Ethiopia, urban livelihood diversification is quite low specifically in North Shewa Zone. Therefore, this study aims to identify the determinants of livelihood diversification strategies for urban households in the North Shewa Zone. The data were collected through both primary and secondary data collection methods. A multistage sampling technique was employed to select 398 household heads from three urban areas of the North Shewa Zone. A multinomial logistic regression model was employed to analyze the determinant of livelihood diversification strategies in the study area. The results of the model revealed that livelihood diversification strategies were determined by the age of the household head, education status, family size, credit access, market access, and training and extension service positively and the dependency ratio was negative. The study recommends that the zonal and regional governments of Ethiopia should develop a comprehensive urban development policy that could empower off-farm and non-farm urban livelihood diversification strategies besides the farm activities.
PUBLIC INTEREST STATEMENT
Urban livelihood diversification is a crucial issue for economic development. This study aims to identify the determinants of livelihood diversification strategies for urban households in the North Shewa Zone. This study revealed that the age of the household head, education status, family size, credit access, market access and training and extension service positively, and dependency ratio are the determinants of urban livelihood diversification strategies in North Shewa Zone, Amhara Region, Ethiopia.
1. Introduction
Livelihood diversification is attracting considerable interest as a tool to cope with economic shocks and resist vulnerability (Helmy, Citation2020). The ultimate goal of livelihood diversification is bringing sustainable livelihood outcomes like securing economic, social and environmental improvement for urban households (Tambe, Citation2022). Households diversify their livelihood as a result of different factors that could be categorized into Push Factors such as income seasonality, credit market failure, and liquidity constraints; Pull Factors such as improvement in education, technology, labor markets, infrastructure, or market access. The former factors are commonly leading to low return strategies (survival-led strategies) while the latter is related to opportunities in the market-leading for high return activities (opportunity-led strategies; Alobo & Bignebat, Citation2017).
Ethiopia is the second-most populous country in the African continent next to Nigeria. Livelihood diversification is believed to be a solution, and an effective strategy for the reduction of poverty and food insecurity in Ethiopia (Yizengaw et al., Citation2015). The more choice and flexibility people have in their livelihood strategies through livelihood diversification, the greater the ability they withstand shocks and stresses. Even though the diversity of household livelihoods is an important feature of urban and rural development, its role is often ignored by policymakers (Getinet & Lorato, Citation2020). Despite the presence of many agricultural policies, Ethiopia’s agricultural production is deemed to be low, and it concentrates mostly on on-farm agricultural development. So far, these policies haven’t included non-agricultural livelihood strategies in the policy framework (Kassie et al., Citation2017).
Despite the fact of its growing importance to Ethiopia’s poor, the necessity of livelihood diversification has gotten little attention. There is a large body of evidence indicating livelihood diversification activities play a substantial influence in increasing household income and cope with different livelihood shocks (Gebru et al., Citation2018; Mekuria & Mekonnen, Citation2018). Several Ethiopian research studies, for example (Admasu et al., Citation2022; Muluneh, Citation2022), have looked at a variety of factors that influence the choice of livelihood diversification alternatives. The distribution of income and wealth status influences which type of livelihood diversification activities’ households choose and implement. However, urban households’ livelihood diversification is not unique, and the factors determining households’ for choosing and adopting livelihood diversification strategies were not yet studied in the North Shewa district.
Therefore, this study aims to examine the determinants of livelihood diversification in Ethiopia with a particular focus on North Shewa Zone. This paper contributes to the literature by inspiring to gain more insight into the factors that affect urban household’s livelihood diversification strategies in North Shewa Zone, Amhara region, Ethiopia. The contribution of the study to the existing literature is three-fold. First, the previous studies have been emphasized the determinants of livelihood diversification strategies for rural households, for instance (Ambaye et al., Citation2021; Ayana et al., Citation2021; Gebru et al., Citation2018; Kassie et al., Citation2017; Washo et al., Citation2021). However, little attention has been given to livelihood diversification in urban settings. Second, many studies evaluated the livelihood diversification strategies in showing a distinct component of livelihood diversification strategies options classified by sector as farm or non-farm, by function as wage employment or self-employment, or by location as on-farm or off-farm and fails to account for complementarity of livelihood diversification strategies, for example (Alobo & Bignebat, Citation2017: Bowen & De Master, Citation2011; Saha & Bahal, Citation2012; Teshome & Edriss, Citation2013). However, this paper evaluates seven livelihood diversification strategy options, namely: On-farm only; Non-farm only, Off-farm only, On-farm+ Non-farm; On-farm + Off-farm; Off-farm + Non-farm; On-farm+ Off-farm + Non-farm. Third, the empirical literature shows that the determinants of livelihood diversification strategies in Ethiopia in general and study area in particular were less researched.
The study was organized into five parts in which the first part of the study deals with an introduction; the second part deals with a review of the related literature; the third part deals with research methodology; the fourth part, deals with results and discussion and the final part of the study deals with the conclusion and recommendation as well as Limitations and areas for further research.
2. Literature review
2.1. Concepts and definitions of livelihood diversification
The term “livelihood” refers to a way of living to sustain one’s life and provide basic needs (Khatun & Roy, Citation2012). Livelihood attempts to capture not just what people do to make a living, but the resources that provide them with the capability to build a satisfactory living, the risk factors that they must consider in managing their resources, and the institutional and policy context that either helps or hinders then in their pursuit of a viable or improving living (Ellis, Citation2003). Livelihood diversification is defined as the process by which rural families construct a diverse portfolio of activities and social support capabilities in their struggle for survival and to improve their standards of living (Ellis, Citation1998). Therefore, livelihood strategies are the combination of activities that people choose to undertake to achieve their livelihood goals (Ellis & Allison, Citation2004). Livelihood diversification is grouping of on-farm, off-farm and non-farm activities to earn a living (Njuguna, Citation2015).
2.2. Determinants of livelihood diversification
There are several evidences in Africa and Ethiopia that show how livelihood diversification can be determined. In a study conducted by Shikur et al. (Citation2021), livelihood strategy choices in peri-urban communities of Hossana town, Hadiya Zone, Southern Ethiopia. The study employed a multivariate probit model. Model results showed that dependency ratio, active labor force in the family, sex of household head, educational levels of household heads, annual total income, market distance, household asset ownership, mass media exposure, achievement motivation, information-seeking behavior, distance to public transport, total cultivated land, livestock (other than ox) and extension contact significantly affected the choice of livelihood strategies. According to the study of Echebiri et al. (Citation2017) on the effect of livelihood diversification on food security status of rural farm households in Abia State Nigeria by using the logit regression model found out, diversification was influenced by household size, amount of credit received, education of the household head, cooperative membership, and monthly income, while food security status was influenced by years of education of household head, credit access, monthly income, age of household head and household size.
Helmy (Citation2020) investigates the evolution of livelihood diversification in Egypt with a particular focus on wealth and urban-rural divides. Using Egypt Labor Market Panel data from 2006 to 2018, it was found that urban households had significantly less livelihood diversification than rural households. Wealthy households in urban areas tended to depend on relatively more specialized livelihood clusters that were stable over time. These findings imply that distress is a potential reason for diversification in urban areas. Ambachew and Ermiyas (Citation2016) employed a multinomial logit model to empirically examine the determinants of rural household livelihood diversification strategy in South Gondar zone, using cross-sectional data. The result of the study revealed that the size of land holding by the rural household had a significant and negative effect on non-farm and off-farm activities, educated household heads were more participated in non-farm and off-farm activities than uneducated heads and also found out male household heads are more participated than the female household head, because in developing countries, females are constrained by cultural challenges and they engaged themselves in house activities.
Wegedie (Citation2018) examines determinants of households’ livelihood strategy choices and impact analysis of urbanization of Bahir Dar city on peri-urban households’ livelihood strategies. Multinomial logit regression analysis and propensity score matching (PSM) model were used. The PSM estimation result shows that there is an insignificant difference between displaced and non-displaced in terms of the main outcome variables, livelihood outcome; however, there is a significant difference in terms of human capital, physical capital, and natural capital. The findings of this study suggest that policymakers need to reflect on the most suitable way of mitigating the negative impacts of farmland loss on peri-urban areas. According to Mulugeta (Citation2009), the paper aims at identifying the determinants of the livelihood strategies of female household heads in Wolenchiti town, in the Eastern Oromia Region of Ethiopia. The study identifies factors determining the choice of livelihood strategies, the study found that age, age at first marriage, household size, number of years of headship, and marital and migration status are the most important demographic factors at work. As regards socio-economic factors, the results of the study show that access to financial, human, social, physical, and natural capital were the leading determinants of livelihood strategies.
Debele and Desta (Citation2016) analyzed livelihood diversification status, challenges, and factors influencing pastoral households’ engagement in livelihood diversification activities in the Bale pastoral livelihood zone. It employed a multinomial logistic regression model. The study found age, education level of household head, size of livestock holding, distance to market, and access to rural credit services were the major determinants of livelihood diversification. Yizengaw et al. (Citation2015) examined the determinants of livelihood diversification strategies: the case of smallholder rural farm households in Debre Elias Woreda, East Gojjam Zone, Ethiopia by using the Multinomial logit model. The results of the study demonstrated that land size, livestock holding size, sex of household head, mass media, market distance, total annual household income, and urban linkage were found to be the significant determinants. Ambachew and Ermiyas (Citation2016) employed a multinomial logit model to empirically examine the determinants of rural household livelihood diversification strategy in the South Gondar zone, using cross-sectional data. Another study was done by Guite et al. (Citation2022) examined livelihood diversifications among the Thadou-Kuki tribes of Manipur using the Simpson index and also found out the determinant of livelihood diversification using multiple regression. The study relies on household-level data from a census survey conducted in 15 villages spread throughout three Thadou-Kuki-dominated regions. The results of the multiple regression show that the distance of the village from the market, the number of farm household members, the number of non-farm household members, wetland area, and access to forest resources all positively impact livelihood diversification, while the average worker educational attainment and cash cropland have negative impacts.
In a study conducted by Admasu et al. (Citation2022), Herfindahl–Hirschman Index and multinomial logistic regression were employed. The study’s findings demonstrate that rather than relying just on agriculture to improve their well-being, households in the study town are pursuing various livelihood diversification options, with livelihood diversification playing an increasingly important role in household income. Diversification as a livelihood strategy depends on several interrelated factors including the context factors households face (including vulnerability to shocks) and the different types of capital that households draw upon to generate their livelihood (Musumba et al., Citation2022). Dirribsa and Tassew (Citation2015) studied the factors that influence households’ livelihood diversification methods in Ethiopian regions Ambo district, Oromia regional state. The result revealed that agroecology, sex, education, farmland size, family size, livestock ownership, participation in social institutions, membership in cooperatives, contact with extension agents, access to sources of credit, and age were all clear determinants of livelihood diversification strategies. Overall, previous earlier research has focused on the causes of rural livelihood diversification; however, livelihood diversification initiatives in Ethiopia’s urban areas have received less attention.
3. Research methodology
3.1. Description of the study area
North Shewa is 1 of 10 zones in the Ethiopian Amhara Region. North Shewa takes its name from the kingdom or former province of Shewa. The Zone is bordered on the south and the west of the Oromia Region, on the north by South Wollo, on the northeast by the Oromia Zone, and on the east by the Afar Region. North Shewa Zone is 1 of the 11 zones under the Amhara regional state with a total area of 17,697.64 square kilometers. The administrative structure is divided into 22 woredas and 5 city administrations and has a population of 2,226, 685. A total of 429,423 households were counted in this Zone, which results in an average of 4.28 persons to a household, and 413,235 housing units. The zone is located at 8.38−10.42 north latitude and 384.4–40.3 east longitude at an altitude of 1500–4000 meters above sea level. The plain is 35.9%, uneven 26.11%, mountainous 25.9%, valleys 11.7%, 0.3% swamps and 0.02% is covered by water and the climate is 35% high, 30.65% highland, 33.7% low and 0.6% snowy. The three largest ethnic groups reported in North Shewa were the Amhara (90.73%), the Oromo (7.14%), and the Argobba (1.71%); all other ethnic groups made up 0.42% of the population. Amharic is spoken as a first language by 92.97%, and 6.32% spoke Oromia; the remaining 0.71% spoke all other primary languages reported. Moreover, 94.71% of the population said they practiced Ethiopian Orthodox Christianity, and 4.91% were Muslim (CSA, Citation2014)
3.2. Data types and source
To achieve the objectives of this research, all required data were collected from primary data sources. The study was conducted with primary data sources from sample households using a pre-tested structured questionnaire by applying face-to-face interviews to reduce the non-response rate and incompleteness of data.
3.3. Sample size and sampling techniques
North Shewa Zone is surrounded by three urban areas, such as “Debre Berhan”, “Shewarobit” and “Mehalmeda”. The choice of sampling technique (probability or non-probability) depends on the purpose of the study. For such quantitative research, a probability sampling technique is appropriate as compared to the non-probability sampling technique because it gives an equal chance of being interviewed for every sample household. From different techniques of probability sampling for this study, multi-stage sampling was used.
For this research, purposive sampling, proportional sampling, and simple random sampling techniques were employed for the selection of the study site. In the beginning, the study site, the North Shewa Zone, will be selected purposively due to the familiarity of the researchers to study areas plays a great role to choose the areas for the study. In the next stage, proportional sampling was used to determine the sample size from each area, to avoid the problem of bias and to represent each area adequately. Finally, simple random sampling techniques were used to select households to take respondents from each area. In this study, Yamane’s (Citation1973) formula was used to determine the sample size. The formula is expressed as follows:
where n is the sample size, N is the population, and e is the error tolerance or margin of error using a 5% level of error or 95% level of confidence. The study used a proportionate or equalized sampling technique to take a sample from each woreda, which is specified as follows.
, where
represents the sample from strata or kebele
, n sample size,
population and
is the number of households in Woreda
. The summary of proportional distribution to the three woredas is summarized in the Table .
Table 1. Number of population and households in the North Shewa Zone
Finally, about 398 sample sizes are taken from Shewarobit, Mehal Meda, and Debre Berhan through a random sampling technique. According to Lavrakas (Citation2008), the term representative sample has many different meanings, along the lines of the sample having the same distribution of the population on some key demographic characteristics and the sampling process must have a components random selection. This study uses simple random sampling techniques as a component and the target group incorporated in the research was living in urban areas that have homogeneous characteristics. Therefore, the samples were representative of the population.
3.4. Methods of data collection
In this study, the following activities were carried out before the survey was done. Firstly questionnaires are translated into the local language (Amharic). The data set for this study was obtained from both primary and secondary data sources, which are qualitative and quantitative. The primary data was collected from structured sample household head interviews.
3.5. Empirical model specification, data analysis, and definition of variables
3.5.1. Empirical model specification
According to Maddala (Citation1986), when the regression or analysis incorporates a mix of explanatory variables and for instance, variables, which are nominal, or scale type (particularly alternative invariants) and when the dependent variable takes more than two nominal categories which are unordered, then multinomial models are appropriate. Specifically, in this study among these models, the multinomial logit model will be applied. This model has the same advantage over the other multinomial models like the multinomial probit model. For instance, the most important advantage is that of computational advantage over the probit model. This is because the multinomial probit model takes several integrals to determine the estimate of one less than the outcome of the dependent variables or categories. The other advantage is that it allows for more than four categories of alternatives, whereas for the multinomial probit model the number of alternatives must be at most four. Consequently, taking those advantages into account, to find the determinants of livelihood diversification, the researchers will use a model called the multinomial logit model.
The core aim of this investigation is to analyze the determinants of livelihood diversification strategies. In this study, the dependent variable livelihood diversification strategies were classified into seven, namely On-farm only; Non-farm only, Off-farm only, On-farm+ Non-farm; On-farm + Off-farm; Off-farm + Non-farm; On-farm+ Off-farm + Non-farm. Based on this, the current study states the standard multinomial logit model as follows:
, LD, is Livelihood Diversification, which is categorical,
represents the independent variables expected to affect diversification of livelihood strategy such as Sex of the household head, Age of the household head, Marital status of Household Head, Education status of a Household Head, Total Family Size of household head, Available technical Skill of the household head, Access to credit, Training and Extension Services, Access to Market and Dependency ratio. The study assumes that if the choice of the household lies in livelihood strategies, rational household heads choose among the seven mutually exclusive livelihood strategy alternatives that offer the maximum utility. This gives rise to the polychotomous choice framework. Hence, the probability of a household diversification j (j = 0 On-farm only; j = 1 Non-farm only, j = 2 Off-farm only, j = 3 both On-farm and Non-farm; j = 4 On-farm + Off-farm; j = 5 Off-farm + Non-farm; j = 6 On-farm+ Off-farm + Non-farm) is given by the following multinomial logit model.
The multinomial probability model assumes that the possible distinct states are exhaustive in that they cover all possibilities. The likelihood function for a sample of independent observations is then
where the subscript i denotes the ith of N individuals and the subscript j denotes the jth m alternatives.
The log-likelihood function is
where is a function of parameters β and explanatory variables. More generally, the number of alternatives may vary across different individuals, so that m choices become mi choices.
The first-order conditions for the maximum likelihood estimator β are that it solves
This is usually nonlinear in β. The distribution of yi- is necessarily multinomial which ensures consistency as then
Maximizing the log-likelihood function with respect to the parameters:
, for
,
The second-order condition becomes
, where
is an indicator variable equal to 1 if j = k and equal to 0 if j ≠ k
Unlike the standard regression analysis, the parameter value (β) is not directly interpretable as the effect of the change in the explanatory variable on the mean or expected value of the dependent variable. In particular, for multinomial logit models, a positive regression parameter does not mean that an increase in the explanatory variables leads to an increase in the probability of that alternative. Instead, interpretation for the multinomial logit model is relative to the reference or base category group, which this study used On-farm only as a base category.
The coefficients need to be adjusted to be marginal effects in the case of the logit model. In other words, the marginal effect, which gives the partial derivatives indicating the change in the probability of the dependent variable relative to a unit change in one of the independent variables needs to be computed. As the relationship between the explanatory variable and the absolute probabilities is non-linear, marginal effects vary according to the choice of vector Xs and, consequently, they will vary among individuals according to the point of evaluation. By differentiating the multinomial logit model, we find the marginal effects of the explanatory variables on the probabilities:
For continuous variables, the marginal effect is the probability change in response to a unit change in the value of the independent variable at the mean value. For dummy variables, the marginal effect is computed as the difference in probabilities of the dependent variable between the group with designated value 1 and the base category. Furthermore, it should be noted that the signs of the beta (β) coefficients are not necessarily the same as that of the marginal effects (Greene, Citation2008).
In this study, different pre- and post-estimation tests are adopted. The Hausman test was employed to check for the existence of independence of irrelevant alternative (IIA). Hausman test is a standardized comparison of model coefficients, using it with mlogit requires that the base outcome be the same in both competing models. In particular, if the most-frequent category (the default base outcome) is being removed to test for IIA, or by determining the base manually, having this, the missing values for the square root of the diagonal of the covariance matrix of the differences is not comforting, but it is also not surprising (Hausman, Citation1978). In addition, multicollinearity tests were carried out using the Variance Inflation Factor (VIF), with a VIF value greater than 10 indicating strong multicollinearity (Gujarati (Citation2004). Heteroskedasticity occurs when the dependent variable’s variance varies across the data (Gujarati, Citation2004). The Breusch–Pagan/Cook–Weisberg test was used to evaluate it. Furthermore, the generalized Hosmer-Lemeshow and pseudo-R-squares statistic tests are employed to determine whether or not the model is fit. By subgrouping the probability calculated from the data, the generalized-Lemeshow statistic determines whether the observed occurrences match the expected events. The null hypothesis states that the differences between observed and expected events are minor, implying that the model is valid or fits the data (El-Habil, Citation2012).
3.5.2. Data analysis
In this study, both econometrics and descriptive statistical tools were employed. To identify the determinants of livelihood diversification choices, multinomial logistics regression model was used. Furthermore, descriptive statistics were employed to identify and examine livelihood activities that are pursued by urban households in the study area. As a means of describing the data, the researcher used frequencies, percentages, mean, minimum, and maximum. The F-test and Chi-square test statistics were also employed to differentiate the statistical significance of variables on livelihood diversification strategies for the continuous and discrete explanatory variables.
3.5.3. Definition of variables
In this study, the dependent variable was livelihood diversification and there are different independent variables (Table .
Table 2. Summary of definition, measurements of variables, and its expected sign
4. Results and discussion
4.1. Descriptive statistics
4.1.1. Demographic characteristics of sample households
4.1.1.1. Sex of the household head
The sex of the household head is one of the important factors expected to affect the household livelihood diversification strategy in the study area. This study involved about 79.4% of male-headed households and 20.6% of female-headed as shown in Table . The result indicated that, out of the total 79.4% of male-headed households 12.5%, 6.4% 25.6%, 30.0%, 7.4%,17.4% and 1% of the households participated into On-farm only, Non-farm, Off-farm only, On-farm + Non-farm, On-farm + Off-farm, Off-farm + Non-farm and On-farm + Off-farm + Nonfarm livelihood strategies respectively. The result also depicts that, out of the total 20.6% of female households 22.1%, 15.6%, 23.4%, 7.8%, 16.9% 13.0% and 1.3 %, of them engaged into On-farm only, Non-farm, Off-farm only, On-farm + Non-farm, On-farm + Off-farm, Off-farm + Non-farm and On-farm + Off-farm + Nonfarm livelihood strategies, respectively. This result indicates that in the study area, the engagement in livelihood diversification strategies for female-headed households is lower than for male-headed. The probable reasons are due to cultural factors that females are assigned to care for the child, perform homework and other social roles. The Chi-square result (χ2 = 28.672; P = 0.000) which was statistically significant at 1% probability level also shows that there was a statistically significant difference between male and female-headed households’ choice to livelihood diversification strategies. This finding is consistent with the study by (Ambachew & Ermiyas, Citation2016); male-headed households have more participated in various livelihood activities than the female-headed households head because in developing countries females are constrained by cultural challenges and they are engaged themselves in house activities.
Table 3. Distribution of livelihood diversification by sex of the respondents
4.1.1.2. Marital status of the household
The marital status of the household is one of the expected variables, which affect the livelihood diversification of the urban people. In the study area, as it can be seen in , 34.8 % of the people are unmarried, and out of this 13.1%, 8.5%, 24.6%, 23.1%, 13.8%, 15.4%, and 1.5% of households were engaged into On-farm only, Non-farm only, Off-farm only, On-farm + Non-farm, On-farm + Off-farm, Off farm+ Non-farm and On-farm + Off-farm + Non-farm livelihood activities, respectively, whereas 1.6% of the sampled households were widowed. Out of these 16.7% are On-farm, also 16.7% are Non-farm only, 50% were engaged in Off-farm only, and 16.7% were engaged in On-farm + Non-farm livelihood activities. Furthermore, majority 57.5% of the people are married, and out of this 15.3%, 7.4%, 24.7%, 28.4%, 6.0%, 17.2% and 0.9% of households were engaged into On-farm only, Non-farm only, Off-farm only, On-farm + Non-farm, On-farm + Off-farm, Off farm+ Non-farm and On-farm + Off-farm + Non-farm livelihood activities, respectively. The result shows that in the study area, married households currently living with their partner diversified their livelihood activities more than others. The Chi-square result (χ2 = 15.024; P = 0.660), unfortunately, was not statistically significant.
Table 4. Distribution of Livelihood diversification by marital status of the respondents
4.1.1.3. Age composition of the household head
The age of the farm household head is one of the expected variables that determine the involvement in different livelihood diversification strategies. Out of the total sampled households, 98.93% are within the active labor force; out of which 14.6%, 8.4%, 25.4%, 25.7%, 8.9%, 16.2%, and 0.8% of households were engaged in On-farm only, Non-farm only, Off-farm only, On-farm + Non-farm, On-farm + Off-farm, Off farm+ Non-farm and On-farm + Off-Farm + nonfarm activity respectively, whereas 1.07% of the sample households are under the age category of >64. Out of which 50%, 25%, and 25% of the respondents diversified their livelihood into On-farm + Off-farm, Off-farm+ Non-farm, and On-farm + Off-farm non-farm livelihood strategies. This result shows that in the study area households within the active labor force diversified their livelihood into different livelihood diversification activities than old aged households. This is because at a younger age (active labor force) households’ choice of a different combination of livelihood strategies will increase while at older ages households became inactive and they are expected to be risk-averse and hence rely more only on their farm livelihood activities. The statistical test result revealed that (χ2 = 31.9; P = 0.000) there was a significant difference between sample household head age and choice of livelihood diversification strategies at 1% probability level. This is consistent with the study by Woldehanna (Citation2000); when household heads get older, they are expected to be less active and hence rely more on-farm activity than on off-farm/non-farm income sources.
4.1.1.4. Household family size
Household family size is one of the expected variables which affect livelihood diversification strategies for urban households. Regarding family structure, the survey data revealed that the maximum family size of sampled households is 10 and the minimum is 1. Table illustrates that the details of sample household family size across different livelihood diversification strategies. The result shows that 48.93%, 49.20%, and 1.87% of the households had family sizes between 1 and 4, 5 and 8, and >9 household members, respectively. To these end, 15.3%, 12.0%, 18.6%, 29.0%, 9.3%, 14.8% and 1.1% of households were engaged into On-farm only, Non-farm only, Off-farm only, On-farm + Non-farm, On-farm + Off-farm, Off farm+ Non-farm and On-farm + Off-farm + Non-farm livelihood activities from household size between 1 and 4 household members. And also, 14.1%, 4.9%, 31.5%, 21.7%, 9.8%, 16.8% and 1.1% of households were engaged On-farm only, Non-farm only, Off-farm only, On-farm + Non-farm, On-farm + Off-farm, Off farm+ Non-farm and On-farm + Off-farm + Non-farm from household size between 5 and 8 household members. This result shows households with large family sizes diversify more than households with small family sizes. This might be to fulfill the family’s basic needs, households with large family sizes engage in different livelihood activities. This shows the household family size impacts the ability of a household to supply labor for diversification activity positively. However, statistical test results indicated that (χ2-value 19.494; P-value = 0.077) there was a statistically significant difference between household family size and their livelihood diversification strategies at a 10% level of significance.
Table 5. Distribution of livelihood diversification by age and family size
4.1.1.5. The educational level of the household head
Education is believed to be an important variable that determines the eagerness of household heads to diversify their livelihood. The results of the survey in the study area in Table show that about 2.41% of household heads did attend (1–4 Grades). Out of them, 22.2%, 0.0%, 33.3%, 11.1% and 11.1% household heads were pursuing On-farm only, Non-farm only, Off-farm only, On-farm + Non-farm, On-farm + Off-farm, Off farm+ Non-farm and On-farm + Off-farm + Non-farm livelihood diversification strategy, respectively. In addition, 13.1% respondents had an educational level of (5–8 grade), out of the 40.8%, 4.1%, 22.4%, 18.4%, 6.1%, 8.2% and 0.0% of the household heads were pursuing On-farm only, Non-farm only, Off-farm only, On-farm + Non-farm, On-farm + Off-farm, Off farm+ Non-farm and On-farm + Off-farm + Non-farm livelihood diversification strategy, respectively. In respect 32.35% respondents had an educational level of (9–12 grade), out of the 11.6%, 10.7%, 22.3%, 28.1%, 9.9%, 16.5% and 0.8% of the household heads were pursuing On-farm only, Non-farm only, Off-farm only, On-farm + Non-farm, On-farm + Off-farm, Off farm+ Non-farm and On-farm + Off-farm + Non-farm livelihood diversification strategy, respectively. The remaining 52.14%, respondents had an educational level of (>12 years of schooling), out of the 9.2%, 8.2%, 27.2%, 26.2%, 9.7%, 17.9% and 1.5% of the household heads were pursuing On-farm only, Non-farm only, Off-farm only, On-farm + Non-farm, On-farm + Off-farm, Off farm+ Non-farm and On-farm + Off-farm + Non-farm livelihood diversification strategy, respectively.
Table 6. Distribution of livelihood diversification by educational level
This indicates that in the study area as the household head’s education level increases the household tends to choose different combination livelihood diversification strategies. The statistical test results (with χ2 = 38.422; P = 0.000) also showed that there was a significant statistical difference among the seven livelihood diversification strategy groups concerning educational level at 1% probability level. This is consistent with the study by Debele and Desta (Citation2016); education is a very important variable that can help households to diversify the urban economy away from agriculture and increase off/non-farm earnings. Education thus has a positive impact on livelihood diversification strategies.
4.1.1.6. Available technical skills of household
It refers to a technical skill that the household has in addition to the main occupation of the household. When the households have technical skills like metal and woodworks/carpenter, house construction, repair services, handicrafts (weaving/spinning of cotton or wool, pottery, and others can diversify their livelihood activities. In the study area out of sampled households, 85.03% of households have technical skills like woodworks/carpenter, house construction, handicrafts, and Pottery. Out of this 14.2%, 7.9%, 25.2%,27.4%, 10.1%, 14.2% and 1.3% of households engaged into On-farm only, Non-farm only, Off-farm only, On-farm + Non-farm, On-farm + Off-farm, Off farm+ Non-farm and On-farm + Off-farm + Non-farm livelihood activities, respectively. And 14.97% of households did not have technical skills. Out of this 16.1%, 10.7%, 25.0%, 14.3%, 5.4%, 28.6% and 0.0% of households engaged into On-farm only, Non-farm only, Off-farm only, On-farm + Non-farm, On-farm + Off-farm, Off farm+ Non-farm and On-farm + Off-farm + Non-farm livelihood activities, respectively (see Table ). This result indicates that in the study area households who have technical skills diversify more than those who did not have available technical skills. Furthermore, the Chi-square result (χ2 = 27.671; P = 0.000) supports that there was a statistically significant difference between household who has the technical skill and do not have in choosing livelihood diversification strategy in the study area at 1% level of significance.
Table 7. Distribution of Livelihood diversification by available technical skill
4.1.2. Institutional characteristics of sample households
4.1.2.1. Access to credit
Credit is one of the most important institutional factors, which help urban households to overcome financial shortage/cash constraints in purchasing inputs and let them participate in different livelihood strategies for the improved wellbeing of the family. The survey results in the study area showed that (see Table ), 45.19% of the sample households received credit from different sources; while 54.81% of them did not. Among the households who get credit access, 8.9%, 14.8%, 27.8%, 26.0%, 7.7%, 13.0% and 1.8% of the households participated into On-farm only, Non-farm only, Off-farm only, On-farm + Non-farm, On-farm + Off-farm, Off farm+ Non-farm and On-farm + Off-farm + Non-farm livelihood diversification strategies respectively. Whereas 54.81% of the households did not get credit access, out of this, 19.0%, 2.9%, 22.9%, 24.9%, 10.7%, 19.0% and 0.5% of households participated into On-farm only, Non-farm only, Off-farm only, On-farm + Non-farm, On-farm + Off-farm, Off farm+ Non-farm and On-farm + Off-farm + Non-farm livelihood diversification strategies, respectively. The result shows that majority of households did not take credit in the study area. According to respondents, the reasons were fear of inability to repay, lack of collateral, and no access to credit. The Chi-square result (χ2 = 27.671; P = 0.000) also revealed that there was a statistically significant difference between credit use and choices of livelihood strategy at a 1% probability level. This is similar to the study by Ambachew & Ermiyas (Citation2016); credit access is found to have a positive impact on the likelihood of choosing livelihood diversification strategies. Access to credit has a positive relation with participation in different livelihood diversification strategies.
Table 8. Distribution of Livelihood diversification by access to credit
4.1.2.2. Training and extension service
Training and extension service plays a great role in teaching, developing new insights, or helping/assisting urban households to adopt improved technologies/practices to improve their production and productivity. The results of the survey in the study area indicated that about 15.24% of the total respondents received training and extension services, whereas 84.76% of sample households replied that they were not received extension services and training in the study area. From those who did not get training and extension service about 15.5%, 7.3%, 27.1%, 22.7%, 9.1%, 17.7% and 0.6% of the households engaged into On-farm only, Non-farm only, Off-farm only, On-farm + Non-farm, On-farm + Off-farm, Off farm+ Non-farm and On-farm + Off-farm + Non-farm livelihood diversification strategies, respectively. However, 8.8%, 14.0%, 14.0%, 40.4%, 10.5%, 8.8% and 3.5% of households engaged into On-farm only, Non-farm only, Off-farm only, On-farm + Non-farm, On-farm + Off-farm, Off farm+ Non-farm and On-farm + Off-farm + Non-farm livelihood diversification activities who received training and extension service (see, Table ). This indicates that in the study area, the urban households who gained training and extension services had more participated in diversified activities because training and extension services provide information and acts that enable households to take advantage of diversification. The Chi-square result (χ2 = 19.57; P = 0.003) also shows in the study area a significant statistical difference between livelihood diversification activities concerning training and extension service at 1% probability level. This finding is similar to the study by Asfaw et al. (Citation2017); the extension service provides the necessary information so that urban households can acquire new skills and knowledge that help to improve production and participate in different livelihood diversification to improve food security.
Table 9. Distribution of livelihood diversification by training and extension services
4.2. Econometric results and discussion
In the econometric analysis, the study applies a multinomial logit model; which is estimated by using the maximum likelihood estimation technique to estimate the parameters of the multinomial logit functions. For effective estimation of the model, various pre-test and post-estimation diagnostics tests were conducted. Thus, the nature of the data set was checked whether it is ready for estimation or not in the next steps. For instance, the study performed tests like multicollinearity test, heteroskedasticity test, independence of irrelevant alternatives test, combination test among the alternatives, and goodness-of-fit test were seriously conducted.
It is critical to check for multicollinearity in the data set before moving on to the basic phases of regression and model interpretation. Thus, the study uses the test of contingency coefficient and variance inflation factors to look for multicollinearity in categorical and continuous explanatory variables. The mean-variance inflation for continuous explanatory variables was less than 10, and the contingency coefficient or correlation coefficient between all categorical explanatory variables was less than 0.85. As a result, both categorical and continuous explanatory variables have a free from multicollinearity problem. Furthermore, the model’s goodness-of-fit was tested using Hosmer Lemeshow, with the results indicating that the model has acceptable fit measures. Finally, independent irrelevance alternatives were tested to whether deleting or removing one alternative outcome variable does not affect the model, the result of the test revealed that adding or removing substitutions from the model does not affect the percentage difference between the remaining results. Therefore, this study confirms that the multinomial logit model is appropriate and applicable. Finally, robust regression has been used to control the problem of heteroskedasticity and non-normality.
4.2.1. Determinants of livelihood diversification strategies
The multinomial logistic regression model was used to identify determinants of livelihood diversification strategies. The model was selected based on the justification illustrated earlier. The dependent variable is the category of urban household’s livelihood diversification strategy, taking a value of “0” if an urban household is pursuing an on-farm livelihood strategy only, a value of “1” if selecting Non-farm only, “2” if selecting Off-farm only, a value of “3” if adopting On-farm + Non-farm activities, “4” if selecting On-farm + Off-farm, a value of “5” if adopting Off-farm + Non-farm and a value of “6” if the choice is On-farm + Off-farm + Non-farm.
The maximum likelihood method was employed to estimate the parameter estimation of the multinomial logit model and statistically significant variables were identified to measure their relative importance in the household’s decision to choose livelihood strategies. The results of the maximum likelihood estimates are presented in Table .
Table 10. Multinomial logit estimation result and the respective marginal effects for livelihood diversification activity choices
The parameter estimates of the multinomial logit model give only the direction of the effect of explanatory variables on the dependent variable, but the estimates neither stand for the actual size of change nor the probabilities (Gujarati, Citation2004). However, the marginal effect measures the expected change in the probability of a given choice that has been made to the unit change in the explanatory variable (Greene, Citation2000). The result of the multinomial logistic regression model and marginal effects are explained as follows:
4.2.1.1. Model results from the interpretation
Table represents the multinomial logit estimation results and the corresponding marginal effects of the livelihood diversification activities. Since there are seven alternatives, the first alternative (On-farm only) is serving as a base or reference category and the remaining alternative’s result is interpreted regarding the base category. Thus, interpretations for significant variables are discussed in the following sections.
Sex of the household head (SEX)
Opposed to prior expectation, the sex of the household head negatively and significantly determined engagement of livelihood diversification strategies into non-farm at 10% probability level of significance. The result implies that those male-headed households are more probably engaged in an on-farm only livelihood strategy and less probably engaged in a non-farm livelihood diversification strategy. Marginal effect results of the model demonstrated that other variables being kept constant, the likelihood of adopting the non-farm strategy in favor of male households decreases by 11.1%, and the opposite is true for female-headed households regarding the on-farm only strategy. However, the sex of the household head positively and significantly determined engagement of livelihood diversification strategies into On-farm + Non-farm at a 1% probability level of significance. The result implies that male-headed households are more likely to diversify their livelihood strategies into On-farm + Non-farm than female-headed households. On the other hand, female-headed households are less likely to diversify their livelihood strategies. The possible reasons are due to cultural factors that females are assigned to care for the child, perform at home works and are busy with other social roles. Marginal effect results of the model demonstrated that other variables being kept constant, the likelihood of male-headed household’s choice of livelihood diversification into On-farm + Non-farm increase by 22.6% than the female household head. This is consistent with the study by Ambachew and Ermiyas (Citation2016), male-headed households are more participated than the female-headed households head because in developing countries females are constrained by cultural challenges and they engaged themselves in house activities.
Age of the household head
As expected, the age of the household influenced positively and significantly the choice of Off-farm + Non-farm at a 1% probability level of significance. Interpretation of the marginal effect implies that if other factors are held constant. As the age of household heads increased the chance that they would positively diversify the likelihood to choose Off-farm+ Non-farm increased by 0.7%. The probable reason is that as the age of the households get older, they are relatively better educated, have better access to technologies, and look for alternative Off-farm/Non-farm livelihood opportunities. This result is consistent with the finding of (Debele & Desta, Citation2016); the age of the household head influenced positively and significantly the household’s livelihood diversification strategy.
Education status of household head
Education level has a positive and significant at 1% level of significance, relationship with household’s livelihood diversification into Non-farm only and Off-farm only, respectively. The marginal effect revealed that the likelihood of a household diversifying into Non-farm only and Off-farm only activities increased by 0.5% and 1% respectively for those urban households with more level of education. In addition, education is positively and significantly related to the household’s livelihood diversification into both (On-farm + Non-farm, On-farm + Off-farm), Off-farm + Non-farm and On-farm + Off-farm + Non-farm activities at 5%, 10%, and 1% probability levels of significance, respectively. The result of the marginal effect depicts that, keeping other factors constant, the likelihood in favor of the households to choose On-farm + Non-farm, On-farm + Off-farm, Off-farm + Non-farm, and combination of On-farm + Off-farm+ Non-farm livelihood diversification strategies will increase by 0.1%, 0.2%, 5.1%, and 0.1% respectively, as the education level of the household increases by 1 year. This indicated that those farmers with high educational levels are more likely to diversify livelihood strategies into all livelihood diversification activities. This implies that the highly educated persons diversify their livelihood options through opting for salaried jobs, self-employment activities, food-for-work programs/public work, commercial activities, artisan activities, handicrafts, tourism, and hotel-related services, unearned income sources (pension, interest, and dividends/from membership of organizations). The result is consistent with those of hree studies (Eshetu & Mekonnen, Citation2016; Gebru et al., Citation2018; Gebreyesus, Citation2016), which found that the educational level of the household has a positive impact on livelihood diversification.
Family size
The study found that household size had positively and significantly affected the choice of Off-farm only, Off-farm + Non-farm, and On-farm + Off-farm + Non-farm at 1% and equally 10% significance level. The marginal effect depicted that ceteris paribus, the likelihood of households to diversify into Off-farm only, Off-farm + Non-farm, and On-farm + Off-farm + Non-farm livelihood strategies increases by 4.6%, 0.9%, and 0.1% respectively, as household size increases by one relative to the base On-farm only. Hence, households with large family sizes are more likely to participate in non/off-farm and/or a combination of activities. The positive association between household size and diversification might be due to the relation between large family size and household labor as well as the corresponding demand for food. The result of this study is in line with the findings of Bird et al. (Citation2022); Adepoju & Obayelu (Citation2013) and Tamerat (Citation2016), while it contradicts from the study done by Gebru et al. (Citation2018); large household size does not mean all the household members are productive labor force. This is since some of the household members.
Training and extension service
As expected earlier, this variable has a positive and significant effect on livelihood diversification into Non-farm only, On-farm + Non-farm, and On-farm + Off-farm + Non-farm livelihood strategies at a 5% probability level of significance. This shows households who get training and extension service are more likely to be engaged in different combinations of livelihood diversification strategies. The marginal effect results of the model showed that, if other factors being constant, the likelihood of diversifying into Non-farm only, On-farm + Non-farm, and On-farm + Off-farm + Non-farm livelihood activities increased by 7.8%, 18.4%, and 0.1% respectively for those who gained training and extension service than the contrary. It is in line with the study by Asfaw et al. (Citation2017) and Eneyew & Bekele (Citation2012).
Market access
As expected, market access has a positive and significantly affected the diversification of livelihood into (On-farm + Non-farm, O-farm + Non-farm + Off-farm) activities at 1% and Off-farm + Non-farm activities at 5% level of significance. This result suggests that a household residing the less far distance to market is more likely to diversify the livelihood strategies into On-farm + Non-farm, Off-farm + Non-farm, and On-farm + Off-farm + Non-farm activities. According to the study, keeping all other variables in the model held constant, the likelihood of diversifying the livelihoods into On-farm + Non-farm, Off-farm + Non-farm, and On-farm + Off-farm + Non-farm activities increase by 18.4%, 15.6%, and 0.1%, respectively. The possible reason for the result could be, that households less far from market places have easy and quick physical access to the market, to transport output and input from and to their residence and the chance of wage labor, and small business (petty trade) and other off-farm and non-farm activities are encouraging as the result diversification is increasing. This is in line with the study by Lorato (Citation2019); households who are living around market areas can diversify their sources of income than those households living far from market areas. However, it contradicts the result of Gebru et al. (Citation2018); as the distance from the household’s home to the market center increases by 1 km. The more households are distant from the market center, the more disadvantaged from diversifying their livelihood income into non-farm options.
Access to credit
As expected it was found to have a positive and significant effect on the level of livelihood diversification strategies into Non-farm only and On-farm + Off-farm + Non-farm livelihood activities at 1% probability level. This indicated that households who get credit access are more likely to be engaged in different livelihood activities than those who did not get it. The marginal effects result of the model revealed that other thing being constant, the likelihood of choosing Non-farm only and On-farm + Off-farm + Non-farm activities increased by 11.9% and 0.1% respectively for those who get credit access to those who did not. In the study area, those who get credit access had diversified their livelihood activities than contrary. Also, credit access was positive and significant with urban households’ likelihood of diversifying their livelihood strategies into Off-farm only and On-farm + Non-farm income activities at a 5% level of significance. Keeping other factors constant, the likelihood in favor of the urban households to choose Off-farm only and a combination of (On-farm + Non-farm), livelihood diversification strategies increases by 4.5% and 2.9% respectively as access to credit increases by one. This finding is in line with the study by Bayu & Gondar (Citation2021); Debele & Desta (Citation2016); and Mentamo & Geda (Citation2016); credit access is found to have a positive impact on the likelihood of choosing livelihood diversification strategies (Citation2017; Access to credit service was found to have a positive effect on livelihood diversification. Hence, providing credit for resource-poor households will enhance livelihood diversification.
Dependency ratio
In contrary to our hypothesis, the dependency ratio was found to have a negative relationship with Off-farm + Non-farm choices of household livelihood diversification strategies and was statistically significant at a 5% probability level. This implies that households with a high dependency ratio have a low probability level to participate in off-farm and non-farm income-generating livelihood diversification strategies. The possible explanation for this could be attributed to the fact that the availability of an increased number of individuals whose age is below 15 and above 64 who are unable to engage themselves in some activities. This means a shortage of working hands to earn from diversified activities for meeting household needs. The marginal effect shows that compared to the base category (On-farm only), an increase in the dependency ratio significantly leads to a 3.8% reduction in the adoption Off-farm + Non-farm livelihood diversification activities. The result of this study is consistent with the finding obtained by Amevenku et al. (Citation2019) and Khatun & Roy (Citation2012).
5. Conclusion and Recommendation
5.1. Conclusion
This study undertook an appraisal of the livelihood strategies for urban development in the North Shewa Xone, Ethiopia. The study employed a cross-sectional survey with qualitative and quantitative methods. Both primary and secondary sources of data were used. The multistage sampling technique was used to select 374 sampled respondent households from three urban areas namely; Debre Berhan, Shewarobit, and Mehal Meda. Descriptive statistics and a multinomial logit regression model were used to identify the livelihood diversification strategies pursued by urban households in the study area.
A multinomial logistic regression model was employed to analyze the determinant of livelihood diversification strategies in the study area. The results of the model revealed that out of 10 explanatory variables included in the model, 8 explanatory variables were found to be signed up to less than 10% probability level. Livelihood diversification strategies were determined by the Age of the household head, education status, family size, credit access, market access, and training and extension service positively and the dependency ratio was negative. The sex of household head positively and significantly determined engagement of livelihood diversification strategies into On-farm + Non-farm at 1% probability level of significance. The age of the household head influenced positively and significantly the choice of Off-farm +Non-farm at 1% probability level of significance. Education level has a positive and significant at 1% level of significance, relationship with household’s livelihood diversification into Non-farm only and Off-farm only, respectively. In addition, education is positively and significantly related to the household’s livelihood diversification into On-farm + Non-farm, Off-farm + Non-farm, and On-farm + Off-farm + Non-farm activities at 5%, 10%, and 1% probability levels of significance, respectively. The study found that household size had positively and significantly affected the choice at 1% and equally 10% significance level. Training and extension services have a positive and significant effect on livelihood diversification into Non-farm only, On-farm + Off-farm, and On-farm + Off-farm + Non-farm livelihood strategies at a 5% probability level of significance. Market access has a positive and significantly affected the diversification of livelihood into (On-farm + Non-farm, On-farm + Non-farm + Off-farm) activities at 1% and Off-farm + Non-farm activities at 5% level of significance. Access to credit services has a positive and significant effect on the level of livelihood diversification strategies into Non-farm only and On-farm + Off-farm + Non-farm livelihood activities at a 1% probability level.
However, the sex of the household head negatively and significantly determined engagement of livelihood diversification strategies in non-farm at 10% probability level of significance. Dependency ratio was found to have a negative relationship with Off-farm + Non-farm choices of household livelihood diversification strategies and statistically significant at 5% probability level. Therefore, it might be concluded that livelihood diversification strategies in the study area were determined by the sex of the household head, age of the household head, education status, family size, credit access, market access, and training and extension service positively, whereas dependency ratio determined negatively.
5.2. Recommendation
Based on the above finding, this study was recommended the following implications from multiple perspectives:
From an individual perspective; targeted intervention should be needed for the female-headed households to enable and empower them to participate in different livelihood diversification activities. Aged households should improve their choice to participate in non-farm/off-farm activities besides farm activity. From the society or local community perspective; it is recommended that awareness for reducing dependency ratio should be created. Dependency ratio could be reduced in two ways: firstly, members of the young age population (below 15 years old) took part in increasing the labor force participation activities in Off-farm + Non-farm as needed. Secondly, providing opportunities for the elderly (above 65 years old) to remain in the workforce longer as well as engage in volunteering, care, and artistic activities and tourism and hotel-related services can provide both social and economic benefits and relieve some of the fiscal pressures related to aging societies
From an institutional perspective; banks and microfinance institutions must give credit access to urban households. Because getting credit from the bank and microfinance institutions will open opportunities for many urban households to diversify their livelihood diversification strategies. From governmental (policymakers) perspectives, the government of Ethiopia should develop a comprehensive urban development policy that could empower off-farm and non-farm urban livelihood diversification strategies besides farm activities. The government and non-governmental organizations should provide training and extension services to urban households and monitoring of extension agents needed. The education level of urban households in the study area was found to be one of the important determinants of livelihood diversification strategy. Thus, the government should focus and invest in the education sector. With regard to access to market, the government should make the market for buying or selling inputs or outputs near to the urban households. In general livelihood, diversification is a key strategy taking place at different levels of the economy, which are usually, but not always directly linked. Thus, in the study area, a diversified economic activity provides an opportunity to manage household food security, reduce poverty, increase living conditions of the society in particular, and improves the Ethiopian economy as a whole.
5.3. Limitations and areas for further research
As this study used cross-sectional data, it is limited to showing the time effect of each livelihood diversification strategy. Future research is recommended for examining the impact of livelihood diversification strategies by using panel data.
Ethical approval
The ethical committee of Debre Berhan University has given permission to conduct the study. Oral approval was obtained from the study respondents.
Acknowledgment
We would like the editor and anonymous reviewers for their supportive comments and suggestions.
Disclosure statement
No potential conflict of interest was reported by the author(s).
Additional information
Funding
Notes on contributors
Girma Mulugeta Emeru
Girma Mulugeta Emeru is a Lecturer in the Department of Economics at Debre Berhan University. His area of interest lies in researching the livelihood diversification strategies, women labor force participation, banking and microfinance, poverty, and food security.
Abebaw Hailu Fikire
Abebaw Hailu Fikire is a lecturer at Debre Berhan University, Ethiopia. His current research interests are development issues like urban and rural development, agricultural economics, food security microeconomic, and macroeconomics policy analysis.
Zemenu Bires Beza
Zemenu Bires Beza is a lecturer at Debre Berhan University. His research interest includes tourism management.
References
- Adepoju, A. O., & Obayelu, O. A. (2013). Livelihood diversification and welfare of rural households in Ondo State, Nigeria. Journal of Development and Agricultural Economics, 5(12), 482–29. https://doi.org/10.5897/JDAE2013.0497
- Admasu, T. T., Damtie, Y. A., & Taye, M. A. (2022). Determinants of livelihood diversification among households in the Sub-Saharan Town of Merawi, Ethiopia. Advances in Agriculture, 2022. Article ID 6600178. https://doi.org/10.1155/2022/6600178
- Alobo, S., & Bignebat, C. (2017). Patterns and determinants of household income diversification in rural Senegal and Kenya. Journal of Poverty Alleviation and International Development, 8(1), 93–126.
- Ambachew, M. D., & Ermiyas, A. (2016). Determinants of rural household livelihood diversification strategy in South Gondar Zone, Ethiopia. Journal of Agricultural Economics, Extension and Rural Development, 4(8), 548–560.
- Ambaye, T. K., Tsehay, A. S., & Hailu, A. G. (2021, April 6). Analysis of the status and determinants of rural households’ access to agricultural extension services: The case ff Jimma Geneti Woreda, Oromia Regional State, Ethiopia. International Journal of Agricultural Extension and Rural Development Studies, 8(1), 52–99. https://ssrn.com/abstract=3820302
- Amevenku, F. K. Y., Asravor, R. K., & Kuwornu, J. K. M. (2019). Determinants of livelihood strategies of fishing households in the volta Basin, Ghana. In Cogent Economics & Finance. 7, 1. https://doi.org/10.1080/23322039.2019.1595291
- Asfaw, A., Simane, B., Hassen, A., & Bantider, A. (2017). Determinants of non-farm livelihood diversification: Evidence from rainfed-dependent smallholder farmers in northcentral Ethiopia (Woleka sub-basin). Development Studies Research, 4(1), 22–36. https://doi.org/10.1080/21665095.2017.1413411
- Ayana, G. F., Megento, T. L., & Kussa, F. G. (2021). The extent of livelihood diversification on the determinants of livelihood diversification in Assosa Woreda, Western Ethiopia. GeoJournal. https://doi.org/10.1007/s10708-021-10379-5
- Bayu, E. K., & Gondar, E. (2021). Determinants variables for women’s participation in non-agricultural livelihood diversification strategies in Ethiopia: A logistic regression model analysis.
- Bird, K., Chabé-Ferret, B., & Simons, A. (2022). Linking human capabilities with livelihood strategies to speed poverty reduction: Evidence from Rwanda. World Development, 151, 105728. https://doi.org/10.1016/j.worlddev.2021.105728
- Bowen, S., & De Master, K. (2011). New rural livelihoods or museums of production? Quality food initiatives in practice. Journal of Rural Studies, 27(1), 73–82. https://doi.org/10.1016/j.jrurstud.2010.08.002
- Central Statistical Agency of Ethiopia (CSA). (2014). National census of Ethiopia. Ministry of Finance and Economic Development.
- Debele, B. N., & Desta, G. D. (2016). Livelihood diversification: Strategies, determinants and challenges for pastoral and agro-pastoral communities of Bale Zone, Ethiopia. International Review of Social Sciences and Humanities, 11(2), 37–51.
- Dirar, S. A., Berhanu, T., Getachew, D., & Azadi, H. (2017). Financial product development and members’ voluntary saving behavior in saving and credit cooperatives in Tigray: A case study in Endamohoni Woreda. Cogent Economics & Finance, 5(1). https://doi.org/10.1080/23322039.2017.1279864
- Dirribsa, S., & Tassew, B. (2015). Analysis of livelihood diversification strategy of rural household: A case study of Ambo District, Oomiya Regional State, Ethiopia. Department of cooperatives & institute of cooperatives & development studies, Ambo University, Oomiya Regional State, Ethiopia. International Journal of Current Research and Academic Review, 3(8), 406–426.
- Echebiri, R. N., Onwusiribe, C. N., & Nwaogu, D. C. (2017). Effect of livelihood diversification on food security status of rural farm households in Abia State Nigeria. Scientific paper series management. Economic Engineering in Agriculture and Rural Development, 17(2017), 1–8.
- El-Habil, A. M. (2012). An application on multinomial logistic regression model. In Pakistan journal of statistics and operation research, 8(2), 271-291. https://doi.org/10.18187/pjsor.v8i2.234
- Ellis, F. (1998). Household strategies and rural livelihood diversification. The Journal of Development Studies, 35(1), 1–38. http://doi.org/10.1080/00220389808422553
- Ellis, F. (2003). A livelihoods approach to migration and poverty reduction.
- Ellis, F., & Allison, E. (2004). Livelihood diversification and natural resource access. Overseas Development Group, University of East Anglia.
- Eneyew, A., & Bekele, W. (2012). Determinants of livelihood strategies in Wolaita, southern Ethiopia. Agricultural Research and Reviews, 1(5), 153–161.
- Eshetu, F., & Mekonnen, E. (2016). Determinants of off farm income diversification and its effect on rural household poverty in Gamo Gofa Zone, Southern Ethiopia. Journal of Development and Agricultural Economics, 8(10), 215–227. https://doi.org/10.5897/JDAE2016-0736
- Gebreyesus, B. (2016). Determinants of livelihood diversification: The case of Kembata Tambaro Zone, Southern Ethiopia. Journal of Poverty, Investment and Development, 23, 1–10.
- Gebru, G. W., Ichoku, H. E., & Phil-Eze, P. O. (2018). Determinants of livelihood diversification strategies in Eastern Tigray Region of Ethiopia. Agriculture and Food Security, 7(1), 62. https://doi.org/10.1186/s40066-018-0214-0
- Getinet, B., & Lorato, T. (2020). The role of rural livelihood diversification strategies for household food security in Southern Ethiopia: Empirical Evidence from Kecha Bira District of Kembata Tembaro Zone. Journal of Finance and Economics, 8(3), 142–151. https://doi.org/10.12691/jfe-8-3-7
- Greene, W. H. (2000). Econometric analysis (4th ed.). International edition. Prentice Hall.
- Greene, W. H. (2008). The econometric approach to efficiency analysis. The measurement of productive efficiency and productivity growth, 1(1), 92–250.
- Guite, S., Sharma, H. I., & Thoudam, L. (2022). Determinants of Livelihood Diversification among the Thadou-Kukis of Manipur, India. Economic Affairs, 67(1s), 79–86. https://doi.org/10.46852/0424-2513.1.2022.15
- Gujarati. (2004). Basic econometrics (4th ed.). The Mcgraw-Hill.
- Hausman, J. A. (1978). Specification tests in econometrics. In Econometrica: Journal of the econometric society, 46(6), 1251–1271. https://doi.org/10.2307/1913827
- Helmy, I. (2020). Livelihood diversification strategies: Resisting vulnerability in Egypt (No. 441). GLO Discussion Paper.
- Kassie, G. W., Kim, S., & Fellizar, F. P., Jr. (2017). Determinant factors of livelihood diversification: Evidence from Ethiopia. Cogent Social Sciences, 3(1), 1–16. https://doi.org/10.1080/23311886.2017.1369490
- Khatun, D., & Roy, B. C. (2012). Rural livelihood diversification in West Bengal: Determinants and Lavrakas, P. J. (2008). Encyclopedia of survey research methods (Vols. 1-0). Agricultural Economics Research Review, 25(347-2016-16910), 115-124. Sage Publications, Inc. https://doi.org/10.4135/9781412963947constraints
- Lavrakas, P. J. (2008). Encyclopedia of survey research methods (Vols. 1-0). Sage Publications, Inc. https://doi.org/10.4135/9781412963947
- Lorato, T. (2019). The determinants of livelihood diversification strategies in Qecha Bira Woreda, Kambata Tambaro Zone, Southern Ethiopia. Journal of Economics and Sustainable Development, 10(9), 6–14.
- Maddala, G. S. (1986). Limited-dependent and qualitative variables in econometrics (No. 3). Cambridge university press.
- Mekuria, W., & Mekonnen, K. (2018). Determinants of crop–livestock diversification in the mixed farming systems: Evidence from central highlands of Ethiopia. Agriculture and Food Security, 7(1), 1–15. https://doi.org/10.1186/s40066-018-0212-2
- Mentamo, M., & Geda, N. R. (2016). Livelihood diversification under severe food insecurity scenario among smallholder farmers in Kadida Gamela District, Southern Ethiopia. Kontakt, 18(4), e258–e264. https://doi.org/10.1016/j.kontakt.2016.09.003
- Mulugeta, M. S. (2009). Determinants of livelihood strategies of urban women: The case of female household heads in Wolenchiti Town, Ethiopia. Research and Perspectives on Development Practice, 6, 2009–6976.
- Muluneh, D. (2022). Determinants of urban household income diversification and its relation to food security in Yejube Town, Amhara National Regional State [Doctoral dissertation].
- Musumba, M., Palm, C. A., Komarek, A. M., Mutuo, P. K., & Kaya, B. (2022). Household livelihood diversification in rural Africa. In Agricultural economics, 53(2), 246-256.
- Njuguna, R. A. (2015). Determinants of choice of alternative livelihood diversification strategies in Solio Resettlement Scheme [ Doctoral dissertation, MSc. Thesis, Department of Agricultural Economics].
- Saha, B., & Bahal, R. (2012). Constraints impeding livelihood diversification of farmers in West Bengal. Indian Research Journal of Extension Education, 12(2), 59–63.
- Shikur, S., Haji, J., & Leza, T. (2021). Factors affecting choice of livelihood strategies in Peri-Urban Communities of Hossana Town, Southern Ethiopia. East African Journal of Sciences, 15(2), 129–140.
- Tambe, S. (2022). Sustainable livelihoods approach. In Teaching and learning rural livelihoods. sustainable development goals series. Cham: Springer. https://doi.org/10.1007/978-3-030-90491-3_4
- Tamerat, T., & Borah, D. (2016). Food Security Condition of Smallholding Farmers in Kilte Awelalo.
- Teshome, B., & Edriss, A. K. (2013). Determinants and patterns of income diversification among smallholder farmers in Akaki district, Ethiopia (No. 634-2016-41518).
- Washo, J. A., Tolosa, S. F., & Debsu, J. K. (2021). Determinants of rural households’ livelihood diversification decision: The case of Didessa and Bedelle District, Bunno Bedelle Zone, Oromia Regional State, Ethiopia. African Journal of Agricultural Research, 17(12), 1573–1580.
- Wegedie, K. T. (2018). Determinants of peri-urban households’ livelihood strategy choices: An empirical study of Bahir Dar city, Ethiopia. Cogent Social Sciences, 4(1), https://doi.org/10.1080/23311886.2018.1562508
- Woldehanna, T. (2000). Economic analysis and policy implications of farm and off-farm employment: A case study in the Tigray region of Northern Ethiopia. Wageningen University and Research.
- Yamane, T. (1973). Statistics: an introductory. analysis–3.
- Yizengaw, Y. S., Okoyo, E. N., & Beyene, F. (2015). Determinants of livelihood diversification strategies: The case of smallholder rural farm households in Debre Elias Woreda, East Gojjam Zone, Ethiopia. African Journal of Agricultural Research, 10(19), 1998–2013. https://doi.org/10.5897/AJAR2014.9192