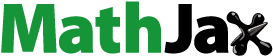
Abstract
The ongoing climate change is vital to exert context-specific responses to allay its adverse effects. The purpose of this study is to examine the perception and determinants of agricultural technology adaptation of teff producers to climate change in North Shewa zone, Ethiopia. Data were collected from 378 farming households through a multistage and simple proportional sampling procedure. Both descriptive and econometric analyses were used in this study. A Heckman probit model and a multinomial logit (MNL) model are used to examine the determinants of perception and adaptation to climate change, respectively. The results of the Heckman probit model revealed that gender, farm experience, access to extension services and information access were found to have a significant influence on the probability of farmers to perceive and/or adapt to climate change. The multinomial logit models showed that education, gender of the household head being male, marital status, farming experience, income, access to information, livestock ownership, tenure status, and access to extension are the main factors that increase adaptive capacity. However, distance to the nearest market and family size negatively and significantly affect the decision to adopt various agricultural technologies. This study recommends that, Future development initiatives should focus on enhencing perception and scaling up climate change adaption technology, which calls for a public-private collaboration and a shared vision of all potential stakeholders.
PUBLIC INTEREST STATEMENT
Currently in Ethiopia, the perception of climate change and adoption of modern agricultural technology are very low. Therefore, this study intends to examine the perception and determinants of agricultural technology adaptation of teff producers to climate change in North Shewa zone. Results showed that gender, marital status, tropical livestock units, distance to market, visit of extension, and cultivated land size are the major determinants of agricultural technology adoption.
1. Introduction
Global climate change is the major challenge imposing irreversible damages on humans’ livelihoods in the twenty-first century (Mohammed et al., Citation2022). The impacts of climate change on the agricultural sector have prompted concern over the magnitude of future global food production (Jahan & Qale Nawi, Citation2022). Climate change is currently considered one of the most important challenges facing workers in the field of crop science and plant breeding in the world (Awaad, Citation2022). The projected changes in climate and increasing climatic risks over the twenty-first century pose serious challenges to agricultural development in developing countries including Pakistan, as cited in Fahad and Jing (Citation2018). Farmers’ risk behaviour towards flood and their willingness to pay (WTP) for flood insurance were taken into consideration as measures for agricultural sustainability and climate change adaptation (Hossain et al., Citation2022).
Agricultural productivity in sub-Saharan Africa (SSA) remains low and is falling farther behind other regions of the world (Shuaibu & Nchake, Citation2021). Agricultural production of perennial crops under current crop management practices, therefore poses, numerous challenges in terms of productivity and profitability as well as environmental degradation (Heidenreich et al., Citation2022). In most developing countries, rising temperature and changing perception rates will most likely hamper success of rain-fed agriculture (Change, Citation2014). Climate change affects farming livelihoods by acting as a hunger-risk multiplier by damaging harvests and lowering crop yields, thereby increasing poverty and food insecurity (Nciizah et al., Citation2022). Climate change severely affects sub-Saharan African economies in several ways: increased temperatures, erratic rainfall variability patterns, and recurrent droughts and floods. Such adverse climate change effects may result in a greater incidence of crop pests, loss of soil moisture content, rapid soil nutrient depletion, and substantial decreases in crop productivity and yields (Bedeke, Citation2022). According to Fahad and Jing (Citation2018), farmers face various constraints in the adoption of certain adaptation measures to deal with climate variability; shortage of labor, insecure land tenure system, lack of market access, poverty, land of governmental support, lack of access to assets, lack of water sources, lack of credit sources, and lack of knowledge and information were the main constraints faced by the farm households.
Ethiopia is identified as one of the poorest country in the world by all standard measures of poverty and faces numerous development challenges that exacerbate its vulnerability to climate change (Sustainable Development Goals Center for Africa (SDGC/A), Citation2019). A great majority of Ethiopia’s poor people reside in rural areas and depend on agriculture for their livelihoods (Tabe Ojong et al., Citation2022). The country’s GDP rises or falls about a year following changes in average rainfall (Berihun & Van Steven, Citation2021). Climate change is already impacting all agricultural sectors by reducing production capabilities as well as by increasing production risks (Syed et al., Citation2022). Effects of global climate change on teff productivity have an influence on Ethiopian food security (Yumbya et al., Citation2014). Fluctuations of climatic parameters are becoming major concerns for teff production as they affect the production and productivity negatively in recent years (Destaw & Fenta, Citation2021).
Studies described that adaptation is the most appropriate and responsive strategy for farmers to minimize the adverse climate change impacts (Kiani et al., Citation2021, as cited in Kamau, Citation2010; Pickson et al., Citation2021). Adaptation in rain-fed teff production can be seen as a promising entry point to cope up with the consequences of climate change amongst the poorest of the poor (Negra, Citation2014). The human response is critical to perceiving and estimating the effects of climate change on production and food supply for abating these negative impacts through adaptation (Ali et al., Citation2017). It requires involvement and coordinated efforts of the local community, and hence, it is important to gain a better understanding of farmers’ perceptions to climate change, the adaptation options to the current drought circumstances, and what limits their actions upon droughts and climate change (Azhoni & Goyal, Citation2018).
Adaptation to climate change needs to be seen as an integral part of a country’s development planning, rather than as a separate issue (Chambwera, Citation2010). There are many studies that have investigated the impacts of climate change on agriculture and possible adaption measures using different models globally (Khanal et al., Citation2021; Warsame et al., Citation2021; Gunawat et al., Citation2022; Karimi et al., Citation2018). Thus, adaptation is one of the man-made efforts that need due attention in the agricultural sector within the endeavour of poverty reduction basing the fact that food production is inherently sensitive to climate and strong inter-linkages exist between climate, agriculture, and economic growth and development. Although Minjar Shenkora and Moretna Jiru districts have high potential for teff production (Giziew & Mebrate, Citation2019), their adoption strategies for climate change are very low. In order to improve the teff varieties in the Minjar Shenkora and Moretna Jiru districts, which are expected to have higher yield under the prevailing climatic change, these locations must be thoroughly explored.
Thus, this study is inspired to gain more insight to improve the perception of climate change with teff cultivation and options to adapt agricultural technology in North Shewa zone, Ethiopia. The contribution of the study to the existing literature is threefold. First, most of the studies looked into the impacts of climate change in Ethiopia in general or emphasized on nationwide circumstances, for example (Gebreegziabher et al., Citation2011; Robinson et al., Citation2012; Solomon et al., Citation2021). However, this study examined the determinant of agricultural technology adoption and implied the impacts on climate change directed to specific cite and crop. Second, there have been few studies to address the economic impact of climate change on Ethiopian agriculture and farm-level adaptations that farmers make to mitigate the potential impact of climate change (Deressa et al., Citation2009; Muluneh, Citation2020; Asmare et al., Citation2019; Mekonnen et al., Citation2021), and there is also a general agreement that the climate change impacts will be different for the different agro-ecological zones (Deressa et al., Citation2009; World Bank, Citation2008; Owusu et al., Citation2021; Ginbo, Citation2022). Given this, no empirical study has been conducted to examine the perception of farmers to climate change in the study area to date to the best of the researchers’ knowledge.
Third, there is also a methodological gap in addressing the issue on how farmers adapt to climate change in Ethiopia, for example (Baylie & Fogarassy, Citation2021; Redda et al., Citation2022; Tesfaye & Nayak, Citation2022). Baylie and Fogarassy (Citation2021) employed the Ricardian approach to estimate the impacts on the net crop income. Even though the applied Ricardian approach includes adaptation, it does not explicitly address how farmers perceive and what adaptation methods they employ. Redda et al. (Citation2022) done a study entitled on perception and determinants of adoption decisions by employing multinomial logit model, but this paper focuses on the determinants and failed to explain how households perceive to climate change. Tesfaye and Nayak (Citation2022) applied a multivariate probit regression model and identified the factors determining farm households’ adoption of climate change adaptation options, but most investigations have not given due attention to farmers’ understanding about climate change. In this regard, this study employed the combination of Hickman selection probit model and multinomial logit model. Based on the above-mentioned research gap, the study mainly focuses on the perception and determinants of agricultural technology adaptation of teff producers to climate change in North Shewa zone, Amhara Regional State, Ethiopia. Considering the problem, this study addresses the following research questions: What are the factors for farmers’ perception to the existing climate change? What are the major adaptation strategies to climate change adopted by farmers?
The study was organized into five parts in which the first part of the study deals with introduction; the second part deals with review of the related literature; the third part deals with research methodology; the fourth part deals with result and discussion; and the final part of the study deals with conclusion and recommendations.
2. Review literature
2.1. Empirical studies and the conceptual framework
Different studies have been undertaken by scholars to understand household’s perception to climate change, to explore adaptation strategies in response to these changes, and to investigate the determinants of perception and choice of adaptation methods. The following table shows different related empirical literatures regarding climate change perception in Ethiopia.
2.1.1. Conceptual framework
Based on theoretical concepts and related studies, determinant factors for climate change adaptation were hypothesized to be demographic, socioeconomic, and institutional features of the households (). Demographic features such as being male, age, educational status, and family size of the household head were assumed to have a positive effect on adaptation decision (A. Asfaw et al., Citation2019). Likewise, socioeconomic structures of households such as income of the household, livestock ownerships, and information access were hypothesized to influence farmers’ adaptation decision positively (Osumanu et al., Citation2017). Institutional features such as land tenure security, credit, and access to agricultural extension workers were supposed to have a positive effect on climate change adaptation (Mihiretu et al., Citation2020). Nonetheless, among some factors, distance to market and age were expected to have a negative impact on adaptation decision (Milkias & Abdulahi, Citation2018).
Figure 1. Conceptual framework.
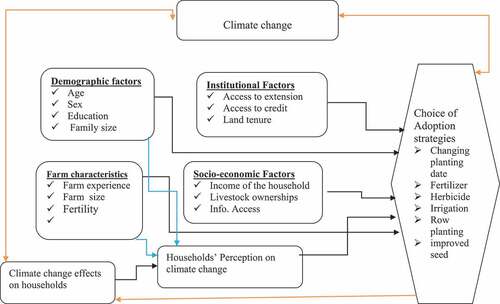
3. Research methodology
3.1. Description of the study area
The study was carried out in the North Shoa Administrative Zones of Amhara Regional State. North Shewa zone is one of the 10 zones of Amhara National Regional State of Ethiopia. This zone is bordered on the south and west by the Oromia region, on the north part by South Wollo and northeast by Oromia zone, and the east by Afar regions. The capital city of North Shewa zone is Debre Berhan, 130 km to the northeast of Addis Ababa. Geographically, the zone is located between 8° 38’ to 10°42’ N and 38°40’ to 40°03’ E, and it consists of 22 rural districts (North Shewa Zone Administration office, Citation2022). According to CSA (Citation2007), the estimated population size of North Shewa zone is 2.16 million and 50.5% of which is male and 49.5% is female. The majority of the population is Amhara ethnic group (95.7%) where the Oromo (2.14%), the Argobba (1.71%), and other ethnic groups (0.45%) also reside in the zone. The dominantly practiced religion is Orthodox Christianity. The zone has a total surface area of about 16,193.6 square kilometres, comprising the highland masses in the west and the lowlands in the east. The topography of the area is characterized by flat to undulating and hilly landscapes, with contrasting tropical, subtropical, and temperate climates. From the total of 22 districts and 5 town administrations, 2 districts, Minjar Shenkora (39° 46` 54” E and 9° 6’ 54” N) and Moretna Jiru (39° 19’ 24” E and 10° 6’ 2”N), were purposely selected based on the potential production of teff.
3.2. Source of data and data collection method
This study is based on a household-level cross-sectional data collected from north Shewa zone farmers in the year of 2020/2021. The data for this study were collected from both primary and secondary sources. The primary data were collected from small-holder teff-producing sample respondents in Minjar Shenkora and Moretna Jiru districts. The data collected through questionnaire give information about the variables of interest and were chosen based on literatures and availability of data.
In addition to the primary data, secondary sources, specifically about temperature and rainfall, were needed so as to look into the trends of climate change in the past years. From the reviewed literatures, trends of temperature and rainfall distributions are treated as the proxy variables for explaining trends on climate. Accordingly, we can examine the coincidence of climate trends from meteorological stations and farmers’ perception on climate change by comparing the data from the two sides. For this matter, metrological stations were consulted.
3.3. Sampling procedure
Targeting at farmers, a multi-stage and hybrid of random and purposive sampling technique was employed to elicit the desired information based on the proportion of size. A multi-stage sampling technique was used to select sample respondent households. In the first phase, two potential teff grower districts, namely, Minjar Shenkora and Moretna Jiru, were selected purposively. In the second stage, six potential kebeles,Footnote1 three from each of the two districts, were selected. In the third phase, proportionally with population size, sample households were selected using simple random sampling techniques from each of the selected kebeles.
Then, proportional sampling was used to take the sample size from each stratum, and this is because to avoid the problem of bias and to represent each stratum adequately. Finally, from each stratum of the randomly selected kebeles, 378 representative sample respondents were selected randomly through taking probability proportional to size of teff-growing households in each kebeles for both groups. In this study, the researcher used the Yamane formula to determine sample size (Yamane, Citation1967). The formula is expressed as:
where n is the sample size, N is the population, and e is the error tolerance or margin of error.
Using 5% level of error or 95% level of confidence, 378 sample sizes will take from households who are engaging in farming through random sampling technique.
The study used a proportionate or equalized sampling technique to take a sample from each woreda, which is specified as follows: , where
represents the sample from strata or kebele
n is the sample size,
is the population, and
is the number of households in each kebele
.
The summary of proportional distribution to the six kebeles is summarized in the below.
Table 1. Sample size determination
3.4. Method of data presentation and analysis
After collecting the data were collected, coded, modified, and presented using . In this study, both descriptive and econometric data analysis techniques were employed. In the descriptive analysis of this study, statistical measures of central tendency and percentage were used to summarize the demographic, socio-economic, and other characteristics of respondents. In the econometric part, Heckman probit and multinomial logit models were employed.
Table 2. Measurements, description of variables, expected sign and sources
3.5. Econometric model specification
To examine the determinants of the adaptation behaviour of farmers, first their perception to climate change was identified. The next was scrutinizing whether the farmers’ adaptation process was in response to the climate change or not (Deressa et al., Citation2009, Citation2010; Gbetibouo, Citation2009; Maddison, Citation2007).
The specific model to be employed varies from researcher to researcher, may be depending upon the type of variables they are interested to incorporate; the scope they try to deal with; the socio-economic, farm characteristics; agro-climatic and institutional challenges they face; and other constraints that the researchers encounter. Despite some departures, the most commonly used models are logit and probit models for analysis of this kind (Deressa et al., Citation2010; Maddison, Citation2007).
The logit regression analysis was used to examine whether farmers perceived climate change and adapted or otherwise (Apata et al., Citation2009; Fosu-Mensah et al., Citation2012; Gbetibouo, Citation2009). Those who adopt the Heckman sample selectivity model argue that adaptation to climate change is a two-step process in which the first involves perceiving the changing climate and then responding to changes through adaptation (Gbetibouo, Citation2009; Maddison, Citation2007).
Probit and logit models have been employed in climate change studies due to the conceptual similarities in agricultural technology adoption and climate change studies (Deressa et al., Citation2009). A binary response model is referred to as a probit model if F is the cumulative normal distribution function, and it is called a logit model if F is the cumulative logistic distribution function. The counter parts of binary probit and logit models, multinomial probit and logit, are used to estimate the effect of explanatory variables on a dependent variable involving multiple choices with unordered response categories. The logistic and normal distributions are both symmetrical around zero and have very similar shapes, except that the logistic distribution has fatter tails. As a result, the conditional probability functions are very similar for both models, except in the extreme tails (Horowitz & Savin, Citation2001).
A sample in which information on the adaptation to climate change is available only for those who perceive the change becomes a censored sample. The Tobit model (also known as a censored regression model; Damodar, Citation2004) will be applied to such kind of situations in which the dependent variable will not be observed for some of the respondents. Hence, we can employ the Tobit model, given that we are interested in analysing the probability of adapting to climate change conditioned on the perception of farmers to the change and the magnitude of adaptation efforts. Discrete technologies are analyzed using the probit or logit model, whereas continuous technologies are usually modelled by the Tobit model or two-limit Tobit (Maddison, Citation2007). Thus, in this study, Heckman probit with selection model was employed.
3.5.1. Heckman probit model
The study applies a simple binary probit model for the case if all farmers respond to the climate change through adaptation and have access to a random sample. However, the reality shows that all farmers may not do so for such phenomenon they face: some farmers may perceive the climate change; some others may not. Some producers may not adapt in response to the climate change though they perceived the change.
Based on Wooldridge (Citation2002), the binary probit model (EquationEquation 1(1)
(1) ) is formulated as:
However, if the binary probit regression method is applied denying those who did not perceive the situation (if we select only those respondents who perceive the climate change), estimates of the parameters obtained from the subset of observations will be biased as well as inconsistent (since the selected respondents will not be the representatives of the population); that is, they will be biased even asymptotically (Damodar, Citation2004). Hence, it calls for further treatment.
Sample selection models by James Heckman address these shortcomings as an alternative for the Maximum Likelihood Method (MLM) though the proposed model is not as efficient as MLM. According to Heckman (Citation1979), sample selection bias may arise in practice for two reasons, either from the investigator or from the target to be investigated. First, there may be self-selection by an individual or data units being investigated. Second, sample selection decision by analysts or data processors is in much similar way of self-selection.
In the process of segregating respondents based on their perception to analyse adjustments on climate change, two stages will be entertained. In a two-stage process, the second stage of adaptation, containing those who responded to the change, is a sub-sample of the whole. Thus, it is likely that the second stage sub-sample is non-random and necessarily different from the first (which is a collection of aware and unaware of the change), and this creates a sample selection bias. Therefore, a two-step maximum likelihood procedure will be used to correct for this selection bias.
The Heckman (Citation1979) sample selectivity probit model proceeds based on the following latent variables.
where
EquationEquation 4(4)
(4) represents selection model showing the perception of farmer p to climate change; and EquationEquation 3
(3)
(3) stands for the outcome model reflecting whether the farmer adapts to the change or not.
X is an n-vector of regressors which are assumed to affect the adaptation farmers.
Z is an m-vector of regressors which seem to influence the perception of farmers to climate change.
β and d are coefficients of explanatory variables to adaptation and perception, respectively.
The latent variable, Y*1p which signifies the likelihood that the farmer will adapt to climate change, depends on whether he perceives, i.e. Y*2P > 0. If ρ is different from zero, implying that there is a correlation between the error terms of selection and outcome models, we will resort to the Heckman probit selection method since the usual probit regression method will be biased (Deressa et al., Citation2010; Maddison, Citation2007; Tazeze et al., Citation2012).
Then the final form of the model is:
where
Y*1pi is a dependent variable: adaptation options. xpi is the vector of explanatory variables for adaptation. β is the vector of coefficients of explanatory variables for adaptation options. Zpi is the vector of explanatory variables for perception of climate change and d is the vector of their coefficients. ρ is the correlation between the two residuals from outcome and selection equations. λ(Zpid) is the inverse Mills ratio. This is equal to .
denotes the standard normal probability density function. Ф is the standard normal cumulative density function. ξp is the new error term.
This model has given the consistent estimates of the parameter vector β. That is, by including the inverse Mills ratio as an additional explanatory variable, the researcher will correct for sample selectivity. There are only two cases where bias will not be a problem: first, if rho = 0, and second, if the correlation between the estimate of lambda and any x variable is zero. It tells us that the adaptation strategies are conditional up on the aforementioned determinants and likelihood of perceiving the climate change.
Consequently, the linear specification of the Heckman probit selection model was given as:
3.5.2. Multinomial logit model
Model choice to study the determinants of adoption decision is based on the number of technologies which are used for study. Binary logit model is the most common way to study single-technology adoption behaviour (Abdulhafedh, Citation2017). Choice model describes the functional relation between the probability of multiple technology adoption and various explanatory variables. Given the modern agricultural technology alternatives (non-adopter, herbicide, improved seed, row planting, changing planting date, fertilizer, and irrigation), which are major practices in the study area. This study estimates a multinomial logit model, which models the modern agricultural technology as a function of demographic, socioeconomic, and institutional characteristic factors to examine the determinants of modern agricultural technology adoption for teff production. Based on this, the current study states the standard multinomial logit model as follow:
, Yi is multiple modern agricultural technology adoption for teff production just categorized as non-adopter, herbicide, improved seed, row planting changing planting date, fertilizer, and irrigation, and
represents the determinants of modern agricultural technology adoption for teff production, i.e., demographic, socioeconomic, and institutional factor and
represents the error term. This gives rise to a polychotomous choice framework. Hence, the probability of modern technology adoption j (j = 0 non-adopter; j = 1 changing planting date; j = 2 fertilizer; j = 3 herbicide; j = 4 irrigation; j = 5; row planting; j = 6 improved seed) is given by the following multinomial logit model. Thus, the linear specification of the multinomial logit outcome model was given as:
3.6. Specification of Variables
4. Results and discussions
4.1. Descriptive results
This section looks into household, farm, and institutional characteristics of the respondents. These characteristics are assumed to have influence on the perception and adaptation strategies of respondents (farmers) included in the study.
4.1.1. Agricultural technology adoption decision across sampled kebeles
summarizes the possible alternative technology adoptions used in the study. Of the total 378 sampled farm households, about 129 (34.13%) are non-adopters. The table reveals that six adaptation options are recorded across the surveyed farmers in the study areas. Of these options, using improved seed was found to be the most frequently practiced adaptation strategy with 89 (23.54%) followed by fertilizer 39 (10.32%); irrigation systems 36 (9.52%); row planting 35 (9.26%); changing planting date 29 (7.67%); and herbicide 21 (5.56%). The sampled kebeles in Amora Bet and Kombolcha do not adopt row planting at all during 2021 cropping season. This situation was directly related to the topography of the kebele, especially the sampled villages, which is difficult to use row planting.
Table 3. Agricultural technology adoption status across kebeles
4.1.2. Household characteristics
Some argue that the role of age in explaining technology adoption is somewhat controversial. Age of the household head plays important role in farm decision-making since the farmer benefits from the experience of an older person as time goes on or enjoy risk-daring behaviour of like his/her younger counterparts (Gbetibouo, Citation2009). points out that the age structure of household heads in the whole sample ranges from 18 years (in Gerba) to 80 years (in Jihur). The mean age is 42.61 with average distribution of 39.028 for Amora Bet, 46.441 for Woyramba, 43.531 for Jihur, 40.838 for Gerba, 43.357 for Kombolcha, and 44 for Bolo Giorgis. The tests indicate that there is a significant difference in ages of the households across the surveyed kebeles.
Table 4. Summary of age and family size of households
This study considers family size as the number of individuals dwelling in a single roof. Family size is assumed as an indicator of the amount of labour available in the family. The distribution of sample respondents in the study area () shows that the average family size is about 4.97. The minimum family size is 1, which is the attribute of all surveyed kebeles, and the maximum family size is 14, in Jihur kebele. The multivariate test (test for equality of means for family size across the four kebeles) assures that there exists a significant difference in means in the given sub-samples.
Though burdens in agrarian society are distributed across all family members, household heads take the coordinating role in their day-to-day activities. Factors such as sex, marital status, and education level of heads also affect the decision-making behaviour in their farming efforts. Dealing with these attributes of respondents help to analyse the motives and determinants of surveyed farmers for taking a certain decision. Due to many socio-cultural values and norms in the study area, males have higher freedom of visiting and participating in different social interactions and in essence have greater access to information. Over 90% of the respondents are males heading their respective family (). Unfortunately, the chi-square test shows that there is no sufficient or strong relationship between sex and their adoption, which is confirmed by the X2 of 9.1286 and p value of 0.104.
Table 5. Summary of partial demographic distribution of households
Marital status is another attribute of the household head, which appears to determine abilities in achieving duties. shows that 88.8% of the respondents have got married and are living together, equally 5.03% are both divorced widowed, and only about 1.06% is alive single. The chi-square test shows that there is a sufficient or strong relationship between marital status and their adoption, which is confirmed by the X2 of 30.251 and p value of 0.011.
Education is one of the very important qualities of farmers to respond to the internal and external stimuli in their working and living environment. It is a crucial factor to understand and interpret agricultural information coming to them from any direction. A better educated farmer can easily react to the information transferred to them by a development agent. As portrays, 36.51% of the respondents are illiterate. Of literate ones, the majority about 51.59% from the total sample attends primary education, 10.85% of respondents attained secondary school, and only 1.06% comes from college and above education. Pearson chi-square test confirmed that the relationship was significant (X2 = 43.3265, p = 0.00).
The study area has huge potential for teff production. Households that have more large number of livestock are likely to adopt more agricultural technologies than their counterparts with less number of livestock as livestock ownership represents wealth (Deressa et al., Citation2010) and thereby have better opportunity to get credit. Livestock often provides cash that farmers can use to buy agricultural inputs. It also plays a very important role by serving as a store of value, source of traction (specially oxen), and provision of manure required for soil fertility maintenance (cited at Deressa et al., Citation2010). This study presents livestock ownership in Tropical Livestock Unit (TLU) summed up from cow, ox, calf, heifer, sheep, goat, mule, donkey, horse, chicken, and bee colony. Of the total respondents (), the minimum, the maximum, and the mean ownership of livestock are 0, 26.85, and 4.97, respectively. The one-way ANOVA and the multivariate tests for inequality of means of livestock ownership over the six kebeles showed significant result.
Table 6. Livestock ownership and income
The amount of farm produce proxies the economic strength or income of the agrarian community in the study area. Farm and non-farm incomes are assumed to increase adaptation to climate change. According to Deressa et al. (Citation2010), farm and non-farm incomes play their own role on perception as they create access to farmers for more information. The data collected in this study reflect that the number of farmers with off-farm income is small. Thus, farm produce is treated as an income of farm households. The income level oscillates between the minimum of 112.2 birr (in Amora Bet) and the maximum of 2929.5 birr (in Gerba) with a mean income of 791.42 birr. The multivariate test in distribution of production in six kebeles shows that there exists significant difference (with 1% probability level) in income level.
4.1.3. Farm characteristics
Farmers with more years of farming experience are better likely to perceive the climate change (Maddison, Citation2007) and to assess the characteristics of technologies for adaptation than younger farmers and, hence, a higher probability of adopting the practice. The data in show that the mean experience ranges from 21.47 to 26.88 years. The one-way analysis of variance dictates a significant difference in the mean farming experience over the sampled kebeles.
Table 7. Farm experience and distribution of farm characteristics
It is assumed that adoption of a farm innovation will be likely to take place earlier on larger farms than on smaller farms (Gbetibouo, Citation2009). Cultivated land size is usually associated with greater wealth, and it is hypothesized to enhance adaptation to climate change. According to Deressa et al. (Citation2010), it is not only the size of the farm that affects to choose a specific adaptation method for climate change but also the specific characteristics of the farm. Fertility of soil, access to irrigation, and farm size as well, therefore, need particular attention so as to assess the determinants of a farmer’s decision. In the study area, the cultivated land size () with minimum and maximum of 1.37 ha (in Bologiorgis) and 2.09 ha (in Jihur), respectively. The land distribution across sample respondents living in the six kebeles shows a significant (at 99% probability level) variation in average land holdings.
4.1.4. Institutional characteristics
Extension services on crop and livestock production and information on climate signify access to the information required to be aware of the changing climate and to make decision on adaptation to the phenomena. Thus, the opportunity to get extension and information sources enhance to adopt new technologies by exposing farmers to new knowledge and technical skills. Obtaining information through extension contacts increased the chance of household to get information on new or improved farm technologies. Out of the total respondents (), about 349 (92.33%) had access to extension services, whereas 29 (7.67%) of them believed to lack the opportunity; they are neither visited nor visit the extension agents. Among many sources of information available to farmers, agricultural extension plays the most important role for evaluating the adoption decision. The minimum proportion of respondents with the service access is 88.1% (in Kombolcha) and the maximum is in Woyramba with 95.35%.
Table 8. Summary of access to institutional setups
The access to information on climate change from either extension agents or any other sources such as various media and organizations is meant to increase awareness (Gbetibouo, Citation2009). Information is one of the attributes of importance for farmers to improve their farm production and productivity. To overcome whatever imperfections exist, farmers can broadly be provided with two things: information through extension services and subsidies and price supports (Maddison, Citation2007). Respondents in this study get various information of their desire from agricultural agents, social extensions, markets, formal and informal meetings (such as church events), media, and educated relatives. About 83.3% of the surveyed households believed to have access to some or all of the aforementioned sources (). The Pearson chi square confirmed that the relationship was statistically significant (X2 = 12.605 & p = 0.027).
Credit is considered to be major constraints in most of the agrarian societies of developing countries, suggesting that households generally do not have enough cash to buy fertilizer. Availability of credit, thus, eases the cash constraints and allows farmers to buy purchased inputs such as fertilizer, improved crop varieties, and irrigation facilities. Of 278 respondents in this study (), an average of 202 (53.44%) had access to credit. The Pearson chi square confirmed that the relationship was statistically significant (X2= 24.25 & p = 0.000).
Many believe that land tenure has frequently been debatable issue as a barrier to technology adaptation, and recent research supports this hypothesis (Maddison, Citation2007). Studies explored that there exists an association between tenure status and the courage that farmers take to welcome new innovations. The notion behind this argument is that with proper property rights, farmers may be motivated to change their amount of land under cultivation to adjust to new climatic conditions.
reveals that 88.36% of the total respondents expressed their feeling of security on their land, and the Pearson chi square confirmed that the relationship was statistically significant (X2 = 21.32 & p =0.000).
4.2. Econometric results
The estimated results of Heckman probit model and multinomial logit model are presented in . Wu-Hausman and Durbin score test is a direct test for endogeneity. The Durbin (score) and Wu-Hausman test of endogeneity in the regression is rejected with large p-value (p = 0.8400) (see Appendix 1). Thus, the test results proved that perception and adoption are exogenous and the study relay on Heckman probit model and multinomial logit model. In addition, the results are run with robust standard errors, given the possibility of heteroskedasticity problem. Furthermore, both continuous and discrete variables were tested for multicollinearity. The computing process involves variance inflation factor (VIF) for continuous variables and contingency coefficients for dummy variables as a means to detect the existence of multicollinearity among variables.
Table 9. Results of the Heckman probit model
Table 10. Results of the marginal effects of the multinomial logit model
4.2.1. Results of Heckman probit model
This study identifies the factors that influence the propensity to perceive as well as the decision to adapt the changing climate. To do so, the sample selection model, Heckman probit model with selection, is employed. The common form of the model has two stages. In the first stage, a dichotomous variable, perception, determines whether or not another variable, adaptation and adaptation, is observed only if perception is equal to 1; in the second stage, the researcher models the expected value of adaptation, conditional on its being observed. The Heckman probit model is, thus, employed to see whether the a priori hypothesis holds or not. If the errors are uncorrelated, then unlink the likelihood and run separate models on the selection and outcome equations (Sweeney, Citation2003). presents the results of the Heckman probit with sample selection model. As in the selection equation where the regressand was binary, representing whether or not a farmer perceived climate change, the regressand in the outcome equation was also binary indicative of whether or not a farmer reacted to the perceived changes through adaptation. The likelihood function for the Heckman probit model was significant (Wald χ2 = 29.251 with p < 0.00), showing a strong explanatory power. Furthermore, the coefficient of inverse Mills ratio is reported as 2.718, and it is significant. That means, in the result, selection bias is a significant issue. Another signal for selection bias is rho = 1.000; the correlation coefficient between the error terms in the two equations is 1 here. Indeed, the researcher is using a sample selection model on this data.
revealed that, of the eight independent variables included only in the perception equation, the probit model estimation shows that five variables were found to be the significant factors determining the farmers’ perception to climate change. Gender, farm experience, and access to extension services on crop affected the probability of perceiving the changing climate significantly and positively at 1% (p < 0.000) level of significance. Also, information access and livestock ownership affect the likelihood of perception positively and significantly at 5% and 10% levels of significance, respectively. The coefficients’ sign also went with what had been hypothesized.
The coefficient of gender is positive and significant for perception of climate change at 1% level of significance. The marginal effect shows that a one-year increase in age of a household raises the likelihood of perceiving the climate change by about 30.9%, holding other factors being constant. This result is in line with Asrat and Simane (Citation2018) and Tazeze et al. (Citation2012).
Another variable with a significant (at 1% level of significance) influence was farm experience. The variable was positively and significantly influenced the farmer’s sight to recognize the climate change. The more the years of farm experience, the higher he/she is likely to perceive the changing climatic conditions. Reviews of the diffusion of new technologies show that farm experience was one of the major determinants of the speed of adoption of techniques of adaptation (Maddison, Citation2007), which might have been the result of perception. In this study, results show that a one-year increase in farm experience of a household raises the likelihood of perceiving the climate change by about 0.5%. The result is consistent with Addis and Abirdew (Citation2021) and Mairura et al. (Citation2021).
The coefficient of access to extension services is positive and significant at 1% level of significance. A unit increase in the access to extension services to predominantly teff producers will have the impact of raising the chance to climate change by about 31.4%. This argument conformed to the previous finding (Adeagbo et al., Citation2021; Nyang’au et al., Citation2021; Teshome et al., Citation2021) that dictates that those farmers with better access to these services are in a better position to understand the changing climatic phenomena.
Similarly, access to information has a significant (at 5% or p < 0.005) and positive influence on farmers’ perception to climate change. This shows that the higher the opportunity of the farmer to climatic information, the higher is his/her perception to any variation in the climate. As access to information services increases by one unit, the probability of recognizing the changing climatic condition increases by 14.8%. This result is consistent with Deressa et al. (Citation2010) and Maddison (Citation2007), which showed that increase in various sources of information on climate change enhances the likelihood of being aware of its impact and thereby the endeavour to resist the bad from the change.
4.2.2. Determinants of adoption
The multinomial logit model was employed with a robust-based computation. Additionally, the Hausman specification test was conducted to identify the existence of problem of IIA. The null hypothesis of the test is that the odds of any two outcomes are independent of another alternative, and when the p-value of the test becomes insignificant, it is in favour of the null hypothesis, and it confirms that the multinomial logit model is appropriate. About 14 variables were supposed to determine the decision of farmers to perceive and adapt to the climate change.
Based on the estimation, the result indicates that the sign of marital status is positive and has a significant effect on changing planting date, row planting adoption, and using irrigation adoption options at 1% and 5% levels of significance, respectively. The marginal effect indicates that if household is married, then probability of adopting changing planting date, using irrigation system, and rowplanting were increased by 1.3%, 4.4%, and 13.9%, respectively, relative to single, being all other things equal. This indicates that household head’s adoption decision is influenced by his/her spouse’s attitude and willingness toward technology, so married household head easily decided to use modern agricultural technology and work together to increase the productivity of teff. This result is consistent with Giziew and Mebrate (Citation2019) and Massresha et al. (Citation2021).
Gender of farmers is positive and significant for adopting herbicide and improved seed at the 5% and 10% levels of significance, respectively. The positive sign of the coefficient indicates that male-headed households were more likely to adopt herbicide and improved seed technology package than female-headed household heads. If household are male headed, then probability of adopting herbicide increased by 4.6% relative to non-adopters, all other things being equal. However, if households are male headed, then probability of adopting improved seed was increased by 0.7% relative to non-adopters, all other things being constant. The possible explanation might be that male-headed households have better access to information, agricultural inputs, and resource endowments. This result is in line with Deressa et al. (Citation2008) and Giziew and Mebrate (Citation2019). However, it is against the result of Bayard et al. (Citation2007). Female farmers have been found to be more likely to adopt natural resource management and conservation practices.
Education was expected to affect perception and adaptation to climate change. Education is found statistically significant in affecting the adoption of changing planting date at 10% level of significance as expected in prior. The likelihood of households for adopting changing planting date increases with an increase in years of education. The marginal effect revealed that if households are educated, then probability of adopting changing planting date increased by 7% relative to non-adopters, being all other things constant. This is because education enables to acquire, analyse, and evaluate information. This result is consistent with the findings of Atinafu et al. (Citation2022) and Massresha et al. (Citation2021). However, it is contradicted with Haile et al. (Citation2022) and Tesfaye et al. (Citation2016), which indicate that attending education may create other job opportunities to participate in non-agricultural activities as employee.
Unexpectedly, the coefficient of family size is negative and significantly influences the adoption of improved seed at 1% level of significance. This is because those farmers who have more family members are less likely to purchase improved seed. The marginal effect revealed that if household family size increased by one, then probability of adopting improved seed decreased by 2.7% relative to non-adopters, being all other things constant. This result is supported by the study by Challa and Tilahun (Citation2014); household with such large number of members outlays its income more on consumption expenditure rather than investing on the new technology. On the contrary, the result is not consistent with Giziew and Mebrate (Citation2019) and Zegeye and Read (Citation2021).
Experience in farming is significant at 1%, affecting the dependent variable positively, meaning that the probability of choosing this adaptation option is intensified as the farmer gets more experience on the trend of climate and on the techniques of farming under the threat of climate change and variability. As one unit (year) of experience comes into being, the likelihood of using changing planting date as an adaptation option increases by 1%, holding other factors being constant. This result is in line with Maddison (Citation2007) and Sedebo et al. (Citation2022). But, it is in contrast to Haile et al. (Citation2022), which indicates that older farmers (more experienced household heads) might be more concerned about being food secured and would not want to take the risk of demanding their crop banks. On the contrary, younger household heads would engage in the markets probably because they are more dynamic to adopt new technologies that enhance productivity.
The coefficient of land tenure security is positive and significant on adoption of changing planting date and using fertilizer at 1% level of significance. reveals that one unit increase in land tenure enhances the probability of using changing planting date and fertilizer adaptation option by 5.5% and 0.2%, respectively, holding other things being constant. This result is confirmed by previous studies (Paltasingh, Citation2018; Zeng et al., Citation2018; Tesfaye & Nayak, Citation2022), stating that land tenure security is a variable which increases the courage of farmers to conserve their land and adopt agricultural technology.
Extension visit is positive and significant at 5% level of significance. The positive association indicates that as farmers’ contact with extension agents increases, the likelihood of adoption to changing planting date. This is because the extension contact helps the farmers to raise their awareness about the characterization and attributes of the technology and its use and impact. Extension gives detailed information, training, and advisory services about the source, use, and importance of the technologies to the farmers to engage in input distribution. The marginal effect indicates that as the frequency extension visit increases, the probability of adopting changing planting date has increased 4.1% relative to non-adopter, holding all other variables constant. This study is in line with the study conducted by Maddison (Citation2007), propounded that farmers who have enjoyed free extension advice are more likely to adapt to climate change by choosing the strategy of changing planting date. Also, this result is consistent with Adeagbo et al. (Citation2021) and Damota et al. (Citation2022); access to extension and awareness of climate change are some of the important determinants of farm-level adaptation.
The coefficient of income is positive and significant on adopting improved seed and row planting at 1% and 10% levels of significance, respectively, as it expected. The marginal effect indicates that as the income of a farmer increases by one ETB, the probability of using improved seed and row planting increases by 1.80% and 9.6%, respectively, relative to non-adopters, while other things remain constant. This is because if households who had an income are more expected to purchase improved seed and use more labor for row planting. This implies that increasing capital to the farmer boosts adoption by enabling him/her to have the capacity to purchase technology and associated inputs which can be used in implementing the technology. Adoption of modern technology for teff production needs capital which can be used in buying inputs like row planting machine as well as hiring labor. This result is consistent with Ahmed et al. (Citation2016) and Belay and Mengiste (Citation2021).
Livestock ownership is positive and significant for adopting row planting, herbicide, and changing planting date at 1%, 5%, and 10% significance levels, respectively. The positive association indicates that as farmers have more tropical livestock, the likelihood of adoption to row planting, herbicide, and changing planting date increases. The marginal effect indicates that when farmers have livestock, the probability of the adoption of row planting, herbicide, and changing planting date increased by 13.6 %, 7.3%, and 0.9%, respectively, holding all other variables constant. This implies that major factor that affects the adoption decision of farmers toward the new agricultural technology adoption encouraged the farmer to utilize the modern farm incentives. This result is confirmed with Siyum et al. (Citation2022) and Tarekegn and Ayele (Citation2020).
The coefficient of information access is positive and significant for the adoptions of row planting at 1% level of significance and as well it is positive and significant for adopting changing planting date, using fertilizer, using herbicide, and using improved seed at 5% level of significance equally, showing that having an information access increases the adoption practice of agricultural technologies. This is because access to information helps the farm households regarding the characterization, sources, application, and importance of the technology. This is in line with Deressa et al. (Citation2010), Maddison (Citation2007), and Sedebo et al. (Citation2022).
As expected, distance to market is negative and significant for adopting improved seed, changing planting date, and using irrigation system at 1%, 5%, and 10% levels of significance, respectively. The negative association indicates that as distance to market increases, the likelihood of adopting those technologies decreases. This result may verify that those framers who live away from service centres such as urban centres, development agent, and market place are less likely to adopt farm technologies. The marginal effect indicates that if distance to market is increased by 1 km, then the probability of adopting improved seed has decreased by 0.1%, 1.1%, and 0.5%, respectively, relative to non-adopter, respectively, holding all other variables constant. The justification for this is that the proximity of farmers to all weather roads and markets is essential for timely input delivery and output disposal and results in less transport cost of inputs and outputs markets and market information the reverse is true. This result is consistent with the previous studies (Milkias & Abdulahi, Citation2018; Sebsibie et al., Citation2015; Mihretie et al., Citation2022).
5. Conclusion and recommendation
North Shewa zone is the potential teff-producing area in Amhara region of Ethiopia. The main theme of this study centres on understanding the perception and adaptation strategies of teff producers to climate change in North Shewa zone. The paper asks farmers whether they perceive and adapt to the climate change. It also attempts to determine the socio-economic, farm, and institutional characteristics with farmers who, despite claiming to have witnessed climate change, have not yet responded to it. The primary source of data was employed, and a total of 378 respondents constituted the sample size which is drawn from six kebeles of the teff-producing areas in the zone. The Heckman selection and multinomial logit models were employed. The results of the Heckman probit model reveal that perception to climate change is significantly and positively affected by gender, farm experience, access to extension services, information access, and livestock ownership, which affect the likelihood of perception positively and significantly. The multinomial logit model shows that access to information was the most important explanatory variable as it was significantly associated with all adaptation options except practicing irrigation system. Gender of the head of the household being male was considerably influential to adapt to climate change using improved varieties of seeds and increased use of herbicides as adaptation practices. Farming experience was significantly associated with the practice of changing planting date. Similarly, access to extension services was a significant determining factor for the adaptation strategy of changing planting date. Land tenure was found to be a significant factor in affecting increased use of fertilizer and changing planting date. Livestock ownership was another determinant linked significantly to the options of using row-planting herbicide and changing planting date. Information access is positive and significant for the adoptions of row planting and as well it is positive and significant for adopting changing planting date, using fertilizer, using herbicide, and using improved seed. Marital status is positively and significantly affected changing planting date, row planting adoption, and using irrigation adoption options. Education is found statistically significant in affecting the adoption of changing planting date. Income of household has a significant effect to adopt improved seed and row planting. Distance to market negatively and significantly affects changing planting date, using irrigation, and improved seed adoption options.
Thus, the following recommendations are suggested. In terms of policy implication, refining the education system through expansion of adult schools and crafting systems that allow farmers to get education would do utmost in hastening climate change perception and adaptation. Moreover, government structures at different levels should establish local meteorological stations to provide up-to-date climate information. Farmers also need to be encouraged to use different media like radio, phone, and television to get access to climate information so that they will be able to adopt and respond to climate change. The Ministry of Agriculture and other donor agencies must invest in institutions such as extension agents, by increasing the availability and quality of extension service, encouraging the participation of farmers in training centres, and providing advisory service to encourage farmers to adopt appropriate climate change adaptation strategies. Banks and other microfinance institutions should provide access to credit with affordable interest rate to secure immediate need of money for the very purpose of purchasing farm inputs and meet the costs associated with using various adaptation strategies. The Government of Ethiopia should create opportunities of land security for the farmers to have their own land and improve the existing market centres through construction of roads. Ethiopian Road Authority should be given sufficient attention for construction of community roads. Gender difference needs special consideration. Therefore, it is necessary to design and implement policies that aim at awareness creation of female households that could be able to easily collect, analyse, and interpret relevant information about climate change and adaptation strategies. Finally, enhancing perception and scaling up of climate change adaptation technologies require a shared vision of all potential stakeholders and public–private partnership.
5.1. Areas for further research
Further studies are recommended to examine the impact of each adaptation strategies in improving the livelihood of farmers and alleviate the problem of food insecurity in the district.
Acknowledgements
We would like to thank the editor and anonymous reviewers for their supportive comments and suggestion.
Disclosure statement
No potential conflict of interest was reported by the authors.
Additional information
Funding
Notes on contributors

Girma Mulugeta Emeru
Girma Mulugeta Emeru Girma Mulugeta Emeru completed his First Degree in Economics from Debre Berhan University and Master’s Degree in Developmental Economics from Hawassa University, Ethiopia. Currently, he is a lecturer of Economics at Debre Berhan University. In his career, Girma has published more than four research articles on agricultural technology adoption, urban livelihood diversification strategies, Ethiopian energy sector, and women labor force participations in reputable journals. He has also reviewed journal manuscripts related to his field of specialization invited from recognized publisher of Taylor & Francis.
Notes
1. Kebele is an Amharic word which means the lowest administrative unit in Ethiopia.
References
- Abdulhafedh, A. (2017). Incorporating the multinomial logistic regression in vehicle crash severity modelling: A detailed overview. Journal of Transportation Technologies, 7(3), 279. https://doi.org/10.4236/jtts.2017.73019
- Addis, Y., & Abirdew, S. (2021). Smallholder farmers’ perception of climate change and adaptation strategy choices in Central Ethiopia. International Journal of Climate Change Strategies and Management, 13(4/5), 463–34. https://doi.org/10.1108/IJCCSM-09-2020-0096
- Adeagbo, O. A., Ojo, T. O., & Adetoro, A. A. (2021). Understanding the determinants of climate change adaptation strategies among smallholder maize farmers in south-west, Nigeria. Heliyon, 7(2), e06231. https://doi.org/10.1016/j.heliyon.2021.e06231
- Ahmed, M. H., Mesfin, H. M., Abady, S., Mesfin, W., Kebede, A., & Aye, G. (2016). Adoption of improved groundnut seed and its impact on rural households’ welfare in Eastern Ethiopia. Cogent Economics & Finance, 4(1), 1268747. https://doi.org/10.1080/23322039.2016.1268747
- Ali, S., Liu, Y., Ishaq, M., Shah, T., Din, I. U., Din, I. U., & Din, I. (2017). Climate change and its impact on the yield of major food crops: Evidence from Pakistan. Foods, 6(6), 39. https://doi.org/10.3390/foods6060039
- Apata, T. G., Samuel, K. D., & Adeola, A. O. (2009). Analysis of climate change perception and adaptation among Arable food crop farmers in South Western Nigeria. No. 1005-2016-79140.
- Asfaw, S., Di Battista, F., & Lipper, L. (2016). Agricultural technology adoption under climate change in the Sahel: Micro-evidence from Niger. Journal of African Economies, 25(5), 637–669. https://doi.org/10.1093/jae/ejw005
- Asfaw, A., Simane, B., Bantider, A., & Hassen, A. (2019). Determinants in the adoption of climate change adaptation strategies: Evidence from rainfed-dependent smallholder farmers in north-central Ethiopia (Woleka sub-basin). Environment, Development and Sustainability, 21(5), 2535–2565. https://doi.org/10.1007/s10668-018-0150-y
- Asmare, F., Teklewold, H., & Mekonnen, A. (2019). The effect of climate change adaptation strategy on farm households welfare in the Nile basin of Ethiopia: Is there synergy or trade-offs? International Journal of Climate Change Strategies and Management, 11(4), 518–535. https://doi.org/10.1108/IJCCSM-10-2017-0192
- Asrat, P., & Simane, B. (2018). Farmers’ perception of climate change and adaptation strategies in the Dabus watershed, North-West Ethiopia. Ecological Processes, 7(1), 1–13. https://doi.org/10.1186/s13717-018-0118-8
- Atinafu, A., Lejebo, M., & Alemu, A. (2022). Adoption of improved wheat production technology in Gorche district, Ethiopia. Agriculture & Food Security, 11(1), 1–8. https://doi.org/10.1186/s40066-021-00343-4
- Awaad, H. A. (2022). Climate change and its impact on sustainable crop production. In Sustainable Agriculture in Egypt (pp. 23–52). Springer. https://doi.org/10.1007/978-3-030-81873-9_2
- Azhoni, A., & Goyal, M. K. (2018). Diagnosing climate change impacts and identifying adaptation strategies by involving key stakeholder organisations and farmers in Sikkim, India: Challenges and opportunities. Science of the Total Environment, 626, 468–477. https://doi.org/10.1016/j.scitotenv.2018.01.112
- Bayard, B., Jolly, C. M., & Shannon, D. A. (2007). The economics of adoption and management of alley cropping in Haiti. Journal of Environmental Management, 84(1), 62–70. https://doi.org/10.1016/j.jenvman.2006.05.001
- Baylie, M. M., & Fogarassy, C. (2021). Examining the economic impacts of climate change on net crop income in the Ethiopian Nile Basin: A Ricardian fixed effect approach. Sustainability, 13(13), 7243. https://doi.org/10.3390/su13137243
- Bedeke, S. B. (2022). Climate change vulnerability and adaptation of crop producers in sub-Saharan Africa: A review on concepts, approaches and methods. Environment, Development and Sustainability, 1–35.
- Belay, A., Recha, J. W., Woldeamanuel, T., & Morton, J. F. (2017). Smallholder farmers’ adaptation to climate change and determinants of their adaptation decisions in the central Rift Valley of Ethiopia. Agriculture & Food Security, 6(1), 1–13. https://doi.org/10.1186/s40066-017-0100-1
- Belay, M., & Mengiste, M. (2021). The ex‐post impact of agricultural technology adoption on poverty: Evidence from north Shewa zone of Amhara region, Ethiopia. International Journal of Finance & Economics. https://doi.org/10.1002/ijfe.2479
- Berihun, D., & Van Steven, P. (2021). Climate variability and macroeconomic output in Ethiopia: The analysis of nexus and impact via asymmetric autoregressive distributive lag cointegration method. Environment, Development and Sustainability, 24, 4064–4087. https://doi.org/10.1007/s10668-021-01604-9
- Challa, M., & Tilahun, U. (2014). Determinants and impacts of modern agricultural technology adoption in west Wollega: The case of Gulliso district. Journal of Biology, Agriculture and Healthcare, 4(20), 63–77.
- Chambwera, M. (2010). Climate change adaptation in developing countries: Issues and perspectives for economic analysis. Iied.
- Change, I. C. (2014). Impacts, adaptation, and vulnerability. Part A: Global and sectoral aspects. Contribution of working group II to the fifth assessment Report of the Intergovernmental Panel on Climate Change, 1132.
- CSA. (2007). Statistical abstract. Addis Ababa.
- Damodar, N. G. (2004). Basic Econometrics-Damodar N. Gujarati. McGraw− Hill.
- Damota, D. D., Gelu, D. G., & Orkaido, K. (2022). Determinants of smallholder farmers participation on wheat row planting and its impact on wheat yield: The case in Mari Mansa Woreda, Dawuro Zone, SNNPR, Ethiopia. Recent Trends in Data Mining and Business Forecasting, 1–13.
- Deressa, T. T., Hassan, R. M., Ringler, C., Alemu, T., & Yesuf, M. (2008). Analysis of the determinants of farmers' choice of adaptation methods and perceptions of climate change in the Nile Basin of Ethiopia [in Amharic] (No. 15 (9) AMH). International Food Policy Research Institute (IFPRI).
- Deressa, T. T., Hassan, R. M., Ringler, C., Alemu, T., & Yesuf, M. (2009). Determinants of farmers’ choice of adaptation methods to climate change in the Nile Basin of Ethiopia. Global Environmental Change, 19(2), 248–255. https://doi.org/10.1016/j.gloenvcha.2009.01.002
- Deressa, T. T., Ringler, C., & Hassan, R. M. (2010). Factors affecting the choices of coping strategies for climate extremes. The case of farmers in the Nile Basin of Ethiopia IFPRI Discussion Paper, 1032.
- Destaw, F., & Fenta, M. M. (2021). Climate change adaptation strategies and their predictors amongst rural farmers in Ambassel district, Northern Ethiopia. Jàmbá: Journal of Disaster Risk Studies, 13(1), 1–11. https://doi.org/10.4102/jamba.v13i1.974
- Fahad, S., & Jing, W. (2018). Evaluation of Pakistani farmers’ willingness to pay for crop insurance using contingent valuation method: The case of Khybe7r Pakhtunkhwa province. Land Use Policy, 72, 570–577. https://doi.org/10.1016/j.landusepol.2017.12.024
- Feyisa, B. W., & Yildiz, F. (2020). Determinants of agricultural technology adoption in Ethiopia: A meta-analysis. Cogent Food & Agriculture, 6(1), 1855817. https://doi.org/10.1080/23311932.2020.1855817
- Fosu-Mensah, B. Y., Vlek, P. L., & MacCarthy, D. S. (2012). Farmers’ perception and adaptation to climate change: A case study of Sekyedumase district in Ghana. Environment, Development and Sustainability, 14(4), 495–505. https://doi.org/10.1007/s10668-012-9339-7
- Gbetibouo, G. A. (2009). Understanding farmers’ perceptions and adaptations to climate change and variability: The case of the limpopo Basin, South Africa (Vol. 849). Intl Food Policy Res Inst.
- Gebreegziabher, Z., Stage, J., Mekonnen, A., & Alemu, A. (2011). Climate change and the Ethiopian economy: A computable general equilibrium analysis. Resources for the Future.
- Ginbo, T. (2022). Heterogeneous impacts of climate change on crop yields across altitudes in Ethiopia. Climatic Change, 170(1–2), 1–21. https://doi.org/10.1007/s10584-022-03306-1
- Giziew, A., & Mebrate, B. (2019). Determinants of the role of gender on adoption of row planting of tef [Eragrostis tef (Zucc.) Trotter] in central Ethiopia. Ethiopian Journal of Science and Technology, 12(1), 19–43. https://doi.org/10.4314/ejst.v12i1.2
- Gunawat, A., Sharma, D., Sharma, A., & Dubey, S. K. (2022). Assessment of climate change impact and potential adaptation measures on wheat yield using the DSSAT model in the semi-arid environment. Natural Hazards, 111, 2077–2096. https://doi.org/10.1007/s11069-021-05130-9.
- Haile, K., Gebre, E., & Workye, A. (2022). Determinants of market participation among smallholder farmers in Southwest Ethiopia: Double-hurdle model approach. Agriculture & Food Security, 11(1), 1–13. https://doi.org/10.1186/s40066-022-00358-5
- Heckman, J. J. (1979). Sample selection bias as a specification error. Econometrica: Journal of the Econometric Society, 47(1), 153–161. https://doi.org/10.2307/1912352
- Heidenreich, A., Grovermann, C., Kadzere, I., Egyir, I. S., Muriuki, A., Bandanaa, J., Clottey, J., Ndungu, J., Blockeel, J., Muller, A., Stolze, M., & Schader, C. (2022). Sustainable intensification pathways in sub-Saharan Africa: Assessing eco-efficiency of smallholder perennial cash crop production. Agricultural Systems, 195, 103304. https://doi.org/10.1016/j.agsy.2021.103304
- Horowitz, J. L., & Savin, N. E. (2001). Binary response models: Logits, probits and semiparametrics. Journal of Economic Perspectives, 15(4), 43–56. https://doi.org/10.1257/jep.15.4.43
- Hossain, M. S., Alam, G. M., Fahad, S., Sarker, T., Moniruzzaman, M., & Rabbany, M. G. (2022). Farmers’ willingness to pay for flood insurance as climate change adaptation strategy in northern Bangladesh. Journal of Cleaner Production, 130584.
- Jahan, M., & Qale Nawi, S. (2022). Challenges and opportunities faced on food production systems in arid and semi-arid regions under climate change conditions. Annals of Agricultural and Crop Sciences, 7.
- Kamau, M. W. (2010). Perceptions of climate change, adaptation and the policy process within the Forestry Commission of Great Britain: A comparative study of England, Scotland and Wales. University of Twente Faculty of Geo-Information and Earth Observation (ITC).
- Karimi, V., Karami, E., & Keshavarz, M. (2018). Climate change and agriculture: Impacts and adaptive responses in Iran. Journal of Integrative Agriculture, 17(1), 1–15. https://doi.org/10.1016/S2095-3119(17)61794-5
- Khanal, U., Wilson, C., Rahman, S., Lee, B. L., & Hoang, V. N. (2021). Smallholder farmers’ adaptation to climate change and its potential contribution to UN’s sustainable development goals of zero hunger and no poverty. Journal of Cleaner Production, 281, 124999. https://doi.org/10.1016/j.jclepro.2020.124999
- Kiani, A. K., Sardar, A., Khan, W. U., He, Y., Bilgic, A., Kuslu, Y., & Raja, M. A. Z. (2021). Role of agricultural diversification in improving resilience to climate change: An empirical analysis with Gaussian paradigm. Sustainability, 13(17), 9539. https://doi.org/10.3390/su13179539
- Liberio, J. U. S. T. I. N. E. (2012). Factors contributing to adoption of sunflower farming innovations in Mlali ward, Mvomero district, Morogoro Region–Tanzania. Unpublished Masters Dissertation, Sokoine University of Agriculture.
- Maddison, D. (2007). The perception of and adaptation to climate change in Africa (Vol. 4308). World Bank Publications.
- Mairura, F. S., Musafiri, C. M., Kiboi, M. N., Macharia, J. M., Ng’etich, O. K., Shisanya, C. A., Okeyo, J. M., Mugendi, D. N., Okwuosa, E. A., & Ngetich, F. K. (2021). Determinants of farmers’ perceptions of climate variability, mitigation, and adaptation strategies in the central highlands of Kenya. Weather and Climate Extremes, 34, 100374. https://doi.org/10.1016/j.wace.2021.100374
- Marie, M., Yirga, F., Haile, M., & Tquabo, F. (2020). Farmers’ choices and factors affecting adoption of climate change adaptation strategies: Evidence from northwestern Ethiopia. Heliyon, 6(4), e03867. https://doi.org/10.1016/j.heliyon.2020.e03867
- Massresha, S. E., Lema, T. Z., Neway, M. M., Degu, W. A., & Shafiullah, M. (2021). Perception and determinants of agricultural technology adoption in North Shoa Zone, Amhara Regional State, Ethiopia. Cogent Economics & Finance, 9(1), 1956774. https://doi.org/10.1080/23322039.2021.1956774
- Mekonnen, M., Abeje, T., & Addisu, S. (2021). Integrated watershed management on soil quality, crop productivity and climate change adaptation, dry highland of Northeast Ethiopia. Agricultural Systems, 186, 102964. https://doi.org/10.1016/j.agsy.2020.102964
- Mihiretu, A., Okoyo, E. N., Lemma, T., & González-Redondo, P. (2020). Small holder farmers’ perception and response mechanisms to climate change: Lesson from Tekeze lowland goat and sorghum livelihood zone, Ethiopia. Cogent Food & Agriculture, 6(1), 1763647. https://doi.org/10.1080/23311932.2020.1763647
- Mihretie, F. A., Tsunekawa, A., Haregeweyn, N., Adgo, E., Tsubo, M., Ebabu, K., Masunaga, T., Kebede, B., Meshesha, D. T., Tsuji, W., Bayable, M., & Berihun, M. L. (2022). Tillage and crop management impacts on soil loss and crop yields in northwestern Ethiopia. International Soil and Water Conservation Research, 10(1), 75–85. https://doi.org/10.1016/j.iswcr.2021.04.006
- Milkias, D., & Abdulahi, A. (2018). Determinants of agricultural technology adoption: The case of improved highland maize varieties in Toke Kutaye District, Oromia Regional State, Ethiopia. Journal of Investment and Management, 7(4), 125–132. https://doi.org/10.11648/j.jim.20180704.13
- Mohammed, U. D., Legesse, S. A., Berlie, A. B., & Ehsan, M. A. (2022). Climate change repercussions on meteorological drought frequency and intensity in South Wollo, Ethiopia. Earth Systems and Environment, 1–11. https://doi.org/10.1007/s41748-022-00293-2
- Muluneh, A. (2020). Impact of climate change on soil water balance, maize production, and potential adaptation measures in the Rift Valley drylands of Ethiopia. Journal of Arid Environments, 179, 104195. https://doi.org/10.1016/j.jaridenv.2020.104195
- Nciizah, T., Nciizah, E., Mubekaphi, C., & Nciizah, A. D. (2022). Smallholder farmers’ adaptation strategies and food security: Experiences from Zimbabwe. In Mupambwa, H.A., Nciizah, A.D., Nyambo, P., Muchara, B., Gabriel, N.N. (eds)., Food Security for African Smallholder Farmers (pp. 267–280). Springer.
- Negra, C. (2014). Integrated national policy approaches to climate-smart agriculture. Insights from Brazil, Ethiopia, and New Zealand. CCAFS Report. www.ccafs.cgiar.org
- Nhemachena, C., & Hassan, R. (2007). Micro-level analysis of farmers adaption to climate change in Southern Africa. International Food Policy Research Institute.
- North Shewa Zone Administration office, 2022. Geographical location and number of populations of the Zone.
- Nyang’au, J. O., Mohamed, J. H., Mango, N., Makate, C., & Wangeci, A. N. (2021). Smallholder farmers’ perception of climate change and adoption of climate smart agriculture practices in Masaba South Sub-county, Kisii, Kenya. Heliyon, 7(4), e06789. https://doi.org/10.1016/j.heliyon.2021.e06789
- Ojo, T. O., & Baiyegunhi, L. J. S. (2021). Climate change perception and its impact on net farm income of smallholder rice farmers in South-West, Nigeria. Journal of Cleaner Production, 310, 127373. https://doi.org/10.1016/j.jclepro.2021.127373
- Osumanu, I. K., Aniah, P., & Yelfaanibe, A. (2017). Determinants of adaptive capacity to climate change among smallholder rural households in the Bongo district, Ghana. Ghana Journal of Development Studies, 14(2), 142–162. https://doi.org/10.4314/gjds.v14i2.8
- Owusu, V., Ma, W., Emuah, D., & Renwick, A. (2021). Perceptions and vulnerability of farming households to climate change in three agro-ecological zones of Ghana. Journal of Cleaner Production, 293, 126154. https://doi.org/10.1016/j.jclepro.2021.126154
- Paltasingh, K. R. (2018). Land tenure security and adoption of modern rice technology in Odisha, Eastern India: Revisiting Besley’s hypothesis. Land Use Policy, 78, 236–244. https://doi.org/10.1016/j.landusepol.2018.06.031
- Pickson, R. B., He, G., & Boateng, E. (2021). Impacts of climate change on rice production: Evidence from 30 Chinese provinces. Environment, Development and Sustainability, 24, 3907–3925. https://doi.org/10.1007/s10668-021-01594-8
- Redda, A., Tana, T., Alemayehu, Y., Hadgu, G., Elias, B., & Girma, A. (2022). Perceptions of climate change and determinants of adaptation decisions of smallholder maize (Zea Mays L.) farmers in Tigray. Northern Ethiopia.
- Robinson, S., Willenbockel, D., & Strzepek, K. (2012). A dynamic general equilibrium analysis of adaptation to climate change in Ethiopia. Review of Development Economics, 16(3), 489–502. https://doi.org/10.1111/j.1467-9361.2012.00676.x
- Sebsibie, S., Asmare, W., & Endalkachew, T. (2015). Agricultural technology adoption and rural poverty: A study on smallholders in Amhara Regional State, Ethiopia. Ethiopian Journal of Economics, 23(683–2017–950), 117–156.
- Sedebo, D. A., Li, G. C., Abebe, K. A., Etea, B. G., Ahiakpa, J. K., Ouattara, N. B., Olounlade, A., & Frimpong, S. (2021). Smallholder farmers’ climate change adaptation practices contribute to crop production efficiency in southern Ethiopia. Agronomy Journal, 113(6), 4627–4638. https://doi.org/10.1002/agj2.20900
- Sedebo, D. A., Li, G., Etea, B. G., Abebe, K. A., Ahiakpa, J. K., Arega, Y., & Anran, Z. (2022). Impact of smallholder farmers’ climate-smart adaptation practices on wheat yield in southern Ethiopia. Climate and Development, 1–15.
- Sertse, S. F., Khan, N. A., Shah, A. A., Liu, Y., & Naqvi, S. A. A. (2021). Farm households’ perceptions and adaptation strategies to climate change risks and their determinants: Evidence from Raya Azebo district, Ethiopia. International Journal of Disaster Risk Reduction, 60, 102255. https://doi.org/10.1016/j.ijdrr.2021.102255
- Shuaibu, M., & Nchake, M. (2021). Impact of credit market conditions on agriculture productivity in sub-Saharan Africa. Agricultural Finance Review, 81(4), 520–534. https://doi.org/10.1108/AFR-05-2020-0063
- Siyum, N., Giziew, A., & Abebe, A. (2022). Factors influencing adoption of improved bread wheat technologies in Ethiopia: Empirical evidence from Meket district. Heliyon, 8(2), e08876. https://doi.org/10.1016/j.heliyon.2022.e08876
- Solomon, R., Simane, B., & Zaitchik, B. F. (2021). The impact of climate change on agriculture production in Ethiopia: Application of a dynamic computable general equilibrium model. American Journal of Climate Change, 10(1), 32–50. https://doi.org/10.4236/ajcc.2021.101003
- Sustainable Development Goals Center for Africa (SDGC/A). (2019). Africa 2030: Sustainable Development Goals Three-Year Reality Check”.
- Sweeney, K. (2003). Implementing and interpreting sample selection models. Department of Political Science, The Ohio State University, USA. Political Research Lab
- Syed, A., Raza, T., Bhatti, T. T., & Eash, N. S. (2022). Climate impacts on the agriculture sector of Pakistan: Risks and amicable solutions. Environmental Challenges, 100433.
- Tabe Ojong, M. P. J., Hauser, M., & Mausch, K. (2022). Does agricultural commercialisation increase asset and livestock accumulation on smallholder farms in Ethiopia? The Journal of Development Studies, 58(3), 524-544. https://doi.org/10.1080/00220388.2021.1983170
- Tarekegn, K., & Ayele, A. (2020). Impact of improved beehives technology adoption on honey production efficiency: Empirical evidence from Southern Ethiopia. Agriculture & Food Security, 9(1), 1–13. https://doi.org/10.1186/s40066-020-00258-6
- Tazeze, A., Haji, J., & Ketema, M. (2012). Climate change adaptation strategies of smallholder farmers: The case of Babilie District, East Harerghe Zone of Oromia Regional State of Ethiopia. Journal of Economics and Sustainable Development, 3(14), 1–12.
- Tesfaye, S., Bedada, B., & Mesay, Y. (2016). Impact of improved wheat technology adoption on productivity and income in Ethiopia. African Crop Science Journal, 24(s1), 127–135. https://doi.org/10.4314/acsj.v24i1.14S
- Tesfaye, T., & Nayak, D. (2022). Climate change adaptation measures by farm households in Gedeo Zone. Ethiopia: An application of multivariate analysis approach. Environment, Development and Sustainability, 1–27. https://doi.org/10.1007/s10668-022-02185-x
- Teshome, H., Tesfaye, K., Dechassa, N., Tana, T., & Huber, M. (2021). Smallholder farmers’ perceptions of climate change and adaptation practices for maize production in Eastern Ethiopia. Sustainability, 13(17), 9622. https://doi.org/10.3390/su13179622
- Teye, E. S., & Quarshie, P. T. (2021). Impact of agricultural finance on technology adoption, agricultural productivity and rural household economic wellbeing in Ghana: A case study of rice farmers in Shai-Osudoku District. South African Geographical Journal, 4(2),1–20. https://doi.org/10.1080/03736245.2021.1962395
- Uddin, M. N., Bokelmann, W., & Dunn, E. S. (2017). Determinants of farmers’ perception of climate change: A case study from the coastal region of Bangladesh. American Journal of Climate Change, 6(1), 151–165. https://doi.org/10.4236/ajcc.2017.61009
- Vinholis, M. D. M. B., Saes, M. S. M., Carrer, M. J., & de Souza Filho, H. M. (2021). The effect of meso-institutions on adoption of sustainable agricultural technology: A case study of the Brazilian low carbon agriculture plan. Journal of Cleaner Production, 280, 124334. https://doi.org/10.1016/j.jclepro.2020.124334
- Warsame, A. A., Sheik-Ali, I. A., Ali, A. O., & Sarkodie, S. A. (2021). Climate change and crop production nexus in Somalia: An empirical evidence from ARDL technique. Environmental Science and Pollution Research, 28(16), 19838–19850. https://doi.org/10.1007/s11356-020-11739-3
- Wooldridge, J. M. (2002). Econometric analysis of cross section and panel data MIT press. Cambridge, MA, 108(2), 245–254.
- World Bank (2008). A country study on the economic impacts of climate change. Environment and Natural Resource Management Report, No. 46946-ET
- Yamane, T. (1973). Statistics: an introductory analysis–3.
- Yumbya, J., Maria, D., Vaate, B. D., Kiambi, D., Kebebew, F., & Rao, K. P. C. (2014). Assessing the effects of climate change on teff in Ethiopia: Implications for food security. Technical report.
- Zegeye, M. B., & Read, R. (2021). Adoption and ex-post impact of agricultural technologies on rural poverty: Evidence from Amhara Region, Ethiopia. Cogent Economics & Finance, 9(1), 1969759. https://doi.org/10.1080/23322039.2021.1969759
- Zeng, D., Alwang, J., Norton, G., Jaleta, M., Shiferaw, B., & Yirga, C. (2018). Land ownership and technology adoption revisited: Improved maize varieties in Ethiopia. Land Use Policy, 72, 270–279. https://doi.org/10.1016/j.landusepol.2017.12.047