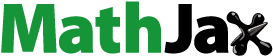
Abstract
This paper provides insights about the information content and predictive ability of the intrinsic value of the firm in an asset pricing context. The intrinsic value of a firm is of great importance for both the management and the investors of the company. We seek to assess whether the value-to-price (V/P) ratio, estimated with the residual income model (RIM), can explain the cross section of stocks returns. The study enhances the literature in the area of asset pricing by developing a new intrinsic value risk factor, which is a zero-investment portfolio that is neutral to the size, book-to-market equity ratio and the momentum effect. Furthermore, we incorporate in the RIM, for the first time, a time-series model that does not rely on analysts’ forecasts for the estimation of the key parameters of the model. A unique dataset from Greece, Italy, Spain and Portugal is utilized, from 31/12/2000 to 30/6/2019, that has a number of idiosyncrasies that are not observable in other developed markets, contributing by this way to the necessary accumulation of non-US research. The results show that the new intrinsic value risk factor absorbs the information content of the HML factor and explains better the cross section of returns, mainly for small size and high book to market value companies.
PUBLIC INTEREST STATEMENT
Finance and accounting researchers, analysts and investors have great interest in examining the intrinsic value of a company, the parameters that affect this value and the role of accounting numbers in the valuation process. Intrinsic valuation assumes that a firms’ value is a function of its expected future payoffs to common shareholders, based on currently available information, and the risk inherent in these payoffs. The present paper further tests whether the intrinsic value of a company, measured with the use of the Residual Income Model can explain the cross section of stocks returns. A unique dataset from Greece, Italy, Spain and Portugal is utilized, from 31/12/2000 to 30/6/2019, that has a number of idiosyncrasies that are not observable in other developed markets, contributing by this way to the necessary accumulation of non-US research.
1. Introduction
Ohlson (Citation1991, Citation1991) and Feltham and Ohlson (Citation1995), in their seminal work, develop the residual income model (RIM) that is used for the estimation of the intrinsic value of a company that provides a link between market values and accounting numbers. Almost all accounting valuation models that value the firm’s intrinsic value based on financial figures such as earnings, book value of equity, book-to-market ratio, etc., are based on some variant of the RIM (Callen, Citation2015). However, the RIM is an application of the Dividend Discount Model (DDM) and the real contribution of Ohlson comes from his modelling of the linear information dynamics (Dechow et al., Citation1999; Lo & Lys, Citation2000).
The present paper tests whether the intrinsic value of a firm, as estimated with the use of a valuation model, can explain the cross section of stocks returns. The intrinsic value (V) of the sample stocks is calculated with the use of the RIM and the resulting estimate of the fundamental value over the market value, i.e. the value-to-price (V/P) ratio, is used to investigate issues related to market efficiency and the predictability of cross-sectional stock returns. In doing so, we treat the intrinsic value of a company as an independent risk factor and examine whether intrinsic value is an asset pricing factor that can explain stock return variability, with the use of a dataset from Greece, Italy, Portugal and Spain that has a number of idiosyncrasies that are not observable in other developed markets.
There are a number of empirical studies that examine the ability of the RIM to explain cross-sectional prices and expected returns in the context of a valuation framework, using data from highly developed markets such the US and UK. Frankel and Lee (Citation1998), Herzberg (Citation1998), and Lee et al. (Citation1999) show that the V/P ratio is a better predictor of cross-sectional returns than book-to-market, firm size, earnings to price (E/P) and dividend to price (D/P). Abarbanell and Bernard (Citation2000) use the RIM to test whether the US stock market is myopic in the sense of overvaluing short-term earnings and undervaluing long-term earnings. Ali et al. (Citation2003) find that the V/P effect is partially concentrated around the future earnings announcements, consistent with the mispricing explanation. Kim et al. (Citation2009) expresse the RIM as a form of the value-to-book (V/B) ratio and find that their valuation measure predicts future returns more accurately. Hwang and Sohn (Citation2010) by regressing future abnormal stock returns, in a sample of US stocks, on the value-to-price ratios of the traditional model and the real option model separately, they find that the real option model has superior return predictability. Dissanaike and Lim (Citation2010), find the sophisticated valuation models, such as RIM, provide better investment strategies. Giamouridis and Montagu (Citation2014) find that the RIM and the option-based model of Hwang and Sohn (Citation2010) provide a strong capability to predict the cross section short-term equity returns. S.C. Lee et al. (Citation2014) show that the Ohlson model can forecast future stock price movements much more accurately in any predicted horizon. Hand et al. (Citation2017) show that the Ohlson (Citation1995) model appears to best explain stock prices. Finally, Pinochi et al. (Citation2019) find that the RIM proves to be extremely effective in signalling overvalued stocks and producing substantial long-short returns.
In contrast to all previous work in the area, we examine the predictive ability of the V/P ratio through the construction of a new risk factor, following the methodology of Fama and French (Citation1993), which captures the intrinsic value of firms. The new risk factor is developed for the first time and is one of the main novelties of the present work. The motivation behind the development of the new risk factor lies within the identification of risk factors that better capture the cross section of stock returns, which has become one of the most controversial areas in the financial economics literature. We introduce the new risk factor because, for the first time, we have developed a risk factor that is based upon the intrinsic value of a company. Up to now all other risk factors are based on other characteristics of a company besides its intrinsic value. Thus, the paper provides evidence towards this direction by introducing a new intrinsic value risk factor for the first time, resulting from the RIM, which has been ignored in the literature up to now. Most studies in the literature evaluate the ability of the RIM and its variants to capture future returns with the use of a contrarian investment strategy. The present work goes one step further and estimates the new intrinsic value risk factor in such a manner as to obtain a monotonic relation between risk and expected returns. This new risk factor is used for the first time in the classical asset pricing models CAPM, Fama-French 3 factor, Carhart 4 factor model), and if it is a priced factor, it should reduce the mean pricing error (absolute value of the intercept) of the asset pricing models.
Most of the studies that deal with the predictive power of the RIM and its variants rely on IBES analysts’ forecasts for the calculation of the intrinsic value. However, the use of analysts’ forecasts is only one way of implementing the RIM and it poses certain limitations to the application of the model. The number of sample stocks depends on the analysts’ coverage and there is always the issue of biased forecasts from analysts. Elgers and Murray (Citation1992) state that the information content of earnings that are impounded in stock prices is not fully incorporated in analysts’ forecasts. Furthermore, Cheng (Citation2005) finds that analysts do not consider the effect of conservative accounting when predicting future earnings and that simple reliance on forecasts made by equity analysts can lead to biased inferences if the variables under examination are correlated with the information items that analysts do not fully incorporate. Thus, the second main novelty of the study is the development of a new way to implement the RIM, which does not rely on analysts’ forecasts overcoming all the shortcomings of their use. Specifically, a time-series model is utilized, and for the first time incorporated into the RIM, for the estimation of the main parameters of the model, such as the future return on equity, in line with the work of Clubb and Naffi (Citation2007).
By using intrinsic value as an independent factor, the results of the present study can be converted to a practical tool, that is to say a successful investment strategy. It is an aspect where, to the best of our knowledge, it is the first to be undertaken in the Continental European, bank-oriented environment. The completely different politico-economical, legal, and institutional framework, the arm’s length system of USA and UK vs. the control-based system of Continental Europe (Drobetz & Pensa, Citation2007), justify the necessity of further exploration of the above relationship. Thus, the main objective of the present paper will be achieved through the analysis of a dataset from Greece, Italy, Portugal and Spain, i.e. four countries from Continental Europe that have a bank oriented economy, contributing in this way the necessary accumulation of non-US and non-UK research. Moreover, literature on the corporate governance issues suggest that there may be strong differences in corporate objectives between the “Anglo-American’ and the Continental European Financial systems-the maximization of shareholders vs. the maximization of all stakeholders objective”, Bancel and Mittoo (Citation2004). Furthermore, the Greek, Italian, Portuguese and Spanish legal system is different from that of the Anglo-Saxon countries. For instance, the American-British legal system is based on common law. The Greek, Italian, Portuguese and Spanish legal system (like the French and German) is based on code law traditions (Alves & Ferreira, Citation2011; Antoniou et al., Citation2008). The above-described institutional, economic and legal environment in the Southern European Region contains many idiosyncrasies that are not observable in other developed markets, thus making the investigation of the relationship between leverage and stock price performance very interesting.
The results showed that the new risk factor, which was developed for the first time and is based on the V/P ratio, enhances the predictive ability of the asset pricing models. Furthermore, the empirical results revealed that the new risk factor affects companies of small size and high book to market value. Finally, we found that the new risk factor absorbs the information content of the HML factor, which is based on the book value, which we derive from the financial statements of a company. Thus, the HML factor can be replaced by a new intrinsic value risk factor in the asset pricing models.
The rest of the paper structures as follows. Section 2 contains a description of the data sources and sample derivation. Section 3 provides information on the methodology. Empirical results of the research model appear in section 4. Finally, section 5 summarizes the research findings and concludes the paper.
2. Sample derivation
The sample used in the empirical tests consists of all non-financial companies listed on the Athens Stock Exchange (ASE), Borsa Italiana S.p.A (Milan Stock Exchange, MSE), NYSE Euronext Lisbon and Bolsa de Madrid (Madrid Stock Exchange) from 2000/12-2019/6. Stock prices, index market prices, market capitalization, accounting data of the sample firms and risk-free rates of return are sourced from Bloomberg Database. The number of sample firms ranges from 510 in 2000 to 628 in 2011, resulting in 128,592 monthly observations. All non-financial companies listed in the final year of the research are included in the initial sample. Also, firms de-listed each year are identified and consequently added to the initial sample. Companies that have changed name under the selected period are identified and treated as a single unit. Moreover, companies that either merged or are acquired over the study period are treated as a new unit following the event. By this way, a selection bias towards historically successful firms is limited to a great extent. Listed companies, which have been under suspension for more than 50% of year t, are excluded from the final sample. Moreover, firms with no available financial information for book, market equity or leverage for at least 12 months in a row are not included in the sample either. Financial data are necessary for the construction of fundamental variables for the various portfolios of each year of the research period. Following Fama and French (Citation1992), we also exclude companies with negative BE/ME ratios at 12/31 of year t–1. Last but not least, stock prices are adjusted for dividends and stock splits.
3. Methodology
The methodology is a based on the following residual income model, which is also called the Edwards-Bell-Ohlson (EBO) valuation technique (Bernard, Citation1995; Edwards & Bell, Citation1961; Feltham & Ohlson, Citation1995; Ohlson, Citation1990, Citation1991, Citation1991):
The model emphasizes the importance of abnormal earnings, the difference between earnings (NIt+i) and a charge for the use of equity capital that is measured by the product of previous periodCitation1990 book value (Bt+i-1) times the cost of equity (ke), for the estimation of the intrinsic value (Vt) of a firm. Specifically, it states that the value of a firm is equal to the sum of its book value (Bt) as of today and the present value of expected future residual income.
The first traces of the residual income model can be found in the early work of Preinreich (Citation2019), Edwards and Bell (Citation1961), and Peasnell (Citation1981, Citation1982) which was further formulated by Ohlson (Citation1991, Citation1991) and Feltham and Ohlson (Citation1995). The RIM is identical to the dividend discount model but expresses the value of the firm in accounting numbers and uses a broad measure of dividends, which are captured by the Clean Surplus Relation (CSR). The CSR dictates that all parameters that affect book value are also included in earnings, thus, it requires that the change in book value from one period to the other is equal to earnings minus dividends.
Expressing net income (NIt+i) in terms of return on equity (ROEt+i) and book value of the previous period (Bt+i-1) transforms Equationequation (1)(1)
(1) to:
Firms that are expected to generate ROE’s in excess of their required rate of return, will create wealth for their shareholders and trade at prices above their book value and vice versa. Firms that neither create nor destroy wealth will have an intrinsic value equal to their book value.
The RIM states the value of a firm as a function of infinite series of abnormal earnings. However, for practical reasons, the forecasted abnormal earnings must be finite; thus, a terminal value must be estimated. The terminal value will represent the value of the firm based on its abnormal earnings earned after the explicit forecasting period. The number of expanding periods (T) should be large enough to allow firms to reach their competitive equilibrium. In line with Frankel and Lee (Citation1998), we expand Equationequation (2)(2)
(2) for 3 periods where the future return on equity for year t + 2 (FROEt+2) is assumed to be earned in perpetuity:
To calculate the intrinsic value of the sample firms four parameters first must be estimated: a) the dividend payout ratio (DPO), b) the future returns on equity (FROEt, FROEt+1, FROEt+2), c) the forecasted book values (Bt, Bt+1, Bt+2) and d) the cost of equity (ke). The dividend payout ratio (DPO), which is the percentage of net income paid out in the form of dividends, is calculated by dividing the total dividends paid out in the most recent fiscal year by the respective net income amount.
The estimation of the forecasted returns on equity is accomplished with a company-specific AR1 time-series model, in line with the work of Clubb and Naffi (Citation2007), for 12 years up to year t-1:
Dechow et al. (Citation1999) and Fairfield et al. (Citation1996) show that the one-year time lag in the above regression adequately captures the time series of annual ROEs and that further decomposition of income statements add little predictive power.Footnote1 The estimated a0,t-1 and a1,t-1 coefficients from the above regression are then used to forecast FROEs for the next 3 years, which are used in conjunction with the dividend payout ratio to estimate the forecasted book values (Bt, Bt+1, Bt+2). It should be noted that the accuracy of the predictions from the AR1 time-series models depends upon their stability:
According to Lo and Lys (Citation2000) when estimating the fundamental values it is important to obtain firm-specific parameters and discount rates. Thus, a firm-specific cost of equity (ke) is adopted, in line with Lee et al. (Citation1999), Abarbanell and Bernard (Citation2000), and Dissanaike and Lim (Citation2010), that reflects the risk premium demanded by market participants to invest in the specific company. The excess return (Rs-Rf) of each sample stock is regressed against the historical market risk premium (RM-Rf) over a five-year rolling window of monthly returns in order to obtain the beta coefficient of the firm:
The cost of equity is then estimated with the use of the following equation:
Having estimated the intrinsic value of each sample firm the Value-to-Price ratio (V/P) is calculated by dividing the value estimation by the market price of the stock at the end of June of each year of the research. The methodology involves for each sample firm the calculation of a) value estimates once a year at 30/6 and b) monthly returns for the following twelve-month period. We choose to use a six-month time lag between the end of the fiscal year and the value estimation date in order to ensure that all fundamental data of the sample firms are well known at the time of the value estimation by market participants. Furthermore, the use of end-of-June stock prices minimises the January effect.
Next, all stocks at the end of June of each year t from 2000 to 2018 are ranked on their size, book-to-market equity ratio οn 31/12 of the previous year (BEt-1/MEt-1), average monthly return of the previous year and V/P ratio independently and allocated into a) two size portfolios (small and large capitalization firms), b) three ΒE/ΜΕ groups (30% stocks low ΒE/ΜΕ portfolio, 40% medium ΒΕ/ΜΕ portfolio and 30% high ΒΕ/ΜΕ portfolio), c) three momentum portfolios (“winner“ top 30% stocks with the highest last year average return, ‘lοser’ portfolio bottom 30% stocks and the ”medium” portfolio 40% stocks) and d) three intrinsic value portfolios (30% of stocks low V/P portfolio, 40% medium V/P portfolio and 30% high V/P portfolio).
The above portfolios are used for the construction of the following risk factors: a) SMB (Small Minus Big), a portfolio long on small-sized stocks and short on big-sized stocks and neutral on the book value, momentum, and intrinsic value effect, b) HML (High Minus Low), a portfolio that is long on high BE/ME stocks and short on low BE/ME stocks and is neutral on the size, momentum, and intrinsic value effect, c) WML (Winners Minus Looser), a portfolio that is long on winner stocks and short on loser stocks and is neutral on the size, book value and intrinsic value effect and d) HVMLV (High Value Minus Low Value), a portfolio long on high V/P ratio stocks and short on low V/P ratio stock, which is neutral on the size, book value and momentum effect.
The intrinsic value risk factor (HVMLV) is constructed in such a manner as to obtain a monotonic relation between risk and expected returns. If HVMLV is a priced factor, it should reduce the mean pricing error (absolute value of the intercept) of the other pricing models examined. The models that are examined are predictive regressions in the form:
where (Ri-Rf)t is the excess returns of stock portfolios in month t, (RM-Rf)t is the market risk premium in month t, using as a market proxy the EURO STOXX 50 index and as the risk-free rate of return the 12-month Treasury-Bill of each country (Greece, Italy, Spain, Portugal), HVMLV is the return of the intrinsic value risk factor for month t, Xt is a vector of the return of control variables, which consists of the common risk factors that have proven to explain stock returns (SMB, HML and WML) and γ’ is the vector of coefficient estimates on the control variables. All regression models are estimated with and without the HVMLV risk factor, in order to observe the change in the explanatory power of the independent variables (i.e. statistical significance and numerical value of the parameters) and the explanatory power of the asset pricing models. The main dependent variable is the excess return of the three (low, medium, high) intrinsic value portfolios. Furthermore, and in order to ensure the robustness of the results, three additional sets of dependent variables are examined a) six portfolios formed at the intersection of the two size and the three V/P deciles and b) nine portfolios formed at the intersection of the three V/P and the three ΒΕ/ΜΕ deciles, c) 54 portfolios formed at the intersection of all the factors.
4. Empirical results
4.1. Descriptive statistics
The findings from the descriptive statistics of the research variables () are in line with those of Banz (Citation1981), Jegadeesh and Titman (Citation1993), Fama and French (Citation1992, Citation1993, Citation1995, Citation1996, Citation1998), Rouwenhorst (Citation1998), and Liew and Vassalou (Citation2014) and support the presence of a value, size and momentum effect.
Table 1. Descriptive Statistics—Variables of the research models
The MRP (market risk premium) has a positive return, as expected. The SMB mimicking portfolio, that proxies for the size effect, has a statistically significant positive average and median returns displaying than small-sized firms have higher returns than big-sized firms. The HML mimicking portfolio, that is long on high book-to-market stocks and short on low book-to-market stocks, has a positive average and median value that is statistically significant at the 5% level of significance. The average and median returns of the WML portfolio show that stocks with high past performance (winners) continue to have above average returns. Finally, the HVMLV portfolio exhibited a positive and statistically significant return indicating that firms with high intrinsic value as compared to their market price outperform firms with low intrinsic value. Furthermore, the average return of the HVMLV factor was the largest among the other risk factors, indicating the profitability of an investment strategy based on the intrinsic value of the firm.
4.2. Regression analysis
The dependent variable in the first step of the analysis is the excess return of the low, medium and high V/P portfolios denoted 1, 2 and 3, respectively, in the first column of . In the first specification of the model the independent factors are the CAPM market risk premium and the HVMLV risk factor (, Panel A), the second specification adds to the analysis the Fama & French SMB and HML risk factors as independent factors (, Panel B), and in the final specification, the Carhart WML factor is added in the model (, Panel C).
Table 2. Regressions of V/P Portfolios against Risk Factors
The factor loadings for the market risk premium turn out as expected, i.e. they are positive, statistically significant and range from 0.47 to 0.54 for all V/P ratio portfolios and model specifications. The low variability of the market risk premium coefficient among the V/P ratio portfolios, for all three specifications of the model, indicates that it cannot capture alone the different risk profiles of the respective portfolios. Thus, it would seem appropriate to add additional explanatory variables to the asset pricing model.
The coefficients of the SMB factor are positive for all cases, ranging from 0.11 to 0.85, revealing a positive relationship between the returns of the V/P ratio portfolios and the size risk factor. Furthermore, it should be noted that the coefficient of the SMB factor takes its largest value at the low V/P ratio portfolios in both models; thus, the size premium is larger for portfolios of firms with low intrinsic value as compared to their market value. Turning to the HML factor, the results show a positive relationship between the returns of the V/P ratio portfolios and the HML mimicking portfolio. When the new HVMLV factor is taken into account, then the HML factor loses its statistical significance. This shows that the new agent essentially absorbs all of the HML factor information.
The HML portfolio captures the value effect, which is measured based on the book value of equity. Thus, the HML factor is based upon accounting data derived from the financial statements of the company. On the other hand, the HVMLV factor also captures the value effect; however, it is based upon the intrinsic value of a company, which is derived from the RIM that takes into account the future prospects of the company along with its risk profile. The striking difference between the HML and the HVMLV factor is that the former is based on accounting data, that reflect the current financial position of a companion, whereas the latter captures the future prospects and the risk profile of the company. The loss of the statistical significance of the HML factor when the newly developed HVMLV risk factor is included in the empirical models reveals the superiority of forward-looking projections in valuing companies.
The step-wise regression methodology provides insights on the incremental power contained in each independent factor. The addition of the momentum factor (WML) does not have as a result a clear pattern in the factor loading, since it ranges from a negative value of −0.32 to a positive value of 0.16 and its statistical significance is not stable. Finally, the coefficients of the HVMLV factor are positive for all cases, ranging from 0.02 to 0.93, taking the highest value at the high V/P ratio portfolios.
The coefficient of the intrinsic value risk factor is positive in model specifications and increases monotonically as we move from the low to the highest V/P portfolios. Thus, when the return of the HVMLV factor rises by 1% we expect that the excess returns of all portfolios will have a percentage increase as their factor loading. Furthermore, in order to examine in depth the importance of the HVMLV risk factor in forecasting the cross section of stock returns, the adjusted R2 is reported for all regression models with and without the HVMLV risk factor. It can be seen that the addition of the intrinsic value risk factor enhances the explanatory power of the asset pricing models for all V/P portfolios and model specifications (increased adjusted R2).
Finally, the constant terms in most of the models are statistically significant. The implication of this finding is that the dependent variable, the performance of portfolios, will exhibit abnormal returns, which cannot be explained by the five-factor model. Thus, the inclusion of the intrinsic value risk factor, though it enhances the predictability of the model, it does not appear to fully explain the variability of stock returns. It should be noted that we report the values of the regression coefficients along with the constant only when the HVMLV factor is included in the model. This is done due to space considerations, and the full set of results is available upon request.
In the next step of the analysis, we control for the size effect by performing the regressions with two additional sets of dependent variables. Specifically, in the first case, the dependent variables are the excess returns of the six portfolios formed at the intersection of the two size and three V/P deciles (Small Cap-Low V/P, Small Cap-Medium V/P, Small Cap-High V/P, Large Cap-Low V/P, Large Cap-Medium V/P, and Large Cap-HighV/P) and the results are reported in .
Table 3. Regressions of V/P portfolios against MRP and HVMLV after controlling for size
Table 4. Regressions of V/P portfolios against Fama-French factors and HVMLV after controlling for size
Table 5. Regressions of V/P portfolios against Carhart factors and HVMLV after controlling for size
When we control for size, the alpha coefficients turn out to be statistically significant along with the market risk premium which is once again positive. We observe that the loading of the new risk factor increases as we move from portfolios consisting of companies with a low V/P ratio to portfolios of companies with a high V/P ratio. This essentially means that the HVMLV factor plays a greater role in undervalued companies than in overpriced companies. Furthermore, the loading of the HVMLV risk factor takes its higher values at the small cap portfolios revealing the particular importance of the intrinsic value factor in small size companies.
presents the results of the regression of the portfolios of size and intrinsic value against the factors of the Fama & French model with the intrinsic value factor. Once again, we find a positive correlation between the returns of the size and value portfolios with the market risk premium, which is statistically significant in all cases, and a positive correlation between the returns of the size and value portfolios with the size factor (SMB) and the book value factor (HML), which is statistically insignificant in many cases.
From the regressions that include the new intrinsic value factor (HVMLV), the results are largely similar to the results of the first step of the analysis, in terms of the coefficients of the market risk premium and the Fama & French risk factors. What is worth mentioning, however, is the fact that the HML factor loses any statistical significance when the new HVMLV factor is entered into the regression equation. Also, we observe is the increased explanatory capacity of the model, when we include the new factor of internal value HVMLV. Finally, it should be noted is that there is a positive correlation between the returns of the dependent variables and the intrinsic value factor. The factor loadings take their highest values in small value portfolios, indicating the greater importance of a firm’s intrinsic value to these companies.
below presents the results of the regression of portfolios of size and intrinsic value against Carhart model factors with the intrinsic value factor. The first group of regressions substantially confirms the results of the previous analysis, which shows a positive correlation between the returns of portfolios of size and value with the market risk premium, which is statistically significant in all cases, and a positive correlation between the returns of the size and value portfolios with the size factor (SMB) and with the book value factor (HML), which is statistically insignificant in most cases.
The results also show that the momentum factor (WML) has a negative relationship with the performance of the dependent variables, which is statistically significant in all cases. The relationship between the momentum factor and the returns of dependent portfolios is higher in absolute terms for portfolios consisting of large companies, which essentially means that the momentum factor is more important for large companies in terms of size. Turning now to the results of regressions with the new risk factor, we observe that there is a statistically significant relationship, mainly in small-cap portfolios, revealing the special importance of the intrinsic value factor in small businesses.
The third step in the analysis involves as dependent variables the excess returns of the nine portfolios formed at the intersection of the three V/P and three ΒΕ/ΜΕ deciles (High BE/ME-Low V/P, High BE/ME-Medium V/P, High BE/ME-High V/P, Medium BE/ME-Low V/P, Medium BE/ME-Medium V/P, Medium BE/ME-High V/P, Low BE/ME-Low V/P, Low BE/ME-Medium V/P, and Low BE/ME-High V/P) and the results are reported in .
Table 6. Regressions of V/P portfolios against MRP and HVMLV after controlling for BE/ME ratio
Table 7. Regressions of V/P portfolios against Fama-French factors and HVMLV after controlling for BE/ME ratio
Table 8. Regressions of V/P portfolios against Carhart Factors and HVMLV after controlling for BE/ME ratio
Controlling for the Book Value effect further corroborates our previous results. As it can be seen in all regressions have an alpha coefficient which is non-statistically significant. The beta coefficient is positive and statistically significant in all cases and remains relatively constant, regardless of the type of portfolio we are referring to. Furthermore, for the loading of the new risk factor is statistically significant in all portfolios but takes the highest values in portfolios that have a high B/P ratio, which means that the new risk factor has a greater impact on companies that are fairly valued.
below presents the results of the portfolio regressions constructed on the basis of book value and intrinsic value and the Fama-French factors with the new intrinsic risk factor. Market risk premium has a positive and strong statistically significant correlation with all portfolios. The size factor (SMB) shows a positive correlation with all portfolios, without much variation in terms of the size of the loading. The book value factor is non-statistically significant in most cases.
The results are similar with the regressions where we have taken into account the new intrinsic value risk factor, in terms of sign, magnitude and statistical significance of the factor loadings. Furthermore, the explanatory power of the model, in all cases, increases when the new intrinsic value factor is included in the analysis.
below presents the results of the regressions that have as dependent variable the returns of the portfolios constructed based on the book value and intrinsic value of the sample companies and as independent variables the returns of the four traditional risk factors (market risk premium, size, book value and momentum), as well as the returns of the new risk factor. It appears that there is once again a positive and strong relationship between the returns of the dependent variables and the market risk premium. The size and the book value risk factor are positively correlated with the returns of the portfolios and this relationship is statistically insignificant in most cases, with the inclusion of the new intrinsic risk factor. This means that the new risk factor absorbs a significant part of the information of the two previous factors. Finally, the existence of the new risk factor does not affect the importance of the momentum risk factor.
HVMLV risk factor.
An alternative method for interpreting the intercepts of the regression models is by employing the Gibbons et al. (Citation1989) GRS statistic to test the hypothesis H0: ai = 0 i, or simply to test the intercepts jointly. The GRS test is performed by running all 36 regression models and computing the intercepts or alphas and then testing whether the alphas are jointly zero. As the estimates of intercepts increase in absolute value, so will the value of the GRS statistic. The equation for the GRS test is presented below Footnote2.
where:
T = number of observations
N = number of dependent variables
k = number of explanatory variables in the regression
â = Ν x 1 vector of estimated intercepts
Ŝ = unbiased estimates of the residual covariance matrix
μκ = k x 1 vector of the factor portfolios’ means
Ω = unbiased estimates of the factor portfolios’ covariance matrix
A larger value of the GRS statistic indicates that intercepts are jointly different from zero and, by extension, the factors of the model cannot adequately explain the variation of returns for a portfolio. A small p-value indicates that we can reject the null that H0: ai = 0 for all i’s.
The GRS statistics are shown in . From the comparison of the 36 empirical models, it is evident that when the intrinsic value risk factor (HVMLV) is added as an explanatory variable, then the predictive power of the model increases, as it is indicated by a lower GRS statistic.
Table 9. GRS statistics of the empirical models
5. Conclusions, policy implications and suggestions for further research
In the present study, the RIM was used in a sample of Greek, Italian, Spanish and Portuguese firms to provide evidence of whether the resulting V/P ratio can explain the cross section of stocks returns. In doing so, an intrinsic value risk factor was constructed, and it was examined if it is a priced risk factor in an asset pricing context. The results of the study contribute to the literature of both the residual income model and asset pricing models in several ways. The utilization of a time series analysis for the estimation of the main parameters of the RIM, complements previous research in the area of the RIM that use analysts’ forecasts. In doing so, the study provides evidence on the usefulness of historical data, which are easily available to investors, for the estimation of the intrinsic value of a firm. Finally, the empirical results show that the resulting V/P ratio can be used as a basis for either a profitable investment strategy or for the construction of an intrinsic value risk factor, which was shown to be priced in the cross-section of the sample stock returns.
The results of the regression models showed that the market risk premium is quite stable, in terms of size and sign, while it shows very little volatility between value to price portfolios. Based on this finding, we conclude that the market risk premium, by itself, is not able to capture the different risk profiles of intrinsic value-to-price portfolios. Therefore, based on the results, the market risk premium, although proved to be a very important factor and should be present in all asset valuation models, it does not, in any way, contain all the information about the cross section of future returns of assets. This finding is in stark contrast to the core assumptions of the capital asset pricing model (CAPM), which argues that market risk premium and the corresponding beta coefficient are the only systemic risk factor. Our analysis clearly shows that there is more than one factor that affects the performance of risky assets.
The loading of the size risk factor (SMB) has a positive correlation with the excess returns of the V/P portfolios, in all models, while its highest value is always in the portfolios of small V/P index, which shows that the effect of size plays a more important role in companies that are overpriced (low V/P). The coefficient of the value risk factor (HML) is positive in all regression models and has a higher absolute value in high V/P index portfolios but loses its statistical significance when the HVMLV factor is included in the valuation model. This essentially means that the new risk factor absorbs the information content of the traditional HML factor and that intrinsic value, as derived from the RIM, contains more information content than book value to explain the cross section of returns. Finally, the HVMLV is positive for all regression models, with the highest value being in high V/P portfolios. The implication of this finding is that we can conclude that the V/P ratio plays a greater role in companies that are undervalued than in companies that are overvalued.
In the second part of the analysis, the portfolios that are the dependent variables are constructed considering two different factors. The first factor is the market capitalization and the second is the ratio of intrinsic value to price (V/P). In this way, we essentially control the results of the regressions for the effect of the size of the company on the intrinsic value.
The results showed, once again, that the beta coefficient of all regressions is positive and statistically significant. This means that the returns of the size and intrinsic value portfolios increase as the market risk premium increases. Also, the coefficient of the new risk factor is positive and statistically significant, especially in portfolios consisting of low size companies, which means that the intrinsic value factor plays a larger role in small companies in terms of size. Therefore, the impact of the new risk factor mainly concerns small companies, which are usually companies that show high growth rates and trade at low intrinsic value to price ratios. Furthermore, the momentum factor (WML) has a negative relationship with the performance of the dependent variables, which is statistically significant in all cases. The relationship between the momentum factor and the returns of the dependent portfolios is higher in absolute terms for portfolios consisting of large companies, which essentially means that the momentum factor is more important for large companies. Turning now to the results of regressions with the new risk factor, we observe that this is statistically significant mainly in small capitalization portfolios, revealing the special importance of the intrinsic value factor in small companies. Finally, we observe that the loading of the new risk factor increases as we move from portfolios consisting of companies with a low V/P ratio to portfolios with companies with a high V/P ratio. This essentially means that the HVMLV factor plays a greater role in undervalued companies than overpriced companies, something that has been seen from our previous analysis and essentially here we confirm our initial findings.
The final stage of the empirical research involved regressions where the dependent variable is the excess return of two-dimensional portfolios, which are based on the book value and the V/P ratio, thus, exploring in combination the impact of the new risk factor on the accounting and intrinsic value of companies. The results show that the loading of the new risk factor HVMLV, which is based on the V/P index and essentially reflects the relationship between intrinsic value, as calculated by the residual income model, and current value, or other words whether a company is overvalued or undervalued, has a positive correlation with the excess returns of all portfolios, regardless of size characteristics, book value over market value, momentum and intrinsic over market value. What is even more important, however, is that the adjusted coefficient of determination, for all regressions, is higher when we take into account the new risk factor, which means that the HVMLV factor improves the explanatory power of the valuation models.
In summary, the creation of the new risk factor helps to better explain the cross section of returns. Also, the new risk factor affects companies of small size and high book-to-market value. Finally, the new risk factor absorbs the information content of the HML factor, which is based on the book value, which we derive from the financial statements of a company. The implications of our results are that market participants and investment professionals may adopt the RIM for the formation of a profitable investment strategy. Furthermore, it was shown that investors can use, the easily available, historical data for the estimation of the intrinsic value of a firm and do not have to rely only on analysts’ forecasts. Finally, investors and financial analysts should take into account, except the size and book value risk factors, the intrinsic value risk factor as an important asset pricing factor when estimating the required rate of return of a company or its cost of capital or when they evaluate and attribute the performance of professionally managed portfolios.
Future studies could examine the consistency with the findings of the present study if the forecasts are made on the basis of analysts’ forecasts or other methods of forecasting the firm’s cash flows, such as suggested by Dechow et al., 1997. The methodology of the present paper involves the application of the RIM with the use of forecasts for the future ROE of the sample firms. Future research may focus on forecast in other key parameters of the model such as predicting future dividend rates or how capital costs are estimated for sample firms. In order to further investigate, it would be very useful to examine the same phenomenon over a longer period of time with a larger number of firms in order to examine whether the results and main findings of the present study remain consistent over time and are independent of the countries concerned.
Disclosure statement
No potential conflict of interest was reported by the author(s).
Additional information
Funding
Notes on contributors
Panagiotis G. Artikis
Panagiotis G. Artikis is a Professor at the University of Piraeus, Department of Business Administration. He is the deputy president of the Department of BA. His research, professional and teaching interests are in the areas of corporate finance, portfolio management, risk management, asset pricing, financial modeling and wealth creation. He has published several papers in international refereed journals, edited volumes and conferences. Prof. Artikis has over 20 years of teaching experience, and he has supervised numerous MBA dissertations and Doctoral Theses. Furthermore, he has received for several years the teaching excellence award from the Athens University of Economics and Business for his work at the Executive MBA.
Christos G. Kampouris
Christos G. Kampouris is an Academic Fellow at the University of Piraeus, Department of Tourism Studies. His research, professional and teaching interests include corporate finance, asset pricing, portfolio management and risk management.
Notes
1. The FROEs were also estimated with an alternative regression model proposed by Beaver & Ryan (2000), FROEt = a0,t-1 + a1,t-1BE/MEt-1 + a2,t-1 ln(1+ ROEt-1). The results, however, did not differ significantly, thus, for sake of brevity are not reported.
2. The GRS statistic has an F distribution with N and T—N—K + 1 degrees of freedom under the assumption that the returns of the explanatory variables are normal, and the true intercepts are zero.
References
- Abarbanell, J., & Bernard, V. (2000). Is the U.S. stock market myopic? Journal of Accounting Research, 38(2), 221–20. https://doi.org/10.2307/2672932
- Ali, A., Hwang, L., & Trombley, M. (2003). Residual-income-based valuation predicts future stock returns: Evidence on mispricing vs. risk explanations. The Accounting Review, 78(2), 337–396. https://doi.org/10.2308/accr.2003.78.2.377
- Alves, P., & Ferreira, M. (2011). Capital structure and law around the world. Journal of Multinational Financial Management, 21(3), 119–150. https://doi.org/10.1016/j.mulfin.2011.02.001
- Antoniou, A., Guney, Y., & Paudyal, K. (2008). The determinants of capital structure: Capital market oriented versus bank-oriented institutions. Journal of Finance and Quantitative Analysis, 43(1), 59–82. https://doi.org/10.1017/S0022109000002751
- Bancel, F., & Mittoo, U. (2004). Cross country determinants of capital structure choice: A Survey of European firms. Financial Management, 33(4), 103–132. https://www.jstor.org/stable/3666330
- Banz, R. W. (1981). The relationship between return and market value of common stocks. Journal of Financial Economics, 9(1), 3–18. https://doi.org/10.1016/0304-405X(81)90018-0
- Bernard, V. (1995). The Feltham-Ohlson framework: Implications for empiricists. Contemporary Accounting Research, 11(2), 733–747. https://doi.org/10.1111/j.1911-3846.1995.tb00463.x
- Callen, J. L. (2015). A selective critical review of financial accounting research. Critical Perspectives on Accounting, 26(C), 157–167. https://doi.org/10.1016/j.cpa.2013.03.008
- Cheng, Q. (2005). The role of analysts’ forecasts in accounting-based valuation: A critical evaluation. Review of Accounting Studies, 10(1), 5–31. https://doi.org/10.1007/s11142-004-6338-4
- Clubb, C., & Naffi, M. (2007). The usefulness of book-to-market and ROE expectations for explaining UK stock returns. Journal of Business Finance and Accounting, 34(1–2), 1–32. https://doi.org/10.1111/j.1468-5957.2006.00662.x
- Dechow, P., Hutton, A., & Sloan, R. (1999). An empirical assessment of the residual income valuation model. Journal of Accounting and Economics, 26(1–3), 1–34. https://doi.org/10.1016/S0165-4101(98)00049-4
- Dissanaike, G., & Lim, K.-H. (2010). The Sophisticated and the simple: The profitability of Contrarian Strategies. European Financial Management, 16(2), 229–255. https://doi.org/10.1111/j.1468-036X.2008.00466.x
- Drobetz, W., & Pensa, P. (2007). Capital structure and stock returns: The European evidence. University of Hamburg, Working paper. http://ssrn.com/abstract=957302
- Edwards, E. O., & Bell, P. W. (1961, January) The theory and measurement of business income. University of California Press.
- Elgers, P., & Murray, D. (1992). The relative and complementary performance of analyst and security-price-based measures of expected earnings. Journal of Accounting and Economics, 15(2–3), 303–316. https://doi.org/10.1016/0165-4101(92)90022-T
- Fairfield, P. M., Sweeney, R. J., & Yohn, T. L. (1996). Accounting classification and the predictive content of earnings. Accounting Review, 71(3), 337–356. https://www.jstor.org/stable/248292
- Fama, E., & French, K. (1992). The cross-section of expected stock returns. Journal of Finance, 47(2), 427–465. https://doi.org/10.1111/j.1540-6261.1992.tb04398.x
- Fama, E., & French, K. (1993). Common risk factors in the returns on stocks and bonds. Journal of Financial Economics, 33(1), 3–56. https://doi.org/10.1016/0304-405X(93)90023-5
- Fama, E., & French, K. (1995). Size and book–to–market factors in earnings and returns. Journal of Finance, 50(1), 131–156. https://doi.org/10.1111/j.1540-6261.1995.tb05169.x
- Fama, E., & French, K. (1996). Multifactor explanations of asset pricing anomalies. Journal of Finance, 51(1), 55–84. https://doi.org/10.1111/j.1540-6261.1996.tb05202.x
- Fama, E., & French, K. (1998). Value versus growth: The international evidence. Journal of Finance, 53(6), 1975–1999. https://doi.org/10.1111/0022-1082.00080
- Feltham, G. A., & Ohlson, J. A. (1995). Valuation and clean surplus accounting for operating and financial activities. Contemporary Accounting Research, 11(2), 689–731. https://doi.org/10.1111/j.1911–3846.1995.tb00462.x
- Frankel, R., & Lee, C. M. C. (1998). Accounting valuation, market expectation, and cross-sectional stock returns. Journal of Accounting and Economics, 25(3), 283–319. https://doi.org/10.1016/S0165-4101(98)00026-3
- Giamouridis, D., & Montagu, C. (2014). The sophisticated and the simple: The profitability of contrarian strategies from a portfolio manager’s perspective. European Financial Management, 20(1), 152–178. https://doi.org/10.1111/j.1468-036X.2011.00627.x
- Gibbons, M., Ross, S., & Shanken, J. (1989). Test of the efficiency of a given portfolio. Econometrica, 57(5), 1121–1152. https://doi.org/10.2307/1913625
- Hand, J. R. M., Coyne, J. G., Green, J. R., & Zhang, X. F. (2017). The use of residual income valuation methods by US sell-side equity analysts. Journal of Financial Reporting, 2(1), 1–29. https://doi.org/10.2308/jfir-51932
- Herzberg, M. (1998). Implementing EBO/EVA analysis in stock selection. Journal of Investing, 7(1), 45–53. Spring. https://doi.org/10.3905/joi.1998.408447
- Hwang, L., & Sohn, B. (2010). Return predictability and shareholders’ real options. Review of Accounting Studies, 15(2), 367–402. https://doi.org/10.1007/s11142-010-9119-2
- Jegadeesh, N., & Titman, S. (1993). Returns to buying winners and selling losers: Implications for stock market efficiency. Journal of Finance, 48(1), 65–91. https://doi.org/10.1111/j.1540-6261.1993.tb04702.x
- Kim, K., Lee, C., & Tiras, S. (2009). Residual income valuation: A new approach based on the value-to book multiple. https://doi.org/10.2139/SSRN.1465855
- Lee, C. M. C., & Swaminathan, B. (1999). Valuing the Dow: A bottom-up approach. Financial Analyst Journal, 55(5), 4–23. https://doi.org/10.2469/faj.v55.n5.2295
- Lee, C. M. C., Myers, J., & Swaminathan, B. (1999). What is the intrinsic value of the Dow? The Journal of Finance, 54(5), 1693–1741. https://doi.org/10.1111/0022-1082.00164
- Lee, S. C., Chen, J. L., & Tsa, M. S. (2014). An empirical investigation of the Ohlson model–a panel cointegration approach. Australasian Accounting Business and Finance Journal and Authors, 8(2), 35–51. http://dx.doi.org/10.14453/aabfj.v8i2.4
- Liew, J., & Vassalou, M. (2000). Can book-to-market, size and momentum be risk factors that predict economic growth? Journal of Financial Economics, 57(2), 221–245. https://doi.org/10.1016/S0304-405X(00)00056-8
- Lo, K., & Lys, T. (2000). The Ohlson model: Contribution to valuation theory, limitations and empirical Implications. Journal of Accounting, Auditing and Finance, 15(3), 321–331. https://doi.org/10.1177/0148558X0001500311
- Ohlson, J. (1990). A Synthesis of security valuation theory and the role of dividends, cash flows, and earnings. Contemporary Accounting Research, 6(2), 648–676.
- Ohlson, J. (1991). The theory of value and earnings, and an introduction to the Ball-Brown analysis. Contemporary Accounting Research, 8(1), 1–19.
- Ohlson, J. (1995). Earnings, book values, and dividends in equity valuation. Contemporary Accounting Research, 11(2), 661–687. https://doi.org/10.1111/j.1911-3846.1995.tb00461.x
- Peasnell, K. (1981, June). On capital budgeting and income measurement. Abacus, 17(1), 52–67. https://doi.org/10.1111/j.1467-6281.1981.tb00101.x
- Peasnell, K. (1982, October). Some formal connections between economic values and yields and accounting numbers. Journal of Business Finance and Accounting, 9(3), 361–381. https://doi.org/10.1111/j.1468-5957.1982.tb01001.x
- Pinochi, M., Fais, F., & Corsiglia, M. (2019). Residual income model and abnormal returns: A comparison to factor styles and sell-side analysts. Business Valuation OIV Journal, 1(1), 19–46. https://www.fondazioneoiv.it/wp-content/uploads/2019/06/Residual-Income-Model.pdf
- Preinreich, G. (1938). Annual survey of economic theory: The theory of depreciation. Econometrica, 6(3), 219–241. https://doi.org/10.2307/1907053
- Rouwenhorst, K. (1998). International momentum strategies. Journal of Finance, 53(1), 267–284. https://doi.org/10.1111/0022-1082.95722