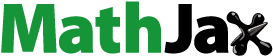
Abstract
The purpose of this study is twofold: First, we examine the capital adjustment process using a partial adjustment framework and second, we test whether capitalization impacts the credit growth of microfinance institutions (MFIs) through the deviation (i.e. the divergence between the actual capital ratio and the implicit long-run target capital). To this end, we use an unbalanced panel dataset of 127 MFIs across 31 countries in Sub-Saharan Africa (SSA) during 2004–2014. We apply the Arellano-Bover/Blundell-Bond two-step Generalized Method of Moments (GMM) Windmeijer bias-corrected standard errors for estimating both the capital and lending models. Standard errors for the long-run effects in the capital equation are approximated with the Delta method. Our findings reveal that profitability contributes to the capitalization of MFIs, whereas portfolio risk and liquidity are negatively associated with MFI capital. We also find that large-scale MFIs have lower capitalization, while small-scale MFIs have higher capitalization relative to medium scale MFIs consistent with the “too big to fail” hypothesis. Nevertheless, we uncover that the legal status of MFIs, deposit growth and economic growth have no direct effects on capitalization. The findings also confirm that there is a capital adjustment difficulty in the microfinance industry in SSA. The constant of the model is also statistically significant and has the highest economic significance suggesting that the capital ratio fluctuates mainly around a firm-specific unobserved time-invariant component. The findings, however, fail to support the hypothesis that capitalization impacts MFI lending behavior through the deviation.
1. Introduction
In many countries, the microfinance sector is now an integral constituent of the financial system (Tchakoute Tchuigoua, Citation2016). In order to increase the resilience of the financial system, adequate capitalization is highly essential. Capitalization refers to the level of equity capital a financial institution has maintained and serves as a buffer against actual, anticipated, and unforeseen losses and an impetus to manage risk-taking behavior. Accordingly, capital requirement has become an important regulation area in financial institutions. Moreover, capital requirement is also one of the major regulation areas of microfinance institutions (MFIs) like commercial banks. In addition to regulatory authorities, market participants might also require MFIs to keep adequate capitalization as it determines MFI solvency.
Unlike banks, MFIs have fewer options to raise capital and might also exhibit higher risk profile and hence, a higher capital adequacy ratio may be essential (Basel Committee on Banking Supervision (BCBS), Citation2010). MFIs are generally highly capitalized financial institutions (Tehulu, Citation2021a). However, the level of capitalization among MFIs varies significantly (Basel Committee on Banking Supervision (BCBS), Citation2010; Tehulu, Citation2021a). Hence, empirical studies are imperative to investigate what determines MFI capitalization. Savings programs for MFIs might not, in most cases, provide the necessary funding to build a financially self-reliant institution given the poor has small sums to save. This research, therefore, considers an alternative form of financing—equity financing—and investigates the capital adjustment process in MFIs.
Since the 1988 Basel capital accord, several studies have also examined the role of capitalization in the lending behavior of financial institutions (Bernanke & Lown, Citation1991; Berrospide & Edge, Citation2010; Gambacorta & Shin, Citation2018; Thibaut & Mathias, Citation2014). The capital crunch hypothesis implies that the most recent level of the capital–asset ratio is relevant to future lending since it is the current level that must meet regulatory standards (Bernanke & Lown, Citation1991). In this respect, the prior empirical studies have followed three different frameworks to examine the capitalization and lending nexus. Some prior studies have used the “book capital–asset ratio” to examine the effect of capitalization on lending (Bernanke & Lown, Citation1991; Gambacorta & Shin, Citation2018). Nevertheless, this framework fails to take into account the regulatory minima. The minimum regulatory capital depends on the total risk weighted asset that in turn depends on the amount of loans granted. Hence, given imperfections in the market for equities, a link between bank capital surplus/shortfall and lending could be expected.
Consequently, the previous studies also used a different mechanism—the regulatory-based capital surplus/shortfallFootnote1—to study how bank capital can affect lending behavior (Covas, Citation2016; Gambacorta & Mistrulli, Citation2004). These papers have explicitly taken into account regulatory capital requirements. Empirical evidence confirms that “banks with higher amounts of capital relative to regulatory requirements are likely to lend more, but an increase in capital requirements will cause banks to lend less” (Covas, Citation2016, p. 4). Covas (Citation2016) argues that the positive association of bank equity-to-total asset ratio with loan growth in the former approach hinges on the positive relationship between lending and capital surplus/shortfall. Nevertheless, these papers also failed to consider the financial institution’s desired target capital that takes into account market constraints apart from the regulatory minima, if any.
The second framework (i.e. the regulatory-based capital surplus/shortfall) implicitly assumes that the target capital of MFIs is the regulatory minima and its proponents argue that it is the capital adequacy ratio relative to the minimum capital requirement that is relevant to predict credit growth. However, in addition to regulatory pressure, other external pressures from market participants including the expectations of institutional and retail depositors and creditors also determine the target capital of MFIs. The managers of different MFIs also have different risk preferences. MFI managers with higher risk aversion may keep higher capitalization to absorb any loss while managers with lower risk aversion may aim for a lower capitalization and increase leverage (debt fundings) to expand credits more. Hence, MFI’s target capital is likely to be different from the regulatory minima. This study hypothesizes that each MFI targets an implicit level of capital ratio resulting from market discipline and bounded below by regulatory requirements, if any, and at each period, MFIs try to adjust as much as they can towards this target. Consequently, empirical research is needed to examine the effect of capitalization on lending behavior through the divergence between the actual capital ratio and the implicit long-run target capital ratio (Berrospide & Edge, Citation2010; Thibaut & Mathias, Citation2014).
The aim of this study is, therefore, two-fold: First, the study identifies the drivers of the capitalization of MFIs in Sub-Saharan Africa (SSA). In SSA, the number of banked households is the lowest (CGAP, Citation2018) and the share of poor people is the highest in the world (Beegle & Christiaensen, Citation2019). Consequently, MFIs are crucial to enhance credit access and other financial services to poor people in Africa. Moreover, the microfinance industry in SSA is unprofitable (Tehulu, Citation2021a). It is also difficult to raise capital in SSA (Hearn et al., Citation2018). Given that regulatory authorities and market participants require MFIs to keep adequate capitalization as it determines MFI solvency, it is imperative that we study the capital adjustment process in the microfinance sector in this region as the level of capitalization among MFIs also varies significantly (Basel Committee on Banking Supervision (BCBS), Citation2010; Tehulu, Citation2021a).
While empirical studies on drivers of MFI capitalization are scant, the existing studiesFootnote2 were not focused on MFIs in SSA (see, Goddard et al., Citation2015; Soumaré et al., Citation2020; Tchakoute Tchuigoua, Citation2016). Goddard et al. (Citation2015) investigated the determinants of US credit union capital-to-assets ratios. Due to the diverse charter type of MFIs where MFIs are organized as banks, non-bank financial institutions, Credit Unions/Cooperatives, non-governmental organizations (NGOs) or Rural Banks, the applicability of their study to MFIs in general is limited since it focuses solely on credit unions, while it is not also confined to MFIs in SSA. Similarly, Tchakoute Tchuigoua (Citation2016) has examined the determinants of buffer capital in microfinance institutions. Since the microfinance industry is of diverse nature—comprising both regulated and unregulated MFIs, the risk-based capital adequacy ratio and the minimum capital requirements (and hence, the capital bufferFootnote3) could be less relevant to studies on MFIs. Soumaré et al. (Citation2020) also examine the capital adjustment process in MFIs using a global dataset. Nevertheless, whether empirical evidences at a global level also hold true at a regional level is also an empirical question as the relationship between capitalization and its determinants might be asymmetric or divergent depending on the region where the MFIs are located; this is also confirmed by our empirical evidences.
Second, beyond examining the capital adjustment process, the article also builds on Berrospide and Edge (Citation2010) and Thibaut and Mathias (Citation2014) and examines whether capitalization influences credit growth through the deviation (i.e. divergence between the actual capital ratio and the long-run target capital). Several studies have examined the drivers of the credit growth of MFIs. However, these prior studies either they do not incorporate capitalization in their econometric model (eg. Wagner & Winkler, Citation2013), or employ the risk-based capital buffer/book capital ratio (eg. Hessou & Lai, Citation2018; Tchakoute Tchuigoua et al., Citation2020; Tehulu, Citation2021b). This study is the first to examine MFI capitalization and credit growth nexus through the deviation as this approach considers MFI’s desired target capital that takes into account market constraints apart from the regulatory minima, if any.
The remaining parts of this article are structured as follows: In Section 2, we provide our review of the related literature and the research hypotheses. In Section 3, we describe the data and methodology including our model specifications for the capital adjustment and lending equation as well as the estimation technique we use to estimate the parameters. In Section 4, we present and discuss the results. Finally, Section 5 provides the conclusions.
2. Literature review and hypotheses
2.1. The capital adjustment process
The capitalization of financial institutions is the result of either a hierarchizing of debt, internal financing, and equity issuing or a trade-off between debt and equity (Tchakoute Tchuigoua, Citation2015). The choice of a particular capital structure is one of the main strategic decision areas of financial and non-financial business organizations. Firms do not appear to choose their capital structure in a random manner (Ross et al., Citation2003). Accordingly, several studies have examined the determinants of capital structure of non-financial firms (Cook & Tang, Citation2010; Flannery & Rangan, Citation2006; Lemmon et al., Citation2008; Oztekin & Flannery, Citation2012) and capital structure/capital adjustment of banks (Berger et al., Citation2008; Berrospide & Edge, Citation2010; Shim, Citation2013; Thibaut & Mathias, Citation2014). However, studies on capital adjustment from the microfinance industry are scant. This study, therefore, heavily borrows from the literature on capital adjustment decisions from the banking industry.
The literature shows that portfolio at risk is one of the drivers of capitalization. The riskiness of the loans held by MFIs could influence the target level of capital as MFIs with poor portfolio quality could avoid the risk of failure by increasing their capitalization (Tchakoute Tchuigoua, Citation2016). Similarly, Tchakoute Tchuigoua (Citation2015) states that MFIs with a lower portfolio risk appear to have easier access to debt financing. This could lead to a positive association of portfolio risk with capitalization. On the other hand, since credit risk has a significant negative effect on profitability (Muriu, Citation2011), MFIs with higher portfolio risk might have lower capital. Moreover, MFI managers who have lower risk aversion may choose riskier credit portfolio and higher leverage which could lead to a negative association of portfolio risk with capitalization. Therefore, our a priori expectation as to the relationship between capitalization and portfolio risk is inconclusive.
The profitability of MFIs is another variable that could predict capitalization. Since it is difficult to raise new equity capital in SSA (Hearn et al., Citation2018) and MFIs do not generally make dividend payments, profitable MFIs could rely on internal financing to external financing to meet their funding needs. Higher earnings also reduce the need to raise debt (Degryse et al., Citation2012). Hence, financial institutions with higher profitability are likely to have higher capitalization (Brendea & Pop, Citation2019; Shim, Citation2013). We can, thus, expect profitability to be positively associated with the capitalization of MFIs.
The scale of MFIs (large scale Vs small scale MFIs) could also affect the level of MFI capitalization. Berger et al. (Citation2008) state that large financial institutions could be more diversified, be more experienced in risk management, and benefit more from government guarantees (too big to fail hypothesis) and hence, may have lower capitalization. Brendea and Pop (Citation2019) also confirm that there is a positive relationship of size with the debt raio (negative association with the capital raio) of firms. Consequently, we expect a negative relationship between the capitalization and size of MFIs.
The target capital ratio of MFIs could also depend on the liquidity of MFI assets. Firms with lower liquid assets may reduce the probability of costly default by increasing their capitalization, that is, by lowering their leverage (Sibilkov, Citation2009). Sibilkov states that the costs of illiquidity are economically substantial as compared to the benefits of leverage, and managers adjust leverage to control these costs. Moreover, since liquid assets have lower risk weights, financial institutions with more asset liquidity may have lower capital (Tehulu, Citation2021a). Therefore, we expect MFIs’ liquidity to be negatively associated with their capitalization.
The availability of deposits could also influence the capitalization of MFIs. As MFIs rely more on deposits rather than equity capital to fund their loans, the total assets will expand but MFI capitalization will decline since by construction capitalization is equity scaled by total assets. Therefore, the expected relationship between capitalization and deposit growth of MFIs is negative. The GDP growth rate, which captures the effect of the business cycle, is also one of the potential determinants of capitalization. The theoretical relationship between capitalization and GDP growth is unclear (Goddard et al., Citation2015). Goddard et al. explain this as follows: Forward-looking financial institutions might increase their capitalization during an upturn in order to be able to absorb losses and increase their resilience during a future downturn. On the other hand, myopic institutions might exploit lending opportunities to the full and deplete capital during an upturn. Hence, the expected relationship is undecided.
Finally, the charter type (legal status) of MFIs could also influence the capitalization of MFIs. NGO MFIs could be poorly leveraged because they are not allowed to mobilize public deposits in certain economies (Lafourcade et al., Citation2005) and rely heavily on donated equity. Therefore, we expect NGOs to have higher capitalization as compared to NBFIs. Similarly, NBFIs may have higher capitalization compared to Micro-banks and Credit unions/cooperatives since they are financed by public equity from local governments and development associations. On the other hand, Rural banks have higher deposit mobilization than MFIs with other charter types (Tehulu, Citation2021a). Consequently, we expect Rural banks, Micro-banks and Credit unions/cooperatives to have lower capitalization relative to NBFIs.
Accordingly, the hypotheses of our study are as follows:
Hypothesis (H1): Portfolio risk has a significant influence on the capitalization of microfinance institutions.
Hypothesis (H2): Profitability has a positive association with the capitalization of microfinance institutions.
Hypothesis (H3): The size of microfinance institutions is negatively related with their capitalization.
Hypothesis (H4): The liquidity of microfinance institutions has a negative effect on their capitalization.
Hypothesis (H5): Deposit growth influences the capitalization of microfinance institutions negatively.
Hypothesis (H6): Economic growth has a significant relationship with the capitalization of microfinance institutions.
Hypothesis (H7): The legal status of microfinance institutions influences their capitalization (NGO microfinance institutions hold higher capitalization as compared to NBFIs while Rural banks, Micro-banks and Credit unions/cooperatives keep lower capitalization relative to NBFIs).
2.2. Capital adjustment process and lending behavior
The question of whether and how capitalization impacts lending behavior has been widely debated since the 1988 Basel capital accord (Bernanke & Lown, Citation1991; Berrospide & Edge, Citation2010; Carlson et al., Citation2013; Covas, Citation2016; Gambacorta & Shin, Citation2018). The prior research establishes that capitalization is one of the determinants of the lending behavior of financial institutions; this is in line with the capital crunch hypothesis. The capital crunch hypothesis implies that banks/MFIs with higher capitalization expand credits more while those with lower capitalization limit their loan supply to fulfill capital requirement since there is capital adjustment difficulty in financial institutions; this shows that capitalization could be positively associated with the credit growth of MFIs.
Alternatively, the literature shows that the link between credit supply and capitalization might depend on the relationship between risk aversion and capitalization; this is in line with the literature that shows the level of capitalization is associated with risk aversion and (Michelangeli & Sette, Citation2016). While Cucinelli (Citation2016) asserts that financial institutions with higher capitalization are more risk averse and limit lending, Michelangeli and Sette (Citation2016) show that higher capitalization is related with a higher likelihood of application acceptance, financial institutions with lower capitalization reject applications by riskier borrowers. Hence, the link between credit supply and capitalization could also depend on the relationship between risk aversion and MFI capitalization.
The literature additionally reveals that the prior studies have applied different frameworks in modeling the channel going from capitalization to loan supply. While a strand of empirical research uses the capital-to-asset ratio directly (Bernanke & Lown, Citation1991; Berrospide & Edge, Citation2010; Carlson et al., Citation2013; Gambacorta & Shin, Citation2018), another strand of research employed the capital adequacy ratio/regulatory-based capital surplus/shortfall (Carlson et al., Citation2013; Covas, Citation2016; Gambacorta & Mistrulli, Citation2004; Karmakar & Mok, Citation2013). Nevertheless, studies that build on the Berrospide and Edge (Citation2010) and Thibaut and Mathias (Citation2014) framework and examine whether capital impacts lending through the divergence between the actual level of capital and the desired level of capital are missing, especially, from the microfinance industry. Consequently, this article also examines whether capitalization influences the credit growth of MFIs through the divergence between the actual capital ratio and the long run target capital.
While the framework based on book capital ratio is criticized for it does not take into account the regulatory minimum capital (Covas, Citation2016), the regulatory-based capital surplus/shortfall also has the limitation of not considering the financial institution’s own target capital (Thibaut & Mathias, Citation2014) which takes into account market constraints apart from the regulatory minima, if any. Moreover, since the microfinance industry is of diverse nature—comprising both regulated and unregulated MFIs, capital requirements could be less relevant to studies on MFIs. Using the deviation from internal targets also offers the advantage of mitigating potential endogeneity issues because most of the endogenous relations are captured in the target component (Thibaut & Mathias, Citation2014).
As a result, in line with the literature,Footnote4 we assume that each MFI targets an implicit level of capital ratio resulting from market discipline and bounded below by regulatory requirements, if any, and at each period, MFIs try to adjust as much as they can towards this target. Hence, the actual capital ratio relative to the long-run target capital (the deviation) is expected to influence the credit growth of MFIs. Accordingly, the article builds on Berrospide and Edge (Citation2010) and Thibaut and Mathias (Citation2014) and tests whether the level of actual capital at time t-1 relative to MFI’s own target capital at time t matters in the lending behavior of MFIs. Given that raising equity capital might be difficult in MFIs in SSA, MFIs with poor capitalization could limit their lending in order to meet regulatory capital requirements or market constraints. Similarly, MFIs with higher capitalization could be less risk averse as they can absorb more loan losses (Michelangeli & Sette, Citation2016). Hence, we expect capital surplus/shortfall to be positively associated with credit growth.
Accordingly, this study tests the following hypothesis as well:
Hypothesis (H8): The capital surplus/shortfall (as measured by the divergence between the actual capital ratio and the long-run target capital ratio) has a positive relationship with the credit growth of microfinance institutions.
3. Data and methodology
3.1. The data
This study employs panel dataset of 127 MFIs across 31 countriesFootnote5 in Sub-Saharan Africa covering 2004–2014. Following previous studies on microfinance institutions (Chikalipah, Citation2019; Muriu, Citation2011; Tchakoute Tchuigoua, Citation2016), MFI-specific data to be used in this study is obtained from the MIX database. This dataset had been available online at www.mixmarket.org. The country-level macroeconomic indicators are publicly available from the World Bank database at http://data.worldbank.org/data-catalog/world-development-indicators.
3.2. Model specifications
Following the work of Berrospide and Edge (Citation2010) and Thibaut and Mathias (Citation2014), the paper implements a stepwise strategy. In a first step, the paper investigates the capital adjustment process in a partial adjustment framework that is rationalized by a cost–benefit analysis. Given adjustment cost, MFIs try to converge towards an implicit target while maximizing their profit. This paper, thus, identifies the determinants of MFI capitalization and its implicit target capital as well as the adjustment speed by using (i) a set of observables from MFI balance sheets and income statements, (ii) macro-economic factor, and (iii) a set of MFI-specific time-invariant components. In a second step, we compute for each MFI and for each period what is called the deviation from the estimated optimal target, i.e. the difference between the actual capital ratio and target (predicted) capital ratio. Finally, we examine whether capitalization impacts credit growth of MFIs through the deviation. Accordingly, the next sections describe our model specifications for the capital and lending behavior equations.
The Capital Adjustment Equation
Building on the prior empirical studies (Berrospide & Edge, Citation2010; Thibaut & Mathias, Citation2014), we specify the long-run target capital ratio as follows:
Therefore, when we expand EquationEq.1(1)
(1) to incorporate all the potential determinants indicated in our hypotheses in Section 2.1, the model for the implicit long-run target capital ratio will be as follows:
Where denotes capitalization, βk (K = 1, 2, 3 … ., 7) are coefficients for the respective predictor variables,
represent coefficients of different legal status dummies (LES) namely micro-banks, rural banks, credit unions/cooperatives and NGOs where the benchmark charter type is NBFI and
is the constant. Other designations are as described in Table . Table also provides descriptions and measurements of the variables.
Table 1. Description of variables and their measurements
Since instantaneous capital adjustment towards the target may not be possible due to adjustment costs, the actual capital ratio of MFIs is assumed to follow a partial-adjustment process of the form (Short-run Model):
Where the parameter is a measure of persistence in MFI capitalization; the speed of adjustment will be (1 −
);
represents the parameters of the respective vectors;
is the coefficient for the time-invariant components (i.e. legal status dummies);
is the constant and includes the individual-fixed effects as well and
denotes the error term.
EquationEquation 3(3)
(3) is the one we estimate. With the set of estimated parameters of EquationEquation 3
(3)
(3) , we can recover the long-run target capital ratio in Equationequation 2
(2)
(2) by first deriving the long-run parameters
,
, and
. Accordingly, we calculate the long-run effect of each firm-specific explanatory variables and the macroeconomic variable as follows:
The long-run effect of the time-invariant components (i.e. legal status dummies) is computed as
The constant including the unobserved individual-fixed effects is calculated as follows:
Once we estimate the implicit target (), the deviation (i.e. capital surplus/shortfall) is calculated as follows:
If the deviation is positive, it shows capital surplus (i.e. the MFI is overcapitalized) and negative values imply capital shortfall (i.e. the MFI is undercapitalized).
The Credit Growth Model Specification
Once we estimate the deviation using EquationEq.7(7)
(7) , we examine the impact of capital surplus/shortfall on credit growth of MFIs using the following lending behavior model that is adopted from Tehulu (Citation2021b):
While Tehulu (Citation2021b) uses the book capital ratio as a measure of capitalization, we use the deviation (DEV) instead. In line with Tehulu (Citation2021b), we include the lag-dependent variable () because we use dynamic panel models to estimate the lending behavior model. Consistent with the prior empirical studies, we also include liquidity (Cucinelli, Citation2016; Hessou & Lai, Citation2018), portfolio risk (Karmakar & Mok, Citation2013), profitability (Hessou & Lai, Citation2018; Laidroo, Citation2012), and size (Laidroo, Citation2012; Thibaut & Mathias, Citation2014) to control for MFI-specific factors (other than capitalization) that could impact the credit growth of MFIs. We add scale dummies (large scale—
and small scale—
) to the set of covariates to control for scale effects since lower gross loan portfolio (GLP) at time t-1 could amplify the credit growth at time t while higher GLP reduces the credit growth of MFIs (Laidroo, Citation2012; Tehulu, Citation2021b) as the calculation of credit growth uses the lag GLP as the base value.
In line with previous researches that document demand-side determinants are also important factors to predict loan growth, we include GDP growth (Berrospide & Edge, Citation2010; Igan & Pinheiro, Citation2011; Laidroo, Citation2012), inflation (Laidroo, Citation2012; Thibaut & Mathias, Citation2014), employment (Bernanke & Lown, Citation1991), and catch-up effect as measured by GDP per capita (Igan & Pinheiro, Citation2011) to control for the demand factors. Finally, we include global financial crisis indicators ( and
) to control for time-fixed effects.
Following Tehulu (Citation2021b) we measure lending behavior (LB) as the growth rate of Gross Loan Portfolio (GLP) where GLP is all outstanding principals including current, delinquent, and renegotiated loans, but not loans that have been written off. Liquidity (LIQ) is calculated as Total cash and cash equivalents scaled by total assets. Size (LNTA) is the size of the microfinance institution measured in terms of the natural logarithm of total assets. Inflation (INF) as measured by the consumer price index reflects the annual percentage change in the cost to the average consumer of acquiring a basket of goods and services. Employment ratio (EMP) is the proportion of a country’s population that is employed. Catch-up Phenomenon (CUP) indicates the level of institutional and economic development of a country and it is measured by the natural logarithm of GDP per capita (current US$). Others as described in Table .
3.3. Estimation methodology
Given our dynamic panel model for the capital equation (EquationEq.3(3)
(3) ), there are some important concerns on what estimation methodology is appropriate since the model contains the lagged dependent variable. Bond (Citation2002) states that the Ordinary Least Squares (OLS) estimator is inconsistent (specifically, at least in large samples, biased upwards), since the lagged dependent variable is positively correlated with the error term (
) due to individual effects (
.). The Fixed Effects estimator eliminates this source of inconsistency in OLS estimator by transforming the equation to eliminate
. Specifically, the mean values of each variable (the dependent variable, the lagged dependent variable, explanatory variables,
and
) are obtained, the original observations are expressed as deviations from these respective means, and then, the OLS estimator is used to estimate these transformed equations; this removes the individual-fixed effects from the transformed equations since the mean of the individual effects
is itself
.
However, in panels with small time periods, the Fixed Effects estimator also induces a “non-negligible correlation between the transformed lagged dependent variable and the transformed error term, so that the Within Groups estimator is also inconsistent here” (Bond, Citation2002, p. 144). The solution is to estimate EquationEquation 3(3)
(3) (EquationEq.3)
(3)
(3) with Generalized Method of Moments (GMM). Since the GMM permits examining the long-run determinants of capitalization, it also allows predicting the implicit target capital. Following the recent literature (Berger et al., Citation2008; Tchakoute Tchuigoua, Citation2016; Thibaut & Mathias, Citation2014), we prefer the GMM estimation method. The Arellano–Bover/Blundell–Bond estimator is more efficient than the Arellano–Bond estimator and thus, allows incorporating more potential determinants. Efficiency is an important concern in studies on the microfinance sector due to large number of missing values. Besides efficiency issues, the Arellano–Bond estimator does not also allow us to estimate the effects of time-invariant components as the differencing removes any time invariant explanatory variables.
Hence, the system-GMM estimator is preferred to the differenced-GMM estimator. Specifically, we use the two-step GMM estimator with Windmeijer bias-corrected standard errors since standard errors estimates of the two-step estimators are severely downward biased (Roodman, Citation2007). After estimating the short-run coefficients by applying the stated estimation technique, we employ EquationEq.4(4)
(4) , EquationEq.5
(5)
(5) , or EquationEq.6
(6)
(6) to recover the long-run coefficients and determine the long-run target capital using EquationEquation 2
(2)
(2) (EquationEq.2)
(2)
(2) . Standard errors for the long-run effects in the capital equation are approximated with the Delta method. For the same reason stated above, the lending equation is also estimated by the Arellano–Bover/Blundell-Bond two-step Generalized Method of Moments (GMM) Windmeijer bias-corrected standard errors.
4. Empirical results and discussions
4.1. The capital adjustment process
4.1.1. Descriptive statistics
The descriptive statistics results (Table ) show that MFIs in SSA have a high capitalization, which is on average 34%; this figure significantly exceeds the minimum regulatory capital requirement of 12% or so that applies to regulated MFIs. The standard deviation, minimum and maximum values reveal that the level of capitalization varies significantly from MFI to MFI. The mean value, standard deviation, minimum, and maximum values for portfolio risk show that risk taking behavior differs among MFIs. While some MFIs have high portfolio quality, other MFIs exhibit high loan default. Regarding profitability, MFIs in SSA have on average a return on assets (ROA) of around negative 1% suggesting that the microfinance sector in SSA is unprofitable. However, while some MFIs are suffering from severe losses (ROA of −85%), some other MFIs are highly profitable and have a ROA as high as 36%. The liquidity of MFIs measured by deposit-to-loan ratio has a mean value of 76%, a standard deviation of 83% and minimum and maximum values of 0 and 12 which implies that the microfinance sector in SSA comprises MFIs with no financial intermediation (non-deposit taking), low financial intermediation, and high financial intermediation.
Table 2. Descriptive statistics
Furthermore, the descriptive statistics results show that the microfinance industry in SSA has rapid deposit growth with an average growth rate of about 52%. From the descriptive statistics, we can observe that the outcome variable (capitalization) and the potential predictor variables (including portfolio risk, profitability, liquidity, deposit growth and size, among others) exhibit significant variability. Accordingly, in the following section, we discuss whether the observed differences in the potential predictor variables explain the variation in capitalization among MFIs in SSA.
4.1.2. Econometric results
The GMM results on the short-run determinants of MFI capitalization are summarized in Table . The findings show that the capitalization of MFIs is moderately persistent. Taking the more accurate model (i.e. Model 2), the coefficient for the lagged capital ratio is 0.4376 implying that when there is a 1% shock to capital in the current period, there will be a 43.76% reduction in the capital ratio in the following period. In other words, the speed of adjustment to equilibrium is only 56.24%, which shows the existence of capital adjustment difficulty in MFIs. This shows capitalization has the potential to predict the lending behavior of MFIs as MFIs with lower capitalization increase credits less to meet capital requirements or market constraints since it is difficult to raise additional equity capital to support more credit expansions; if MFIs can adjust their capitalization instantaneously, then it will be the lending behavior that predicts capitalization since MFIs can adjust their capital to achieve the desired credit growth.
Table 3. Drivers of MFI capitalization (short run relationships)
The results also revealed that portfolio risk has a significant negative effect on capitalization at 10 percent level of significance. This could be in part due to risk preferences of MFI managers. MFI managers who have lower risk aversion might choose riskier credit portfolio and higher leverage while those managers with higher risk aversion might focus on credit worthy borrowers and keep higher capital as a buffer against actual, expected and unforeseen losses. Hence, such MFI manager risk preferences could lead to a negative relationship between portfolio risk and capitalization. This finding suggests that MFIs with higher risk need to devise and follow appropriate risk management policies to limit risk levels and build capital buffer, and thereby increasing their resilience. The result is consistent with the findings of Goddard et al. (Citation2015) who found that a rise in non-performing loans ratio leads to a decrease in US credit union capital-to-asset ratio. However, our finding contradicts with the findings of Tchakoute Tchuigoua (Citation2016) and Soumaré et al. (Citation2020) that documented MFI capital is linked to loan portfolio quality negatively suggesting that risk preferences of MFI managers depend on the location of the MFIs.
We also found that profitability significantly contributes to the capitalization of MFIs consistent with our hypothesis. Since it is difficult to obtain external equity capital in SSA (Hearn et al., Citation2018) and MFIs do not generally make dividend payments, profitable MFIs could rely on internal financing to external financing to meet their funding needs. This finding is consistent with the literature that documents profitability is positively related with the capital ratio of firms (Brendea & Pop, Citation2019; Tchakoute Tchuigoua, Citation2016). Our empirical results suggest that capital adjustment difficulty may pose serious concerns and capital constraint could be a major obstacle to the performance of MFIs since the microfinance industry in SSA is unprofitable (Tehulu, Citation2021a). Consequently, MFIs need to devise and implement strategies that could improve their profitability. In light of previous research that used an international sample of MFIs and revealed that the effect of profitability on MFI capital is positive but insignificant (Soumaré et al., Citation2020), our findings establish the relative vital role of financial sustainability (profitability) in the capital adjustment process of MFIs in SSA.
The liquidity (as measured by the deposits-to-loans ratio) of MFIs is negatively and significantly associated with capitalization, confirming our a priori expectation. The result is statistically significant at 99% confidence interval. The finding supports Tehulu (Citation2021a) who found that Rural banks have higher liquidity but lower capitalization. This is in line with theoretical expectations that financial institutions with more asset liquidity hold lower capital since the required minimum risk-based regulatory capital is lower in these institutions as liquid assets have lower risk weights. It may also imply that MFIs with lower liquid assets reduce the probability of costly default by increasing their capitalization (Sibilkov, Citation2009). The result suggests that the expected costs of illiquidity are economically considerable as compared to the benefits of leverage and MFIs with lower liquidity control these costs by reducing leverage.
The findings also show that large-scale MFIs have lower capitalization, while small-scale MFIs have higher capitalization implying that the capitalization of MFIs is negatively related to their size, as expected. These findings are consistent with the “too big to fail” (TBTF) hypothesis. TBTF hypothesis implies that since large financial institutions are so important to the economy and that their failure could cause a drastic domino effect for the entire economy, there could be government intervention when they are in financial trouble and hence, large financial institutions may engage in moral hazard behavior due to those protective policies (Mattana et al., Citation2015). The negative relationship between capitalization and size of MFIs could also be due to stronger risk management culture in large MFIs than small MFIs (Hessou & Lai, Citation2018). MFIs with higher loan defaults or risky portfolios are required to maintain a higher capital buffer to absorb the actual and expected loan losses. Large firms can also access debt financing more easily than small firms (Brendea & Pop, Citation2019). Our finding is in line with Tchakoute Tchuigoua (Citation2016) who uncovered that the size of MFIs is negatively related to their capital adequacy ratio and buffer capital.
The sign of deposit growth is also in line with theoretical expectations. As MFIs mobilize more savings and rely more on deposits for funding the loans, total assets will increase and this in turn reduces the capital ratio, other things ceteris paribus. However, the result is not statistically significant. This could be due to the fact that the deposit mobilization (as measured by deposit-to-asset ratio) of MFIs in SSA is more or less constant across time despite the rapid growth of MFI’s deposits (Tehulu, Citation2021a) and this could be the rationale for the statistically insignificant result. The findings also revealed that business cycle has a negative but insignificant effect on the capitalization of MFIs. This result supports Soumaré et al. (Citation2020) that, using a global dataset, revealed MFI capital is negatively related to the business cycle. The insignificant coefficient in our case implies that fluctuation in the economy has little effect on the capitalization of MFIs in SSA and hence, MFI capital in SSA is relatively resilient to macroeconomic shocks.
Although Tehulu (Citation2021a), using One-Way ANOVA and the multiple linear regression, showed that capitalization significantly differs across legal status, the GMM results here do not support such evidence when other variables are included in the model; we found that legal status has no significant effect on MFI capitalization. This implies that the other variables included in the model have absorbed the predictive power of legal status. In other words, capitalization differences across legal status seem to be due to the included economic fundamentals and not due to any other factors. The results do not support our hypothesis that states “The legal status of microfinance institutions influences their capitalization”.
Finally, we uncovered that the constant is statistically significant and has the highest economic significance. This is consistent with the literature that assert capital ratio fluctuates mainly around a firm-specific unobserved time-invariant component (Berrospide & Edge, Citation2010; Flannery & Rangan, Citation2006; Thibaut & Mathias, Citation2014). Our findings are free from omitted variables bias as the Sargan test results in Table have confirmed that the instruments are uncorrelated with the residuals. The results also show that there is no autocorrelation in the residuals as revealed by Arellano-––Bond test for zero second-order autocorrelation in first-differenced errors, AR (2).
4.2. Capital adjustment process and MFIs’ credit growth
In order to examine the effect of capitalization on MFI lending behavior through the divergence between the actual capital ratio at time t-1 and the long-run target capital at time t, we first derived the long-run coefficients from the short-run coefficients in Table using the approach described in Section 3.2. The standard errors are approximated by the Delta method. Next, we estimated the target capital using the long-run coefficients. Then, we calculated the capital surplus/shortfall as the difference between the actual capital ratio at time t-1 and the desired capital at time t. Finally, we tested whether the capital surplus/shortfall (i.e. the deviation) predicts the lending behavior of MFIs.
The findings revealed the same kinds of explanatory variables to be the long-run determinants of capitalization as the short-run determinants. We found that portfolio risk, profitability, liquidity, large-scale indicator, and small-scale indicator are the important factors to explain capitalization in the long run (Table ) consistent with the short-run findings (Table ). Table also shows that the constant is statistically significant. Now the coefficients are greater than their respective short-run coefficients due to the existence of persistency in MFI capitalization. Moreover, the descriptive statistics show that the mean values of credit growth and deviation are 42% and 4%, respectively, suggesting that MFIs in SSA have rapid credit growth and are on average overcapitalized. The standard deviation, maximum and minimum values for credit growth are 0.81, 10.61, and −0.95, respectively, while that for deviation are 0.21, 0.95, and −0.93, respectively. These suggest that there is significant variance in credit growth and deviation among MFIs in SSA. In the following paragraphs, we discuss whether the variation in the deviation significantly explains the variation in credit growth of MFIs in SSA.
Table 4. Drivers of MFI capitalization (long run relationships)
The results on the capital surplus/shortfall (deviation) and credit growth nexus are summarized in Table . The results fail to support the prior empirical studies (Berrospide & Edge, Citation2010; Thibaut & Mathias, Citation2014) that show capitalization could impact the lending behavior of financial institutions through the deviation (i.e. the divergence between the actual capital ratio and the long-run target capital). In this regard, Berrospide and Edge (Citation2010) have revealed that there is a positive and significant relationship between bank loan growth and excess capital, although the economic significance is small. They showed that, in the long-run, if capital exceeds (falls short of) its target level by 1%, there could be roughly a 0.25 percentage point increase (reduction) of annualized loan growth. Similarly, Thibaut and Mathias (Citation2014) also found an economically and statistically significant association of aggregate capitalization index at year t − 1 with the change in aggregate lending at year t relative to year t − 1. However, our findings show that, in the microfinance industry, capitalization does not impact lending behavior through the divergence between the actual capital ratio at time t-1 and the target capital at time t (Table : Model 1). In other words, our findings have failed to confirm the positive association of capitalization with the credit growth of MFIs through the deviation.
Table 5. Capitalization and credit growth: GMM results
Tehulu (Citation2021b) documented that capitalization (as measured by the book capital ratio) positively and significantly influences the credit growth of MFIs in SSA. Hessou and Lai (Citation2018) also revealed a positive association of credit union capitalization at a point in time and its ability to extend loan in the next period. Hence, we can conclude that MFI managers consider the current book capital ratio (and not the deviation) in determining the extent of credit growth in subsequent year. It also implies that the long-run target capital is not as such binding and MFIs might violate strict adherence to their target capital in order to achieve the desired credit growth. The book capital ratio has superior predictive power over the deviation possibly because the book capital ratio is easily observable and hence, regulatory authorities might increase their monitoring of MFIs with relatively low capital-to-asset ratio. Hence, MFIs may not prefer holding relatively low capital ratio and possibly limit lending to adjust their capitalization upwards perhaps towards the industry median/mean capital ratio in order to circumvent increased supervision by regulatory authorities.
In regard to the above, unregulated MFIs are expected to behave like the regulated ones in response to capital regulation due to market discipline as the former will otherwise be unfavorably rated by the market participants and be at a disadvantage when their capitalization is low and deviates from the regulated ones. Generally, any MFI (regulated or unregulated) with lower capital ratio might be unfavorable rated by market participants such as institutional and retail depositors or creditors and this market discipline may also pressure such MFIs to limit their credit growth in order to adjust their capital upwards. These views are in line with the herding behavior theory.
The herding behavior theory asserts that firms may adjust their capital structure towards the industry median/mean capital structure since the costs of deviating from the group/industry may be greater than its benefits. In this respect, using industry mean capital structure, Brendea and Pop (Citation2019) revealed that firms exhibit herding behavior; more specifically, firms follow the mean industry capital structure. Similarly, using industry median capital structure, Camara (Citation2017) found evidence of herding behavior in financing decision in Services industry. Accordingly, MFIs with lower book capital ratio could limit their credit supply to increase their capital to the industry median capital ratio while MFIs with higher capital ratio expand credits more since they have adequate capitalization to absorb any anticipated and actual loan losses. Hence, future research shall test whether capitalization influences credit growth through the divergence between the actual capital ratio and the industry median/mean capitalization.
Finally, as a robustness test, we also examine for any asymmetric effect of capitalization (deviation) on credit growth depending on whether the MFIs are over/under capitalized, regulated or non-regulated and with for-profit or non-profit status. Although the prior literature show that capitalization has asymmetric effect on credit growth (Carlson et al., Citation2013; Thibaut & Mathias, Citation2014), we fail to find evidence supporting an asymmetric effect of capitalization on MFI credit growth (Model 2 in Table ). It is argued that the effect of capitalization on loan growth for over-capitalized banks could be less marked since “banks cannot force agents to borrow while they can prevent them from getting funds: the extent of the increase in lending is possibly more sensitive to changes in the demand than the supply of credit” (Thibaut & Mathias, Citation2014, p. 16). In this respect, Thibaut and Mathias found that in countries/periods where banks are under-capitalized, the growth rate of aggregate lending tends to decrease significantly while such patterns do not exist during episodes of aggregate over-capitalizations.
Similarly, Carlson et al. (Citation2013) found that the elasticity of bank lending with respect to capital ratios is higher when capital ratios are relatively low. Nevertheless, in the microfinance industry, we do not find asymmetric effect of capitalization on credit growth across over-capitalized and under-capitalized MFIs. The results also show that capitalization (deviation) has no asymmetric effect on the credit growth of MFIs across the regulation status (Table : Model 3) and profit status (Table : Model 4) of MFIs. Therefore, in light of our findings and the findings of previous studies (Hessou & Lai, Citation2018; Tehulu, Citation2021b), we can infer that it is the book capital ratio (and not the deviation) that matters in the lending behavior of MFIs in SSA.
5. Conclusions
The purpose of this study is twofold: First, we examine the capital adjustment process using a partial adjustment framework and second, we test whether capitalization impacts the credit growth of microfinance institutions (MFIs) through the deviation (i.e. the divergence between the actual capital ratio and the implicit long-run target capital). In this respect, we use unbalanced panel dataset of 127 MFIs across 31 countries in Sub-Saharan Africa (SSA) during 2004–2014. We apply the Arellano–Bover/Blundell-Bond two-step Generalized Method of Moments (GMM) Windmeijer bias-corrected standard errors for estimating both the capital and lending models. Standard errors for the long-run effects in the capital equation are approximated with the Delta method.
Our findings revealed that profitability contributes to the capitalization of MFIs, whereas portfolio risk and liquidity are negatively associated with MFI capital. We also show that large-scale MFIs have lower capitalization, while small-scale MFIs have higher capitalization relative to medium scale MFIs consistent with the “too big to fail” hypothesis. Nevertheless, we uncover that the legal status of MFIs, deposit growth, and economic growth have no direct effects on capitalization. We also confirm that there is capital adjustment difficulty in the microfinance industry in SSA. The constant of the model is also statistically significant and has the highest economic significance suggesting that the capital ratio fluctuates mainly around a firm-specific unobserved time-invariant component. The findings, however, fail to support the hypothesis that capitalization impacts MFI lending behavior through the deviation (i.e. the divergence between the actual capital ratio and the implicit long-run target capital). Our study also fails to provide evidence for asymmetric effect of capitalization on credit growth across MFIs that are over/under capitalized, regulated, or non-regulated and with for-profit or non-profit status. The results are free from omitted variable bias as revealed by the Sargan test results.
The findings have the following practical and theoretical implications: Given profitability is positively and significantly associated with MFI capitalization, our findings suggest that capital adjustment difficulty may pose serious concerns and capital constraint could be a major obstacle to the performance of MFIs since the microfinance industry in SSA is unprofitable (Tehulu, Citation2021a). Consequently, MFIs need to devise and implement strategies that could improve their profitability. In addition, since portfolio risk is negatively associated with MFI capitalization, appropriate risk management policies and practices are also vital to build capital buffer and increase the resilience of the microfinance industry. The article also contributes to the literature in several ways. The study provides empirical evidence on what drives MFI capitalization, especially in SSA. In light of previous literature and our empirical findings, the study also shows that the relationships between capitalization and some of its determinants are asymmetric or divergent depending on the region the MFIs are located. Moreover, the paper also provides new evidence that capitalization does not predict MFI credit growth through the divergence between the actual capital ratio and the long-run target capital.
Finally, in light of our findings, we suggest that future studies examine the existence of herding behavior in the capital adjustment process of microfinance institutions as MFIs may adjust their capital ratio to the industry median/mean capital ratio given that the costs of deviating from the group/industry may be greater than its benefits. We also suggest that future research shall use larger dataset and investigate if the relationship between capitalization and its determinants is asymmetric depending on the size or legal status of MFIs. The use of GMM requires large number of observations so that research results do not suffer from bias associated with the instrument count. Future studies shall also test whether capitalization influences credit growth through the divergence between the actual capital ratio and the industry median/mean capitalization.
Disclosure statement
No potential conflict of interest was reported by the author(s).
Additional information
Funding
Notes on contributors
Tilahun Aemiro Tehulu
Tilahun Aemiro Tehulu (PhD) is currently an Associate Professor in the Department of Accounting and Finance, College of Business & Economics, Bahir Dar University, Ethiopia. His research interests include microfinance institutions, financial institutions and markets, finance, micro and small enterprises, corporate governance and taxation. He has published several articles in different scholarly journals such as the International Journal of Emerging Markets (Emerald) and Development in Practice (Taylor & Francis), among others. The author can be contacted at: [email protected]
Notes
1. The regulatory based capital surplus/shortfall (also called capital buffer) is the difference between the risk based capital adequacy ratio and the minimum capital requirement.
2. Two worth-mentioning studies are identified: While Goddard et al. (Citation2015) investigated the determinants of US credit union capital-to-assets ratios, before and after the implementation of the current capital adequacy regulatory framework in 2000, Tchakoute Tchuigoua (Citation2016) examined drivers of capital buffer in microfinance institutions based on 292 MFIs worldwide over the period 2004 to 2009.
3. Due to data unavailability on risk based capital adequacy ratio, Tchakoute Tchuigoua (Citation2016) has measured capital buffer as the difference between the book capital ratio and the minimum capital requirement. However, the use of the book capital ratio in place of the risk based capital adequacy ratio could also distort the true value of MFI capitalization.
4. See, Berrospide and Edge (Citation2010) and Thibaut and Mathias (Citation2014).
5. The lists of countries included in our analysis comprise Angola, Benin, Burkina Faso, Burundi, Cameroon, Central African Republic, Chad, Congo, Democratic Republic of the, Congo, Republic of the, Cote d’Ivoire (Ivory Coast), Ethiopia, Gambia, The, Ghana, Guinea, Kenya, Madagascar, Malawi, Mali, Mozambique, Namibia, Niger, Nigeria, Rwanda, Senegal, Sierra Leone, South Africa, Swaziland, Tanzania, Togo, Uganda and Zambia.
References
- Basel Committee on Banking Supervision (BCBS). (2010). Microfinance activities and the core principles for effective banking supervision. Bank for International Settlements.
- Beegle, K., & Christiaensen, L. (2019). Accelerating poverty reduction in Africa. World Bank.
- Berger, A. N., DeYoung, R., Flannery, M. J., Lee, D., & Oztekin, O. (2008). How do large banking organizations manage their capital ratios? Journal of Financial Services Research, 34(2–3), 123–19. https://doi.org/10.1007/s10693-008-0044-5
- Bernanke, B., & Lown, C. S. (1991). The credit crunch. Brookings Papers on Economic Activity, 22(2), 205–248. https://doi.org/10.2307/2534592
- Berrospide, J. M., & Edge, R. M. (2010). The effects of bank capital on lending: What do we know, and what does it mean? International Journal of Central Banking, 6(4), 5–54.
- Bond, S. R. (2002). Dynamic panel data models: A guide to micro data methods and practice. Portuguese Economic Journal, 1(2), 141–162. https://doi.org/10.1007/s10258-002-0009-9
- Brendea, G., & Pop, F. (2019). Herding behavior and financing decisions in Romania. Managerial Finance, 45(6), 716–725. https://doi.org/10.1108/MF-02-2018-0093
- Camara, O. (2017). Industry herd behaviour in financing decision making. Journal of Economics and Business, 94, 32–42. https://doi.org/10.1016/j.jeconbus.2017.08.001
- Carlson, M., Shan, H., & Warusawitharana, M. (2013). Capital ratios and bank lending: A matched bank approach. Journal of Financial Intermediation, 22(4), 663–687. https://doi.org/10.1016/j.jfi.2013.06.003
- CGAP. (2018). Empowering the poor through financial services.
- Chikalipah, S. (2019). Optimal sources of financing for microfinance institutions in Sub-Saharan Africa. Development in Practice, 29(3), 395–405. https://doi.org/10.1080/09614524.2018.1519011
- Cook, D. O., & Tang, T. (2010). Macroeconomic conditions and capital structure adjustment speed. Journal of Corporate Finance, 16(1), 73–87. https://doi.org/10.1016/j.jcorpfin.2009.02.003
- Covas, F. (2016). Bank capitalization and loan growth. The Clearing House.
- Cucinelli, D. (2016). Can speed kill?: The cyclical effect of rapid credit growth: Evidence from bank lending behavior in Italy. The Journal of Risk Finance, 17(5), 562–584. https://doi.org/10.1108/JRF-03-2016-0035
- Degryse, H., De Goeij, P., & Kappert, P. (2012). The impact of firm and industry characteristics on small firms’ capital structure. Small Business Economics, 38(4), 431–447. https://doi.org/10.1007/s11187-010-9281-8
- Flannery, M. J., & Rangan, K. P. (2006). Partial adjustment toward target capital structures. Journal of Financial Economics, 79(3), 469–506. https://doi.org/10.1016/j.jfineco.2005.03.004
- Gambacorta, L., & Mistrulli, P. E. (2004). Does bank capital affect lending behavior? Journal of Financial Intermediation, 13(4), 436–457. https://doi.org/10.1016/j.jfi.2004.06.001
- Gambacorta, L., & Shin, H. S. (2018). Why bank capital matters for monetary policy. Journal of Financial Intermediation, 35, 17–29. https://doi.org/10.1016/j.jfi.2016.09.005
- Goddard, J., McKillop, D., & Wilson, J. O. (2015). Regulatory change and capital adjustment of US credit unions. Journal of Financial Services Research, 50(1), 29–55. https://doi.org/10.1007/s10693-015-0217-y
- Hearn, B., Oxelheim, L., & Randoy, T. (2018). The institutional determinants of private equity involvement in business groups–The case of Africa. Journal of World Business, 53(2), 118–133. https://doi.org/10.1016/j.jwb.2016.02.002
- Hessou, H., & Lai, V. S. (2018). Basel III capital buffers and Canadian credit unions lending: Impact of the credit cycle and the business cycle. International Review of Financial Analysis, 57, 23–39. https://doi.org/10.1016/j.irfa.2018.01.009
- Igan, D., & Pinheiro, M. (2011). Credit growth and bank soundness: Fast and furious? (International Monetary Fund, WP/11/278).
- Karmakar, S., & Mok, J. (2013). Bank capital and lending: An analysis of commercial banks in the United States (Working Papers 18). Banco de Portugal, Economics and Research Department.
- Lafourcade, A. L., Isern, J., Mwangi, P., & Brown, M. (2005). Overview of the outreach and financial performance of microfinance institutions in Africa. MIX.
- Laidroo, L. (2012). Lending growth determinants and cyclicality: Evidence from CEE banks. 4th International Conference “Economic Challenges in Enlarged Europe” Conference Proceedings. Tallinn University of Technology, Tallinn, Estonia.
- Lemmon, M. L., Roberts, M., & Zender, J. F. (2008). Back to the beginning: Persistence and the cross-section of corporate capital structure. The Journal of Finance, 63(4), 1575–1608. https://doi.org/10.1111/j.1540-6261.2008.01369.x
- Mattana, P., Petroni, F., & Rossi, S. P. S. (2015). A test for the too-big-to-fail hypothesis for European banks during the financial crisis. Applied Economics, 47(4), 319–332. https://doi.org/10.1080/00036846.2014.959654
- Michelangeli, V., & Sette, E. (2016). How does bank capital affect the supply of mortgages? Evidence from a randomized experiment. Monetary and Economic Department of the Bank for International Settlements, BIS Working Papers No 557.
- Muriu, P. W. (2011). Microfinance profitability [ PhD Thesis]. Birmingham Business School, University of Birmingham.
- Oztekin, O., & Flannery, M. (2012). Institutional determinants of capital structure adjustment speeds. Journal of Financial Economics, 103(1), 88–112. https://doi.org/10.1016/j.jfineco.2011.08.014
- Roodman, D. (2007). How to do xtabond2: An introduction to “difference” and “system” GMM in stata. Working Paper Number 103, The Center for Global Development.
- Ross, S. A., Westerfield, R. W., & Jaffe, J. F. (2003). Corporate finance (Sixth ed.). McGraw-Hill/Irwin.
- Shim, J. (2013). Bank capital buffer and portfolio risk: The influence of business cycle and revenue diversification. Journal of Banking & Finance, 37(3), 761–772. https://doi.org/10.1016/j.jbankfin.2012.10.002
- Sibilkov, V. (2009). Asset liquidity and capital structure. Journal of Financial and Quantitative Analysis, 44(5), 1173–1196. https://doi.org/10.1017/S0022109009990354
- Soumaré, I., Tchakoute Tchuigoua, H., & Hessou, H. T. S. (2020). Are microfinance institutions resilient to economic slowdown? Evidence from their capital ratio adjustment over the business cycle. Economic Modelling, 92, 1–22. https://doi.org/10.1016/j.econmod.2020.07.006
- Tchakoute Tchuigoua, H. (2015). Capital structure of microfinance institutions. Journal of Financial Services Research, 47(3), 313–340. https://doi.org/10.1007/s10693-013-0190-2
- Tchakoute Tchuigoua, H. (2016). Buffer capital in microfinance institutions. Journal of Business Research, 69(9), 3523–3537. https://doi.org/10.1016/j.jbusres.2016.01.034
- Tchakoute Tchuigoua, H., Soumaré, I., & Hessou, H. T. S. (2020). Lending and business cycle: Evidence from microfinance institutions. Journal of Business Research, 119, 1–12. https://doi.org/10.1016/j.jbusres.2020.07.022
- Tehulu, T. A. (2021a). Do location and legal status matter in microfinance institutions’ performance? Evidence from Sub-Saharan Africa. Development in Practice, 31(3), 404–420. https://doi.org/10.1080/09614524.2020.1853060
- Tehulu, T. A. (2021b). What drives microfinance institution lending behavior? Empirical evidence from Sub-Saharan Africa. International Journal of Emerging Markets. https://doi.org/10.1108/IJOEM-08-2020-1002
- Thibaut, D., & Mathias, L. (2014). Bank capital adjustment process and aggregate lending. Banque de France.
- Wagner, C., & Winkler, A. (2013). The vulnerability of microfinance to financial turmoil– Evidence from the global financial crisis. World Development, 51, 71–90. https://doi.org/10.1016/j.worlddev.2013.05.008