Abstract
Despite increasing research on the relationship between behavioral factors and investment performance, little is known about the perspective of emerging markets like Bangladesh. This paper investigates the mediating role of socio-political factors in explaining the relationship between behavioral factors and investment performance in the Dhaka Stock Exchange (DSE). Using structural equation modeling (SEM) on 1,123 completed responses, we find that social and political environment mediates investors’ behavior in translating into investment performance. The finding suggests behavioral factors and the socio-cultural context of Bangladesh can explain market anomalies seen for decades in the largest stock exchange in the country and demands further research in this direction. Additionally, results show herding effect has the strongest effect on investment performance through combined mediation effect of social and political factors. The study results have significant implications for both theoretical and practical analysts by contributing to the evolution of post-neoclassical finance theories from an emerging market viewpoint and suggesting the probable causes behind historical market instability in DSE, respectively.
1. Introduction
It is widely documented that, since inception in 1950s, finance as an independent academic field has gone through several transformations which, often termed as paradigm shifts, can be marked by inherent epistemological characteristics of the field (Gippel, Citation2012). Notably, during 1960s and 1970s, finance, in search for deterministic approaches to generating knowledge, established several models and theories, popularly known as modern finance theories (e.g., Capital Asset Pricing Model in 1960; Efficient Market Hypothesis in 1970; Black-Scholes-Merton Option Pricing Model in 1973), that helped it being recognized as a science discipline. This neoclassical finance was particularly dominated by a single logical basis of the institutionalized form of rational expectations approach of economics and that single-handedly defined the boundary of the research agenda in finance at that time (Gippel, Citation2012; Watson, Citation2007). However, later, in the verge of several catastrophic market anomalies during past few decades, the neoclassical finance was challenged and, unfortunately, it failed to provide satisfactory explanations to the market failures or anomalies often created by the noise traders (Ramiah et al., Citation2015). The naïve efforts of neo-classical finance to justify anomalies as “market inefficiencies” and transitory and subject to correction through arbitrage could not get proper ground as empirical evidence showed persistent anomalies in the financial market during 1980s till last decade (Keim, Citation2008). In late 1970s and early 1980s, Michael Jenson, founder and editor of Journal of Financial Economics, referring to recurring anomalies, expressed his concerns for the validity of the Efficient Market Hypothesis as he argued that the market data persistently inconsistent to the theory (Jensen, Citation1978). Also the deterministic mathematical models (e.g., Capital Asset Pricing Model, Arbitrage Pricing Theory) were challenged on the ground that they take “ … unrealistic assumptions in order to provide boundary conditions for a mathematical solution” (Nawrocki & Viole, Citation2014:11). In similar vein, by harshly criticizing the neo-classical paradigm as dead, Colander et al. (Citation2010) argued that, in a typical economic (or financial) model, assumptions and/or limitations are not explicitly depicted as such and, hence, at times of “exceptional” economic and financial crises, the deterministic models cease to give proper explanations (moreover, “exceptional” can be a contested concept as crisis recurs/sustain often in the financial market). Moreover, substantial empirical evidence shows that individuals with irrational behavior (often termed as noise traders) actively take part in the liquidity trading, speculation and hedging and that also challenges the EMH (Foster & Viswanathan, Citation1990, Citation1993; De Long et al., Citation1990).
Thus, the researchers urge for new theory and model that may address the causes of alleged “exceptionalities” by the deterministic mathematical models (Nawrocki & Viole, Citation2014) and this new shift in paradigm is termed as post-neoclassical approach (Davis, Citation2006, Citation2008). In the post-neoclassical approach, finance academics, researchers, practitioners, and intellectuals put forth rigorous efforts to explore alternative ways to solve issues (e.g., persistent anomalies) of the neo-classical finance. In doing so, finance now embracing new philosophical stance, the behavioral finance school of thoughts (Zahera & Bansal, Citation2018), where the idea of treating investors as “economic rational being” is challenged on the ground that investors are not “natural” rational decision maker (Rahman & Gan, Citation2020; Sachdeva et al., Citation2022). In addition, it is argued that the investors are subject to bounded rationality where less optimal/efficient decision is being made with incomplete information which are processed through cognitive bias associated with various behavioral factors (Jain, Walia, Gupta et al., Citation2020). Hence, all deterministic models are de-emphasized with novel ways, which explores on how such (ir)rationality or erratic behavior of individuals is being considered within a theory or model (Gippel, Colander et al., Citation2010). In similar vein, evidence from neuroscience, cognitive psychology, sociology, and evolutionary biology also rejects the notion that human is (instrumentally) rational and wealth-maximizing being (Gippel, Citation2012). Thus, objective of the post-neoclassical finance is not to falsify the traditional claim that humans are inherently rational, rather it highlights the fact that investment decision is subject to human characteristics and psychological process (Sachdeva et al., Citation2022) that complicates the reason of human mind and, hence evolving the field more so into behavioral finance that intends to make sense of the market “anomalies” through a more dynamic and fluid conception of rationality or behavior of economic agents.
Based on empirical evidence, while explaining persistent human errors or irrational behavior of traders in making economic decision (e.g., investment decision), a range of psycho-behavioral areas has been researched within the behavioral finance school of thoughts. Research areas covered within this new behavioral finance area are representative bias (Shah et al., Citation2018), availability bias (Meng, Citation2017; Shah et al., Citation2018), overconfidence (Ahmed & Duellman, Citation2013; Jain, Walia, Gupta et al., Citation2020; Trejos et al., Citation2018), anchoring (Furnham & Boo, Citation2011; Meub & Proeger, Citation2016), gamblers fallacy (Henderson, Citation2012; Hens & Vlcek, Citation2011), loss aversion bias (Aggarwal & Damodaran, Citation2019; Barberis et al., Citation2001; Best & Grauer, Citation2016; Jain, Walia, Gupta et al., Citation2020), mental accounting (Barberis et al., Citation2016; Richards, Citation2014; Rockenbach, Citation2004), market information (Hong et al., Citation2000), interpersonal influence (Hoffmann & Broekhuizen, Citation2009), moral hazard (could not find any paper), risk tolerance (Pak & Mahmood, Citation2015; Son & Nguyen, Citation2018), knowledge (Hoffmann & Broekhuizen, Citation2009; Nuzula et al., Citation2019), etc. These areas can further be grouped under broad theories in behavioral finance such as heuristics theory (Shah et al., Citation2018; Trejos et al., Citation2018), prospect theory (Kahneman & Tversky, Citation1979; Son & Nguyen, Citation2018), herding effect (Jain, Walia, Gupta et al., Citation2020; Kabir & Shakur, Citation2018; Metawa et al., Citation2018; Silva et al., Citation2019), market factors (Nuzula et al., Citation2019), and subjective expected utility theory (Nawrocki & Viole, Citation2014).
Although the above-mentioned factors are widely researched, there is dearth of such studies conducted in the context of an emerging economy like Bangladesh. The arguments of alleged, “market-anomalies” and investors’ rationality is also questionable in the context of empirical evidence of the Bangladeshi stock markets. Bangladeshi stock market experienced its first major crash in 1996 when within a short period of time in Dhaka Stock Exchange (DSE), “(all share price) index rose from about 800 points in June, 1996 to around 3,600 in November, 1996” (S. U. Ahmed et al., Citation2012) and, then it started to fall and came down to 488 in 1999. This market crash or alleged “anomaly” repeated in 2011. The bubble started to form in late 1999 since when within just over a year’s period the DSE (SPI) index more than doubled and reached to 6800 points at the end of 2010; and then, as usual, the index started to fall and came down to 4200 in September 2011 (S. U. Ahmed et al., Citation2012). Recently, the DSE has started to face high volatility again. The repeated bubble-crash and high volatility can no more be considered as mere market inefficiencies and subject to natural market correction, rather behavioral aspects needed to be explored to have better understanding about the Bangladeshi stock markets (M. T. Rahman et al., Citation2017).
Hence, this study intends to explore the comprehensive factors of behavioral finance that influence investors’ decision-making process in the context of Bangladesh and, subsequently, supplement the literature from an emerging economy’s perspective. In addition, it is worth mentioning that the paper has taken the initiative of focusing on two new aspects other than the widely accepted factors of behavioral finance (as discussed above). The newly researched two other unique factors, socio-culture and political environment, are considered in this study which is a unique contribution of this study as well. As Bangladesh is a high context culture, it’s unique social and political environment is deemed to influence investment decisions through factors. The paper proceeds in the following order. Section 2 discusses the key characteristics/arguments of the relevant theories of behavioral finance and, subsequently, in Section 3, hypothesis and the Model are deduced and methodological aspects are discussed. Section 4 reports the findings of the data. Section 5 discusses the empirical implications. Section 6 illustrates the conclusion.
2. Theoretical framework
The focus of this study is to assess the influence of various psycho-behavioral factors on investment decisions. Initially a little insight into behavioral factors is discussed that includes evidence of behavioral finance in investment decisions. The independent variables for the study are built based on the behavioral finance theories and evidence such as heuristic, prospect, market, and herding. Subsequently, apart from the traditional behavioral factors, socio-cultural, and political factors are discussed as they may be considered as unique factors for the Bangladeshi context.
2.1. Heuristics
Systematic researches on cognitive Heuristics, linking to the concept of rationality, started in 20th century (Gigerenzer, Citation2008). The main arguments relate to how probability or material information (broadly rationality) is compromised when decision making focuses more on “self-defined satisfaction” over mathematical optimization. One of the elements of Heuristic is the Anchoring where investors come to a final decision by adjusting (may not be sufficient) some preliminary information (Matsumoto et al., Citation2013). Individual investor’s processual evaluation of initial information and adjustments to that can be referred to as “cognitive shortcut” (Kahneman & Tversky, Citation1974). Evidence also shows that overconfidence is another aspect of Heuristics that influences individual decision making (Pauschunder, Citation2017). It is to be noted that the overconfident investors are not considered to be incompetent investors rather it is their judgment or evaluation of information deviates than that of the actual situation (Trejos et al., Citation2018); and, surprisingly, such investors are confident about their ability regarding understanding financial market (Subash, Citation2012). Representativeness is another dimension of Heuristics where investors make investment decision in an uncertain situation based on “similar” cases where similarity concept is contestable as investors often ignore critical comparability factors (e.g., base rate, sample size) in the decision-making process (Meng, Citation2017). Availability bias is another element of Heuristics that illustrates how investors often make decision based on information/cases that they can easily recall and relate (Meng, Citation2017). Thus, decisions taken with such a manner are subject to estimation bias. Finally, investors sometimes ignore the law of statistical independence and form decision based on association of future outcome with past results (Barron & Leider, Citation2010). Such decision-making approach that are not based on statistical rigor is referred to as gambler’s fallacy, which often influence investment decisions. With this background, it is clear that, due to the presence of the elements (representativeness, availability bias, overconfidence, anchoring, and gamblers fallacy) of the Heuristics, investors end up in making irrational investment decisions, which subsequently has an impact on investment performance.
2.2. Prospect theory
Prospect theory was established on the evidence based on experimental studies that unfolded human irrational behavior to traditional utility theory when faced with expected gains and losses (Benartzi & Thaler, Citation1995; Best & Grauer, Citation2016; Kahneman & Tversky, Citation1979). In relation to the prospect theory, evidence shows that investors are loss averse in nature when followed by a prior loss and, in contrast, more susceptible to take risk followed by a prior gain (Barberis et al., Citation2001; Thaler & Johnson, Citation1990). From another angle, it is often argued that the investors seek for less risky investment choices when faced with a fear of realizing loss, and subsequently emotional pain, out of a decision. This behavior is termed as regret aversion bias which is supported by evidence (Zeelenberg et al., Citation1996). Experimental study conducted by Zeelenberg et al. (Citation1996) shows that people faced with decision dilemma in uncertainty prefer to take loss-minimizing decision. Mental Accounting, another element of prospect theory, states that investors often segment a whole investment decision into several small parts and treat each part discretely. However, this evaluation technique or mental accounting process is inherently flawed (from the perspective of rational portfolio theory) as the portfolio to be assessed as a whole not in such a decomposed manner (Rockenbach, Citation2004). Assessment of small units of investment separately cannot balance the performance of whole portfolio in an integrated manner. With reference to this discussion, it is clear that, due to the presence of the elements (loss aversion, regret aversion and mental accounting) of the Prospect Theory, investors end up in making irrational investment decisions, which subsequently has an impact on investment performance.
2.3. Market factors
Various market factors such as price fluctuations, price/return trends, fundamentals underlying stocks are considered to be vital elements that influence investment decisions but these factors are not free from behavioral biases. In other words, investors’ characteristics, emotions and psychology are also subject to behavioral factors which eventually influence their investment decisions (Jain, Walia & Gupta, Citation2020). In a rational paradigm, any material information should be discounted properly in determining fair value of financial assets but investors often construct valuation which are subject to their internal customs, level of knowledge, values, beliefs, perceptions, self-defined reasoning, memory, emotion, and environmental factors which make the decision-making process complicated (Nuzula et al., Citation2019). Decisions influenced by such factors are referred to as irrational and often results in under-reaction or over-reaction to any new information, reliance on momentum or trends and valuing more on short-term opportunistic outcomes (Barberis & Thaler, Citation2003; De Bondt & Thaler, Citation1985; Lai et al., Citation2001; Nuzula et al., Citation2019; Waweru et al., Citation2008). With such arguments this study uses market factors and uses them to construct behavioral factors which affect the decision-making process of investors in the capital market.
2.4. Herding effect
Research suggests that herding is relatively a common behavioral-phenomenon that can be traced in various academic disciplines such as psychology, investment, and auction (Satish & Padmasree, Citation2018). In case of financial market, investors subject to herding ignore material information and are influenced by peers in making investment decisions (Barber & Odean, Citation2000). Particularly, herding behavior of the investors is accused for creating noise or volatility (Silva et al., Citation2019) and, often lead to speculative bubble (Sachdeva et al., Citation2021) in the financial market. A study conducted by Hoffmann and Broekhuizen (Citation2009) found that incompetent investors and those who perceive investment decision is too risky to handle are more prone to follow others. Moreover, the study also found that, in many instances, investors are influenced by peers such intensely that they change their own preferences even with lower performing stocks (Hoffmann & Broekhuizen, Citation2009). The study infers that it may happen as investors are more inclined to social environment to collect information and, hence, often fall under peer pressure to comply. In similar vein, it is argued that, “ … investors’ self-image based on in-group preferences and the perception of group belongingness confirms the role of social identification in an investment decision” (Nuzula et al., Citation2019:52). A recent study conducted by Espinosa-Méndez and Arias (Citation2020) found that, in the Australian Stock Market, herding behavior is evident during COVID-19 pandemic period where investors abstained from investing when faced with crisis situation. This phenomenon is explained as “panic behavior” in crisis situation when investors, due to lack of time and information to analyze uncertain situation, compromise rationality and follow crowd decision (Abd-Alla, Citation2020; Sachdeva et al., Citation2021). However, contrasting result is also reported about herding behavior. A recent study shows that the herding behavior does not significantly influence investment decision (Rahman & Gan, Citation2020). In any case, it is worth noticing that the herding behavior is much researched in recent times (Chauhan et al., Citation2020) as its influence is widely accepted for forming speculative bubble and stock market volatility and, hence, the purpose of this study is to find out how individual decision-making at the DSE is also influenced by interpersonal influences and, subsequently, overall effect on the market.
2.5. Socio-culture and political factors within behavioral paradigm
Investor behavior studies have been conducted in various different countries and it is found that the country of origin affects investor behavior in varying ways. Apart from the commonly researched behavioral finance factors, it is to be noted that socio-culture is another unique dimension, which can alter the expected results of a behavioral study, particularly between developed and developing and/or emerging economies. A study conducted by Son and Nguyen (Citation2018) showed how culture could alter the relationship between prospect theory value and future return between Korean and US stock market. Another study conducted by Chua et al. (Citation2010) revealed that interpretation of information by investors could vary with their socio-cultural orientation, individualism or collectivism. Likewise, a recent study on individual decision making in India shed light on the fact that individuals in India react differently in ambiguous situation due to unique cultural values and are influenced more so by its collectivism which can differ from the developed world and, hence, the researchers urged that further studies are needed in this area (Aggarwal & Damodaran, Citation2019). Having this background, as Bangladesh belongs to a high context culture, its influence on investment decisions of individuals has been explored in this study. From a different angle, political factors and their impact on the capital market is also quite prominent in the context of Bangladesh. Existing research shows that investment decisions of a specific group of investors are responsive to the local political environment (Hood et al., Citation2014). Overall, socio-culture and political factors influences the behavioral factors (i.e., Heuristics, Prospect Theory, Herding Effect, Market Factors), which in eventually effects the investment performance. Therefore, socio-culture and political factors serves as mediator in the relationship between behavioral factors and investment performance. This is a novel area to be researched for the investors of Bangladesh and therefore the political environment is examined in this study as well.
3. Research model
With reference to above discussion, Heuristics, Prospect Theory, Herding Effect, Market Factors, Socio-cultural and Political factors are considered in formulating the conceptual framework (). Various behavioral biases, along with socio-cultural and political factors, used as independent variables to explore how they affect investment decision which in turn affect investment performance.
3.1. Hypothesis
To sum up the ongoing theoretical discussion and research model, the following hypotheses (H) are derived for testing:
H1: Behavioral factors have an impact on investment performance.
H2: There is a mediation effect of political factors between the relationship of behavioral factors and investment performance.
H3: There is a mediation effect of social (socio-cultural) factors between the relationship of behavioral factors and investment performance.
H4: There is a combined mediation effect of social and political factors among the relationship of behavioral factors and investment performance.
3.2. Data and methods
3.2.1. Survey instrument
To evaluate the developed hypotheses and to measure constructs of the framework, multi-item scales were used. All items were assessed by using a six-point Likert scale ranging from 1 (Extremely disagree) to 6 (Extremely agree). Summary of the questionnaire is presented in . Moreover, this study evaluated control variables for testing the relationship between the latent variables. Age, trading experience, income and invested amount in DSE have been tested against the investment performance to check the controlling effects. The questionnaire was developed with a total of 45 questions, out of them 34 questions were used for evaluating seven constructs, three questions were used as the screening questions and the rest of the questions were used for assessing the demographic profile of the respondents (see ).
Table 1. Summary of the questionnaire
3.2.2. Sampling and data collection
The target was to collect a relatively large sample for this study as observing behavioral relationship calls for a bigger sample, though it was suggested that for quantitative research at least 100 respondents should be studied to have a statistically conclusive result (Hair et al., Citation1999; Saunders et al., Citation2009). Luong and Thu Ha (Citation2011) and Sochi (Citation2018) collected 172 and 203 samples, respectively, in somewhat similar studies examining the relationship between behavioral factors and investment performance.
In this study, from the whole population of stockbroker-house-customers listed in DSE, 30 stockbroker-house-customers were selected by considering their size and duration of operation. According to Sudman and Blair (Citation1999), to reduce the error of data collection, a judgmental sampling method should be applied, as this allows to select the participants according to their expertise and knowledge relevant to the study (Littig, Citation2009; Plake & Impara, Citation2001). Accordingly, a judgmental sampling method was applied on the 30 stockbroker-house-customers and respondents were picked based on their active association, invested amount and experience of stock trading.
A pilot test was conducted with 10% of the total sample to check the data quality and normality. The result of the pilot test was acceptable to continue further analysis. In the second stage, 2000 questionnaires were circulated and received 1157 responses (58% response rate). Finally, after removing the incomplete responses the total sample size of 1,123 is used to conduct structural equation modeling (SEM). According to Jak et al. (Citation2020), from power analysis sample size of 1,123 is sufficient for SEM analysis. The demographic profile of the respondent is presented in .
Table 2. Demographic profile
3.3. Methodology
The methodology applied for data analysis can be divided into two segments. At the first stage, descriptive statistics and other statistical tests such as, mean, median, standard deviation, skewness, kurtosis, and correlation of coefficients were analyzed and results are presented in . Statistical programming Language R is used for the data analysis and specifically, the lavaan package is used for conducting SEM analysis. A two-step modeling approach is applied for this study to perform SEM (Anderson & Gerbing, Citation1988). At first, the measurement model is investigated such as reliability, factor loading, and goodness-of-fit for every scale linked to this study. In the second step structural model is examined to see the comprehensive connection between the different variables and checked how each variable reacts in the model. Nevertheless, this step also examined all paths of proposed models and estimated the fit indices of the structural model such as chi-square p-value, CFI, TLI, GFI, AGFI, RMSEA, and SRMR.
Table 3. Descriptive statistics and correlation analysis
4. Analysis and results
4.1. Measurement of validity and reliability
presents the results of confirmatory factor analysis evaluating the validity and reliability of the constructs. Values of comparative fit index (CFI) = 0.97, Tucker-Lewis index (TLI) = 0.96, goodness-of-fit index (GFI) = 0.97, adjusted goodness-of-fit index (AGFI) = 0.96, Chi-square p-value (p) = 0.00, root mean square error of approximation (RMSEA) = 0.05, and Standardized Root Mean Square Residual (SRMR) = 0.06 indicate that measurement model fits satisfactorily, as the values of CFI, TLI, GFI exceeding 0.90 and AGFI exceeding 0.8 indicates the goodness-of-fit (Bentler, Citation2007; Fan & Wang, Citation1998). Additionally, the acceptable value of χ2 p-value is less than 5%, RMSEA and SRMR is less than 0.08 (Anderson & Gerbing, Citation1988; Browne & Cudeck, Citation1993).
Table 4. Reliability and discriminant validity
Details are presented in Table which shows that each item factor loading (FL) is above 0.50. Moreover, the Cronbach’s alpha (α) and composite reliability (CR) values are more than the cutoff value of 0.70 (Cronbach & Warrington, Citation1951; Nunnally, Citation1978). Additionally, the average variance extracted (AVE) demonstrates the convergent validity of the items and all of them were above the threshold value of 0.5 (Fornell & Larcker, Citation1981).
4.2. Structural model
Following the confirmatory factor analysis results, this study moved forward to the second stage of the two-step modeling. represents the SEM, which shows the evidence of fitting the hypothesized model significantly (p = 0.00, CFI = 0.96, TLI = 0.96, RMSEA = 0.5, SRMR = 0.07, GFI = 0.97, AGFI = 0.96) within the indices of the threshold value (Anderson & Gerbing, Citation1988; Bentler, Citation2007; Browne & Cudeck, Citation1993; Fan & Wang, Citation1998).
4.3. Hypothesis testing
For hypothesis testing four models are developed according to the research model presented earlier. and present the model fitness and hypothesis testing results, respectively.
Table 5. Model fitness indices
Table 6. Hypothesis testing
Model 1 presents the interaction between behavioral factors and investment performance. All fitness measures confirm the model fitness (Anderson & Gerbing, Citation1988; Bentler, Citation2007; Browne, Citation1993; Fan & Wang, Citation1998) and hypothesis 1 testing results accept the hypothesis significantly (Coefficients = −0.21 to 0.14). However, the results of hypothesis testing for hypothesis 1 indicate that each variable of behavioral factor has a significant impact (p-value <0.2) and lend support to H1 that behavioral factors have impact on investment performance.
In model 2, political factor was included as a mediator between behavioral factors and investment performance. Threshold values of all fitness indicators demonstrate the fitness of model 2 (Anderson & Gerbing, Citation1988; Bentler, Citation2007; Browne, Citation1993; Fan & Wang, Citation1998). Results of hypothesis testing indicate that there is a significant direct and total mediator effect of political factors between behavioral factors and investment performance (Coefficients = 0.01 and −0.06). Moreover, after the inclusion of mediator, all variables of behavioral factors have a significant coefficient value (p-value <0.2). Thus, results accept the hypothesis that there is a mediation effect of political factors between the relationship of behavioral factors and investment performance.
Next model 3 included social (culture) factor as the mediator among behavioral factors and investment performance. The fitness indices here support the goodness of fit of the model (Anderson & Gerbing, Citation1988; Bentler, Citation2007; Browne, Citation1993; Fan & Wang, Citation1998). The results of hypothesis testing demonstrate the significant direct and complete mediating effect of social factor in between behavioral factors and investment performance (Coefficients = 0.02 and −0.06). The results of the hypothesis testing reveals that there is a mediation effect of social factor in determining the relationship between behavioral factors and investment performance.
Finally, model 4 included both political and social factor as mediating factors in determining the relationship between behavioral factors and investment performance. Revealed results were found to be positive. All fitness indices were within the tolerable threshold value (Anderson & Gerbing, Citation1988; Bentler, Citation2007; Browne, Citation1993; Fan & Wang, Citation1998). After the combined inclusion, all variables of behavioral factors register a positive impact on investment performance. These results lend support to the hypothesis that there is a full mediating effect of political and social factor among behavioral factors and investment performance.
presents the result of applying control variables which indicates that there is no controlling impact of Age, Gender, Trading Experience, Monthly Income, Investment at the Stock Exchange on dependent variable, investment performance.
Table 7. Control variables estimation
5. Discussion
Overall, findings of the study indicate that behavioral factors: heuristic, prospect, market, and herding, do have impact in determining investment performance of the investors. This is consistent with findings by several studies (Waweru et al., Citation2008; Lehenkari & Perttunen, Citation2004; Barberis et al., Citation2001; Barberis & Thaler, Citation2003; Tan et al., Citation2008; & Durand et al., Citation2008). Sochi (Citation2018) also found the presence of heuristic, prospect theory and market biases in DSE. Historically, the principal stock exchange of the country, (DSE), has been quite volatile. It has experienced several crashes in 1996, 2011 and 2019. Key reasons identified behind these crashes are short-term profit motive and lack of knowledge base of investors to make rational choices (S.U. Ahmed et al., Citation2014). They are susceptible to errors and irrational behaviors that substantiates arguments in favor of post-neoclassical behavioral finance theories (Colander et al., Citation2010; Gippel, Citation2012). As a result, the results of this study showing evidence of behavioral biases among DSE investors are justifiable.
The presence of mediating impact of political factors in defining the relationship between behavioral factors and investment performance is an insightful findings form this study. DSE experienced a huge rise in share price index after the general elections in 1996 and 2008 (M.A. Rahman et al., Citation2013). This may be due to the positive expectations of the investors from the newly formed government and can be termed as post-election effect. However, this jubilation did not continue due to subsequent abrupt fall in stock prices (S. U. Ahmed et al., Citation2012). Political consideration does have an impact in the investment decision-making process and investors do know about this and are responsive to the domestic political environment (Hood et al., Citation2014). Again, statistical significance of the mediating role of social factor found in the study is consistent with existing literature where it was found that cultural orientation of the investors may lead towards varying interpretations of information by the investors (Chua et al., Citation2010; Son & Nguyen, Citation2018). Typical cultural factors of Bangladeshi investors featured by reciprocity, social consciousness, and myopic investment behavior proved to be statistically significant. Accordingly, contrary to the previous studies dismissing investors to be ignorant (Securities and Exchange Commission (SEC), Citation2006), the findings of the study suggest that investors do consider the socio-political environment of the country while making their investment decisions.
Finally, the full mediating effect of political and social factors in determining the relationship between behavioral factors and investment performance provides ground to the post-neoclassical finance theories and confirms that anomalies exist in investors behavior in DSE (Sochi, Citation2018). However, these behavioral biases can be better explained by investors’ consciousness about the socio-political environment and their investment decisions and outcome are not solely based on rationality.
6. Conclusion
In this paper, an important but understudied aspect, namely, socio-political factors have been examined in explaining the behavior of investors linking with investment performance. The findings of the study reveal an explanation to the complex balancing act of DSE investors in managing risk and return. Cultural mindset, political developments in the country, particularly general election dates, influence investment decision-making process.
Given these findings, this research has a series of theoretical and practical implications for practitioners, scholars and policy makers. It relates to the concerns of policy makers and regulators, who are trying to bring stability in the capital market of Bangladesh and prevent frequent crashes. Also, the practitioners such as stock brokers, bankers, investment managers will be benefited by knowing that, contrary to the conventional belief, investors consider information before investing which are indeed reflected in their investment performance. Theoretical analysts may take the discussion further in evolving the post-neoclassical finance theories to accommodate the fluid conception of rationality in shaping the behavior of investors. However, like most of the studies this research is not also free from shortcomings. Inclusion of respondents only from DSE limits the generalization ability of the paper to entire investment climate of Bangladesh. A more comprehensive study by extending the theoretical outreach, including respondents from Chittagong Stock Exchange and broadening the sample size might be conducted in the future to have a better understanding.
correction
This article has been corrected with minor changes. These changes do not impact the academic content of the article.
Disclosure statement
No potential conflict of interest was reported by the author(s).
Additional information
Funding
Notes on contributors
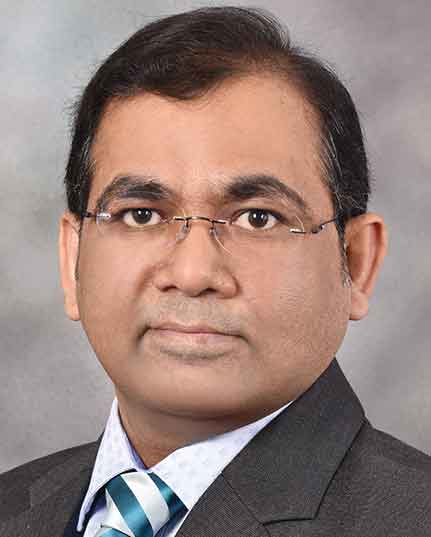
Sarwar Uddin Ahmed
Sarwar Uddin Ahmed currently serves as the Professor and Dean of Academic Affairs of Monash College Programs at Universal College Bangladesh (UCB). He came to UCB from Independent University, Bangladesh (IUB), where he was the Dean and Professor of Finance at the School of Business and Entrepreneurship (SBE). Professor. Ahmed has authored over 100 articles, books, book chapters, reports, and conference papers in peer-reviewed journals. His research interests cut across behavioral finance, climate finance, corporate social performance, and Environmental, Social and Governance (ESG) risk management. He has served as the Chief Editor, Conference Chair and sits on high-impact journal editorial boards.
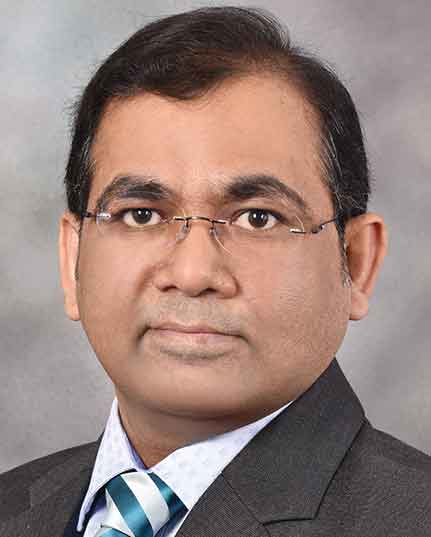
Samiul Parvez Ahmed
Sarwar Uddin Ahmed currently serves as the Professor and Dean of Academic Affairs of Monash College Programs at Universal College Bangladesh (UCB). He came to UCB from Independent University, Bangladesh (IUB), where he was the Dean and Professor of Finance at the School of Business and Entrepreneurship (SBE). Professor. Ahmed has authored over 100 articles, books, book chapters, reports, and conference papers in peer-reviewed journals. His research interests cut across behavioral finance, climate finance, corporate social performance, and Environmental, Social and Governance (ESG) risk management. He has served as the Chief Editor, Conference Chair and sits on high-impact journal editorial boards.
References
- Abd-Alla, M. H. (2020). Sentimental herding: The role of covid-19 crisis in the Egyptian stock market. Copernican Journal of Finance and Accounting, 9(3), 9–22. https://doi.org/10.12775/CJFA.2020.009
- Abdin, S. Z. U., Farooq, O., Sultana, N., & Farooq, M. (2017). The impact of heuristics on investment decision and performance: Exploring multiple mediation mechanisms. Research in International Business and Finance, 42(2017), 674–688. https://doi.org/10.1016/j.ribaf.2017.07.010
- Aggarwal, D., & Damodaran, U. (2019). Ambiguity attitudes and myopic loss aversion: Experimental evidence using carnival games. Journal of Behavioral and Experimental Finance, (2019), 25.https://doi.org/10.1016/j.jbef.2019.100258
- Ahmed, A. S., & Duellman, S. (2013). Managerial overconfidence and accounting conservatism. Journal of Accounting Research, 51(1), 1–30. https://doi.org/10.1111/j.1475-679X.2012.00467.x
- Ahmed, S. U., Islam, M. Z., Mahtab, H., & Hasan, I. (2014). Institutional investment and corporate social performance: Linkage towards sustainable development. Corporate Social Responsibility and Environmental Management, 21(1), 1–13. https://doi.org/10.1002/csr.1298
- Ahmed, S. U., Uchida, S., & Islam, M. Z. (2012). Stock market crashes: Reasons implications. KEIEI TO KEIZAI, 91(4), 55–66. https://ssrn.com/abstract=2342519
- Anderson, J. C., & Gerbing, D. W. (1988). Structural equation modeling in practice: A review and recommended two-step approach. Psychological Bulletin, 103(3), 411. https://doi.org/10.1037/0033-2909.103.3.411
- Barber, B. M., & Odean, T. (2000, January/February). Too many Cooks spoil the profits: The performance of investment clubs. Financial Analysts Journal, 70(1), 17–25. https://doi.org/10.2469/faj.v56.n1.2326
- Barberis, N., Huang, M., & Santos, T. (2001). Prospect theory and asset prices. Quarterly Journal of Economics, 116(1), 1–53. https://doi.org/10.1162/003355301556310
- Barberis, N., Mukherjee, A., & Wang, B. (2016). Prospect Theory and Stock Returns: An Empirical Test. The Review of Financial Studies, 29(11), 3068–3107. https://doi.org/10.1093/rfs/hhw049
- Barberis, N., & Thaler, R. (2003). Asurvey of behavioral finance. In G. Harris & M. Stulz (Eds.), Handbook of the Economics of Finance (Vol. 1, no.1, pp. 1053–1128). Elsevier.
- Barron, G., & Leider, S. (2010). The role of experience in the Gambler’s Fallacy. Journal of Behavioral Decision Making, 23(1), 117–129. https://doi.org/10.1002/bdm.676
- Benartzi, S., & Thaler, R. (1995). Myopic loss aversion and the equity premium puzzle. The Quarterly Journal of Economics, 110(1), 73–92. https://doi.org/10.2307/2118511
- Bentler, P. M. (2007). On tests and indices for evaluating structural models. Personality and Individual Differences, 42(5), 825–829. https://doi.org/10.1016/j.paid.2006.09.024
- Best, M. J., & Grauer, R. R. (2016). Prospect theory and portfolio selection. Journal of Behavioal and Experimental Finance, 11(2016), 13–17. http://dx.doi.org/10.1016/j.jbef.2016.05.002
- Browne, M. W, Cudeck, R. (1993). Alternative ways of assessing model fit. In K. A. Bollen, & J. S. Long (eds), Testing structural equation models (vol. 154, pp. 136–162).
- Chauhan, Y., Ahmad, N., Aggarwal, V., & Chandra, A. (2020). Herd behaviour and asset pricing in the Indian stock market. IIMB Management Review, 32(2), 143–152. https://doi.org/10.1016/j.iimb.2019.10.008
- Chua, C. T., Goh, J., & Zhang, Z. (2010). Expected volatility, unexpected volatility, and the cross-section of stock returns. The Journal of Financial Research, 33(2), 103–123. https://doi.org/10.1111/j.1475-6803.2010.01264.x
- Colander, D., Goldberg, M., Haas, A., Kirman, A., Juselius, K., Sloth, B., & Lux, T. (2010). The financial crisis and the systemic failure of academic economics. In Q. Rob, & R. W. Kolb (Eds.), Lessons from the financial crisis: causes, consequences and our economic future (pp. 427–436).John Wiley.
- Cronbach, L. J., & Warrington, W. G. (1951). Time-limit tests: Estimating their reliability and degree of speeding. Psychometrika, 16(2), 167–188. https://doi.org/10.1007/BF02289113
- Davis, J. (2006). The turn in economics: Neoclassical dominance to mainstream pluralism? Journal of Institutional Economics, 2(1), 1–20. https://doi.org/10.1017/S1744137405000263
- Davis, J. (2008). The turn in recent economics and return of orthodoxy. Cambridge Journal of Economics, 32(3), 349–366. https://doi.org/10.1093/cje/bem048
- De Bondt, W. F. M., & Thaler, R. (1985). Does the stock market over react? Journal of Finance, 40(3), 793–805. https://doi.org/10.1111/j.1540-6261.1985.tb05004.x
- De Long, J., Shleifer, A., Summers, L., & Waldman, J. (1990). Noise trader risk in financial markets. Journal of Political Economy, 98(4), 703–738. https://doi.org/10.1086/261703
- Durand, R. B., Newby, R., & Sanghani, J. (2008). An Intimate Portrait of the Individual Investor. The Journal of Behavioral Finance, 9(4), 193–208. https://doi.org/10.1080/15427560802341020
- Espinosa-Méndez, C., & Arias, J. (2020). Herding behaviour in Asutralian stock market: Evidence on COVID-19 effect. Applied Economics Letters, 281, 1898–1901. https://doi.org/10.1080/13504851.2020.1854659
- Fan, X., & Wang, L. (1998). Effects of potential confounding factors on fit indices and parameter estimates for true and misspecified SEM models. Educational and Psychological Measurement, 58(5), 701–735. https://doi.org/10.1177/0013164498058005001
- Fornell, C., & Larcker, D. F. (1981). Evaluating structural equation models with unobservable variables and measurement error. Journal of Marketing Research, 18(1), 39–50. https://doi.org/10.1177/002224378101800104
- Foster, F. D., & Viswanathan, S. (1990). A theory of the interday variations in volume, variance, and trading costs in securities markets. Review of Financial Studies, 3(4), 593–694. https://doi.org/10.1093/rfs/3.4.593
- Foster, F. D., & Viswanathan, S. (1993). The effect of public information and competition on trading volume and price volatility. Review of Financial Studies, 6(1), 23–56. https://doi.org/10.1093/rfs/6.1.23
- Furnham, A., & Boo, H. C. (2011). A literature review of the anchoring effect. The Journal of Socio-Economics, 40(1), 35–42. http://dx.doi.org/10.1016/j.socec.2010.10.008
- Gigerenzer, G. (2008). Why heuristics work? Association for Psychological Science, 3(1), 20–29. https://doi.org/10.1111/j.1745-6916.2008.00058.x
- Gippel, J. K. (2012). A revolution in finance? Australian Journal of Management, 38(1), 125–146. https://doi.org/10.1177/0312896212461034
- Hair, J. F., Anderson, R. E., Tatham, R. L., & Black, W. C. (1999). Análisismultivariante (Vol. 491). Prentice Hall.
- Henderson, V. (2012). Prospect theory, liquidation, and the disposition effect. Management Science, 58(2), 445–460. https://doi.org/10.1287/mnsc.1110.1468
- Hens, T., & Vlcek, M. (2011). Does prospect theory explain the disposition effect? Journal of Behavioral Finance, 12(3), 141–157. https://doi.org/10.1080/15427560.2011.601976
- Hoffmann, A. O. I., & Broekhuizen, T. L. J. (2009). Susceptibility to and impact of interpersonal influence in an investment context. Journal of the Academy ofMarketing Science, 37(4), 488–503. https://doi.org/10.1007/s11747-008-0128-7
- Hong, H., Lim, T., & Stein, J. (2000). Bad news travels slowly: Size, analyst coverage, and the profitability of momentum strategies. Journal of Finance, 55(1), 265–295. https://doi.org/10.1111/0022-1082.00206
- Hood, M., Nofsinger, J., & Varma, A. (2014). Conservation, discrimination, and salvation: Investors’ social concerns in the stock market. Journal of Financial Services Research, 45(1), 5–37. https://doi.org/10.1007/s10693-013-0162-6
- Jain, J., Walia, N., & Gupta, S. (2020). Evaluation of behavioral biases affecting investment decision making of individual equity investors by fuzzy analytic hierarchy process. Review of Behavioral Finance, 12(3), 297–314. https://doi.org/10.1108/RBF-03-2019-0044
- Jak, S., Jorgensen, T. D., Verdam, M. G., Oort, F. J., & Elffers, L. (2020). Analytical power calculations for structural equation modeling: A tutorial and Shiny app. Behavior Research Methods, 53, 1–22. https://doi.org/10.3758/s13428-020-01479-0
- Jensen, M. (1978). Some anomalous evidence regarding market efficiency. Journal of Financial Economics, 6(2–3), 95–101. https://doi.org/10.1016/0304-405X(78)90025-9
- Kabir, M. H., & Shakur, S. (2018). Regime-dependent herding behavior in Asian and Latin American stock markets. Pacific-Basin Finance Journal, 47(1), 60–78. http://dx.doi.org/10.1016/j.pacfin.2017.12.002
- Kahneman, D., & Tversky, A. (1974). Judgment under uncertainty: Heuristics and biases. Science, 85(4157), 1124–1131. http://links.jstor.org/sici?sici=0036-8075%2819740927%293%3A185%3A4157%3C1124%3AJUUHAB%3E2.0.CO%3B2-M
- Kahneman, D., & Tversky, A. Prospect theory: An analysis of decision under risk. (1979). Econometrica, 47(2), 263–291. Chapter 6. https://doi.org/10.2307/1914185
- Keim, D. (2008). Financial market anomalies. In S. N. Durlauf & L. E. Blume (eds.), The New Palgrave Dictionary of Economics Blume. Palgrave Macmillan, London. https://doi.org/10.1007/978-1-349-58802-2_569
- Lai, M., Low, K. L. T., & Lai, M.-L. (2001). Are Malaysian investors rational? Journal of Psychology and Financial Markets, 2(4), 210–215. https://doi.org/10.1207/S15327760JPFM0204_5
- Lehenkari, M., & Perttunen, J. (2004). Holding onto the losers: Finish evidence. The Journal of Behavioral Finance, 5(2), 116–126. https://doi.org/10.1207/s15427579jpfm0502_5
- Littig, B. (2009). Interviewing the elite–interviewing experts: Is there a difference? Springer.
- Luong, L. P., & Thu Ha, D. T.(2011). Behavioral factors influencing individual investors’ decision-making and performance: A surveyt the Ho Chi Minh stock exchange. Unpublished M.Sc. Thesis.Umeå School of Business.
- Matsumoto, A. S., Fernandes, J. L. B., Ferreira, I. K. L., & Chagas, P. C. (2013). Behavioral finance: A study of affect heuristic and anchoring in decision making of individual investors. SSRN Electronic Journal. https://doi.org/10.2139/ssrn.2359180
- Meng, S. (2017). Availability heuristic will affect decision-making and result in bias, 2017 3rd International Conference on Management Science and Innovative Education (MSIE 2017).
- Metawa, N., Hassan, M. K., Metawa, S., & Safa, M. F. (2018). Impact of behavioral factors on investors;’ financial decisions: Case of the Egyptian stock market. International Journal of Islamic and Middle Eastern Finance and Management, 12(1), 30–55. https://doi.org/10.1108/IMEFM-12-2017-0333
- Meub, L., & Proeger, T. (2016). Can anchoring explain biased forecasts? Experimental Evidence, Journal of Behavioral and Experimental Finance, 12(2016), 1–13. http://dx.doi.org/10.1016/j.jbef.2016.08.001
- Nawrocki, D., & Viole, F. (2014). Behavioral finance in financial market theory, utility theory, portfolio theory and the necessary statistics: A review. Journal of Behavioral and Experimental Finance, 2(2014), 10–17. https://doi.org/10.1016/j.jbef.2014.02.005
- Nunnally, J. C. (1978). Psychometric theory (2nd edit.) mcgraw-hill. Hillsdale, NJ, 416.
- Nuzula, N. F., Sisbintari, I., Suhadak, & Handayani, S. R. (2019). The use of technical analysis, source of information and emotion and its influence on investment decisions. Journal of Behavioral and Experimental Finance, 12(2016), 1–13. https://doi.org/10.1016/j.jbef.2019.02.004
- Pak, O., & Mahmood, M. (2015). Impact of personality on risk tolerance and investment decisions: A study on potential investors of Kazakhstan. International Journal of Commerce and Management, 25(4), 370–384. https://doi.org/10.1108/IJCoMA-01-2013-0002
- Pauschunder, J. M. (2017). Nudgitize Me! A behavioral finance approach to minimize losses and maximize profits from heuristics and biases. International Journal of Management Excellence, 10(2), 1241–1256. http://dx.doi.org/10.2139/ssrn.2930824
- Plake, B. S., & Impara, J. C. (2001). Ability of panelists to estimate item performance for a target group of candidates: An issue in judgmental standard setting. Educational Assessment, 7(2), 87–97. https://doi.org/10.1207/S15326977EA0702_1
- Rahman, M., & Gan, S. S. (2020). Generation Y investment decision: An analysis using behavioral factors. Managerial Finance, 46(8), 1023–1041. https://doi.org/10.1108/MF-10-2018-0534
- Rahman, M. A., Hasan, M. B., & Ahsan, A. M. (2013). Stock Market performance under different government periods: Evidence from Bangladesh. Universal Journal of Accounting and Finance, 1(2), 42–50. https://doi.org/10.13189/ujaf.2013.010202
- Rahman, M. T., Hossain, S. Z., & Habibullah, M. (2017). Stock market crash in Bangladesh: The moneymaking psychology of domestic investors. American Journal of Theoretical and Applied Business, 3(3), 43–53. https://doi.org/10.11648/j.ajtab.20170303.12
- Ramiah, V., Xu, X., & Moosa, I. A. (2015). Neoclassical finance, behavioural finance and noise traders: A review and assessment of the literature. International Review of Financial Analysis, Neoclassical Finance, 41, 89–100. https://doi.org/10.1016/j.irfa.2015.05.021
- Richards, T. (2014). Investing psychology: The effect of behavioral finance on investment choice and bias. Wiley.
- Rockenbach, B. (2004). The behavioral relevance of mental accounting for the pricing of financial options. Journal of Economic Behavior & Organization, 53(2004), 513–527. https://doi.org/10.1016/S0167-2681(03)00097-0
- Sachdeva, M., Lehal, R., Gupta, S., & Garg, A. (2021). What make investors herd while investing in the Indian stock market? A hybrid approach. Review of Behavioral Finance. https://doi.org/10.1108/RBF-04-2021-0070
- Sachdeva, M., Lehal, R., Gupta, S., & Gupta, S. (2022). Infleunce of contextual factors on investment decision-making: A fuzzy-AHP approach. Journal of Asia Business Studies. https://doi.org/10.1108/JABS-09-2021-0376
- Satish, B., & Padmasree, K. (2018). An empirical analysis of herding behaviour in Indian stock market. International Journal of Management Studies, 3(3), 124–132. https://doi.org/10.18843/ijms/v5i3(3)/15
- Saunders, M., Lewis, P., & Thornhill, A. (2009). Research methods for business students (Fifth ed.). Pearson Education Limited.
- Securities and Exchange Commission (SEC). 2006. NotificationEC/CMRRCD/2006-158/Admin/02-06
- Shah, S. Z. A., Ahmad, M., & Mahmood, F. (2018). Heuristic biases in investment decision-making and perceived market efficiency: A survey at the Pakistan stock exchange. Qualitative Research in Financial Markets, 10(1), 85–110. https://doi.org/10.1108/QRFM-04-2017-0033
- Silva, P. V. J. D. G., Klotzle, M. C., Pinto, A. C. F., & Gomes, L. L. (2019). Herding behavior and contagion in the cryptocurrency market. Journal of Behavioal and Experimental Finance, 22(2019), 41–50. https://doi.org/10.1016/j.jbef.2019.01.006
- Sochi, M. H. (2018). Behavioral factors influencing investment decision of the retail investors of dhaka stock exchange: An empirical study. The Cost and Management, 46(1), 20–29. https://www.icmab.org.bd/wp-content/uploads/2019/12/03.Behavioral-Factors-.pdf
- Son, N. T., & Nguyen, N. M. (2018). Prospect theory value and idiosyncratic volatility: Evidence from the Korean stock market. Journal of Behavioral and Experimental Finance, 21(2018), 113–122. https://doi.org/10.1016/j.jbef.2018.11.006
- Subash, R. (2012). Role of behavioral finance in portfolio investment decisions: Evidence from India. Charles University in Prague Faculty of Social Sciences Institute of Economic Studies.
- Sudman, S., & Blair, E. (1999). Sampling in the twenty-first century. Journal of the Academy of Marketing Science, 27(2), 269–277. https://doi.org/10.1177/0092070399272010
- Tan, L., Chiang, T. C., Mason, J. R., & Nelling, E. (2008). Herding behavior in Chinese stock markets: An examination of A and B shares. Pacific-Basin Finance Journal, 16(1–2), 61–77. https://doi.org/10.1016/j.pacfin.2007.04.004
- Thaler, R. H., & Johnson, E. J. (1990). Gambling with the house money and trying to break even: The effects of prior outcomes on risky choice. Management Science, 36(6), 643–660. https://doi.org/10.1287/mnsc.36.6.643
- Trejos, C., Deemen, A. V., Rodríguez, Y. E., & Gomez, M. (2018). Overconfidence and disposition effect in the stock market: A micro world based setting. Journal of Behavioral and Experimental Finance, 21(2018), 61–69. https://doi.org/10.1016/j.jbef.2018.11.001
- Watson, M. (2007). Searching for the Kuhnian moment: The Black-Scholes-Merton formula and the evolution of modern finance theory. Economy and Society, 36(2), 326–338. https://doi.org/10.1080/03085140701254340
- Waweru, N., Munyoki, M., & Uliana, E. (2008). The effects of behavioral factors in investment decision-making: A survey of institutional investors operating at the Nairobi stock exchange. International Journal of Business and Emerging Markets, 1(1), 24–41. https://doi.org/10.1504/IJBEM.2008.019243
- Zahera, S. A., & Bansal, R. (2018). Do investors exhibit behavioral biases in investment decision making? A systematic review. Qualitative Research in Financial Markets, 10(2), 210–251. https://doi.org/10.1108/QRFM-04-2017-0028
- Zeelenberg, M., Beattie, J., Pligt, J. V. D., & Vries, N. K. D. (1996, February). Consequences of Regret aversion: Effects of exoected feedback on risky decision making. Organizational Behavior and Human Decision Processes, 65(2), 148–158. https://doi.org/10.1006/obhd.1996.0013
Appendix A
Table A1. Survey instrument