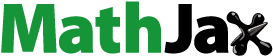
Abstract
FinTech has raised the risk-taking level of financial institutions. This paper aims to explore FinTech Credit (FTC) scale of non-financial institutions and the risk-taking level of financial institutions into Opiela’s model, constructs objective functions and constraints for representative financial institutions by conducting theoretical analysis and research hypothesis. It also explores the relationship between FTCscale and the proportion of agriculture-related loans. Based on the balanced panel data of 31 provinces and municipalities in China from 2009 to 2017, the individual fixed effects model is used to test the research hypothesis. Then, based on the balanced panel data of 31 provinces and municipalities in China from 2009 to 2017, the research hypothesis was tested using an individual fixed-effects model to explore the relationship between FTCscale and the proportion of agriculture-related loans. The results show that the FTCscale can increase the share of agriculture-related loans in financial institutions. Still, the percentage of agriculture-related loans and e-commerce factors increase at a marginal decreasing rate. Furthermore, the study shows that marketization and real estate development also indirectly affect the proportion of agricultural loans through the mediating part of the FTCscale. Finally, policy recommendations are proposed to develop FTC and the implementation of rural revitalization strategy.
1. Introduction
Rural revitalization depends on the development of agricultural economy. The development of the agricultural economy depends on the growth of agricultural total factor productivity, and the input of agricultural loans is a necessary condition for the growth of total factor productivity (Li & Wu, Citation2018). The increase of agriculture-related loans is also conducive to the “three rural problems” and thus to the implementation of rural revitalization strategy. However, with the withdrawal of many rural financial networks and the intensification of the separation of institutions and funds from farmers, the coverage rate of agriculture-related loans is still low, and the supply of agriculture-related loans is still insufficient (Yin et al., Citation2019). On 29 January 2019, the People’s Bank of China, the Banking and Insurance Regulatory Commission, the Securities Regulatory Commission, the Ministry of Finance, and the Ministry of Agriculture and Rural Affairs jointly issued the “Guidance on Financial Services for Rural Revitalization The guiding opinions also clearly put forward the active implementation of the project of Internet financial services for “three rural areas” and regulate the development of Internet finance (IF) in rural areas.
IFapproximates financial technology (FinTech) in China (Chen, Citation2016; He & Ong, Citation2014; Zhang et al., Citation2020; Zhang & Zhuang, Citation2020). FinTech innovations include financial institutions such as banks, securities, and insurance, and non-financial institutions such as Internet companies. Both the International Monetary Fund (IMF) and the U.S. Treasury Department consider that the FTC can practice financial inclusion and have a positive attitude toward it. In 2016 and 2017, the Party Central Committee and the State Council attempted to serve the “three rural areas” and achieve rural revitalization through FinTech innovation by financial and non-financial institutions. The “Guiding Opinions on Financial Services for Rural Revitalization” issued by the People’s Bank of China and four other ministries and commissions in 2019 also continued this policy theme. The Guidance on Financial Services for Rural Revitalization issued by the People’s Bank of China and four other ministries in 2019 also continues this policy keynote. Therefore, FinTech innovation of financial institutions and FTC of non-financial institutions are expected to become the two carriages for serving the “three rural areas” and practicing rural revitalization. Apart from directly serving the “three rural areas” and increasing agricultural-related loans, is there a spillover effect of FTC on the proportion of agricultural-related loans of financial institutions? If so, what kind of spillover effect is there? Is there any trust factor (Roh et al., Citation2022) affect FTC?
1.1. Review of literature
Fintech and agriculture has a close bonding in terms of sustainable development. Due to the digital marketplace, fintech aid agricultural sustainability (Anshari et al., Citation2019). Recent research revealed that Fintech firms’ portfolios have a advanced financial threat (Najaf et al., Citation2020). In addition, due to block chain technology, fintech has significant impact on the environment (Schinckus, Citation2021). The existing literature revolves around risk, cost, impact on financial institutions’ operating performance (Schinckus, Citation2020), and influencing factors regarding agriculture-related loans and e-commerce factors (Z. Hu et al., Citation2019). Regarding risks and costs, a large body of literature argues that agriculture-related loans are riskier and more costly than non-agricultural loans and e-commerce factors (Avkiran & Morita, Citation2010; Dong et al., Citation2012; Huang et al., Citation2006; Lin et al., Citation2019; Zhao et al., Citation2014). Regarding the impact on financial institutions’ operating performance, some literature has argued that agriculture-related loans can enhance the operating performance of financial institutions (Guo & Jia, Citation2009). Regarding the factors influencing agriculture-related loans and e-commerce factors the main ones are government subsidies, urban-rural differences, financial decentralization, GDP growth rate, agricultural output and population size, interest rates and credit construction and bank concentration (Holly Wang, Citation2008; Jin & Turvey, Citation2002; Y. P. Chen et al., Citation2010; Ran et al., Citation2020). In general, the existing literature provides a large number of useful studies on agriculture-related lending. However, there is a lack of research on whether the FTC scale has spillover effects on the proportion of financial institutions’ agricultural loans and what spillover effects exist.
Therefore, this study proposes research hypotheses based on theoretical analysis and tests the research hypotheses based on balanced panel data of 31 provinces and municipalities from 2009 to 2017 to study “whether the scale of FTC stimulates financial institutions to increase agricultural loans and increase investment.” This article’s innovations and contributions are mainly reflected in the following three aspects: First, based on the effect of FTC on the risk-taking level of financial institutions, this article is the first to study the spillover effect of FTCscale on the proportion of agricultural loans. Second, this paper is the first to empirically examine the path through which the marketization level and real estate development affect the share of agriculture-related loans through the partial intermediary effect of the FTC scale. Third, this paper’s findings provide further theoretical support for the policy of “actively implementing the Internet financial services ‘three rural’ projects”.
The rest of the paper is organized as follows: the second part constructs a theoretical model for theoretical analysis and proposes research hypotheses; the third part conducts research design, the fourth part conducts empirical analysis and robustness tests based on 31 provincial and urban areas’ balanced panel data from 2009 to 2017, the fifth part conducts mediating effects analysis, and finally concludes the full paper and proposes policy recommendations.
2. Model construction and research hypothesis
2.1. Basic assumptions and model construction
The FTC will enhances the risk-taking level of banks and other financial institutions (Cui, Citation2020; Li et al., Citation2021; Turuev, Citation2020; Zver’kova, Citation2019). Referring to the extension of Kishan and OPIELA (Citation2012), this paper first introduces the scale of FTC and risk-taking level into the model to construct the theoretical analysis framework. The basic assumptions of the model are as follows.
Hypothesis 1: Representative financial institutions (the financial institutions in this article refer to commercial banks, postal savings banks, rural credit cooperatives, etc., however, commercial banks and postal savings do not have the same power or willingness to invest in FinTech services) that obtain funds and issue loans by absorbing various deposits) aim at maximizing profits and their asset-liability equation is:
. Where, R is deposit reserve, B is government bond, N is non-agricultural loan, A is agricultural loan, D is deposit, and K is capital.
Hypothesis 2: Representative financial institutions only hold statutory reserves and do not hold excess reserves. The statutory reserve ratio is. Therefore, 且
. Learning from the practice of Guo and Shen (Citation2016), the rate of return of the statutory reserve is set to zero. To respond to temporary liquidity needs and maximize profits, representative financial institutions also hold government bonds
with strong liquidity but low yields, with a yield rate of
.
Hypothesis 3: The non-agricultural loan of a representative financial institution increases with the increase of its risk-taking level, but the growth rate decreases at the margin. Let
,
. Where,
is the risk-taking level of representative financial institutions, and
is the non-agricultural loan tendency of representative financial institutions. The interest rate of non-agricultural loans is
, because loan interest rates have been market-oriented. Therefore, representative financial institutions are price takers in the non-agricultural loan market and
.
Hypothesis 4: The agricultural loan of a representative financial institution also increases with the increase of its risk-taking level, and the growth rate is also decreasing marginally. Let
,
. Where,
is the risk-taking level of the representative financial institution, and
is the agricultural loan tendency of the representative financial institution. The interest rate of agriculture-related loans is
. Similarly, representative financial institutions are price takers in the agriculture-related loan market, and
.
Agricultural loans are riskier and more costly than non-agricultural loans (Liu et al., Citation2019; Weber & Musshoff, Citation2012). Hence, representative financial institutions are more willing to invest in non-agricultural loans. Therefore, the propensity for non-agricultural loans is greater than the tendency for agricultural loans, .
Hypothesis 5: The level of risk-taking of a representative financial institution increases with the size of FinTech credit, i.e., . where
is the FTC size
is the initial risk-taking level of the representative financial institution, that is, the risk-taking level when the FTC size is zero;
is the risk-taking propensity, that is, the marginal change in the risk-taking level of the representative financial institution as the FTC size increases. c is the risk-taking propensity, i.e., the marginal change in the representative financial institution’s risk-taking level as the FTC size increases.
Hypothesis 6: Taking a reference from Kopecky and VanHoose (Citation2004), the cost of managing asset-liability items for a representative financial institution is set as a quadratic cost function, which is
Where are constants representing the unit marginal administrative costs of non-agricultural loans, agricultural loans, deposits, and capital, respectively.
is a constant representing the initial fixed cost of the representative financial institution, i.e., the cost that the representative financial institution would have to incur even if it did not do any business.
The marginal management cost per unit for non-agricultural and agricultural loans is the post-loan management cost per unit. There may be differences regarding the post-loan management of non-agricultural and agro-related loans: lenders of non-agricultural loans are usually located in cities with relatively convenient transportation, while lenders of agricultural loans are usually located in cities with relatively convenient locations and may have inconvenient transportation. However, the amount of agricultural loans is small, and representative financial institutions can use remote and spot checks for post-lending management; even if representative financial institutions do not use remote and spot checks for post-lending management of agricultural loans (Chen & Ge, Citation2015; Ge et al., Citation2017; Hsu, Citation2012; Ma et al., Citation2019). It will also include the increased costs due to traffic inconvenience in loans’ interest rate before issuing. In this way, the unit post-loan management costs of agricultural loans and non-agricultural loans are not much different, and unit marginal management costs are almost the same. For this reason, this article assumes that the unit marginal management costs of non-agricultural loans and agricultural loans are similar, which is defined as .
Government bonds do not require post-loan management, so their management costs can be set as a constant .
In addition, the deposit market and capital market have also been market-oriented. Representative financial institutions are also price takers in the deposit market and capital market. The deposit interest rate is denoted as and the cost of capital is denoted as
.
Based on the above assumptions, the objective functions and constraints of the representative financial institutions are as follows:
2.2. Model solution and research hypothesis
Combining Equationequation (2)(2)
(2) , the partial derivative of
on both sides of Equationequation (1)
(1)
(1) can be obtained:
Bringing (2) into (3) so that can be obtained:
Divide both sides of Equationequation (4)(4)
(4) by
to obtain:
Press “(numerator + denominator)/denominator = (numerator + denominator)/denominator” on both sides of Equationequation (5)(5)
(5) , and then take the reciprocal to get:
The right side of Equationequation (6)(6)
(6) is the proportion of agricultural loans, and the partial derivatives of
on both sides of Equationequation (6)
(6)
(6) can be obtained:
Let,
. Then, Equationequation (7)
(7)
(7) can be expressed as:
Since , and
,
, therefore
From Equationequation (9)(9)
(9) , as the scale of FTCincreases, the proportion of agricultural loans increases.
From Equationequation (8)(8)
(8) , the partial derivative of F on both sides of the equation can be obtained:
Since , and
. therefore
From Equationequation (11)(11)
(11) , the growth rate of the proportion of agricultural loans is diminishing marginally.
EquationEquation (10)(10)
(10) take the limit to
to get:
According to (12), the growth rate of the proportion of agricultural loans will eventually converge to zero.
Therefore, by combining (9), (11) and (12), the following research hypotheses can be obtained:
Research Hypothesis H: FTC scale may increase the ratio of agricultural loans, but the growth rate will slow down slightly.
3. Research design
3.1. Sample selection and data sources
This article tests the research hypothesis using the amount of loans issued by FinTech credit (also known as Peer-to-Peer (P2P) online lending). Given that the earliest available FTC loan amounts are from 2009, and that China implemented considerable administrative interventions on the FTC in 2018, it is more reasonable to exclude them from the sample. Therefore, this paper selects 31 provinces and cities from 2009 to 2017 to constitute a balanced panel of FTCloan amounts for empirical analysis. Among them, the amount of P2P online loans is obtained from Zero2IPO Finance-Zero1 Intelligence; the data related to the construction of 31 provincial and urban Internet financial associations and research institutions are obtained from the China Social Organization Public Service Platform and manually collated; other data are obtained from the National Bureau of Statistics, the People’s Bank of China, and Wind database.
In addition, this article interpolates the 2017 marketization process index by Winsorizing the continuous variables with an upper and lower 1% Winsorize tail.
3.2. Variable description
3.2.1. Explained variable
The explained variable in this article is the proportion of agricultural loans. For this reason, the explanatory variable is designed to take the value of “agricultural loan balance/loan balance*100” for each year in 31 provinces and municipalities.
The reasons for choosing the loan balance are as follows: First, the newly issued agricultural loans each year can reflect the loan structure, but this data is not available. Second, the loan balance can also reflect the financial market. Cheng (Citation2009) used loan balance as an explained variable when studying the transmission of China’s monetary policy. Chen et al. (Citation2018) calculated the structural indicator of the banking industry’s concentration based on the loan balance, and this article uses the loan balance as the basis to calculate the structural indicator of the proportion of agricultural loans in the banking industry.
3.3. Key variable
The key variable in this article is the size of FinTech credit. For this reason, the key variable is designed, and the value is the amount of loans issued by P2P platforms each year in 31 provinces and municipalities.
Considering the research hypothesis that FTC size has a marginal decreasing effect on the share of agricultural loans, the square root of it is taken as
.
3.3.1. Control variable
Based on the existing literature, this paper selects urbanization rate, GDP growth rate, agricultural output, total provincial population, and agricultural non-performing loans as control variables.
(1) Urbanization rate (): the value is “urban population/(urban population + rural population)” of each province, municipality.
(2) GDP growth rate (): the value is the GDP growth rate of each province, municipality, and district.
(3) Agricultural output value (): referring to Yin et al. (Citation2020), the value is the natural logarithm of the agricultural output value of each province, municipality, and municipality, that is
, where
is the agricultural output value of each province.
(4) Provincial total population (): the value is the total population of each province, urban area.
(5) Agriculture-related non-performing loan ratio Due to the lack of provincial and municipal data, the value is taken as the “non-performing loan ratio of agriculture, forestry, animal husbandry fishery” for each year. This article will test it for robustness.
In addition to the above control variables, this article also considers the following control variables:
(6) Real estate sales and its quadratic term
; (7) Marketization level
and its quadratic term
; (8) Special rectification
taken from 2011 to 2015 0, take 1 in 2016 and 2017, and take its interaction term with time
; (9) Government encouragement
, take 1 from 2014 to 2015, take 0 for the rest of the year, and take its interaction term with time
for the same reason. (10) Value-added of the financial industry
. (11) The credit gap
. (12) Mobile phone penetration rate
and its quadratic term
. (13) The number of Internet users
and its quadratic term
. (14) Education level
. (15) The construction of self-regulatory organizations
. (16) Fiscal policy
. (17) Monetary policy
and its interaction terms with fiscal policy
. (18) Time
, the value is “year-2008.” (Limited to space, reasons for choosing control variables are available on request)
3.4. Model design
To test the research hypothesis H, the following individual fixed effects model was designed.
Where, denotes the share of agriculture-related loans in year
in the i-th province and city,
is the intercept term,
is the individual effect in the i-th province and city, and
is the random error term.
is the key variable, and
is the control variable listed in the previous article. In order to prevent endogeneity,
,
and
are lagging by one period, respectively,
,
and
.
4. Result and discussion
4.1. Descriptive statistics
shows the descriptive statistics of the main variables. From descriptive statistics: First, the average value of agriculture-related loans is 28.9727%, and the maximum value is 46.3149%, while the minimum value is only 2.6312%, which is consistent with the basic situation of unbalanced development in China. The average value of FinTech’s credit size is 0.2098 billion yuan, and the maximum value is 580.019 billion yuan. This is consistent with the basic situation of uneven development in China at the inter-provincial level, while the time dimension indicates that the scale of FTC is increasing faster. Third, the average value of agricultural output value is 170.48 billion yuan. The minimum value is only 0.0745 billion yuan. The maximum value is 483.27 billion yuan, which is also consistent with the basic situation of uneven development years and 0 for the rest of the year. Fifth, the time variable’s minimum value is 1, and the maximum value is 9 because the sample period is nine years.
Table 1. Descriptive statistics of main variables
4.2. Regression result
Individual fixed effects models can alleviate the endogeneity problem caused by omitted variables. For this reason, the individual fixed effects model FE is used for estimation in this paper. Using a gradual increase in control variables, the results of the FE-based estimation model (a) are shown in : , column (1) shows the estimation results without controlling for fiscal policy and monetary policy, column (2) shows the estimation results after adding fiscal policy, column (3) shows the estimation results after adding further monetary policy, and column (4) is the estimated result after additional fiscal policy, and monetary policy interaction terms are added.
Table 2. FE regression results of model (a)
4.3. Key variables
From columns (1) to (4) of , the coefficients of the key variable are all significantly positive at the 5% significance level, that is, the share of agriculture-related loans
increases with the increase of FTC size, but the growth rate slows down with the increase of FTC size, and eventually no longer increases. Therefore, the research hypothesis
is valid.
4.4. Control variable
From column (4) of : First, the coefficient of urbanization rate is negative, probably because as the urban population increases, the rural population decreases and the effective demand for agricultural loans decreases, but it is not significant. Second, the coefficient of agricultural output value
is significantly positive at 1% significance level, probably because the increase in agricultural output value, on the one hand, increases the demand for agriculture-related loans, on the other hand, the increase in output value also indicates that agricultural development prospects are better and less risky, so that financial institutions have increased their enthusiasm to invest in agriculture-related loans. Third, real estate sales
is significantly negative at the 1% significance level. Simultaneously, the second term is not significant, indicating that real estate sales have reduced the proportion of agricultural loans, possibly due to the crowding-out effect of real estate development on agriculture-related loans. Fourth, the marketization level
is significantly positive at 1% significance level, and the quadratic term is significantly negative at 1% significance level, i.e., there is an inverted “U” type relationship between the marketization level and the share of farm-related loans. The relationship between the level of marketization and farm-related loans will be studied in a separate paper. Fifth, the interaction terms
between special regulation and government encouragement and time are significantly negative at the 1% significance level, i.e., both regulation and encouragement reduce the share of agriculture-related loans, probably because special regulation suppresses the scale of FinTech credit; while encouragement leads to “deposit” moving, also to some extent. The reason for this is that the special remediation has suppressed the scale of FinTech credit, while the encouragement has led to “deposit moving,” which has also suppressed the share of agriculture-related loans to some extent. Sixth, the interaction term it
of fiscal policy and monetary policy is significantly negative at 1% significance level, indicating that both fiscal policy and monetary policy have a negative impact on the share of agriculture-related loans. The possible reason is that the active fiscal policy and loose monetary policy stimulate the development of the secondary and tertiary industries, and financial institutions increase the credit allocation to the secondary and tertiary industries, thus reducing the share of agriculture-related loans as a result, the proportion of agriculture-related loans was reduced.
4.5. Robustness test
Double clustering adjustment of standard errors both individually and temporally can overcome the effects of problems, such as autocorrelation and heteroskedasticity on statistical inference [23]. Columns (1) to (4) all use double clustered standard errors to increase the estimation results’ reliability. Also, the key variables in columns (1) to (4) of are all significant at the 5% significance level, which is itself a robustness test. Here, further robustness tests are conducted by adding control variables, considering event shocks, and using dynamic panels.
After increasing the control variables, considering the impact of events, and adopting dynamic panels, the key variables’ coefficients are significantly positive. Therefore, model (a) is robust.
5. Mediating effect analysis
This section conducts mediating effects analysis and seeks to further investigate the relevant impact mechanisms.
5.1. Can the level of marketization affect the share of agriculture-related loans through the scale of FinTech credit?
Column (4) of shows an inverted U-shaped relationship between the level of marketization and the share of loans related to agriculture, and the level of marketization can directly affect the percentage of loans related to agriculture. On the other hand, the level of marketization affects total factor productivity and enterprise efficiency. The size of FTC may be affected by both total factor productivity and enterprise efficiency. Thus, the marketization level may also affect FinTech credit’s size and the proportion of agriculture-related loans. So, is there such a path of influence? Here, drawing on the test procedure proposed by Huang and Pan (Citation2015) and J. Hu et al. (Citation2013), the following model is set up to test it.
In the preceding model, is the mediating variable:
Without the inclusion of mediating variables, model Path A estimation is performed. Suppose the coefficient
or
of marketization level
or its quadratic term
is significant. In that case, it indicates that the marketization level has an aggregate effect on the share of agriculture-related loans, and the subsequent analysis continues, otherwise terminate.
The regression of model Path B is conducted to determine the effect of marketization on FTC size.
The model Path C is estimated after adding the mediating variables, if
or
in Path B and
or
in Path C are significant, the mediating effect exists. If
in Path C is substantial, then
plays a partial mediating effect, and if
is not marked, then
plays a full mediating effect.
If at least one of
or
in Path B and
or
in Path C is significant, the mediating effect is yet to be tested by Sobel’s test.
shows the test results for the mediating effect when the mediated factor is the market level. In Path A and its quadratic terms are significant at the 1% significance level, indicating the existence of a total effect; the quadratic term of
in Path B is significant at the 10% significance level,
and its quadratic term in Path C are significant at the 1% significance level, indicating the existence of a partial mediating effect;
in Path C is significant at the 5% significance level, indicating the existence of a partial mediating effect. In Path C
is significant at the 5% significance level, indicating that
plays a partial mediating role.
Table 3. Test results of the mediation effect of the currency marketization level as the mediation factor
Therefore, the level of marketization also affects the share of agriculture-related loans through the transmission of FTC size. In addition, the regression results of Path B show that there is an inverted U-shaped relationship between the level of marketization and the size of FinTech credit.
5.2. Can real estate development affect the proportion of agriculture-related loans through the scale of FinTech credit?
Column (4) of shows that there is a negative relationship between real estate sales and the proportion of agriculture-related loans. On the other hand, FTC funds may flow into real estate and promote real estate development, which in turn further stimulates the development of FTCscale, thus affecting the risk-taking level of financial institutions and ultimately the share of agriculture-related loans. So, do real estate sales affect the proportion of agriculture-related loans through the scale of FinTech credit?
The mediating effects were tested by comparing the level of marketization with as the mediated factor. The results are shown in .
Table 4. Test results of the intermediary effect of real estate sales by the intermediary factor
From , in Path A are significant at the 5% significance level, indicating a total effect; In Path B
are positive at the 10% significance level and in Path C
are significant at the 1% significance level, indicating a mediating effect; In Path C
is significant at the 5% significance level, indicating a partial mediating effect of .
Therefore, real estate sales also affect the proportion of agriculture-related loans through FTC size transmission. Also, Path B’s regression results show that real estate sales contribute to the growth of FTC size.
In summary, the marketization level and real estate development directly affect the proportion of agriculture-related loans and indirectly affect the proportion of agriculture-related loans through part of the intermediary effect of the FTC scale.
6. Conclusions
FTC is an important FinTech innovation, as the level of risk-taking of banks and other financial institutions will likely change the structure of farm-related loans and non-farm loans, given that farm-related loans are riskier than non-farm loans. Can the development of FTC change the structure of farm-related loans and non-farm loans? Can FTC scale stimulate banks and other financial institutions to increase the proportion of farm-related loans? Studying these questions is of great theoretical value and practical significance to promote rural revitalization and alleviate the “three rural areas” problems.
This paper extends the model of Opiela by introducing FTC scale and risk-taking level into the model of Opiela. Constructing objective functions and constraints for representative financial institutions, conducting theoretical analysis, and proposing research hypotheses. Then, based on the amount of FTC loans in 31 provinces and municipalities in China from 2009 to 2017, an individual fixed-effects model was used to study the relationship between FTCscale and the share of agriculture-related loans. The results of the study show that the scale of FTC can increase the proportion of agriculture-related loans, and the larger the amount of FTC loans, the higher the proportion of agriculture-related loans; however, as the amount of FTC loans grows, the rate of increasing the proportion of agriculture-related loans tends to diminish. The findings of the study is useful for the financial policy-makers in the credit management and other relevant financial departments.
7. Recommendation for policymakers and future research direction
First, given that the scale of FTC is conducive to increasing the proportion of agriculture-related loans, it is recommended that relevant authorities continue to encourage the development of new FinTech business models, such as FTC, to stimulate banks and other financial institutions to increase the proportion of agriculture-related loans. Second, in view of the diminishing marginal effect of the scale of FTCto increase the share of agriculture-related loans and the higher cost of agriculture-related loans, it is recommended that the relevant departments consolidate the foundation of FinTech innovation (e.g., increase the construction of rural big data platforms) so that financial institutions can reduce the pre-lending and in-lending costs of agriculture-related loans using technology. Third, given that marketization and real estate development also indirectly affect the share of agriculture-related loans through FTC-scale transmission. Therefore, in addition to continuing to encourage the development of FinTech credit, the proportion of agriculture-related loans can also be adjusted indirectly through the construction of marketization and real estate regulation. Hence, it is suggested that the relevant departments should consider it when formulating policies. However, if the FTC scale rises, it may also affect deposit and loan interest rates, thus changing banks and other financial institutions’ credit allocation behavior. Therefore, it is a future research direction to consider the impact of FTCscale on deposit and loan interest rates and then change the proportion of loans related to agriculture.
Correction
This article has been republished with minor changes. These changes do not impact the academic content of the article.
Disclosure statement
No potential conflict of interest was reported by the author(s).
Additional information
Funding
References
- Anshari, M., Almunawar, M. N., Masri, M., & Hamdan, M. (2019). Digital marketplace and FinTech to support agriculture sustainability. Energy Procedia, 156(9), 234–16. https://doi.org/10.1016/j.egypro.2018.11.134
- Avkiran, N. K., & Morita, H. (2010). Benchmarking firm performance from a multiple-stakeholder perspective with an application to Chinese banking. Omega, 38(6), 501–508. https://doi.org/10.1016/j.omega.2009.12.007
- Chen, L. (2016). From fintech to finlife: The case of fintech development in China. China Economic Journal, 9(3), 225–239. https://doi.org/10.1080/17538963.2016.1215057
- Chen, F.-W., Feng, Y., & Wang, W. (2018). Impacts of financial inclusion on non-performing loans of commercial banks: Evidence from China. Sustainability, 10(9), 3084. https://doi.org/10.3390/su10093084
- Chen, Y., & Ge, Y. (2015). Spatial point pattern analysis on the Villages in China’s poverty-stricken Areas. Procedia Environmental Sciences, 271, 98–105. https://doi.org/10.1016/j.proenv.2015.07.098
- Chen, Y. P., Liu, M., & Zhang, Q. (2010). Development of financial intermediation and the dynamics of urban–rural disparity in China, 1978–1998. Regional Studies, 44(9), 1171–1187. https://doi.org/10.1080/00343400903365052
- Cheng, Y. (2009). Reforms of the agricultural Bank of China. The Chinese Economy, 42(5), 79–97. https://doi.org/10.2753/ces1097-1475420505
- Cui, W. (2020). Research on the Green Finance Development Model of Commercial Banks Based on FinTech. Finance and Market, 5(4), 260. https://doi.org/10.18686/fm.v5i4.2608
- Dong, F., Lu, J., & Featherstone, A. M. (2012). Effects of credit constraints on household productivity in rural China. Agricultural Finance Review, 72(3), 402–415. https://doi.org/10.1108/00021461211277259
- Ge, Y., Yuan, Y., Hu, S., Ren, Z., & Wu, Y. (2017). Space–time variability analysis of poverty alleviation performance in China’s poverty-stricken areas. Spatial Statistics, 21(1), 460–474. https://doi.org/10.1016/j.spasta.2017.02.010
- Guo, P., & Jia, X. (2009). The structure and reform of rural finance in China. China Agricultural Economic Review, 1(2), 212–226. https://doi.org/10.1108/17561370910927444
- Guo, P., & Shen, Y. (2016). The impact of Internet finance on commercial banks’ risk taking: Evidence from China. China Finance and Economic Review, 4(1), 1-18. https://doi.org/10.1186/s40589-016-0039-6
- He, G., & Ong, L. H. (2014). Chinese rural cooperative finance in the era of post-commercialized rural credit cooperatives. The Chinese Economy, 47(4), 81–98. https://doi.org/10.2753/ces1097-1475470404
- Holly Wang, H. (2008). Economic value of tradable farmland uses rights and mortgage loans in China. Agricultural Finance Review, 68(2), 289–300. https://doi.org/10.1108/00214660880001231
- Hsu, S. (2012). Role of informal finance in China’s continuing economic development. The Chinese Economy, 45(1), 28–45. https://doi.org/10.2753/ces1097-1475450102
- Hu, Z., Ding, S., Li, S., Chen, L., & Yang, S. (2019). Adoption intention of fintech services for bank users: An empirical examination with an extended technology acceptance model. Symmetry, 11(3), 340. https://doi.org/10.3390/sym11030340
- Hu, J., Kim, J.-B., & Zhang, W. (2013). Insider Trading and stock price crash risk: International evidence from a natural experiment. SSRN Electronic Journal , 21, 1 - 69. https://doi.org/10.2139/ssrn.2359069
- Huang, Y.-T., & Pan, W.-C. (2015). Hypothesis test of mediation effect in causal mediation model with high-dimensional continuous mediators. Biometrics, 72(2), 402–413. https://doi.org/10.1111/biom.12421
- Huang, J., Rozelle, S., & Wang, H. (2006). Fostering or stripping rural China: Modernizing agriculture and rural to urban capital flows. The Developing Economies, 44(1), 1–26. https://doi.org/10.1111/j.1746-1049.2006.00001.x
- Jin, Y., & Turvey, C. G. (2002). Hedging financial and business risks in agriculture with commodity‐linked loans. Agricultural Finance Review, 62(1), 41–57. https://doi.org/10.1108/00214870280001128
- Kishan, R. P., & OPIELA, T. P. (2012). Monetary policy, bank lending, and the risk-pricing Channel. Journal of Money, Credit and Banking, 44(4), 573–602. https://doi.org/10.1111/j.1538-4616.2012.00502.x
- Kopecky, K. J., & Van Hoose, D. (2004). A model of the monetary sector with and without binding capital requirements. Journal of Banking & Finance, 28(3), 633–646. https://doi.org/10.1016/S0378-4266(03)00038-4
- Li, Y., Stasinakis, C., & Yeo, W. M. (2021). Fintech and banking efficiency: Evidence from Chinese Commercial Banks. SSRN Electronic Journal, 2(15), 1-43. https://doi.org/10.2139/ssrn.3782616
- Li, W., & Wu, P. (2018). Rural financial development, agricultural fiscal investment and rural residents income. In DEStech transactions on economics, business and management. icems. https://doi.org/10.12783/dtem/icems2018/25595
- Lin, L., Wang, W., Gan, C., & Nguyen, Q. T. T. (2019). Credit constraints on farm household welfare in Rural China: Evidence from Fujian Province. Sustainability, 11(11), 3221. https://doi.org/10.3390/su11113221
- Liu, T., He, G., & Turvey, C. G. (2019). Inclusive finance, farm households entrepreneurship, and inclusive rural transformation in rural poverty-stricken Areas in China. Emerging Markets Finance and Trade, 55(9), 1–30. https://doi.org/10.1080/1540496x.2019.1694506
- Ma, X., Wang, J., Zhao, L., & Han, J. (2019). The effects of social capital on farmers’ wellbeing in China’s undeveloped poverty-stricken areas. China Agricultural Economic Review, 12(1), 108–121. https://doi.org/10.1108/caer-06-2016-0087
- Najaf, K., Schinckus, C., & Yoong, L. C. (2020). VaR and market value of fintech companies: An analysis and evidence from global data. Managerial Finance.
- Ran, M., Chen, L., & Li, W. (2020). Financial deepening, spatial spillover, and Urban–Rural income disparity: Evidence from China. Sustainability, 12(4), 1450. https://doi.org/10.3390/su12041450
- Roh, T., Yang, Y. S., Xiao, S., & Park, B. I. (2022). What makes consumers trust and adopt fintech? An empirical investigation in China. Electronic Commerce Research, 10, 1–33. https://link.springer.com/article/10.1007/s10660-022-09578-0
- Schinckus, C. (2020). The good, the bad and the ugly: An overview of the sustainability of blockchain technology. Energy Research & Social Science, 69, 101614. https://doi.org/10.1016/j.erss.2020.101614
- Schinckus, C. (2021). Proof-of-work based blockchain technology and Anthropocene: An undermined situation? Renewable and Sustainable Energy Reviews, 152(6), 111682. https://doi.org/10.1016/j.rser.2021.111682
- Turuev, I. B. (2020). FinTech as a new dimension for financial institutions: Problems and solutions. Finance and Credit, 26(9), 2049–2076. https://doi.org/10.24891/fc.26.9.2049
- Weber, R., & Musshoff, O. (2012). Is agricultural microcredit really more risky? Evidence from Tanzania. Agricultural Finance Review, 72(3), 416–435. https://doi.org/10.1108/00021461211277268
- Yin, Z., Meng, L., & Sha, Y. (2020). Determinants of agriculture-related loan default: Evidence from China. BuletinEkonomiMoneter Dan Perbankan, 23, 129–150. https://doi.org/10.21098/bemp.v23i0.1160
- Yin, Z., Qiu, M., & Gan, L. (2019). Information contents of collateral under heterogeneous borrower qualities on the bank loans market in China. China Economic Review, 57(5), 101326. https://doi.org/10.1016/j.chieco.2019.101326
- Zhang, A., Wang, S., Liu, B., & Liu, P. (2020). How fintech impacts pre‐ and post‐loan risk in Chinese commercial banks. International Journal of Finance & Economics, 25(4), 497-683. https://doi.org/10.1002/ijfe.2284
- Zhang, T., & Zhuang, Y. (2020). Research on the impact of fintech event on Chinese commercial banks’ stock price. International Journal of Wireless and Mobile Computing, 18(3), 289. https://doi.org/10.1504/ijwmc.2020.106781
- Zhao, J., Zhang, J., & Barry, P. J. (2014). Do formal credit constraints affect the rural household consumption in China? Agricultural Economics (ZemědělskáEkonomika), 60(No. 10), 458–468. https://doi.org/10.17221/161/2013-agricecon
- Zver’kova, T. N. (2019). FinTech and banks: A revolution that never happened. Finance and Credit, 25(7), 1501–1513. https://doi.org/10.24891/fc.25.7.1501