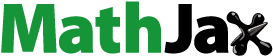
Abstract
The main objective of this paper is to investigate determinants of non-performing loans in the Middle East and North Africa region by exploring the role of bank-specific and macroeconomic factors, particularly in the period of the global financial crisis, as well as the COVID-19 pandemic, as a health crisis that translates to an economic crisis. This study includes 74 banks belonging to 11 MENA countries over the period 2005–2020 and uses the two-stage system generalized method of moment estimator. To conduct a comparative analysis, the whole sample is divided into two sub-samples. The first one is related to the Middle East countries and the second one covers North African countries. The empirical findings indicate that the level of non-performing loans is more sensitive to bank specifics than macroeconomic factors. When it comes to macroeconomic factors, macroeconomic environment and institutional quality significantly affect the level of NPLs. However, no significant effect has been detected regarding the impact of the COVID-19 pandemic.
1. Introduction
It is a well-known fact that banking institutions are essential to the economic growth of a country as they help in the easy flow of credits, which leads to investment opportunities in the productive sectors. Therefore, the soundness of banking institutions is an important factor of the stability of the overall financial system. The efficient and effective performance of the banking industry over time guarantees the financial stability of any nation (Gnawali, Citation2018), particularly in the countries whose financial systems are the backbones of the economies.
As far as the Middle East and North Africa (MENA henceforth) region countriesFootnote1 are concerned, the banking sector is one of the major sources of financing. Banks play a vital role in the growth and stability of the financial system. The collapse of the banking sector is to a large extent driven by credit risk, which is expressed by the degree of non-performing loan (NPL henceforth) rate. NPLs are defined as loans that have not received payments for at least three months (International Monetary Fund, Citation2015). The case of NPLs is widely spread and its extraction is one of the most important concerns when looking to avoid bank failure and economic stagnancy. Regarding the MENA countries, the level of NPLs exceeds the worlds average. It does not only affect banks profitability and solvency; the financial stability and overall political stability of the whole region might be affected.
NPLs are considered to be a “financial pollution” (Zeng, Citation2012), as well as an important determinant of banking instability. According to the International Monetary Fund (Citation2015), NPLs are seen as a drag on economic activity, especially in the countries where banks are the key players in financial intermediation. The harmful effects of NPLs have prompted many researchers to study them from different viewpoints. Many studies have investigated determinants of NPLs in different countries and regions (Bayar, Citation2019; Boudriga et al., Citation2010; Jabbouri & Naili, Citation2019; Memdani, Citation2017; Rachid, Citation2019).
In the MENA region, banks still constitute the prime part in providing financial services. The economy of the MENA region is characterized by being a bank-based economy, where the banking sector dominates the financial system. The banking sector is one of the most closely supervised industries in the MENA region, following the view that banking distress has a greater adverse impact on economic activity than any other industry failure (Sahut & Mili, Citation2011).
The MENA region shares some common characteristics related to language, culture, and geography. However, despite these similarities, the region countries can be categorized into two main groups. The first group are the oil exporters, mainly comprised of the Gulf Cooperation Council (GCC) countries, generally the Middle East countries (World Bank, Citation2015). Another group are mostly non-GCC countries, which are oil importers and generally located in North Africa. We can notice clearly that oil exporting countries are mostly dependent on the oil sector. Their financial systems are more incorporated into global markets; in addition to that, they are more sensitive to oil price fluctuations.
On the contrary, oil importing countries have more diversified economies. They mainly rely on tourism, agriculture, and foreign direct investment. Several factors make the banking sector in the MENA region a compelling laboratory to study this issue, especially nowadays when the COVID-19 pandemic has caused a massive economic turmoil in the region through simultaneous challenges, e.g., a drop in domestic and external demand, low oil prices, disrupted global trade chains, and tightening financial conditions in the region.
The MENA region entered the COVID-19 crisis with chronic low growth, macroeconomic imbalances (Arezki et al., Citation2019). The expenditure decline was smaller than the revenue fall-off, which reflects pressing demands to spend on policy responses to the pandemic. The MENA countries had to borrow to finance the deficits. The pandemic was estimated to increase the regions public debt to about 54% of GDP in 2020 (World Bank, Citation2021), accelerating the rise in public debt during the past decade.
Despite significant efforts made by the MENA countries to improve their banking sectors and risk management instruments over the last few decades, the region incurred an NPL-to-total-gross-loan ratio of 4.6%, which was higher than the worlds average of 4.1% and lower than South Asias 7.8% in 2014 (Sharma, Citation2016). However, the growth in the MENA countries is projected to be faster in 2022–2023 than an average 2010–2019, reflecting a growth acceleration relative to a distribution from the pandemic and oil production (World Bank, Citation2022).
The main objective of this study is to empirically investigate the fundamental determinants of NPLs in the MENA region over the 2005–2020 period by taking into consideration several factors. Apart from exploring the impact of the essential factors, we strive to determine whether the recent COVID-19 pandemic has significantly affected the level of NPLs in this region.
To the best of our knowledge, no study has yet included bank specifics, industry specifics, macroeconomic factors, global financial crisis, as well as a health crisis, in terms of the COVID-19 pandemic, to explain the changes in the level of NPLs in the MENA region. Thus, this study aims to fill this literature gap and contribute to the existing literature. Due to several economic, financial, and social differences among the MENA countries, the whole sample is divided into the Middle East countries and North African countries. To provide robust and more efficient results and solve the problem of endogeneity, the SGMM method has been chosen as the most appropriate method.
In other words, what is evident when reviewing the literature, there has been no study yet that simultaneously includes bank-specifics, industry specifics, macroeconomic factors, global financial crisis and the COVID-19 pandemic to explain the changes in the level of NPLs in the MENA region. This study, therefore, comes to extend the existing literature on the determinants of NPLs.
The remainder of this paper is structured as follows: Section 2 contains a literature review, Section 3 presents the data and empirical methods, Section 4 covers the empirical findings, Section 5 is a robustness check of the effect of the COVID-19 pandemic, while Section 6 provides conclusions and addresses some related policy implications.
2. Literature review
There is an extensive literature related to the determinants of NPLs. A substantial growth of NPLs in the context of the global financial crisis and the repercussions of the COVID-19 pandemic on the economy raised scholars and researchers
awareness of the need to investigate the determinants of the quality of bank loans. However, after the global financial crisis, tighter capital and liquidity requirements have been placed on banks with Basel III, which aims to help the global banking system to improve its safety during bad times. As a result of Basel III regulations, banks are better positioned to absorb the severe impact of the COVID-19 pandemic.
Although there is a wide range of the factors/variables influencing NPLs (e.g., Louzis et al., Citation2012; Beck et al., Citation2015; Dinçer et al., Citation2018; Kartikasary et al., Citation2020; Muhović & Subić, Citation2019; Radivojevic & Jovovic, Citation2017; Shkodra & Ismajli, Citation2017; Škarica, Citation2014; Tanasković & Jandrić, Citation2015) they are usually classified based on bank-specific factors, macroeconomic factors, and institutional quality.
2.1. Bank specifics and NPLs
Literature on the determinants of NPLs has identified several bank specifics with mixed results. The level of profitability, the bank size, the bank capital and, income diversification are strongly studied as the main determinants of NPLs. For example, it was reported that there is a negative association between the level of profitability and the level of risk measured by the ratio of NPLs. Godlewski (Citation2005) concluded that the higher ratio of profitability is associated with the lower ratio of NPLs and vice versa. Similarly, Makri et al. (Citation2014) found a negative relationship between bank profitability and NPLs. Banks with high income are less likely to adopt speculative behavior and risk-taking. Additionally, they are less involved in risky investments (Berger & DeYoung, Citation1997). The negative association between bank profitability and NPLs was also confirmed by the work of Hue (Citation2015). The author concludes that banks with a higher ratio of ROA are faced to a lower ratio of NPLs.
While there is unanimity regarding the negative relationship between bank profitability and NPLs, the association between bank capital and NPLs remains inconclusive. From the one side, a positive association between the high ratio of capital and the high level of NPLs can be explained as follows. Having a higher capital adequacy ratio, a bad loan decision without sufficient guarantees is usually finished by a loss of the principal and the interest income which increases the level of NPLs (Rajan, Citation1994). From the other side, more capitalized banks experienced lower levels of NPLs. This relationship can be explained by the high expertise of these banks in hedging and managing credit risks (Keeton, Citation1999).
Literature on the association between bank diversification and credit risk (NPLs) also provides mixed results. The first part of the literature supported the negative relationship and concluded that more diversified banking activities reduce bank risks and increase bank profitability (Brahmana et al., Citation2018; Sharma & Anand, Citation2018). The second part of the literature argued that bank diversification measured by non-interest income leads to more bank risks and lowers bank profitability (eg., Githaiga, Citation2020; Hai Trung, Citation2021). These authors conclude that non-traditional banking activities are associated with a problem of information asymmetry that leads to higher agency costs and erodes bank decision-making.
One of the studies from the relevant literature on the MENA region is of Boudriga et al. (Citation2010). The authors examined bank-specifics and institutional environment as determinants of NPLs. The authors used 46 banks located in 12 MENA countries during the period 2002–2006. Their findings demonstrate that NPLs are not only impacted by bank specific factors but also that the business and institutional environment can threaten the stability of the banking industry and the financial system.
2.2. Macroeconomic factors and NPLs
Besides bank specifics and industry specifics, the macroeconomic environment was strongly explored as an important factor that determines the level of NPLs. In this regard, De Bock and Demyanets (Citation2012) explore the determinants of NPLs in emerging countries from 1996 to 2010 using panel data regression. They reveal that economic growth has a negative impact on NPLs while exchange rate and credit growth have a positive impact on NPLs. Their models point out that there is a significant link between macroeconomic aggregates and the financial system.
Louzis et al. (Citation2012) studied both macroeconomic and bank specific determinants of NPLs in Greece for different categories of loans (consumer loans, business loans, and mortgage loans) over 2003Q1-2009Q3 by applying dynamic panel regression analysis. They find that economic growth is negatively associated with NPLs while unemployment, lending interest rate, public debt, and bad management positively affect NPL growth.
Further, Alnabulsi and Kozarević (Citation2021) investigate the interdependence between NPLs, financial stability, and economic growth in the Jordanian banking sector from 2006 to 2019. The authors found a negative and significant relationship between GDP growth rate and NPL ratio. Additionally, the regression analysis of NPLs relationship with financial stability and economic growth in the Jordan case study reveals that 60.4% of the variability in the NPL rate can be explained by the influence of selected indicators of economic growth and financial stability (e.g., GDP growth rate, unemployment rate, inflation rate, capital adequacy ratio, etc.). Swamy (Citation2012) examines macroeconomic determinants of NPLs in India by using panel regression analysis during the period 1997–2009. The author finds that better credit risk management practices need to be taken into consideration for bank lending activities, especially to those banks that have low operating efficiency and low capitalization.
In the Baltic countries, Citation2017 use NPLs as a measure of loan portfolio quality. They investigate macroeconomic and bank-specific factors of NPLs through unbalanced panel regression for 27 banks during the 2005–2014 period. It is found that unemployment, one lagged value of domestic credit to the private sector, one lagged value of NPLs, growth of gross loans, and the global financial crisis have a positive impact on NPLs, while economic growth, one lagged value of equity to total assets, return on assets, and return on equity have a negative impact on NPLs.
Furthermore, Klein (Citation2013) investigates the determinants of NPLs in 16 Central, Eastern, and South-Eastern European countries by using panel data regression over the period 1998–2011. The author finds that NPLs tend to increase when unemployment rises, the exchange rate depreciates, and inflation is high. Also, country-specific factors such as the euro area GDP growth and the global risk aversion have a direct impact on banks asset quality. Klein finds, however, that NPLs are sensitive to bank-specific factors such as equity to total assets and loan growth rate. These bank-specific factors are significant in the pre-crisis and post-crisis periods.
In South-Eastern Europe countries, Ćurak et al. (Citation2013) investigated determinants of NPLs in 69 banks from 2003 to 2010 by using dynamic panel regression. They find that lower economic growth, higher inflation, and higher interest rate are associated with higher NPLs in addition to bank-specific factors used in the study, for example, bank size, return on assets, and solvency. To investigate macroeconomic determinants of NPLs in 28 EU countries from 2000 to 2013, Roman and Bilan (Citation2015) conduct panel regression and learn that macroeconomic factors have a strong and critical influence on the quality of assets. Additionally, the authors find that there is a significant increase of bank loans to the private sector expressed as a share of GDP. In that regard, public finance may trigger the quality of bank loans once government debt breaks a certain threshold (about 97% of GDP).
2.3. Institutional quality and NPLs
While bank specifics and macroeconomic conditions are strongly explored in the NPLs literature, less abundant studies are focused on the institutional environment as a determinant of NPLs. Recently; Yilmaz (Citation2019) explores macroeconomic, institutional, and bank-specific factors behind NPLs in emerging market economies over the period 2000–2013 by employing dynamic panel regression. The findings of the study reveal that not only bank-specific factors but also macroeconomic and institutional factors are significant for an efficient and sound banking sector. Therefore, regulators and policymakers should improve the institutional context and stabilize the economic environment for the efficient functioning of the banking sector.
From an economic cycle perspective, state-owned banks need to strengthen adequate measures to sharpen their NPL management. Ahmad (Citation2013) examines institutional determinants of corruption and information sharing and their impact on NPLs in Pakistan from 2001 to 2010, using ordinary least squares (OLS). He finds that corruption has a positive impact on NPLs while economic growth and lending interest rate have a negative impact on NPLs.
Using a sample of 46 banks of 12 MENA countries during the period 2002–2006, Boudriga et al. (Citation2010) examined the determinants of NPLs in the MENA region. The authors reveal that rule of law has a negative relationship with the level of the NPL ratio. A higher level of rule of law is associated with a lower level of NPLs, and vice versa.
3. Sample and empirical approach
3.1. The sample
To investigate determinants of NPLs in the MENA region in a comprehensive manner, we use a sample of MENA banks over the period 2005–2020. The initial sample covers 123 banks. However, several banks are excluded due to the issue of availability and continuity for some variables, especially NPLs. For that reason, the final sample is made of 74 banks. These banks are located in 11 countries of the MENA region. This sample covers only conventional (i.e., non-Islamic) banks. The number of banks by country is given in Appendix 1.
Due to several economic, financial, and regulation differences, the sample is divided into two sub-samples. The first one is related to the Middle East countries and covers 56 banks while the second one is related to North African countries and covers 18 banks.
According to Shekhar et al. (Citation2015), an elevated level of NPLs has an impact on impairing bank balance sheets, depressing credit growth, and delaying output recovery. The level of NPLs is determined by bank-specific factors, industry specifics, macroeconomic factors, and the global financial crisis. As bank-specific factors, we use return on assets (ROA), return on equity (ROE), bank size (BS), capital adequacy ratio (CAR), loan loss provision (LLP), non-interest income (NII), and loan to deposit ratio (LTD). Variables that reflect industry-specifics are relative to the level of concentration (CONC) and competition (LERN) while macroeconomic factors are growth rate of gross domestic product (GDPG), inflation rate (INF), and unemployment rate (UNEM).
As it has already been mentioned, some studies have addressed the linkage between institutional quality and NPLs. For example, Bayar (Citation2019) examines macroeconomic, institutional, and bank-specific factors of NPLs in emerging market economies. Specifically, regarding Central and Eastern Europe and Southeast countries, Tanasković and Jandrić (Citation2015) highlight both macroeconomic and institutional determinants of NPLs. Hence, in this study, we introduce three proxies of institutional quality: (i) control of corruption (CCOR), (ii) political stability (POLIS), and (iii) rule of law (RUL). Unlike previous studies, we extend the existing literature by taking into consideration the effect of the health crisis, i.e., the COVID-19 pandemic. We, therefore, introduce two additional proxies. The first proxy is the number of active cases (CASES) and the second one is the number of fatalities (DEATHS).
The data used in this study are collected from three main sources. Accounting and financial variables are collected from the annual reports of each bank, i.e., Refinitiv Eikon database. Macroeconomic variables are collected from the World Bank databaseFootnote2 [the World Development Indicators (WDI) database 2005–2020], and the information on the COVID-19 pandemic is retrieved from the Worldometer.
3.2. Empirical approach and model specification
The problem of endogeneity is considered as one of the most serious problems in corporate and banking finance modeling. The OLS and the fixed- and random-effect (FE and RE) models always face several problems such as omitted variable bias and measurement errors. To overcome this problem and following Bolarinwa et al. (Citation2021), and Umar and Sun (Citation2018), in this study we use the two-step system generalized method of moment estimator (SGMM henceforth) proposed by Blundell and Bond (Citation1998). This method deals with the problem of endogeneity and provides robust and more efficient results (Danisman & Tarazi, Citation2020; Hakimi et al., Citation2021; Teixeira & Queirós, Citation2016; Zhou et al., Citation2014).
To investigate the main determinants of NPLs in the MENA region, we follow an empirical strategy based on four steps. The first one focuses on the main determinants of NPLs such as bank specifics and industry specifics. The second step considers the main determinants of NPLs and tests the effect of macroeconomic factors and the global financial crisis. The third step checks whether institutional quality can affect the level of NPLs. Hence, we introduce CCOR, POLIS, and RUL. As a robustness check, the fourth step includes the pandemic COVID-19. We introduce in the econometric model two proxies—CASES and DEATHS.
We extend the study of Khan et al. (Citation2020) by including the variables that reflect financial environment, macroeconomic conditions, and the COVID-19 pandemic as a determinant of NPLs. The econometric model is given in equation (1):
Where; is a matrix of macroeconomic environment that covers GDPG, INF, and UNEM.
is a matrix of institutional quality that covers CCOR, POLIS, and RUL.
is a matrix of the COVID-19 variables measured by CASES and DEATHS. All variable definitions and measurements are listed in Table .
Table 1. Definitions and measurements of the variables
3.3. Summary statistics and correlation matrix
The statistics displayed in Table indicate that the average mean of NPLs is 8.10%, with a maximum value of 58.13%, and 0.01% as a minimum value. The two proxies of bank performance recorded on average 1.93% for ROA and 13.66% for ROE.
Table 2. Descriptive statistics
As far as the bank capital is concerned, banks in the MENA region registered an average capital adequacy ratio of 14.98%, with a maximum level of 40.35% and 1.62% as a minimum value. Based on these statistics, we can conclude that banks in the MENA region are on average well-capitalized except for some banks that recorded low capital ratio.
Table also indicates that the mean value of bank size is 9.84, with a minimum value of 5.05 and a maximum value of 18.08. As a proxy of liquidity risk, we can observe that the average level of liquidity risk measured by the loan-to-deposit ratio is 81.92%, with a maximum value of 215.32%.
The highest growth rate is 26.17 % against −21.46 % as the weakest rate. The average value of the inflation rate is 3.84%, with a maximum value of 84.86 % and a minimum value of −4.86%. These statistics point to an unstable macroeconomic environment. The statistics indicate that the average values of the institutional quality are 0.18 for CCOR, −0.17 for POLIS, and 0.22 for RUL. From these statistics, we can conclude that on average the MENA countries have recorded a weak institutional environment.
After having some information about all variables, the following step consists of checking for the multicollinearity issue. The correlation between all independent variables is given in Appendix 2. From the table, we can note that this correlation is very weak. Thus, we confirm that there is no significant problem with multicollinearity.
To double-check the multicollinearity problem, we perform variance inflation factors (VIFs). The results are given in Table . A value of 1 indicates there is no correlation between the variables in the model. A value between 1 and 5 indicates moderate correlation while a value greater than 5 indicates a potentially severe correlation between the variables in the model.
Table 3. Variance inflation factors (VIFs)
From Table , we conclude that all values are between 1 and 5, which informs about moderate correlation and the absence of a potentially severe correlation between the variables in the model.
4. Empirical analysis
4.1. Empirical results for the whole sample
As the sample is divided into two sub-samples, one for the Middle East countries and another for North African countries, the empirical results are discussed as follows. We firstly discuss the empirical findings related to the estimates of the whole sample, which are given in Table . Then we discuss the findings related to the Middle East countries and interpret the results of North African countries.
Table 4. Empirical results of the NPL determinants—whole sample
The diagnostic tests of Sargan and serial correlation tests do not reject the null hypothesis of the validity of over-identifying restrictions and the absence of correlation. Both the p-value of the Sargan test and the p-value of the AR (2) test of Arellano and Bond are greater than 5% (Arellano & Bond, Citation1991). We inform that columns (1) and (2) are relative to the estimation of the model (1). Columns (3) and (4) are relative to the estimation of the model (2) when we include the global financial crisis and the macroeconomic specifics. Columns (5) and (6) are relative to the estimation of the model (3) when we check the effect of the institutional quality.
The results of the model (1) displayed in columns (1) and (2), Table , indicate that the dependent variable (NPLs) is positively and significantly associated with the lagged variable. This means that the level of NPLs in the current year is positively correlated with the level of NPLs in the previous year.
Concerning the effect of bank profitability, we notice that higher banking profitability significantly decreases the level of NPLs. Both ROA and ROE coefficients are negative and the Z-statistics are significant. A 1% increase in bank profitability measured by ROA decreases the NPLs ratio by 4.6%. This means that an increase in banking profitability significantly decreases the level of NPLs. Also, it can be concluded that a higher level of NPLs contributes to lowering the effective management of funds. This result is in line with the findings of Citation2017. Moreover, Athanasoglou et al. (Citation2008) study the Greek banking system and find that there is a negative relationship between credit risk and bank profitability. Consistent with the idea, Berger and DeYoung (Citation1997) argue that highly profitable banks are less likely to become involved in risky activities that can lead to loan defaults in the future. They suggest a negative relationship between bank profitability and NPLs.
Banking literature has argued that large banks are more experienced in risk management and have sufficient provisions to hedge credit risk. In that regard, several empirical studies report that large banks are less exposed to credit risk. The empirical findings indicate that the coefficient of bank size is positive and significant with the dependent variable. This means that large banks record a higher level of NPLs. Some larger banks follow liberal credit policy, thus the chances of NPLs are higher as compared to smaller banks.
Additionally, large banks may face a problem of governance that negatively affects decision-making and deteriorates the quality of loans. This result is in line with the findings of Misra and Dhal (Citation2010), who examine the pro-cyclical management of banks in India. Due to balance sheet constraints, some of small banks in India show greater managerial efficiency than the larger banks. Moreover, Rajha (Citation2016) studies the determinants of NPLs in the Jordanian banking sector and finds a positive relation between NPLs and bank size, explaining that large banks are not necessarily more effective in screening their clients compared to smaller banks.
The findings also indicate that the capital adequacy ratio is negatively and significantly associated with the dependent variable, i.e., NPLs. A 1% increase in bank capital decreases the NPL ratio by 2.9%. It is reported that well-capitalized banks are less exposed to credit risk. A high level of capital adequacy ratio makes banks less prone to engage in optimistic risk-taking. Thus, many banks appear to make vast efforts to perform prudent and wise lending to minimize the likelihood of potential NPLs. This finding corroborates the work of Shrieves and Dhal (Citation1992). For the MENA region, Jabbouri and Naili (Citation2019) also find a negative correlation between capital adequacy ratio and NPLs. An explanation of this result is that many MENA countries increased the minimum capital requirements to limit excessive lending.
The level of NPLs is found to be more sensitive to the level of non-interest income. The coefficient of non-interest income is positive and significant. This means that more diversified bank activities lead to more credit risk measured by the NPL ratio. The explanation of this sign is the potential inverse effect of diversification. Explicitly, credit risk of banks without any competitive advantages increases. This result is consistent with Louzis et al. (Citation2012), Chaibi and Ftiti (Citation2015), and Stiroh (Citation2004), who support a positive relationship between non-interest income and NPLs.
The results of the effect of the global financial crisis and macroeconomic specifics relative to model (2) are given in columns (3) and (4) of Table . Contrary to the expected sign, we reveal that the global financial crisis exerts a negative and significant effect on the level of NPLs. Theoretically and empirically, it has been argued that the global financial crisis reduces bank profitability and stability, and increases bank risks.
In this study, we find that the financial crisis decreases bank NPLs in the MENA region. This result can be explained by several reasons. In the period of crisis, banks become more rigid when approving loans, hence bank credit activity is limited, and consequently bank NPLs decrease. This result is in line with Ozili (Citation2019), who examines the impact of the behavior of NPLs in the European systemic and non-systemic banks. The author reveals that after the financial crisis, NPLs have had a negative and significant relationship with the economic cycle, arguing that those systematic banks have had higher level NPLs in the era of post-crisis.
Concerning the effect of macroeconomic conditions, the empirical findings indicate that an increase in the growth rate of GDP, inflation rate, and unemployment significantly increase the level of NPLs. The effect of inflation and unemployment is expected since an increase in the inflation rate increases financial expenses and reduces the ability of borrowers to pay their loans. This result is consistent with Fofack (Citation2005), who finds a positive relationship confirming that a higher inflation rate leads to higher loan defaults. Jabbouri and Naili (Citation2019) find a positive impact of inflation on NPLs contrary to several studies arguing that an increase in inflation decreases the level of NPLs. Similarly, higher unemployment rate increases the probability of default since borrowers are unable to meet their engagements. Our result is in line with Ćurak et al. (Citation2013), Fofack (Citation2005), and Salas and Saurina (Citation2002), who conclude that the unemployment rate plays a key role in explaining the NPL ratio trajectories.
Theoretically, it has been disclosed that in a stable macroeconomic environment proxied precisely by the economic growth, banks grant more credits to finance investment. Stable macroeconomic conditions enhance the ability of borrowers to pay their credits. However, the findings of the current study support the opposite association. A higher GDP growth rate leads to higher level of credit risk. The results of this paper support a positive relationship between GDP growth and NPLs. This relationship is questionable as higher GDP growth rate will lead to a higher earning capacity of borrowers. This result is consistent with Mondal (Citation2016), who explores the sensitivity of NPLs to macroeconomic variables in the banking sector of Bangladesh. Moreover, Tabak et al. (Citation2007) investigate the Brazilian banking sector and find a positive relation between NPLs and GDP growth. In the same line, Shingjergji (Citation2013) examines the impact of macroeconomic variables on NPLs in Albania over the period 2005–2012 and reveals a positive relationship between GDP growth and NPLs.
Columns (5) and (6), Table , are relative to the results of model (3) when we check the effect of the institutional quality. The findings indicate that the three institutional variables significantly affect the level of NPLs. More precisely, we conclude that more control of corruption and an effective rule of law significantly decrease the NPL ratio. However, more political stability leads to a higher level of NPLs.
The findings of the study indicate that more control of corruption decreases the level of NPLs. This means that countries that adopt a strict strategy to fight corruption limit bank risk-taking and personal relationships aiming to grant credits without sufficient guarantees. This result is in line with Hasan and Ashfaq (Citation2021), who find that the negative impact of corruption on banks asset quality suggests that corruption adversely affects the loan portfolio of the banking sector. Toader et al. (Citation2018) investigates the effect of corruption on banking stability in emerging markets and discover that better corporate governance can increase financial stability, thus reducing NPLs.
Similarly, we conclude that rule of law significantly decreases the level of NPLs. A strong rule of law compels banks to respect the standard of credit distribution. Additionally, in countries with a strong rule of law, there is strong protection of creditors rights, which leads to a decrease in credit risk. This result is consistent with Boudriga et al. (Citation2010), who examine the determinants of NPLs in the MENA region and reveal that rule of law has a negative relationship with the level of the NPL ratio. A higher level of rule of law, a lower level of NPLs, and vice versa.
Contrary to the effect of control of corruption and rule of law, we find that more political stability experiences a higher level of NPLs in the MENA region. This result can be interpreted in a way that in the period of political stability, financial markets and banking sectors are stable according to the theory of behavioral finance. In this case, thinking of more return, banks may grant loans and finance some risky projects that finish by a loss of the principal and the interest. Hence, it results in an increase in NPLs. Therefore, loan decisions are affected by the magnitude of pressure from political lobbying. Loans will gain from the strong political connection but with lower quality. This result is in harmony with Hu et al. (Citation2004), who argue that state-owned banks are more open to political lobbying and the administrative pressure that results in a higher level of NPLs.
4.2. Empirical results for the Middle East countries
Similarly to the results of the whole sample, the results for the Middle East showed in Table indicate that the diagnostic tests of Sargan and serial correlation tests do not reject the null hypothesis of the correct specification. Both the p-value of the Sargan test and the p-value of the AR (2) test of Arellano and Bond are greater than 5%.
Table 5. Empirical result of the NPL determinants—the Middle East countries
The results reveal that the lagged variable is positively and significantly associated with the dependent variable. This means that the NPL level in a year positively and significantly depends on the level of the previous year.
The findings also indicate the level of bank profitability measured by ROA and ROE significantly decreases the NPL ratio. A 1% increase in bank profitability measured by ROE decreases the NPL ratio by 19.3%. More profitable banks experience a lower level of NPLs. Moreover, the high level of NPLs decreases banks profitability. This result is consistent with the work of Godlewski (Citation2005), who uses ROA as a performance indicator in examining the impact of banks profitability in Spain and finds that there is a negative relationship between ROA and NPLs.
As in the case of the whole sample, we conclude that well-capitalized banks in the Middle East experience lower levels of NPLs. A 1% increase in bank capital decreases the NPL ratio by 12.2%. The rationale behind the negative relation is that banks with high capital adequacy ratio are more likely to engage in prudent lending to sustain their capital. This result is in line with Berger and DeYoung (Citation1997), and Keeton and Morris (Citation1987). More generally, the result is well-explained by the moral hazard hypothesis. Less capitalized banks will more likely engage in excessive risk-taking, and vice versa.
In contrast to the findings of the whole sample, the results for the Middle East indicate that more bank activity diversification reduces the level of NPLs. The coefficient of non-interest income is found to be negatively and significantly associated with the NPL level. This means that a higher bank diversification significantly contributes to a lower NPL ratio, which confirms the diversification hypothesis of Hu et al. (Citation2004) that less diversified banks must take additional risk on their loan portfolio to generate more profits. This result is also consistent with the findings of Ghosh (Citation2015).
The results in Table show that more bank competition significantly decreases the level of NPLs. Contrary to the results of the whole sample and North African countries, the findings indicate that a more concentrated banking system experiences a higher level of NPLs while higher bank competition significantly reduces the NPL ratio. This result can be explained by the view that bank concentration may reduce NPLs by enhancing market power and bank profits. Thus, high profits can provide a buffer against shocks. This result is consistent with Beck and Demirguc-Kunt (Citation2006). In the same line of idea, Allen and Gale (Citation2004) analyze the effect of bank concentration on bank stability and conclude that greater bank competition may be good for efficiency but troublesome for financial stability.
As far as the effect of institutional quality is concerned, similarly to the whole sample, we conclude that the three institutional variables significantly affect the level of NPLs in the Middle East countries. More precisely, we find that more control of corruption and an effective rule of law significantly decrease the NPL ratio while more political stability leads to a higher level of NPLs.
4.3. Empirical results for North African countries
The empirical results of the determinants of NPLs in North African countries are showed in Table . Like the results of the whole sample, the Sargan and serial correlation tests do not reject the null hypothesis of the correct specification. Both the p-value of the Sargan test and the p-value of the AR (2) test of Arellano and Bond are greater than 5%.
Table 6. Empirical results of the NPL determinants—North African countries
Contrary to the findings of the whole sample and the Middle East countries, we reveal that the capital adequacy ratio is positively and significantly associated with the level of NPLs. In other words, more capitalized banks are more exposed to bank credit risk. This result can be explained in the following way—more capitalized banks experience a higher level of NPLs. In some cases, well-capitalized banks may adopt risk-taking behavior by granting bad loans with insufficient guarantees. Bad quality of loans, weak governance, and high level of information asymmetry in North African countries increase the level of NPLs.
The results in Table also indicate that a higher concentrated banking system in North African countries lowers the level of NPLs. The competition-fragility view sets that more competition among banks leads to more fragility, as theoretically modeled by Marcus (Citation1984) and Keeley (Citation1990). In a more competitive environment with more pressures on profit, banks have higher incentives to take more risks, resulting in higher fragility. This result is in line with Jiménez et al. (Citation2013), who support the view that those banks in highly competitive environments will take deliberate steps to minimize risk and gain a favorable risk management perception among investors and regulators compared to the rival banks. As a result, countries with a more competitive banking sector should experience a lower level of NPLs.
Similarly to the findings of the whole sample, the results indicate that an increase in the inflation rate and unemployment significantly increase the level of NPLs. A 1% increase in the inflation rate increases the NPL ratio by 18.2%. The effect of inflation and unemployment is expected since an increase in the inflation rate increases financial expenses and reduces the ability of borrowers to pay their loans. Klein (Citation2013) confirms these findings with the empirical evidence in Central, Eastern, and Southeastern Europe (CESEE). Similarly, a higher unemployment rate increases the probability of default since borrowers are unable to meet engagement. Our result is in line with (Louzis et al., Citation2012; Vogiazas & Nikolaidou, Citation2011).
Regarding the effect of institutional quality and contrary to the result of the whole sample and the Middle East, we conclude that the control of corruption does not exert any significant effect on the level of NPLs. This means that at this level of control of corruption and the main strategy implemented to fight corruption are not effective and efficient in reducing the level of NPLs in North African countries. Hence, strong effort is needed to fight and control corruption in this region. We also reveal that an effective rule of law and more political stability significantly decrease the NPL ratio.
5. Robustness check: the effect of the COVID-19 pandemic
As a robustness check, in the fourth step, we include the COVID-19 pandemic as a determinant for NPLs. We introduce two proxies in the econometric model. The first proxy is CASES and the second one is DEATHS. The empirical findings are displayed in Table .
Table 7. Empirical results of the effect of the COVID-19 pandemic
From Table , we note that there is no significant effect regarding the effect of the COVID-19 pandemic on the level of NPLs. Thus, we confirm that the pandemic does not exert any significant effect. The short time of observation of the year 2020 seems to be without a significant effect. It is not significant for the whole sample, the Middle East and North African countries as well.
6. Conclusions and policy recommendations
The purpose of this paper is to investigate the main determinants of NPLs across the MENA region by exploring the role of bank specifics, macroeconomic factors, the global financial crisis, and the effects of the COVID-19 pandemic. The study uses a sample of 74 banks located in 11 MENA countries over the 2005–2020 period, with an application of the two-stage SGMM. For the benefit of comparative analysis, this study divides the MENA region into the Middle East countries and North African countries.
Overall, the empirical findings reveal that the level of bank profitability significantly decreases the level of NPLs for both the Middle East and North African countries. This means that more profitable banks in the region experience a lower level of NPLs. Contrary to the results of North African countries, for which we find that capital adequacy ratio has a positive and significant relationship with the level of NPLs, banks with higher capital adequacy ratio in the Middle East countries show that they are more prudent in lending and, as a result, they have a lower NPL ratio. Moreover, the banks in the Middle East countries show that bank diversification has a negative association with the level of NPLs. The findings also indicate that bank competition in the Middle East countries significantly increases the level of NPLs. Contrary to the results of the whole sample and North Africa, we learn that a more concentrated banking system in the Middle East experiences a higher level of NPLs, and vice versa, higher bank competition in the Middle East significantly reduces the NPL ratio.
As far as the effect of macroeconomic conditions in the region is concerned, we conclude that the relation between economic growth and NPLs has a positive direction. Further, the relationship between unemployment and NPLs is also positive. For the inflation rate, we find that the higher inflation followed by an interest rate floating in the MENA region results in a higher level of NPLs. Moreover, the study reveals significant insights for policymakers, bank managers, as well as financial analysts. In addition, fiscal policies should be directed to create an environment that will boost market activity and enhance economic growth and improve investors confidence through effective valuation.
When it comes to the effect of institutional quality, the study concludes that control of corruption, rule of law, and political stability have a significant effect in the Middle East countries. On the contrary, in North African countries, we find that the control of corruption does not have any significant effect on the NPL ratio. Notwithstanding, rule of law and political stability show a significant impact on the NPL ratio in North Africa.
The results could bring some important policy implications either for policymakers or for banks. First, to control the level of NPLs, more attention should be granted to the factors such as bank specifics, macroeconomic factors, and institutional quality. To manage credit risk, the banks in the MENA countries and the two groups of countries need to be more profitable and well-capitalized. Secondly, governments of these countries need to work strongly to guarantee a stable macroeconomic environment and strong institutional quality. The results of this paper can be generalized so to apply to other countries or country groups. There are numerous studies that a high-level of bank profitability and bank capital, as well as stable macroeconomic conditions, decrease the level of NPLs. However, the studies that deal with the effect of institutional quality on the level of NPLs are less abundant. The current study reports under which conditions all these factors can significantly decrease the level of NPLs in the MENA region.
Like any other, this research has certain limitations. One of the main concerns is a short period of observations of the COVID-19. This short interval partly explains the non-significant effect of the number of active cases and the number of fatalities on the level of NPLs. Alternatively, it can be assumed that credit risk caused by the pandemic (especially related to the loans approved to small and mid-size enterprises and certain industries such as hospitality and tourism, transport, etc.) has still not been materialized, and the extension of the period of observations of the COVID-19 for the years 2021 and 2022 in future research should reveal the true extent of the overall impact. Moreover, related to future research, the inclusion of additional control variables may further improve the empirical results of this study.
Disclosure statement
No potential conflict of interest was reported by the author(s).
Additional information
Funding
Notes
1. As of January 2021, the UNICEF provides a classification of the MENA region that includes twenty countries, that is, Algeria, Bahrain, Djibouti, Egypt, Islamic Republic of Iran, Iraq, Jordan, Kuwait, Lebanon, Libya, Morocco, Oman, Qatar, Saudi Arabia, State of Palestine, Sudan, Syrian Arab Republic, Tunisia, United Arab Emirates, and Yemen.
2. Data related to the growth rate of GDP and the inflation rate are collected from the World Bank Indicators during the period 2005–2020 through this link https://databank.worldbank.org/reports.aspx?source=world-development-indicators&preview=on#.
References
- Ahmad, F. (2013). Corruption and information sharing as determinants of non-performing loans. Bsrj, 4(1), 87–23. https://doi.org/10.2478/bsrj-2013-0008
- Allen, F., & Gale, D. (2004). Competition and financial stability. Journal of Money, Credit, and Banking, 36(3), 453–480. https://doi.org/10.1353/mcb.2004.0038
- Alnabulsi, K., & Kozarević, E. (2021). Interdependence between non-performing loans, financial stability and economic growth. 10th International Scientific Symposium “Region, Entrepreneurship, Development” (RED 2021), Osijek, Croatia, June 17. In M. Leko Šimić & B. Crnković (Proceedings of 10th International Scientific Symposium „Region, Entrepreneurship, Development”) ( Eds.) Eds. http://www.efos.unios.hr/red/wp-content/uploads/sites/20/2021/07/RED_2021_Proceedings.pdf.
- Arellano, M., & Bond, S. (1991). Some tests of specification for panel data: Monte Carlo evidence and an application to employment equations. Review of Economic Studies, 58(2), 277–297. https://doi.org/10.2307/2297968
- Arezki, R., Lederman, D., Abou Harb, A., Fan, R. Y., & Nguyen, H. (2019). Reforms and external imbalances: The labor-productivity connection in the Middle East and North Africa. 4th ed. Serial Publications “MENA Economic Update”, World Bank. https://doi.org/10.1596/978-1-4648-1408-2
- Athanasoglou, P. P., Brissimis, S. N., & Delis, M. D. (2008). Bank-specific, industry-specific and macroeconomic determinants of bank profitability. Journal of International Financial Markets, Institutions and Money, 18(2), 121–136. https://doi.org/10.1016/j.intfin.2006.07.001.
- Bayar, Y. (2019). Macroeconomic, institutional and bank-specific determinants of non-performing loans in emerging market economies: A dynamic panel regression analysis. Journal of Central Banking Theory and Practice, 8(3), 95–110. https://doi.org/10.2478/jcbtp-2019-0026
- Beck, T., & Demirguc-Kunt, A. (2006). Small and medium-size enterprises: Access to finance as a growth constraint. Journal of Banking & Finance, 30(11), 2931–2943. https://doi.org/10.1016/j.jbankfin.2006.05.009
- Beck, R., Jakubik, P., & Piloiu, A. (2015). Key determinants of non-performing loans: new evidence from a global sample. Open Economies Review, 26(3), 525–550. https://doi.org/10.1007/s11079-015-9358-8
- Berger, A. N., & DeYoung, R. (1997). Problem loans and cost efficiency in commercial banks. Journal of Banking & Finance, 21(6), 849–870. https://doi.org/10.1016/S0378-4266(97)00003-4
- Blundell, R., & Bond, S. (1998). Initial conditions and moment restrictions in dynamic panel data models. Journal of Econometrics, 87(1), 115–143. https://doi.org/10.1016/S0304-4076(98)00009-8
- Bolarinwa, S. T., Akinyele, O., & Vo, X. V. (2021). Determinants of nonperforming loans after recapitalization in the Nigerian banking industry: Does efficiency matter? Managerial and Decision Economics, 42(6), 1509–1524. https://doi.org/10.1002/mde.3323
- Boudriga, A., Taktak, N. B., & Jellouli, S. (2010). Bank specific, business and institutional environment determinants of banks nonperforming loans: Evidence from MENA countries. Working Paper No. 547, Economic Research Forum.
- Brahmana, R., Kontesa, M., & Gilbert, R. E. (2018). Income diversification and bank performance: Evidence from Malaysian banks. Economics Bulletin, 38(2), 799–809 http://www.accessecon.com/Pubs/EB/2018/Volume38/EB-18-V38-I2-P79.pdf.
- Chaibi, H., & Ftiti, Z. (2015). Credit risk determinants: Evidence from a cross-country study. Research in International Business and Finance, 33(C), 1–16. https://doi.org/10.1016/j.ribaf.2014.06.001
- Ćurak, M., Pepur, S., & Poposki, K. (2013). Determinants of non-performing loans – Evidence from Southeastern European banking systems. Banks and Bank Systems, 8(1), 45–53. https://www.businessperspectives.org/index.php/component/zoo/determinants-of-non-performing-loans-evidence-from-southeastern-european-banking-systems.
- Danisman, G. O., & Tarazi, A. (2020). Financial inclusion and bank stability: Evidence from Europe. European Journal of Finance, 26(18), 1842–1855. https://doi.org/10.1080/1351847X.2020.1782958
- De Bock, R., & Demyanets, A. (2012). Bank asset quality in emerging markets: Determinants and spillovers. International Monetry Fund Working Paper, WP/12/71.
- Dinçer, H., Yuksel, S., & Adah, Z. (2018). Relationship between non-performing loans, industry, and economic growth of the African economies and policy recommendations for global growth. In A. Coşkun Özer (Ed.), Globalization and trade integration in developing countries (chapter 9) (pp. 203–228). IGI Global. https://doi.org/10.4018/978-1-5225-4032-8.ch009
- Fofack, H. L. (2005). Nonperforming loans in Sub-Saharan Africa: Causal analysis and macroeconomic implications. Policy Research Working Paper No. 3769, World Bank, Washington, D. C.
- Ghosh, A. (2015). Banking-industry specific and regional economic determinants of non-performing loans: Evidence from US states. Journal of Financial Stability, 20(C), 93–104. https://doi.org/10.1016/j.jfs.2015.08.004
- Githaiga, P. N. (2020). Human capital, income diversification and bank performance–an empirical study of East African banks. Asian Journal of Accounting Research, 6(1), 95–108. https://doi.org/10.1108/AJAR-06-2020-0041
- Gnawali, A. (2018). Non-performing asset and its effects on profitability of Nepalese commercial banks. International Journal of Research in Business Studies and Management, 5(9), 39–47. https://www.ijrbsm.org/papers/v5-i9/5.pdf
- Godlewski, C. J. (2005). Bank capital and credit risk taking in emerging market economies. Journal of Banking Regulation, 6(2), 128–145. https://doi.org/10.1057/palgrave.jbr.2340187
- Hai Trung. (2021). Diversification and bank performance: The case of Vietnamese commercial banks. Journal of Economic and Banking Studies, 1, 23–34. https://hvnh.edu.vn/medias/tapchi/vi/07.2021/system/archivedate/ee9f97ca_B%C3%A0i%20c%E1%BB%A7a%20L%C3%AA%20H%E1%BA%A3i%20Trung.pdf
- Hakimi, A., Boussaada, R., & Karmani, M. (2021). Are financial inclusion and bank stability friends or enemies? Evidence from MENA banks. Applied Economics, 54(21), 2473–2489. https://doi.org/10.1080/00036846.2021.1992342
- Hasan, R., & Ashfaq, M. (2021). Corruption and its diverse effect on credit risk: Global evidence. Future Business Journal, 7(1), 1–13. https://link.springer.com/article/10.1186/s43093-021-00060-1
- Hue, N. T. M. (2015). Non-performing loans: Affecting factor for the sustainability of Vietnam commercial banks. Journal of Economics and Development, 17(1), 93–106. https://jed.neu.edu.vn/Uploads/JED%20Issue/2015/Vol%2017%20No1/Article%206_JED_Vol%2017_Number%201.pdf
- Hu, J.-L., Li, Y., & Chiu, Y.-H. (2004). Ownership and nonperforming loans: Evidence from Taiwans banks. Developing Economies, 42(3), 405–420. https://doi.org/10.1111/j.1746-1049.2004.tb00945.x
- International Monetary Fund. (2015). The treatment of nonperforming loans. 18th meeting of the IMF committee on balance of payments statistics.
- Jabbouri, I., & Naili, M. (2019). Determinants of nonperforming loans in emerging markets: Evidence from the MENA region. Review of Pacific Basin Financial Markets and Policies, 22(4), 1–33. https://doi.org/10.1142/S0219091519500267
- Jiménez, G., Lopez, J. A., & Saurina, J. (2013). How does competition affect bank risk-taking? Journal of Financial Stability, 9(2), 185–195. https://doi.org/10.1016/j.jfs.2013.02.004
- Jolevski, L. (2017). Non-performing loans and profitability indicators: The case of the Republic of Macedonia. Journal of Contemporary Economic and Business Issues, 4(2), 5–20. https://www.econstor.eu/handle/10419/193475
- Kartikasary, M., Marsintauli, F., Serlawati, E., & Laurens, S. (2020). Factors affecting the non-performing loans in Indonesia. Acounting, 6(2), 97–106. https://doi.org/10.5267/j.ac.2019.12.003
- Keeley, M. C. (1990). Deposit insurance, risk, and market power in banking. American Economic Review, 80(5), 1183–1200. https://www.jstor.org/stable/2006769
- Keeton, W. R. (1999). Does faster loan growth lead to higher loan losses? Economic Review-Federal Reserve Bank of Kansas City, Federal Reserve Bank of Kansas City, 84 2 , 57–76. https://www.proquest.com/openview/71ac5a538ac57394755a0ee54c60a2b2/1?pq-origsite=gscholar&cbl=47211
- Keeton, W. R., & Morris, C. S. (1987). Why do banks loan losses differ. Economic Review, 72(5), 3–21. https://www.kansascityfed.org/documents/1255/1987-Why%20Do%20Banks'%20Loan%20Losses%20Differ%3F.pdf
- Khan, M. A., Siddique, A., & Sarwar, Z. (2020). Determinants of non-performing loans in the banking sector in developing state. Asian Journal of Accounting Research, 5(1), 135–145. https://doi.org/10.1108/ajar-10-2019-0080
- Klein, N. (2013). Non-performing loans in CESEE: Determinants and impact on macroeconomic performance. International Monetry Fund Working Paper,/13/72.
- Louzis, D. P., Vouldis, A. T., & Metaxas, V. L. (2012). Macroeconomic and bank-specific determinants of non-performing loans in Greece: A comparative study of mortgage, business, and consumer loan portfolios. Journal of Banking and Finance, 36(4), 1012–1027. https://doi.org/10.1016/j.jbankfin.2011.10.012
- Makri, V., Tsagkanos, A., & Bellas, A. (2014). Determinants of non-performing loans: The case of Eurozone. Panoeconomicus, 61(2), 193–206. https://doi.org/10.2298/PAN1402193M
- Marcus, A. J. (1984). Deregulation and bank financial policy. Journal of Banking & Finance, 8(4), 557–565. https://doi.org/10.1016/S0378-4266(84)80046-1
- Memdani, L. (2017). Macroeconomic and bank specific determinants of non-performing loans (NPLs) in the Indian Banking Sector. Studies in Business and Economics, 2(2), 125–135. https://doi.org/10.1515/sbe-2017-0026
- Misra, B. M., & Dhal, S. (2010). Pro-cyclical management of banks non-performing loans by the Indian Public Sector Banks. BIS Asian Research Papers, 16, 1–23. https://www.bis.org/repofficepubl/arpresearch201003.08.pdf June 2010
- Mondal, T. (2016). Sensitivity of non-performing loan to macroeconomic variables: Empirical evidence from Banking Industry in Bangladesh. Global Journal of Management and Business Research, 16(4), 31–38. https://globaljournals.org/item/5883-sensitivity-of-non-performing-loan-to-macroeconomic-variables-empirical-evidence-from-banking-industry-of-bangladesh
- Muhović, A., & Subić, J. (2019). Analysis and impact of main macro and microeconomic factors on the growth on NPL-s in the emerging financial markets. Ekonomika, 65(4), 21–30. https://doi.org/10.5937/ekonomika1904021M
- Ozili, P. K. (2019). Non-performing loans in European systemic and non-systemic banks. Journal of Financial Economic Policy, 12(3), 409–424. https://doi.org/10.1108/JFEP-02-2019-0033
- Rachid, S. (2019). The determinants of non-performing loans: Do institutions matter? A comparative analysis of the Middle East and North Africa (MENA) and Central and Eastern European (CEE) countries. Journal of Advanced Studies in Finance, 10(2), 96–108. https://doi.org/10.14505//jasf.v10.2(20).03
- Radivojevic, N., & Jovovic, J. (2017). Examining of determinants of non-performing loans. Prague Economic Papers, 26(3), 300–316. https://doi.org/10.18267/j.pep.615
- Rajan, R. (1994). Why bank credit policies fluctuate. The Quarterly Journal of Economics, 109(2), 399–441. https://doi.org/10.2307/2118468
- Rajha, K. S. (2016). Determinants of non-performing loans: Evidence from the Jordanian banking sector. Journal of Finance and Bank Management, 4(1), 125–136. https://doi.org/10.15640/jfbm.v5n1a5
- Roman, A., & Bilan, I. (2015). An empirical analysis of the macroeconomic determinants of non-performing loans in EU28 banking sector. Revista Economica, 67(2), 108–127. http://economice.ulbsibiu.ro/revista.economica/archive/6729roman_bilan.pdf
- Sahut, J.-M., & Mili, M. (2011). Banking distress in MENA countries and the role of mergers as a strategic policy to resolve distress. Economic Modelling, 28(1–2), 138–146. https://doi.org/10.1016/j.econmod.2010.09.017
- Salas, V., & Saurina, J. (2002). Credit risk in two institutional regimes: Spanish commercial and saving banks. Journal of Financial Service Research, 22(3), 203–224. https://doi.org/10.1023/A:1019781109676
- Sharma, K. K. (2016). Chart alert: MENA NPL to gross loans ratio lower than South Asia but higher than world average. Algorithm Research.
- Sharma, S., & Anand, A. (2018). Income diversification and bank performance: Evidence from BRICS nations. International Journal of Productivity and Performance Management, 67(9), 1625–1639. https://doi.org/10.1108/IJPPM-01-2018-0013
- Shekhar, A., Bergthaler, W., Garrido, J. M., Ilyina, A., Jobst, A., Kang, K., Kovtun, D., Liu, Y., Monaghan, D., & Moretti, M. (2015). A strategy for resolving europes problem loan. In IMF staff discussion note 15/19. Intrnational montery fund 1–37. https://doi.org/10.5089/9781513591278.006
- Shingjergji, A. (2013). The impact of macroeconomic variables on the non performing loans in the Albanian Banking Sector during 2005-2012. Academic Journal of Interdisciplinary Studies, 2(9), 335–339. https://doi.org/10.5901/ajis.2013.v2n9p335
- Shkodra, J., & Ismajli, H. (2017). Determinants of the credit risk in developing countries: A case of Kosovo banking sector. Banks and Bank Systems, 12(4), 90–97. https://doi.org/10.21511/bbs.12(4).2017.08
- Shrieves, R. E., & Dhal, D. (1992). The relationship between risk and capital in commercial banks. Journal of Banking and Finance, 16(2), 439–457. https://doi.org/10.1016/0378-4266(92)90024-T
- Škarica, B. (2014). Determinants of non-performing loans in Central and Eastern European countries. Financial Theory and Practice, 38(1), 37–59. https://doi.org/10.3326/fintp.38.1.2
- Stiroh, K. J. (2004). Diversification in banking: Is noninterest income the answer? Journal of Money, Credit, and Banking, 36(5), 853–882. https://doi.org/10.1353/mcb.2004.0076
- Swamy, V. (2012). Impact of macroeconomic and endogenous factors on non performing bank assets. International Journal of Banking and Finance, 9(1), 27–47. http://dx.doi.org/10.2139/ssrn.2060753
- Tabak, B. M., Guerra, S. M., Araújo Lima, E. J., & Jung Chang, E. (2007). The stability-concentration relationship in the Brazilian Banking System. Working Paper No. 145, Banco Central do Brasil, 1–29. https://doi.org/10.1016/j.intfin.2007.04.004.
- Tanasković, S., & Jandrić, M. (2015). Macroeconomic and institutional determinants of non-performing loans. Journal of Central Banking Theory and Practice, 4(1), 47–62. https://doi.org/10.1515/jcbtp-2015-0004
- Teixeira, A. A., & Queirós, A. S. (2016). Economic growth, human capital and structural change: A dynamic panel data analysis. Research Policy, 45(8), 1636–1648. https://doi.org/10.1016/j.respol.2016.04.006
- Toader, T., Onofrei, M., Popescu, A.-I., & Andrieș, A. M. (2018). Corruption and banking stability: Evidence from emerging economies. Emerging Markets Finance and Trade, 54(3), 591–617. https://doi.org/10.1080/1540496X.2017.1411257
- Umar, M., & Sun, G. (2018). Determinants of non-performing loans in Chinese banks. Journal of Asia Business Studies, 12(3), 273–289. https://doi.org/10.1108/JABS-01-2016-0005
- UNICEF. (2021). Middle East and North Africa. https://www.unicef.org/mena/
- Vogiazas, S. D., & Nikolaidou, E. (2011). Investigating the determinants of nonperforming loans in the Romanian banking system: An empirical study with reference to the Greek crisis. Economics Research International, 2011, Article ID 214689, 214689:1–214689:13. https://doi.org/10.1155/2011/214689
- World Bank. (2015). Global financial development database.
- World Bank. (2021). MENA economies face rapid accumulation of public debt; strong institutions will be key to recovery.
- World Bank. (2022). Global outlook: Global economic prospects.
- Yilmaz, B. (2019). Macroeconomic, institutional and bank-specific determinants of non-performing loans in emerging market economies: A dynamic panel regression analysis. Journal of Central Banking Theory and Practice, 8(3), 95–110. https://doi.org/10.2478/jcbtp-2019-0026
- Zeng, S. (2012). Bank non-performing loans (NPLS): A dynamic model and analysis in China. Modern Economy, 3(1), 100–110. https://doi.org/10.4236/me.2012.31014
- Zhou, Q., Faff, R., & Alpert, K. (2014). Bias correction in the estimation of dynamic panel models in corporate finance. Journal of Corporate Finance, 25(1), 494–513. https://doi.org/10.1016/j.jcorpfin.2014.01.009