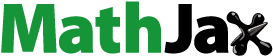
Abstract
This paper investigates the effect of intellectual capital (IC) and its components on the efficiency of Vietnamese commercial banks from 2007 to 2019 using the two-step Data Envelopment Analysis approach. Banks’ efficiency scores are firstly estimated, while the relationship between IC and bank efficiency is examined in the second stage. The results indicate a positive relationship between IC and banks’ pure technical efficiency, allocative efficiency, and total cost efficiency. When observing the effect of IC decompositions, the findings show that only human capital enhances all types of bank efficiency. Furthermore, bank size and liquidity risk are significant drivers of Vietnamese bank efficiency. Therefore, our findings suggest that bank managers should focus on intellectual capital, particularly human capital, to strengthen bank efficiency further.
1. Introduction
The growth of innovation and technologies and an increasingly competitive environment have made knowledge-based resources crucial in creating value and substituting the traditional factors of firms’ production (Al-Musali & Ismail, Citation2016; Xu & Li, Citation2019). In which intellectual capital is seen as a critical resource that could ensure a firm achieves superior economic, financial, and market performance (Sannino et al., Citation2021) and thereby determine an organization’s success under the resource-based view (RBV) paradigm (Barney, Citation1991). Since intellectual capital provides additional value to the organization and is considered one of the key sources of sustainable competitive advantage, this topic has attracted much attention from academic researchers and practitioners (Rehman, Bresciani et al., Citation2021).
Indeed, intellectual capital (IC) is even more relevant to the banking sector because it helps to identify the degree of a bank’s performance in comparison to its rivals (Meles et al., Citation2016; Ting et al., Citation2021). Tran and Vo (Citation2018) also provided some explanations on the appropriateness of IC in the banking industry. Because banking operations heavily rely on customers and bank products are not manufactured goods, banks must offer the highest quality and various services to customers to survive. Banks have intensively invested in human resources, brand names, systems, and processes. Given that IC is a multi-dimensional resource of experience, knowledge, and practical capabilities, it would help banks enhance their effectiveness and efficiency, thus maintaining long-term competitive advantage.
The evidence of the relationship between IC and bank performance is mixed. Early studies focus on bank performance using accounting/market-based measures (e.g., return on assets, return on equity, Tobin’s Q ratio). Several studies using bank data in developed markets have shown that IC is a significant determinant of bank performance (Bollen et al., Citation2005; Clarke et al., Citation2011; Joshi et al., Citation2013; Meles et al., Citation2016; Mention & Bontis, Citation2013; Riahi‐Belkaoui, Citation2003; Ståhle et al., Citation2011; Tan et al., Citation2007; Youndt et al., Citation2004; Zeghal & Maaloul, Citation2010). In the same vein, several studies in emerging markets have claimed the same conclusion (Firer & Williams, Citation2003; Goh, Citation2005; Le, Nguyen, McMillan et al., Citation2020; Mondal & Ghosh, Citation2012; Pendo, Citation2020; Poh et al., Citation2018; Singh et al., Citation2016; Smriti & Das, Citation2018; Tran & Vo, Citation2018)
Lotto (Citation2019) stated that operating efficiency is one of the crucial factors determining bank success. However, the evidence of IC on bank efficiency is relatively scanty (Adesina, Citation2019; Duho, Citation2020; Ting et al., Citation2021; Vidyarthi, Citation2019; Vidyarthi & Tiwari, Citation2019). The literature in IC components-bank efficiency nexus also yields confounding findings. Several studies have indicated a negative relationship between relation capital and bank efficiency (Ting et al., Citation2021), while others have found opposite findings (Mondal & Ghosh, Citation2012). The insignificant impact of IC components on bank efficiency is also documented by Tandon et al. (Citation2016), Alhassan and Asare (Citation2016), and Mention and Bontis (Citation2013). Due to different measures of bank efficiency used and significant differences in regulatory and economic environments in which banks have operated, evidence on IC–bank efficiency nexus is inconclusive. This study will revisit this matter using the Vietnamese banking system in its distinguished setting.
Vietnam boasts one of the leaders in the economic performance of the Association of Southeast Asian Nations (ASEAN), with an average annual economic growth of 6.2% from 2007 to 2019. It is not surprising that this country is classified as Asia’s next dragon. As the financial market is still developing, the remarked growth of the Vietnamese economy is attributed to the banking system. Maintaining efficiency is one of main concerns of academics and practitioners and Vietnamese authorities. Because the literature argues that IC is one of the increasingly crucial factors contributing to the success of banks, this necessitates investigating whether IC has any effect on bank efficiency in Vietnam. Since Vietnam entered World Trade Organization in 2007, the presence of foreign banks in the market has increased the challenge to the growth of local banks’ deposits and loans, thus, may affect their efficiency. To overcome this challenge, banks should pay more attention to IC as their long-term goal (Le, Nguyen, McMillan et al., Citation2020).
This study contributes to the literature in several ways. Most prior studies have examined the effect of IC on bank performance using accounting measures, whereas our study looks at the relationship between IC and bank efficiency. Although a few studies have considered the effect of IC on bank efficiency (Ting et al., Citation2021; Vidyarthi, Citation2019; Vidyarthi & Tiwari, Citation2019), the evidence in emerging markets, especially ASEAN region, is limited. Thus, this study will provide a comprehensive understanding of the impact of intellectual capital on the efficiency of the banking system. To the best of our knowledge, this research is the first attempt to examine the relationship between IC (and its components) and bank efficiency (e.g., cost efficiency, technical efficiency and allocative efficiency) in Vietnam using a two-stage DEA framework. This study, therefore, provides significant implications for bank managers and policymakers.
Using panel data of 30 commercial banks in Vietnam between 2007 and 2019, the findings show a positive effect of IC on bank efficiency. When observing IC components, the results indicate that only human capital can stimulate three types of bank efficiency. Additionally, allocative efficiency is positively affected by capital employed efficiency and structural capital efficiency.
The remainder of this paper is structured as follows. Section 2 reviews the relevant literature. Section 3 describes the methodology and data employed for the study. Section 4 discusses empirical results while Section 5 concludes this study.
2. Literature review
One may argue that an individual firm in each industry sector has a heterogeneous set of tangible/physical (e.g., property, plant, equipment, physical technologies, production facilities, and raw materials) and intangible resources (competencies, capabilities, skills, tacit and explicit knowledge, culture, relationships, brands, and patents). The resource-based view paradigm suggests that these resources constantly contribute to an organization’s value creation (Barney, Citation1991). Alternatively, a firm’s resources and capabilities can result in superior performance and competitive advantage (Rehman, Ashfaq et al., Citation2021). In which IC, as one of important firm’s resources, plays a crucial roles in the value generating process (Barney, Citation1991; Kamath, Citation2007; Sannino et al., Citation2021). Therefore, many empirical studies have attempted to examine whether IC could improve bank performance. Note that understanding the definition of IC is important since it will determine how IC can be measured.
It is acknowledged that the concept of intellectual capital remains controversial (Marr & Moustaghfir, Citation2005). Different disciplines and perspectives from various fields (e.g., economics, strategy, finance, accounting, human resources, reporting and disclosure, marketing, and communication) define IC differently. More specifically, Giacosa et al. (Citation2017) argued that IC addresses knowledge-related difficulties in businesses. Additionally, Sullivan (Citation2000) defined IC as the total value of intangible assets that are not declared in the corporate’s balance sheet, while Bontis (Citation1998) considers IC as the stock of knowledge in an organization. Ghe and Burz (Citation2008) asserted that IC could convert knowledge into value-generating resources. In a broad view, IC is described as knowledge-related intangible assets that comprise intellectual resources, intellectual property, and intellectual competencies (F.-C. Chen et al., Citation2014).
Given various definitions and data availability, several traditional measurements of IC and its components have been proposed and applied in multiple disciplines. In particular, Saint‐Onge (Citation1996) and Stewart (Citation2007) conceptualized IC as the sum of human capital, structural capital, and customer capital. Bontis (Citation1998), however, classified IC into financial capital, human capital, and structural capital. Alternatively, several papers categorize IC as human capital, structural capital, and relational capital (Kujansivu, Citation2005; Petty & Guthrie, Citation2000; Sveiby, Citation1997). Following most prior studies on intellectual capital, we focus on one of the most common approaches as proposed by Pulic (Citation2004) or the so-called value-added intellectual coefficient (VAIC).Footnote1 This method is widely used in many sectors and industries by assuming that the value creation efficiency in organizations is the contribution of all organization’s resources (e.g., human capital, physical capital, and structural capital).
Using the VAIC framework, many studies have attempted to investigate the association between the IC and bank performance and yielded conflicting results. Meles et al. (Citation2016) found a strong positive correlation between IC and bank performance. This finding is in line with the studies of Bollen et al. (Citation2005); M. C. Chen et al. (Citation2005); Clarke et al. (Citation2011); Diez et al. (Citation2010); Ng (Citation2006); Tan et al. (Citation2007); Tovstiga et al. (Citation2007); Wang et al. (Citation2005); Youndt et al. (Citation2004), Zeghal and Maaloul (Citation2010), Anifowose et al. (Citation2017), Joshi et al. (Citation2013), Mehralian et al. (Citation2012), Tiwari and Vidyarthi (Citation2018), Rehman, Elrehail, Alsaad and Bhatti (Citation2021), and Rehman, Ashfaq et al. (Citation2021). By contrast, studies by Firer and Williams (Citation2003), Gan and Saleh (Citation2008), Ståhle et al. (Citation2011), Williams et al. (Citation2011), and Zeghal and Maaloul (Citation2010) have failed to prove that organizations with a good source of human capital, well-established capital structures, and well-organizational processes boost firm’s performance.
In contrast to using conventional measures of bank performance, bank efficiency has received much attention from academic research. This inspires several studies to examine the effect of intellectual capital on bank efficiency. Vidyarthi and Tiwari (Citation2019), using a panel of 37 Indian banks, indicated the positive but minimal impact of the aggregate IC on cost efficiency, revenue efficiency, and profit efficiency. This result is comparable with Adesina (Citation2019), who claims the positive effect of IC on banks’ efficiency in 31 African countries. When observing the IC components, Onumah and Duho (Citation2020) concluded that human capital is the main determinant of pure technical and cost efficiencies in Ghana, while profit efficiency is positively affected by human capital efficiency and capital employed efficiency. Similarly, Duho (Citation2020) demonstrated the positive relationship between IC and bank efficiency, and human capital is the main driver. On the other hand, Ting et al. (Citation2021) suggested that bank branches’ efficiency in Taiwan is not affected by human capital and structural capital but is negatively associated with relational capital. Due to the mixed evidence of the effect of IC in emerging markets, especially the ASEAN region, this study revisits this disagreement in the context of the Vietnamese banking system
It is acknowledged that limited studies examine the impact of intellectual capital on either bank profitability (Le, Nguyen, McMillan et al., Citation2020; Tran & Vo, Citation2018) or bank risk (Nguyen et al., Citation2021). It is argued that the resources from IC, in the form of experience, knowledge, and practical capabilities, may contribute to banks’ efficiency, but their direct impacts have received little attention (Vidyarthi and Tiwari (Citation2019); Dumay (Citation2016)). By assessing the impact of IC on bank efficiency (e.g., cost efficiency, technical efficiency, and allocative efficiency), this study will offer a better understanding of the effect of IC in the Vietnamese banking system.
3. Methodology and data
This study examines the relationship between intellectual capital and bank efficiency using a two-stage Data Envelopment Analysis (DEA) approach. In the first stage, bank efficiency is estimated using DEA. In the second stage, bank efficiency scores are then regressed against intellectual capital estimated from VAIC framework as proposed by Pulic (Citation2004) while controlling for other factors.
3.1. Measuring bank efficiency—the DEA approach
It is worth noting that bank efficiency is primarily calculated using either the parametric method (stochastic frontier approach—SFA) and the non-parametric method (Data Envelopment Analysis—DEA; Berger & Mester, Citation1997). Bauer et al. (Citation1998) argue that there is no best method to measure efficiency. Weill (Citation2004) affirms the well-matched average efficiency scores measured by DEA and SFA approaches. Similarly, Çelik (Citation2012) emphasize that both SFA and DEA results are similar. Indeed, each of them has its own advantages and disadvantages as documented by many studies such as Ho et al. (Citation2021), Le et al. (Citation2021). DEA is selected to measure bank efficiency in our study since it does not required a priori production function and is more appropriate to our small sample size (Boubaker et al., Citation2022).
DEA was firstly developed by Farrell (Citation1957) and extended by Charnes et al. (Citation1978). The two major DEA models, constant returns to scale (CRS) and variable returns to scale (VRS), are widely used in many empirical studies. This study uses input-oriented DEA under variable returns to scale (VRS) assumption to estimate bank cost efficiency (Le, Citation2018; Le, Citation2020; Le et al., Citation2021; T. Ngo & Le, Citation2019; T. Ngo & Tripe, Citation2017). Consequently, the VRS cost minimization DEA model is constructed as follows:
Subject to
where = 1, 2, 3, …, n denotes the number of Vietnamese banks, using a vector of
inputs
= (
for which they pay prices
= (
to generate a vector of s outputs
= (
;
illustrates the vector of input and output weights.
The optimal value of input demand vector = (
to minimize costs for bank j with the given input prices
can be identified by solving the linear programming problem (1). The cost efficiency (
) of bank j is measured by the proportion of the minimum cost and the actual cost:
Following Sealey and Lindley (Citation1977), banks are seen as an intermediary employing personnel and physical capital to transform deposits into earning assets. As a result, net loans () and other earning assets (
) are the two outputs, and total funding (
), fixed assets (
), and personnel expenses (
) are used as three inputs. In addition, the corresponding prices of inputs are financial capital price (
), physical capital price (
), and labour price
, respectively.
3.2. Measuring bank intellectual capital and its decompositions
The VAIC literature suggests different approaches to measuring IC.Footnote2 However, the conventional VAIC methodology is used in this study as it provides standardized and consistent measures (Shiu, Citation2006). The conventional VAIC methodology is assessed as an innovative approach both theoretically and methodologically, and it does not contradict or modify any of the fundamental principles of accounting (Iazzolino & Laise, Citation2013).
Following Pulic (Citation2004), Meles et al. (Citation2016), and Tran and Vo (Citation2018), and Le, Nguyen, McMillan et al. (Citation2020), the value-added intellectual coefficient (VAIC) is estimated as follows:
where denotes the capital employed efficiency;
represents the human capital efficiency;
is the structural capital efficiency.
Furthermore, the estimation of the total value added () is necessary to measure the VAIC decompositions. Following Le, Nguyen, McMillan et al. (Citation2020), the total value added is obtained as
where
is a bank’s operating profit;
denotes personnel expenses (salaries, wages, and other benefits), and
is the bank’s amortization and depreciation. Once
is estimated, the
elements are calculated as follows:
=
/
where
is measured as the book value of equity.
=
/
, where
refers to staff cost.
=
/
, where
represents structural capital and is calculated as
=
/
.
3.3. Model specifications
In the next stage, the cost efficiency scores as derived from the DEA approach are regressed against IC, (its subcomponents in a separated model), and other explanatory variables. Following Adesina (Citation2019), Batir et al. (Citation2017), Meles et al. (Citation2016), our following baseline model is formed:
Where is bank efficiency (
,
, and
) derived from the input-oriented DEA method (see, section 3.1);
is intellectual capital (see, section 3.2);
and
are the vectors of bank-specific features and macroeconomic factors, respectively. The subscript
represents a bank (j = 1, 2, …, 30) while t,
denote the time trend and the error term, respectively. When considering the effect of IC components, VAIC in Equationequation (5)
(5)
(5) is replaced by
,
, and
(see, section 3.2)
For bank-specific characteristics, bank size () and liquidity risk (
) are used.
as measured by the natural logarithm of total assets, is used to control for bank size. Large banks with market power should pay less for their inputs, thus improve their efficiency (Le, Citation2018). Dietrich and Wanzenried (Citation2014) however contend that smaller banks can improve their performance because of reducing the asymmetric information problems.
, the liquid assets to total assets ratio, is used to control for the effect of liquidity risk (Sharma et al., Citation2013). Sharma et al. (Citation2013) state that holding more liquid assets tends to reduce bank performance. By contrast, banks that hold more liquid assets are less likely defaulted, thus improving bank performance (Bordeleau & Graham, Citation2010; Le, 2017c).
For macroeconomic variables, as measured by the ratio of domestic credit extended to the private sector by banks to GDP is used to control for the impact of banking sector expansion (Adesina, Citation2019). The Herfindahl-Hirschman Index (
), calculated by the sum of the squared market shares of each bank’s assets for a particular year, is proxied for market concentrationFootnote3 (García-Herrero et al., Citation2009).
is close to 0 for a perfectly competitive market and equals 1 in the case of a monopoly. A lower (higher) value of
means a higher (lower) competition level. We use the annual GDP growth rate (
) to examine the influences of economic expansion on bank efficiency (Haris et al., Citation2019). Finally, the annual inflation rate (
) is employed to control for inflation consequences (Perry, Citation1992).
Because the value of bank efficiency score ranges between 0 and 1, the Tobit regression is recommended to overcome this limitation (Kumbhakar & Lovell, Citation2000). The Tobit model also account for the features of the distribution of efficiency metrics and thereby, producing consistent results (Sufian & Noor, Citation2009). Following Adesina (Citation2019); Batir et al. (Citation2017); Shaban and James (Citation2018), the Tobit regression (so-called one-sided censored Tobit model) is used to accommodate for the narrow [0, 1] range of efficiency values as derived from DEA approach in the first stage which are then regressed against with VAIC and its sub-components in the second stage.
To avoid the endogeneity problem that may arise (e.g., the probability of causality between bank efficiency and market concentration and other determinants), the system GMM proposed by Arellano and Bover (Citation1995) is used for robustness checks. The GMM estimator accounts for unobserved heterogeneity and the persistence of the dependent variable into consideration (Arellano, Citation2002), thus producing consistent parameters. In other words, the use of ample instruments results in estimated coefficients more efficient (Le, Citation2021a; Le et al., Citation2022; Le & Ngo, Citation2020).
3.4. Data
Note that several criteria on data collection were applied. Banks with more than five years of data are only included to examine the effect of intellectual capital on bank efficiency. Also, only local commercial banks are chosen to ensure homogenous among DMUs in DEA because they are the most active players, while foreign bank affiliates, wholly foreign-owned banks and joint-venture banks are relatively constrained to operate in the Vietnamese market (Le, Citation2020, Citation2021b; Le et al., Citation2019). This thus arrives at a panel data of 30 Vietnamese commercial banks from 2007 to 2019 which accounted for more than 80% of total assets in the industry. Due to several bank mergers over the studied period,Footnote4 this arrives at an unbalanced panel with 378 observations. Bank-specific information is obtained from banks’ financial statements on consolidated basis following Vietnamese Accounting Standards and the database constructed by Le et al. (Citation2022). Macroeconomic indicators are taken from the World Bank Database.
Table presents the descriptive statistics for all variables over the period 2007–2019. A mean (a standard deviation) of TE, AE, and CE are 0.720 (0.196), 0.727 (0.149), and 0.537 (0.216) respectively.Footnote5 The key independent variable, VAIC, has the mean of 4.783 and the standard deviation of 2.279. Moreover, the mean (standard deviation) values of IC sub-elements, CEE, HCE, and SCE are 0.299 (0.138), 3.816 (2.124), and 0.669 (0.280) correspondingly.
Table 1. Descriptive statistics of variables
In addition, variance inflation factors (VIF) test is used to examine the potential multicollinearity problem in this study.Footnote6 The correlation matrix among variables used is displayed in Table . Together, these results confirm no multicollinearity issues.
Table 2. Correlation matrix
4. Empirical results
4.1. Intellectual capital and bank efficiency
Table illustrates the effect of IC on bank efficiency over the period 2007–2019, using the Tobit regression.
Table 3. The relationship between IC and bank efficiency—Tobit regressions
The positive coefficients on in all models suggest that intellectual capital can improve bank cost efficiency and its decompositions (pure technical efficiency and allocative efficiency). This finding supports the view of the RBV theory that intellectual resources play a critical role in bank performance. This finding is comparable with those of Onumah and Duho (Citation2020), Vidyarthi (Citation2019), and Vidyarthi and Tiwari (Citation2019), who found that intellectual capital as a significant value-creating resource can improve business activities, thus strengthening their efficiency and generating higher returns.
Regarding bank-specific variables, the coefficients on in all models imply that larger banks are more efficient than smaller counterparts. This finding is comparable with the studies by Peng et al. (Citation2017), Vu and Nahm (Citation2013), and Assaf et al. (Citation2011). Two main explanations are provided. First, large banks can lower costs of their inputs if it associates with market power. Second, large banks may have the advantage of increasing returns to scale by either allocating fixed costs over a greater volume of services or obtaining efficiency gains from a specialized workforce (Hauner, Citation2005). Additionally, the positive coefficients on
in three modes suggest that more liquid banks are more efficient than less liquid peers. Nonetheless, this finding supports the view of the opportunity costs hypothesis that holding a higher fraction of liquid assets improves bank performance (Assaf et al., Citation2011; Bordeleau & Graham, Citation2010; Le, Citation2017b; Le, Nguyen, McMillan et al., Citation2020).
Regarding macroeconomic variables, the positive coefficients on demonstrate that the more level of market concentration, the more cost efficiency that banks can achieve. As per the structure-conduct-performance hypothesis, banks with market power can pay lower rates on customer deposits, thus enjoying cost savings (Le & Ngo, Citation2020; Saona, Citation2016). Phan et al. (Citation2016) also indicate that strong competition may induce banks to increase deposit interest rates to attract customers, thus reducing bank efficiency. Furthermore, the findings also show that banking sector expansion (
) is positively associated with allocative efficiency (
) and cost efficiency (
) but insignificantly related to technical efficiency (
). This is somewhat comparable to the early suggestion that bank cost efficiency is strongly affected by banking development (Gardener et al., Citation2011; Levine et al., Citation2000). The coefficient of
is positive and statistically significant as expected, suggesting that economic growth increases the demand for banking products and services, thus increasing bank efficiency. This result supports the well-documented literature on the relationship between economic growth and financial sector performance (Le, Nguyen, Tran et al., Citation2020). The negative coefficients on
imply that a higher inflation rate lowers bank efficiency because it directly affects macroeconomic and financial instability. These findings are similar to those of Vu and Nahm (Citation2013), and Hermes and Nhung (Citation2010).
4.2. Intellectual capital and bank efficiency: sub-elements analysis
We further extend our analysis by decomposing the intellectual capital into three sub-elements, namely capital employed efficiency (), human capital efficiency (
), and structural capital efficiency (
).
Table indicates that the coefficients of are positive and statistically significant in all models, suggesting that human capital efficiency is one of the most important factors to determine bank efficiency in Vietnam. Inkinen (Citation2015) emphasizes that human capital representing employees’ knowledge, education, capabilities, and skills is considered as the most crucial intangible asset. Thus, human capital efficiency is the most essential contributors to bank efficiency. Nonetheless, this is in line with the findings of Mention and Bontis (Citation2013) and Adesina (Citation2019), who demonstrated that human capital contributes directly and indirectly to bank performance.
Table 4. The relationship between IC subcomponents and bank efficiency
As can be seen in Table , and
are only positively and significantly related to
, suggesting that capital employed, and structural capital efficiency are the main drivers of allocative efficiency in Vietnam. This is somewhat comparable with the findings of Alhassan and Asare (Citation2016), Tandon et al. (Citation2016), and Mention and Bontis (Citation2013), who found insignificant relationship between structural capital and bank efficiency, and Adesina (Citation2019), who claimed insignificant effects of capital employed on bank efficiency. Finally, similar results of other control variables are also obtained.
4.3. Robustness checks
As stated in section 3.2, the potential endogeneity issues between bank efficiency and bank characteristics and macroeconomic conditions may arise (Adesina, Citation2019). The system GMM as suggested by Arellano and Bover (Citation1995) is used to overcome possible bias due to unobserved heterogeneity and endogeneity. We re-estimate the model using the system GMM with one-year lag of all explanatory variables. The results of using system GMM are presented in Table .
Table 5. The IC, and its decomposition—bank efficiency nexus using system GMM
The p-values of the Hansen test are statistically not significant in any of the models, implying no over-identifying constraints. Alternatively, all conditions for the moments are satisfied, and the instruments are valid. Also, the p-value of AR1 is statistically significant, thus rejecting the hypothesis of the non-existence of the first-order autocorrelation between the first residual differences. However, this does not imply unreliable estimations because the p-values of AR2 in our models are statistically insignificant (Arellano & Bond, Citation1991). Together, the moment conditions are met. Nonetheless, the results shown in Table confirm our above findings.
5. Conclusions and research implications
This research investigated the impact of intellectual capital on bank efficiency in Vietnam between 2007 and 2019 using a two-stage DEA approach. In the first stage, technical, allocative, and cost efficiencies are estimated from the input-oriented DEA under variable returns to scale assumption. The correlation between these calculated efficiency scores and intellectual capital is then examined in the second stage. The findings showed that intellectual capital could enhance banks’ pure technical efficiency (TE), allocative efficiency (AE), and overall cost efficiency (CE). This thus confirms the relevance of the resource-based view theory to banking system that organizations should utilize their intangible resources and capabilities to create superior performance and competitive advantage in such knowledge-intensive industries (Barney, Citation1991). Nonetheless, our findings suggested that bank managers in Vietnam should focus on intangible resources to operate more efficient in the long term. In terms of regulatory perspective, the authorities should promote further initiatives to spill over the ideas of intellectual capital development among Vietnamese banks.
The findings revealed that human capital efficiency improves all three efficiency measures when observing the effect of intellectual capital components. Bank managers should further put more attention on human capital development and fully optimize the utilization of human resources in each operational process. For example, the investment in human capital may include training, education, and other incentives to accelerate more skillful employees within a business.
Furthermore, the findings also demonstrated a positive relationship between liquidity and bank efficiency, suggesting that the authorities should encourage banks to hold more liquid assets to enhance bank efficiency and also strengthen bank stability. Additionally, the policymakers should further promote banking development and implement appropriate measures of target inflation control to maintain the efficiency of the Vietnamese banking system.
The study may have some of the following limitations. Future research may investigate whether a non-linear relationship between intellectual capital and bank efficiency may exist. Thus, this may require researchers to further evaluate how much intellectual capital banks should invest, given the non-linear relationship. Furthermore, our research only examined one country over a short period, suggesting that future studies should examine this correlation in other emerging markets with a similar banking structure to Vietnam for robustness checks. Given the growth of fintech credit development and its substantial impact on the banking system (Le, Citation2022a, Citation2022b; Le et al., Citation2021), future research needs to account for this matter when examining effect of intellectual capital on bank efficiency.
Acknowledgements
This research is funded by the University of Economics and Law, Vietnam National University, Ho Chi Minh City, Vietnam.
Disclosure statement
No potential conflict of interest was reported by the author(s).
Additional information
Funding
Notes
1. This method relies on three sub-elements: structural capital efficiency (SCE), human capital efficiency (HCE), and capital employed efficiency (CEE), and makes use of these decompositions to estimate the Value-Added Intellectual Coefficient (VAIC; Pulic, Citation2004).
2. Please see, Bayraktaroglu et al. (Citation2019) for comprehensive discussions on VAIC techniques.
3. We are aware that other market concentration metrics such as the Lerner index and the Rosse-Panzar could be used. However, these non-structure measurements have some significant shortcomings as discussed by Dietrich and Wanzenried (Citation2014).
4. Please see, Le (Citation2017) for more information.
5. Please see Appendix A3 for more details of estimated efficiency scores over the sample period.
6. Please see Appendix A2 for the VIFs statistics report.
References
- Adesina, K. S. (2019). Bank technical, allocative and cost efficiencies in Africa: The influence of intellectual capital. The North American Journal of Economics and Finance, 48, 419–18. https://doi.org/10.1016/j.najef.2019.03.009
- Alhassan, A. L., & Asare, N. (2016). Intellectual capital and bank productivity in emerging markets: Evidence from Ghana. Management Decision, 54(3), 589–609. https://doi.org/10.1108/MD-01-2015-0025
- Al-Musali, M. A., & Ismail, K. N. I. K. (2016). Cross-country comparison of intellectual capital performance and its impact on financial performance of commercial banks in GCC countries. International Journal of Islamic and Middle Eastern Finance and Management, 9(4), 512–531. https://doi.org/10.1108/IMEFM-03-2015-0029
- Anifowose, M., Rashid, H. M. A., & Annuar, H. A. (2017). Intellectual capital disclosure and corporate market value: Does board diversity matter? Journal of Accounting in Emerging Economies, 7(3), 369–398. https://doi.org/10.1108/JAEE-06-2015-0048
- Arellano, M. (2002). Sargan’s intrumental variables estimation and the generalized method of moments. Journal of Business & Economic Statistics, 20(4), 450–459. https://doi.org/10.1198/073500102288618595
- Arellano, M., & Bond, S. (1991). Some tests of specification for panel data: Monte Carlo evidence and an application to employment equations. The Review of Economic Studies, 58(2), 277–297. https://doi.org/10.2307/2297968
- Arellano, M., & Bover, O. (1995). Another look at the instrumental variable estimation of error-components models. Journal of Econometrics, 68(1), 29–51. https://doi.org/10.1016/0304-4076(94)01642-D
- Assaf, A. G., Barros, C. P., & Matousek, R. (2011). Technical efficiency in Saudi banks. Expert Systems with Applications, 38(5), 5781–5786. http://doi.org/10.1016/j.eswa.2010.10.054
- Barney, J. (1991). Firm resources and sustained competitive advantage. Journal of Management, 17(1), 99–120. https://doi.org/10.1177/014920639101700108
- Batir, T. E., Volkman, D. A., & Gungor, B. (2017). Determinants of bank efficiency in Turkey: Participation banks versus conventional banks. Borsa Istanbul Review, 17(2), 86–96. https://doi.org/10.1016/j.bir.2017.02.003
- Bauer, P. W., Berger, A. N., Ferrier, G. D., & Humphrey, D. B. (1998). Consistency conditions for regulatory analysis of financial institutions: A comparison of frontier efficiency methods. Journal of Economics and Business, 50(2), 85–114. https://doi.org/10.1016/S0148-6195(97)00072-6
- Bayraktaroglu, A. E., Calisir, F., & Baskak, M. (2019). Intellectual capital and firm performance: An extended VAIC model. Journal of Intellectual Capital, 20(3), 406–425. https://doi.org/10.1108/JIC-12-2017-0184
- Berger, A. N., & Mester, L. J. (1997). Inside the black box: What explains differences in the efficiencies of financial institutions? Journal of Banking & Finance, 21(7), 895–947. https://doi.org/10.1016/S0378-4266(97)00010-1
- Bollen, L., Vergauwen, P., & Schnieders, S. (2005). Linking intellectual capital and intellectual property to company performance. Management Decision, 43(9), 1161–1185. https://doi.org/10.1108/00251740510626254
- Bontis, N. (1998). Intellectual capital: An exploratory study that develops measures and models. Management Decision, 36(2), 63–76. https://doi.org/10.1108/00251749810204142
- Bordeleau, É., & Graham, C. (2010). The impact of liquidity on bank profitability. Working Paper No. 2010-38, Bank of Canada, Ontario.
- Boubaker, S., Le, T. D., & Ngo, T. (2022). Managing bank performance under COVID‐19: A novel inverse DEA efficiency approach. International Transactions in Operational Research, 1–17. https://doi.org/10.1111/itor.13132
- Çelik, S. (2012). The measurement of efficiency in Turkish banking sector: The comparison of parametric and non-parametric models. Banks Association of Turkey, Bankers, 23(82), 88–106.
- Charnes, A., Cooper, W. W., & Rhodes, E. (1978). Measuring the efficiency of decision making units. European Journal of Operational Research, 2(6), 429–444. https://doi.org/10.1016/0377-2217(78)90138-8
- Chen, M. C., Cheng, S. J., Hwang, Y., & Bontis, N. (2005). An empirical investigation of the relationship between intellectual capital and firms’ market value and financial performance. Journal of Intellectual Capital, 6(2), 159–176. https://doi.org/10.1108/14691930510592771
- Chen, F.-C., Liu, Z.-J., & Kweh, Q. L. (2014). Intellectual capital and productivity of Malaysian general insurers. Economic Modelling, 36, 413–420. https://doi.org/10.1016/j.econmod.2013.10.008
- Clarke, M., Seng, D., & Whiting, R. H. (2011). Intellectual capital and firm performance in Australia. Journal of Intellectual Capital, 12(4), 505–530. https://doi.org/10.1108/14691931111181706
- Dietrich, A., & Wanzenried, G. (2014). The determinants of commercial banking profitability in low-, middle-, and high-income countries. The Quarterly Review of Economics and Finance, 54(3), 337–354. https://doi.org/10.1016/j.qref.2014.03.001
- Diez, J. M., Ochoa, M. L., Prieto, M. B., & Santidrian, A. (2010). Intellectual capital and value creation in Spanish firms. Journal of Intellectual Capital, 11(3), 348–367. https://doi.org/10.1108/14691931011064581
- Duho, K. C. T. (2020). Intellectual capital and technical efficiency of banks in an emerging market: A slack-based measure. Journal of Economic Studies, 47(7), 1711–1732. https://doi.org/10.1108/JES-06-2019-0295
- Dumay, J. (2016). A critical reflection on the future of intellectual capital: From reporting to disclosure. Journal of Intellectual Capital, 17(1), 168–184. https://doi.org/10.1108/JIC-08-2015-0072
- Farrell, M. J. (1957). The measurement of productive efficiency. Journal of the Royal Statistical Society: Series A (General), 120(3), 253–281. https://doi.org/10.2307/2343100
- Firer, S., & Williams, S. M. (2003). Intellectual capital and traditional measures of corporate performance. Journal of Intellectual Capital, 4(3), 348–360. https://doi.org/10.1108/14691930310487806
- Gan, K., & Saleh, Z. (2008). Intellectual capital and corporate performance of technology-intensive companies: Malaysia Evidence. Asian Journal of Business and Accounting, 1(1), 113–130.
- García-Herrero, A., Gavilá, S., & Santabárbara, D. (2009). What explains the low profitability of Chinese banks? Journal of Banking & Finance, 33(11), 2080–2092. https://doi.org/10.1016/j.jbankfin.2009.05.005
- Gardener, E., Molyneux, P., & Nguyen-Linh, H. (2011). Determinants of efficiency in South East Asian banking. The Service Industries Journal, 31(16), 2693–2719. https://doi.org/10.1080/02642069.2010.512659
- Ghe, B., & Burz, R. (2008). Intellectual capital in the enterprise of the Future–Enterprise based on knowledge. Editura Mirton.
- Giacosa, E., Ferraris, A., & Bresciani, S. (2017). Exploring voluntary external disclosure of intellectual capital in listed companies: An integrated intellectual capital disclosure conceptual model. Journal of Intellectual Capital, 18(1), 149–169. https://doi.org/10.1108/JIC-01-2016-0019
- Goh, P. C. (2005). Intellectual capital performance of commercial banks in Malaysia. Journal of Intellectual Capital, 6(3), 385–396. https://doi.org/10.1108/14691930510611120
- Haris, M., Yao, H., Tariq, G., Malik, A., & Javaid, H. M. (2019). Intellectual capital performance and profitability of banks: Evidence from Pakistan. Journal of Risk and Financial Management, 12(2), 56. https://doi.org/10.3390/jrfm12020056
- Hauner, D. (2005). Explaining efficiency differences among large German and Austrian banks. Applied Economics, 37(9), 969–980. https://doi.org/10.1080/00036840500081820
- Hermes, N., & Nhung, V. T. H. (2010). The impact of financial liberalization on bank efficiency: Evidence from Latin America and Asia. Applied Economics, 42(26), 3351–3365. https://doi.org/10.1080/00036840802112448
- Ho, T. H., Nguyen, D. T., Ngo, T., & Le, T. D. (2021). Efficiency in Vietnamese banking: A meta-regression analysis approach. International Journal of Financial Studies, 9(3), 41. https://doi.org/10.3390/ijfs9030041
- Iazzolino, G., & Laise, D. (2013). Value added intellectual coefficient (VAIC): A methodological and critical review. Journal of Intellectual Capital, 14(4), 547–563. https://doi.org/10.1108/JIC-12-2012-0107
- Inkinen, H. (2015). Review of empirical research on intellectual capital and firm performance. Journal of Intellectual Capital, 16(3), 518–565. https://doi.org/10.1108/JIC-01-2015-0002
- Joshi, M., Cahill, D., Sidhu, J., & Kansal, M. (2013). Intellectual capital and financial performance: An evaluation of the Australian financial sector. Journal of Intellectual Capital, 14(2), 264–285. https://doi.org/10.1108/14691931311323887
- Kamath, G. B. (2007). The intellectual capital performance of the Indian banking sector. Journal of Intellectual Capital, 8(1), 96–123. https://doi.org/10.1108/14691930710715088
- Kujansivu, P. (2005). Intellectual capital performance in Finnish companies. Paper presented at the 3rd Conference on Performance measurement and management Control (EIASM), Nice, France, September 22-23, 2005.
- Kumbhakar, S., & Lovell, C. (2000). Stochastic Frontier Analysis. Cambridge University Press. http://dx.doi.org/10.1017/cbo9781139174411
- Le, T. D. (2017). The efficiency effects of bank mergers: An analysis of case studies in Vietnam. Risk Governance & Control: Financial Markets & Institutions, 7(1), 61–70. http://dx.doi.org/10.2139/ssrn.2822944
- Le, T. D. (2017b). The interrelationship between net interest margin and non-interest income: Evidence from Vietnam. International Journal of Managerial Finance, 13(5), 521–540. https://doi.org/10.1108/IJMF-06-2017-0110
- Le, T. D. (2018). Bank risk, capitalisation and technical efficiency in the Vietnamese banking system. Australasian Accounting Business & Finance Journal, 12(3), 42–61. http://dx.doi.org/10.14453/aabfj.v12i3.4
- Le, T. D. (2020). Multimarket contacts and bank profitability: Do diversification and bank ownership matter? Cogent Economics & Finance, 8(1), 1–21. https://doi.org/10.1080/23322039.2020.1849981
- Le, T. D. (2021a). Can foreign ownership reduce bank risk? Evidence from Vietnam. Review of Economic Analysis, 13(2), 1–24. http://dx.doi.org/10.2139/ssrn.3878912
- Le, T. D. (2021b). Geographic expansion, income diversification, and bank stability: Evidence from Vietnam. Cogent Business & Management, 8(1), 1–23. https://doi.org/10.1080/23311975.2021.1885149
- Le, T. D. (2022a). The roles of financial inclusion and financial markets development in Fintech credit: Evidence from developing countries. International Journal of Blockchains and Cryptocurrencies, 2(4), 339–349. https://doi.org/10.1504/IJBC.2021.120374
- Le, T. D. (2022b). A shift towards household lending during fintech era: The role of financial literacy and credit information sharing. Asia-Pacific Journal of Business Administration, ahead-of-print(ahead-of-print). https://doi.org/10.1108/APJBA-07-2021-0325
- Le, T. D. Q., Ho, T. H., Ngo, T., Nguyen, D. T., & Tran, S. H. (2022). A dataset for the Vietnamese banking system (2002-2021). Data, 7(9), 120. https://doi.org/10.3390/data7090120
- Le, T. D., Ho, T. H., Nguyen, D. T., & Ngo, T. (2021). Fintech credit and bank efficiency: International evidence. International Journal of Financial Studies, 9(3), 1–16. https://doi.org/10.3390/ijfs9030044
- Le, T. D., Ho, T. H., Nguyen, D. T., & Ngo, T. (2022). A cross-country analysis on diversification, Sukuk investment, and the performance of Islamic banking systems under the COVID-19 pandemic. Heliyon, 8(3), e09106. https://doi.org/10.1016/j.heliyon.2022.e09106
- Le, T. D., & Ngo, T. (2020). The determinants of bank profitability: A cross-country analysis. Central Bank Review, 20(2), 65–73. https://doi.org/10.1016/j.cbrev.2020.04.001
- Le, T. D., Nguyen, D. T., & McMillan, D. (2020). Intellectual capital and bank profitability: New evidence from Vietnam. Cogent Business & Management, 7(1), 1859666. https://doi.org/10.1080/23311975.2020.1859666
- Le, T. D., Nguyen, V. T., Tran, S. H., & McMillan, D. (2020). Geographic loan diversification and bank risk: A cross-country analysis. Cogent Economics & Finance, 8(1), 1–20. https://doi.org/10.1080/23322039.2020.1809120
- Le, T. D., Tran, S. H., & Nguyen, L. T. (2019). The impact of multimarket contacts on bank stability in Vietnam. Pacific Accounting Review, 31(3), 336–357. https://doi.org/10.1108/PAR-04-2018-0033
- Levine, R., Loayza, N., & Beck, T. (2000). Financial intermediation and growth: Causality and causes. Journal of Monetary Economics, 46(1), 31–77. https://doi.org/10.1016/S0304-3932(00)00017-9
- Lotto, J. (2019). Evaluation of factors influencing bank operating efficiency in Tanzanian banking sector. Cogent Economics & Finance, 7(1), 1664192. https://doi.org/10.1080/23322039.2019.1664192
- Marr, B., & Moustaghfir, K. (2005). Defining intellectual capital: A three‐dimensional approach. Management Decision, 43(9), 1114–1128. https://doi.org/10.1108/00251740510626227
- Mehralian, G., Rajabzadeh, A., Sadeh, M. R., & Rasekh, H. R. (2012). Intellectual capital and corporate performance in Iranian pharmaceutical industry. Journal of Intellectual Capital, 13(1), 138–158. https://doi.org/10.1108/14691931211196259
- Meles, A., Porzio, C., Sampagnaro, G., & Verdoliva, V. (2016). The impact of the intellectual capital efficiency on commercial banks performance: Evidence from the US. Journal of Multinational Financial Management, 36, 64–74. https://doi.org/10.1016/j.mulfin.2016.04.003
- Mention, A. L., & Bontis, N. (2013). Intellectual capital and performance within the banking sector of Luxembourg and Belgium. Journal of Intellectual Capital, 14(2), 286–309. https://doi.org/10.1108/14691931311323896
- Mondal, A., & Ghosh, S. K. (2012). Intellectual capital and financial performance of Indian banks. Journal of Intellectual Capital, 13(4), 515–530. https://doi.org/10.1108/14691931211276115
- Ng, A. W. (2006). Reporting intellectual capital flow in technology‐based companies: Case studies of Canadian wireless technology companies. Journal of Intellectual Capital, 7(4), 492–510. https://doi.org/10.1108/14691930610709130
- Ngo, T., & Le, T. (2019). Capital market development and bank efficiency: A cross-country analysis. International Journal of Managerial Finance, 15(4), 478–491. https://doi.org/10.1108/IJMF-02-2018-0048
- Ngo, T., & Tripe, D. (2017). Measuring efficiency of Vietnamese banks: Accounting for nonperforming loans in a single-step stochastic cost frontier analysis. Pacific Accounting Review, 29(2), 171–182. https://doi.org/10.1108/PAR-06-2016-0064
- Nguyen, D. T., Le, T. D., & Ho, T. H. (2021). Intellectual capital and bank risk in Vietnam: A quantile regression approach. Journal of Risk and Financial Management, 14(1), 1–15. https://doi.org/10.3390/jrfm14010027
- Onumah, J. M., & Duho, K. C. T. (2020). Impact of intellectual capital on bank efficiency in emerging markets: Evidence from Ghana. International Journal of Banking, Accounting and Finance, 11(4), 435–460. https://doi.org/10.1504/IJBAAF.2020.110303
- Pendo, K. S. (2020). Does investing in intellectual capital improve financial performance? Panel evidence from firms listed in Tanzania DSE. Cogent Economics & Finance, 8(1), 1802815. https://doi.org/10.1080/23322039.2020.1802815
- Peng, J.-L., Jeng, V., Wang, J. L., & Chen, Y.-C. (2017). The impact of bancassurance on efficiency and profitability of banks: Evidence from the banking industry in Taiwan. Journal of Banking & Finance, 80, 1–13. https://doi.org/10.1016/j.jbankfin.2017.03.013
- Perry, P. (1992). Do banks gain or lose from inflation? Journal of Retail Banking, 14(2), 25–31.
- Petty, R., & Guthrie, J. (2000). Intellectual capital literature review. Journal of Intellectual Capital, 1(2), 155–176. https://doi.org/10.1108/14691930010348731
- Phan, H. T. M., Daly, K., & Akhter, S. (2016). Bank efficiency in emerging Asian countries. Research in International Business and Finance, 38, 517–530. https://doi.org/10.1016/j.ribaf.2016.07.012
- Poh, L. T., Kilicman, A., Ibrahim, S. N. I., & McMillan, D. (2018). On intellectual capital and financial performances of banks in Malaysia. Cogent Economics & Finance, 6(1), 1453574. https://doi.org/10.1080/23322039.2018.1453574
- Pulic, A. (2004). Intellectual capital–does it create or destroy value? Measuring Business Excellence, 8(1), 62–68. https://doi.org/10.1108/MF-08-2014-0211
- Rehman, S. U., Ashfaq, K., Bresciani, S., Giacosa, E., & Mueller, J. (2021). Nexus among intellectual capital, interorganizational learning, industrial internet of things technology and innovation performance: A resource-based perspective. Journal of Intellectual Capital. https://doi.org/10.1108/JIC-03-2021-0095
- Rehman, S. U., Bresciani, S., Ashfaq, K., & Alam, G. M. (2021). Intellectual capital, knowledge management and competitive advantage: A resource orchestration perspective. Journal of Knowledge Management. https://doi.org/10.1108/JKM-06-2021-0453
- Rehman, S. U., Elrehail, H., Alsaad, A., & Bhatti, A. (2021). Intellectual capital and innovative performance: A mediation-moderation perspective. Journal of Intellectual Capital. https://doi.org/10.1108/JIC-04-2020-0109
- Riahi‐Belkaoui, A. (2003). Intellectual capital and firm performance of US multinational firms: A study of the resource‐based and stakeholder views. Journal of Intellectual Capital, 4(2), 215–226. https://doi.org/10.1108/14691930310472839
- Saint‐Onge, H. (1996). Tacit knowledge the key to the strategic alignment of intellectual capital. Planning Review, 24(2), 10–16. https://doi.org/10.1108/eb054547
- Sannino, G., Nicolò, G., & Zampone, G. (2021). The impact of intellectual capital on bank performance during and after the NPLs crisis: Evidence from Italian banks. International Journal of Applied Decision Sciences, 14(4), 419–442. https://doi.org/10.1504/IJADS.2021.115997
- Saona, P. (2016). Intra-and extra-bank determinants of Latin American Banks’ profitability. International Review of Economics & Finance, 45, 197–214. https://doi.org/10.1016/j.iref.2016.06.004
- Sealey, C. W., Jr, & Lindley, J. T. (1977). Inputs, outputs, and a theory of production and cost at depository financial institutions. The Journal of Finance, 32(4), 1251–1266. https://doi.org/10.2307/2326527
- Shaban, M., & James, G. A. (2018). The effects of ownership change on bank performance and risk exposure: Evidence from Indonesia. Journal of Banking & Finance, 88, 483–497. https://doi.org/10.1016/j.jbankfin.2017.02.002
- Sharma, P., Gounder, N., & Xiang, D. (2013). Foreign banks, profits, market power and efficiency in PICs: Some evidence from Fiji. Applied Financial Economics, 23(22), 1733–1744. https://doi.org/10.1080/09603107.2013.848026
- Shiu, H.-J. (2006). The application of the value added intellectual coefficient to measure corporate performance: Evidence from technological firms. International Journal of Management, 23(2), 356–365.
- Singh, S., Sidhu, J., Joshi, M., & Kansal, M. (2016). Measuring intellectual capital performance of Indian banks: A public and private sector comparison. Managerial Finance, 42(7), 635–655. https://doi.org/10.1108/MF-08-2014-0211
- Smriti, N., & Das, N. (2018). The impact of intellectual capital on firm performance: A study of Indian firms listed in COSPI. Journal of Intellectual Capital, 19(5), 935–964. https://doi.org/10.1108/JIC-11-2017-0156
- Ståhle, P., Ståhle, S., & Aho, S. (2011). Value added intellectual coefficient (VAIC): A critical analysis. Journal of Intellectual Capital, 12(4), 531–551. https://doi.org/10.1108/14691931111181715
- Stewart, T. A. (2007). The wealth of knowledge: Intellectual capital and the twenty-first century organization. Currency.
- Sufian, F., & Noor, M. A. N. M. (2009). The determinants of Islamic banks’ efficiency changes: Empirical evidence from the MENA and Asian banking sectors. International Journal of Islamic and Middle Eastern Finance and Management, 2(2), 120–138. http://dx.doi.org/10.1108/17538390910965149
- Sullivan, P. H. (2000). Value driven intellectual capital: How to convert intangible corporate assets into market value. John Wiley & Sons, Inc.
- Sveiby, K. E. (1997). The new organizational wealth: Managing & measuring knowledge-based assets. Berrett-Koehler Publishers.
- Tandon, K., Purohit, H., & Tandon, D. (2016). Measuring intellectual capital and its impact on financial performance: Empirical evidence from CNX nifty companies. Global Business Review, 17(4), 980–997. https://doi.org/10.1177/0972150916645703
- Tan, H. P., Plowman, D., & Hancock, P. (2007). Intellectual capital and financial returns of companies. Journal of Intellectual Capital, 8(1), 76–95. https://doi.org/10.1108/14691930710715079
- Ting, I. W. K., Chen, F.-C., Kweh, Q. L., Sui, H. J., & Le, H. T. M. (2021). Intellectual capital and bank branches’ efficiency: An integrated study. Journal of Intellectual Capital. https://doi.org/10.1108/JIC-07-2020-0245
- Tiwari, R., & Vidyarthi, H. (2018). Intellectual capital and corporate performance: A case of Indian banks. Journal of Accounting in Emerging Economies, 8(1), 84–105. https://doi.org/10.1108/JAEE-07-2016-0067
- Tovstiga, G., Tulugurova, E., & Bontis, N. (2007). Intellectual capital practices and performance in Russian enterprises. Journal of Intellectual Capital, 8(4), 695–707. https://doi.org/10.1108/14691930710830846
- Tran, D. B., & Vo, D. H. (2018). Should bankers be concerned with Intellectual capital? A study of the Thai banking sector. Journal of Intellectual Capital, 19(5), 897–914. https://doi.org/10.1108/JIC-12-2017-0185
- Vidyarthi, H. (2019). Dynamics of intellectual capitals and bank efficiency in India. The Service Industries Journal, 39(1), 1–24. https://doi.org/10.1080/02642069.2018.1435641
- Vidyarthi, H., & Tiwari, R. (2019). Cost, revenue, and profit efficiency characteristics, and intellectual capital in Indian Banks. Journal of Intellectual Capital, 21(1), 1–22. https://doi.org/10.1108/JIC-05-2019-0107
- Vu, H., & Nahm, D. (2013). The determinants of profit efficiency of banks in Vietnam. Journal of the Asia Pacific Economy, 18(4), 615–631. https://doi.org/10.1080/13547860.2013.803847
- Wang, W. Y., Chang, C., & Bontis, N. (2005). Intellectual capital and performance in causal models: Evidence from the information technology industry in Taiwan. Journal of Intellectual Capital, 6(2), 222–236. https://doi.org/10.1108/14691930510592816
- Weill, L. (2004). Measuring cost efficiency in European banking: A comparison of frontier techniques. Journal of Productivity Analysis, 21(2), 133–152. https://doi.org/10.1023/B:PROD.0000016869.09423.0c
- Williams, J., Peypoch, N., & Barros, C. P. (2011). The Luenberger indicator and productivity growth: A note on the European savings banks sector. Applied Economics, 43(6), 747–755. https://doi.org/10.1080/00036840802599859
- Xu, J., & Li, J. (2019). The impact of intellectual capital on SMEs’ performance in China: Empirical evidence from non-high-tech vs. high-tech SMEs. Journal of Intellectual Capital, 20(4), 488–509. https://doi.org/10.1108/JIC-04-2018-0074
- Youndt, M. A., Subramaniam, M., & Snell, S. A. (2004). Intellectual capital profiles: An examination of investments and returns. Journal of Management Studies, 41(2), 335–361. https://doi.org/10.1111/j.1467-6486.2004.00435.x
- Zeghal, D., & Maaloul, A. (2010). Analysing value added as an indicator of intellectual capital and its consequences on company performance. Journal of Intellectual Capital, 11(1), 39–60. https://doi.org/10.1108/14691931011013325
Appendices
Table A1. Variables’ measuring used in the efficiency estimation
Table A2. The VIFs statistics result
Table A3. The average efficiency scores 2007–2019