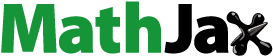
Abstract
Poverty reduction has become a top goal in the socio-economic development strategies of countries and a topic of interest for researchers. A number of prior empirical studies have identified the importance of financial inclusion to poverty reduction. However, most of these studies assess the effect of financial inclusion on income poverty reduction. There are relatively few studies evaluating the impact of financial inclusion, especially in terms of household use of financial products and services, on multidimensional poverty reduction. This study uses a multivariate probit model with a dataset from Vietnam to estimate the effect of financial inclusion in terms of household use of financial products and services and other factors on multidimensional poverty. The results show that financial inclusion reduces multidimensional poverty. Specifically, households owning bank accounts, having savings at banks, using debit cards, credit cards, or investing in stocks or bonds are less likely to fall into multidimensional poverty. Based on the findings, we provide several recommendations for policymakers to increase the level of households’ use of financial products and services.
1. Introduction
Prior studies have examined various factors affecting poverty reduction, in which financial inclusion (FI) has been identified as an important driver of poverty alleviation (Álvarez-Gamboa et al., Citation2021; Churchill & Marisetty, Citation2020; Park & Mercado, Citation2018; Sethi & Acharya, Citation2018; Yang & Fu, Citation2019). However, there are not many studies investigating the impact of FI on multidimensional poverty reduction using household-level data. Therefore, we conduct this study to explore the impact of FI in terms of household use of financial products and services on multidimensional poverty in Vietnam.
Poverty is a global problem, attracting the attention of scholars and countries. In Vietnam, poverty reduction has always been considered as one of the key tasks in the socio-economic development strategies of the Government. The Government of Vietnam has implemented a number of programs to reduce poverty, such as the Vietnam Sustainable Development Strategy for 2011–2020, The 2030 Agenda for Sustainable Development, the Project on Vocational training for rural workers up to 2020, the Project on developing the microfinance system in Vietnam until 2020, vocational training policies for rural workers … The living standard of Vietnamese people has improved significantly thanks to these efforts. According to the General Statistics of Vietnam (2022), the rate of multidimensionally poor households in Vietnam decreased significantly, from 9.2% in 2016 to 4.8% in 2020. However, the poverty reduction rate is still low compared to the targets set out by the Government. According to the National Target Program on Sustainable Poverty Reduction and Social Security for the period from 2021 to 2025 of the Government of Vietnam, the multidimensional poverty rate of the country should be reduced by at least 25% by 2025, and the poverty reduction rate should be maintained at 1–1.5% per annum. However, the actual reductions were 1.3%, 1.1% and 0.9% in 2017, 2018 and 2020, respectively. Thus, although certain successes have been achieved, the rate of poverty reduction is still under expectation. In order to help the country accelerate poverty reduction, it is important to identify the factors that promote poverty reduction.
FI is concerned with the affordable provision of financial services, ensuring access to the right financial products and services for the disadvantaged and low-income people (Dixit & Ghosh, Citation2013; Evans & Alenoghena, Citation2017; Kumar, Citation2013; Mohseni-Cheraghlou, Citation2015). In recent years, FI has received global attention in its mission to eliminate hunger and reduce poverty to build a stable and equitable society where everyone enjoys economic development achievements.
Recognizing the importance of FI to poverty reduction, the Government of Vietnam has issued a number of policies to promote the development of FI, typically Decision No. 1726/QD-TTg dated 5 September 2016 of the Prime Minister approving the project to improve accessibility to banking services for the economy (Vietnam Prime Minister, Citation2016), Decision No. 241/QD-TTg dated 23 February 2018 of the Prime Minister approving the scheme to promote payments through banks for public services (Vietnam Prime Minister, Citation2018), and Decision No. 149/QD-TTg dated 20 January 2020 of the Prime Minister approving the financial inclusion strategy to the year 2025, and orientations to the year 2030 (Vietnam Prime Minister, Citation2020). Thanks to these efforts, access to financial services in Vietnam has achieved positive results. According to the State Bank of Vietnam (Citation2021a), compared to 2016, in 2020 the number of commercial bank branches increased by 10%, equivalent to 1,009 branches; the number of automatic teller machines (ATMs) increased by 12.39%, equivalent to 2,164 ATMs. In addition, bank branches or official credit funds are available in 20.1% of the communes in rural areas. The usage level of financial products and services also increased sharply. According to the State Bank of Vietnam’s (Citation2021a) supply-side data, by the end of 2020, the number of individual accounts reached 100.4 million, which increased by 66.8% compared to that in 2015. Furthermore, the total number of bank cards in circulation reached 112.5 million, an increase of 58.5% compared to 2015. The question of interest is whether the continuous efforts in promoting FI contribute to poverty reduction in Vietnam? Therefore, it is necessary to examine the impact of FI on multidimensional poverty in Vietnam.
Prior studies have documented the importance of FI to the reduction of income-based poverty (Beck et al., Citation2007; Burgess & Pande, Citation2005; Sethi & Acharya, Citation2018; Tran & Le, Citation2021). However, income is not a comprehensive measure of poverty, because it does not reflect the lack of various basic human needs, especially those that cannot be bought with money. In some cases, a person may not be poor in terms of income, but has difficulties accessing basic health, education, and information services. Therefore, it is necessary to consider the impact of FI on various poverty dimensions.
To fill this gap, some recent studies have addressed the importance of FI to multidimensional poverty reduction, such as Yang and Fu (Citation2019), Churchill and Marisetty (Citation2020), and Álvarez-Gamboa et al. (Citation2021). However, they are still quite few, and there are still certain limitations to these studies. Specifically, Yang and Fu (Citation2019) examine the role of FI on multidimensional poverty in rural China only in terms of access to FI. Churchill and Marisetty (Citation2020) investigate the role of FI in India on three dimensions of FI only, including access to banking services, access to loans and access to insurance. This study also considers only three aspects of multidimensional poverty, which are health, education, and living standards. Other aspects of multidimensional poverty such as access to information, clean water, and sanitation have not been taken into account. Álvarez-Gamboa et al. (Citation2021) examine the influence of FI from a macro perspective based on the level of access and usage of financial products on Ecuador’s multidimensional poverty reduction. Thus, there is still little evidence on the impact of FI in terms of the use of financial products and services on multidimensional poverty reduction at the household level. Our study adds to the current literature by investigating the role of FI using household-level data on multidimensional poverty. We also provide evidence of the impact of households’ participation in financial markets on poverty reduction.
Our study contributes to the current literature in several aspects. Firstly, this study provides further evidence of the role of FI in reducing multidimensional poverty in a developing country. Secondly, this study examines the role of FI in reducing multidimensional poverty in terms of household use of financial products and services. Furthermore, this study also adds evidence of the role of FI in terms of household participation in financial markets in multidimensional poverty reduction, particularly households with investments in stocks or bonds.
We structure our paper as follows: Section 2 covers the literature, Section 3 discusses the methodologies and data, Section 4 shows the results and discussions, and Section 5 concludes the paper.
2. Literature review
FI is an issue of interest around the world because of its economic and social benefits to the poor in particular and to the sustainable economic development of countries in general. FI receives a great deal of attention from the public and researchers in the early 2000s, stemming from a study showing that poverty is related to financial exclusion (Babajide et al., Citation2015). FI refers to the provision of financial services at a reasonable cost to individuals, especially the disadvantaged and low-income people (Evans & Alenoghena, Citation2017; Mohseni-Cheraghlou, Citation2015). These services include access to an account at a financial institution, mobile payments, savings, credit and insurance products (Allen et al., Citation2016; Demirgüç-Kunt & Klapper, Citation2012; Mohseni-Cheraghlou, Citation2015). An FI system maximizes the use and access to financial services, while minimizing involuntary financial exclusion (Cámara & Tuesta, Citation2014). Without the appropriate provision of financial services, individuals may turn to high-cost informal financial sources. Thus, promoting FI plays an important role in alleviating poverty and reducing income inequality in a country.
Studies on the impact of FI on poverty reduction are approached from both macro level (nations or regions) and micro level (households). From a macro level perspective, Chithra and Selvam (Citation2013) argue that FI is necessary for poverty alleviation because it gives people access to funds for setting up businesses, investing in production and business activities, thereby increasing their income levels. Park and Mercado (Citation2018) also suggest that FI reduces poverty, because when more people have access to financial services, they can participate in business activities. Khan et al. (Citation2022) examine the impact of FI on poverty, income inequality and financial stability using unbalanced data of 54 African countries in the period from 2001 to 2019. The results show that FI reduces poverty, income inequality and improves financial stability.
Sethi and Acharya (Citation2018) examine the effects of FI on the standard of living of people across 31 countries. The results show that providing affordable financial services improves the income of the poor. For low-income groups, the provision of low-cost credit stimulates people to take out loans and organize their own production activities, thus increasing production and the number of jobs. The impact of FI on the agricultural sector is obvious. FI helps farmers to apply new technology to increase productivity and outputs, leading to general economic growth. Kelkar (Citation2010) shows that improving access to finance significantly reduces the indebtedness of farmers. Inoue (Citation2011) indicates that the lack of access to formal financial services including loans, savings, insurance, and payments causes individuals to rely on informal sources of finance, which leads to potentially high cost burdens for those with low incomes. Tran and Le (Citation2021) examine the impact of FI on poverty measured by income in 28 European countries. The results show that FI has a negative impact on all three poverty lines of 1.9, 3.2 and 5 USD per day.
FI provides an opportunity for everyone to get access to formal banking services with certain benefits such as confidentiality and better control on spending and cash management. These benefits motivate people to put their savings into the financial system instead of using unofficial saving methods, such as keeping money at home, or storing gold, silver, and jewelry (Demirgüç-Kunt & Singer, Citation2017). These savings generate sources of funds for the financial market. The financial market will ensure the efficient allocation of these funds to long-term investment projects. In this way, financial markets reduce liquidity risk—the risk caused by a lack of capital flowing into the market and encourage more investment (Sethi & Acharya, Citation2018).
From a micro level perspective, Koomson et al. (Citation2020) examine the impact of FI on poverty and poverty vulnerability of Ghanaian households with a dataset from the Living Standards Survey of Ghana in 2016 and 2017. The results show that increased FI has two effects on household poverty. First, it is associated with a 27% reduction in a household’s likelihood of poverty; second, it prevents a household from experiencing future poverty by 28%. Women-headed households have a greater opportunity to reduce poverty through improved FI than male-headed households. Moreover, FI reduces poverty and vulnerability more in rural areas than in urban areas. Dogan et al. (Citation2022) employ logistic regression using data from Turkish Household Budget and Consumption Expenditure Survey to analyze the impact of FI on three income-based poverty measures. The results show that an increase in FI leads to a decrease in poverty. Therefore, policies should be implemented to improve FI of households.
Thus, there have been a number of studies exploring the impact of FI on poverty in terms of income. However, the income measure has not yet reflected the lack of various essential needs in life, especially those that cannot be bought with money and those that cannot be replaced.
The question of interest is whether FI reduces poverty when the poor are in multidimensional poverty? To answer this question, Yang and Fu (Citation2019) build an evolutionary game model to examine the equilibrium strategies of financial institutions and the low-income people in poverty reduction in China. Due to a high poverty rate and severe financial exclusion in China’s rural areas, the authors examine the poverty reduction effect of FI on the poor with different labor capacity in 21 rural Chinese provinces from 2010 to 2016 based on China Family Panel’s survey. In this study, the multidimensional poverty index of the working-age population in rural areas is calculated according to the “dual cutoff method” of Alkire and Foster with five dimensions: income, health, education, insurance and employment. The authors also use control variables representing individual characteristics such as gender, age, household registration, marital status and household characteristics such as household size, rate of family burden … The results show that there is a difference in the poverty reduction effect of FI among the poor with different working capacities. If financial institutions provide loans to the working-age population, they can achieve both the objectives of sustainable development and poverty alleviation. And FI development in terms of utility and usability can significantly decrease multidimensional poverty. The authors also show that the influence and the marginal impact of gender on poverty of the rural population are significantly negative, reflected in the fact that men are less likely to be multidimensionally poor than women, other things being equal. The coefficient and marginal impact of marital status are positive, indicating that people who are unmarried, cohabiting, divorced, and widowed are more likely to fall into poverty than the married population. The coefficient and marginal impact of household registration are positive, indicating that it is more likely for rural residents to fall into poverty than those without rural household registration. The coefficient and marginal effect of household size and the correlation between family burden and multidimensional poverty are negative, implying that rural populations with lower family burdens are more likely to escape poverty, and rural populations with higher family burdens are more likely to be multidimensionally poor.
Álvarez-Gamboa et al. (Citation2021) examine the effect of FI on multidimensional poverty in Ecuador’s provinces in the period from 2015 to 2018. To assess the effect of FI on multidimensional poverty, the authors use principal component analysis (CPA) recommended by Cámara and Tuesta (Citation2014) to develop the FI index based on two dimensions: access and usage. Access is reflected by two indicators: the first is the geographical accessibility of financial institutions (headquarters, branches, agents, ATMs), non-banking correspondents (NBC), points of sale (POS) per 1,000 square kilometers; The second is demographic access, which measures service points per 10,000 adults. Usage is measured by how frequently and intensively the users use financial services. In the study, the multidimensional poverty rate is built based on people’s living conditions through 12 indicators: (1) No elementary and high school attendance, (2) Lack of access to higher education for economic reasons, (3) Failure to complete an educational program, (4) Employment for children and young people, (5) Unemployment or underemployment, (6) No allocation of the pension system. (7) Extreme income poverty, (8) Lack of public water network service, (9) Overcrowding, (10) Lack of housing, (11) No drainage and (12) Lack of garbage collection service. The poverty index is the proportion of people deprived in at least one third of the weighted indicators (k ≥ 33.3%), where k is the poverty line representing the level of deprivation from the simultaneous assessment of the above indicators. The control variables are the population working in the main sectors and the proportion of the population aged 15–49 years who do not know how to use information devices (satisfying three characteristics simultaneously: (1) no use of mobile phones, (2) no use of computers in the last 12 months, (3) no use of the Internet in the last 12 months). In addition, the spatial variables used in this study include population density and the distance from each province to the center of the economic area that the province is integrated into. Moreover, using SARAR-type spatial panel data models, the space is used as an explanatory variable to determine the existence of inter-provincial spatial effects. The results show a strong negative relation between FI and multidimensional poverty. Individual estimates for FI indicators show that the existence of financial institutions, as measured by the financial access index, is a driver of poverty reduction. On the opposite side, the authors argue that the use of financial services is not significantly important because it is related to the low financial literacy of the people of Ecuador, where 9 out of 10 people never studied finance. However, its negative effect on poverty reduction is clear. Overall, FI is a useful tool for economic development and multidimensional poverty reduction in Ecuador.
Churchill and Marisetty (Citation2020) use survey data of 45,000 Indian households to examine the impact of FI on multidimensional poverty. In their study, FI is measured on three dimensions: access to banking services, access to loans/credit and access to insurance. Multidimensional poverty is measured using three proxies: household deprivation score based on the multidimensional poverty index (MPI) approach (Alkire & Santos, Citation2010), household poverty probability (PPI index) and a binary variable reflecting households below (and above) the multidimensional poverty line. In particular, the MPI is calculated based on three household characteristics including health, education, and standard of living, and applying equal weights of 1/3 for each dimension. Some control variables are used in the model such as age, sex, marital status, family size, education, property ownership, employment, religion, living area and the number of children in the household. The results show that FI contributes to multidimensional poverty reduction. Each aspect of FI also helps reduce multidimensional poverty, in which access to insurance has the strongest impact, followed by access to banking services and, finally, access to credit. Since households with access to credit also have access to banking services, there may be a high correlation between these two dimensions. Therefore, the authors add a further test that removes access to banking services and includes only access to credit and insurance in the FI index. The results continue to show the important role of insurance in reducing multidimensional poverty. This finding can be explained by the fact that poor households are often financially excluded and thus do not have strategies to deal with income shocks (Zhang & Posso, Citation2017), and this worsens the poverty problem. However, with FI, especially access to insurance, their resilience to such income shocks will increase that provides an opportunity for them to escape poverty. In addition to insurance, the impact of bank account access suggests that increasing access to various bank accounts empowers poor households and encourages them to invest in human capital, which in turn helps reduce poverty (Ashraf et al., Citation2010). Similarly, the effect of credit can be explained by the literature regarding access to credit to increase income, health, consumption and earning opportunities, which then leads to poverty reduction (Babajide et al., Citation2015). Based on the above findings, Churchill and Marisetty (Citation2020) suggest that promoting FI is a possible solution to reduce multidimensional poverty in India. Policymakers should consider the promotion of FI as a means of eradicating hunger, reducing poverty and improving social welfare.
To assess the impact of credit on multidimensional poverty of poor farmers in Indonesia, Nuryitmawan (Citation2021) uses propensity score matching method with a dataset collected from the Family Living Standards Survey in Indonesia in 2007 and 2014. The results show that credit programs for poor farmers initiated by credit institutions have helped farmers out of poverty significantly. Besides, the study also adds some control variables such as education, household property ownership and agricultural land ownership. The results show that education provides three times greater opportunity to help poor farmers escape poverty. Meanwhile, household property ownership helps them escape poverty 1.4 times faster. This means that in order to eliminate hunger and reduce multidimensional poverty among farming households, besides providing credit, more attention must be paid to education and asset sufficiency of the households.
Besides FI, a number of studies have shown the influence of other control variables on multidimensional poverty. Betti et al. (Citation2002) examine the factors influencing multidimensional poverty based on UK Household Survey data from 1991 to 1997. In the study, the dependent variable is the logit of the multidimensional poverty index of household i at time t, which is measured by the weighted sum of the relative deprivation of each poverty indicator. The explanatory variables in the model include those representing household characteristics such as gender, age, occupation, education, marital status of the household head, geographic region, and household size. The parameters are estimated using Marginal Maximum Likelihood. The results show that except for unemployment, the remaining variables all affect the multidimensional poverty index. Ningaye et al. (Citation2011) explore multidimensional, unidimensional poverty status and the determinants of multidimensional poverty in Cameroon using logistic regression with the ECAM II survey dataset of the Cameroon Statistical Institute in 2001. In the study, the dependent variable is a binary variable representing multidimensional poverty. The results show that the living area significantly affects multidimensionally poverty, but household size and gender of the household head do not. Tran et al. (Citation2015) examine the determinants of income poverty and multidimensional poverty based on the VHLSS datasets in 2007, 2008 and 2010. The findings show that household size and multidimensional poverty have a convex function; the multidimensional poverty rate decreases significantly when the education level of the household head increases. The study also shows that there is a difference in multidimensional poverty levels among different ethnic groups. Alkire et al. (Citation2015) investigate the influence of household heads’ education and gender, the household size, and religion on multidimensional poverty using a binary logistics model. In this study, multidimensional poverty is a binary variable, taking the value of 1 if a household is multidimensionally poor (ci≥ k = 33.3%;) and 0 if otherwise. They document that except for religion, the remaining variables are statistically significant at the 5% level. Le and Nguyen (Citation2018) investigate the determinants of multidimensional poverty in Vietnam using the binary regression method with the 2014 VHLSS data. The results show that ethnicity and demographic factors such as the number of years of schooling, qualifications, the age and the area of residence of the household head have an influence on multidimensional poverty.
Alimi and Okunade (Citation2020) assess the role of FI and information and communication technologies (ICT) in poverty reduction in 27 Sub-Saharan African (SSA) countries between 2004 and 2017. The results indicate that FI and ICT dissemination are crucial in reducing poverty in SSA countries. Therefore, to reduce poverty in the SSA region, governments should strengthen financial inclusion strategies in conjunction with the latest ICT infrastructure development, including the use of the Internet. Ajisafe et al. (Citation2018) examine the impact of monetary policy and financial inclusion on poverty in Nigeria between 1986 and 2015. The results show that FI and monetary policy have an impact on poverty levels depending on how FI or monetary policy is measured. The results also show that only FI supported by loans and advances to small and medium enterprises by bank deposits has the desired effect on poverty levels, while the increase in deposits in rural bank branches impoverishes the poor in rural areas.
Based on the analysis and discussions from Sarma (Citation2008), Chithra and Selvam (Citation2013), Sethi and Acharya (Citation2018), Park and Mercado (Citation2018), Churchill and Marisetty (Citation2020), Koomson et al. (Citation2020), Alimi and Okunade (Citation2020), and Nuryitmawan (Citation2021), we construct Diagram 1 presenting the framework of the role of FI in poverty reduction.
Diagram 1. The role of FI in poverty reduction.
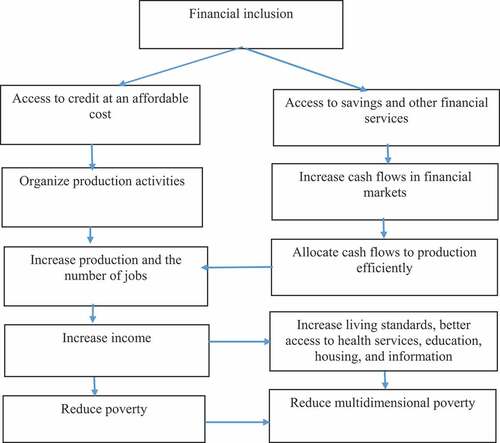
From a review of the literature, it can be seen that there is a link between FI and poverty reduction. However, the impact of FI on multidimensional poverty has not been investigated extensively. Furthermore, there are certain limitations in the prior studies in terms of the level of data and the measurement of FI and poverty. Some recent studies on the effects of FI on multidimensional poverty such as Yang and Fu (Citation2019) and Álvarez-Gamboa et al. (Citation2021) only examine the impact of FI on multidimensional poverty reduction from a macro perspective. Churchill and Marisetty (Citation2020) investigate the role of FI using household-level data. However, this study only considers three aspects of multidimensional poverty, which are health, education, and living standards. Other aspects of multidimensional poverty have not been taken into account. Thus, there is relatively little evidence of the impact of FI on multidimensional poverty in terms of the use of financial products and services at the household micro-level.
In this study, we expect to find more evidence of the effect of FI and other factors on multidimensional poverty using household data. Based on the results from the prior studies, we expect that FI reduces multidimensional poverty. Thus, our hypothesis is stated as follows:
H1: There is a negative relation between financial inclusion and multidimensional poverty in Vietnam
3. Research methods and data sample
3.1. Data source
We use the data collected from the VHLSS conducted by the General Statistics Office of Vietnam in 2016 and 2018. Vietnam started to acknowledge and collect information on multidimensional poverty in 2016, and the VHLSS is a rotating panel data. Households selected in the VHLSS survey sample for the period 2010–2018 were designed from the 2009–2010 Vietnam Population and Housing Census, while the survey sample in the VHLSS 2020 was designed from the 2019 Vietnam Population and Housing Census, so we could only create panels from VHLSS 2016 and 2018. The survey sample includes 9,399 households in 2016, and 9,396 households in 2018, of which 4,200 households were surveyed in 2016. After excluding observations with missing values for any relevant variables, our final sample consists of 2,760 households.
3.2. Variable measurement
To assess the impact of FI on multidimensional poverty, we propose a research model with the dependent variable being multidimensional poor households (MPH). The independent variables reflecting FI include five variables, representing three aspects of FI: 1) Financial services (households with a bank account (BAC), households with bank savings (SAV), and households using an ATM card (ATM)); 2) Credit (Households using a credit card (CRE)); 3) Participating in financial markets (Households with assets such as stocks or bonds (STOCK)).
The control variables used in the model include seven variables reflecting the characteristics of the household or household head: the household area of residence (ARE), household size (HSIZE), access to production and business information (INF), gender of the household head (SEX), age of the household head (AGE), marital status of the household head (MAR) and education of the household head (HEDU). The instrumental variable used in the model is the variable representing whether a household has a plot of land or a house other than its current residence (EST).
3.2.1. Measurement of multidimensional poor households (MPH)
According to Decision No. 59/2015/QD-TTg of the Prime Minister of Vietnam (Vietnam Prime Minister, Citation2015), the multidimensional poverty index in Vietnam in the period from 2016 to 2020 is calculated according to the Alkire-Foster method, which includes five dimensions: education, medical services, housing, clean water and sanitation, and access to information. Each dimension consists of two indicators. The deprivation threshold of each component indicator (first cutoff point) is presented in Table .
Table 1. Multidimensional poverty measurement
Each dimension has equal weight, and the indicators in each dimension also have equal weight. So each indicator has a weight of 1/10. The deprivation threshold for basic social services or the threshold of multidimensional deprivation (second cut-off point) in Vietnam for the period 2016–2020 is set at 3. Any household depriving at least three out of ten indicators is considered as lacking access to basic social services or multidimensionally poor.
In addition, according to Decision No. 59/2015/QD-TTg of the Prime Minister of Vietnam (Vietnam Prime Minister, Citation2015), multidimensional poor households are determined as follows: (1) In the urban area, a poor household is one meeting one of the following two criteria: having average income per capita per month of VND900,000 or less; or having average income per capita per month from VND900,000 to VND1,300,000 and depriving at least three indicators measuring the lack of access to social services. (2) In the rural area, a poor household is one meeting one of the following two criteria: having average income per capita per month of VND700,000 or less; or having average income per capita per month from VND700,000 to VND1,000,000 and depriving at least three indicators measuring the lack of access to social services.
3.2.2. Measurement of financial inclusion
BAC and SAV reflect whether a household has a bank account or savings in the past 12 months; ATM and CRE reflect whether a household has used ATM or credit card services in the past 12 months. STOCK reflects whether a household has invested in stocks or bonds in the past 12 months. The expected sign of BAC, SAV, ATM, CRE and STOCK is negative, meaning that FI reduces a household’s probability of falling into multidimensional poverty.
EST reflects whether a household has a plot of land or other housing in addition to the current residence. ARE indicates a household’s area of residence, which is determined according to the List of administrative units of Vietnam in 2015. Accordingly, households living in wards/towns are classified as urban areas; Households living in communes are classified as rural areas. HSIZE is the number of members of a household. INF shows whether a household received production and business information from the commune radio system in the past 30 days. SEX represents the gender of the household head. AGE is the age of the household head. MAR reflects the marital status of the household head, which is determined as whether the household head has a spouse who is recognized by law or custom, or living with another person of the opposite sex as husband and wife; or whether the household head is unmarried, widowed, divorced or separated. The education level of the household head (HEDU) represents the highest degree achieved by the household head, classified into the following groups: no degree, primary school, secondary school, undergraduate degree or postgraduate degree.
We show the measurements of the variables in Table .
Table 2. Measurements of the variables
3.3. Research model
Since multidimensional poverty (MPH) is a binary variable, we use a binary logistic regression model to assess the impact of FI on multidimensional poverty. Logistic models are developed to estimate event probability based on univariate or multivariate regressions (Walker & Duncan, Citation1967). The logistic model is estimated using the Maximum Likelihood Estimation method. The binary logistic regression model has been used by many researchers to study the effects of FI on poverty reduction, typically those of Asante (Citation2018), Churchill and Marisetty (Citation2020), and Chhorn (Citation2021). Besides, according to Dartanto and Nurkholis (Citation2013), the logistic model is very useful in assessing the influence of households’ demographic characteristics on the poverty status of the households. This method is also used by Betti et al. (Citation2002), Ningaye et al. (Citation2011), and Tran et al. (Citation2015) to examine the effect of households’ demographic characteristics on multidimensional poverty.
FI variables are likely to be endogenous in the model of the impact of FI on poverty reduction. A number of studies have documented that FI plays an important role in poverty reduction (Burgess & Pande, Citation2005; Beck et al., Citation2007a; Sethi & Acharya, Citation2018; Tran & Le, Citation2021; Churchill & Marisetty, Citation2020; Nuryitmawan, Citation2021; Álvarez-Gamboa et al., Citation2021). On the other hand, several previous studies have shown poverty leads to financial exclusion (Babajide et al., Citation2015; Yang & Fu, Citation2019; Zhang & Posso, Citation2017). Therefore, to control the endogeneity problem, we use a regression model with an instrumental variable. This method is also employed by some researchers in investigating the impact of FI on poverty reduction in other countries (Chhorn, Citation2021; Geda et al., Citation2008; Koomson et al., Citation2020). In this study, we use real estate, which is households with a plot of land or a house other than their current residence, as an instrumental variable. Real estate is considered a reliable collateral, so it is an important factor in the credit decision-making of a credit institution. Zhu and De’Armond (Citation2005) also document that real estate and housing of households have an influence on the household’s ability to access credit. We also find a low correlation (6.4%) between real estate and multidimensional poverty in our study. Therefore, real estate can satisfy the requirement of an instrumental variable.
The dependent variable (MPH) and FI variables are binary, so we use the multivariate probit (Mvprobit) model proposed by Cappellari and Jenkins (Citation2003) to deal with the endogenous problem. In addition, a number of previous studies (Kim & DeVaney, Citation2001; Sanya & Olumide, Citation2017; Zhu & De’Armond, Citation2005) point out that some determinants of poverty and FI such as age, marital status, education, gender, income of the household head, household size, interest rates and loan terms, subsidies and household’s real estate may be related. So there could be multicollinearity between FI and the control variables in our model. Therefore, we use the Mvprobit model with robust standard error to overcome this issue.
Based on the theories about the role of FI and other control factors on multidimensional poverty identified in prior studies such as Betti et al. (Citation2002), Ningaye et al. (Citation2011), Tran et al. (Citation2015), Churchill and Marisetty (Citation2020), and Álvarez-Gamboa et al. (Citation2021), and to overcome the endogeneity problem in the research model, we propose a regression model with the instrumental variable in two stages as follows:
Model (1) shows the relationship of real estate (EST) and other variables to FI (FI). Model (2) shows the effect of FI (FI) and other control variables on multidimensional poverty (MPH).
where MPH is multidimensional poverty, MPH is 1 if the household is multidimensionally poor, and 0 if otherwise. We propose five variables representing FI (FI) in three aspects: 1) Financial services (BAC is households with a bank account, SAV is households with bank savings; ATM is households using an ATM card); 2) Credit (CRE is households using a credit card; 3) Participating in the financial market (STOCK is households investing in stocks or bonds). The EST is real estate as the instrumental variable. The control variables in the model include seven variables reflecting the characteristics of the household or household head: the household’s area of residence (ARE), household size (HSIZE), household’s access to information on production and business (INF), the gender of the household head (SEX), the age of the household head (AGE), the marital status of the household head (MAR), education of the household head (HEDU); uit and εit are random error; i is the surveyed household, t is the year.
4. Results and discussions
4.1. Descriptive statistics of the sample
Descriptive statistics of the variables for the whole sample and for multidimensionally poor and non-poor households are presented in Table .
Table 3. Descriptive statistics of the variables
Table shows that, out of a total of 2,760 households with full survey data in 2016 and 2018, the percentage of households accessing financial products and services is still low. The highest rate is the percentage of households using ATM cards, accounting for 39.9%, in which the percentage of the non-poor group is 41.7%, and the poor group is 3.7%; Next is the percentage of households with bank accounts, accounting for 28.2%, in which the percentage of the multidimensional poor group is only 2.2%. The percentage of households with bank savings is 13.9%, in which the multidimensional poor group does not have any deposits at banks. The lowest percentage is that of households investing in stocks and bonds, accounting for 0.4%, in which no multidimensionally poor household invested in stocks. The second lowest is the proportion of households using credit cards, accounting for only 2.5%, in which the multidimensionally poor group does not use any credit card.
The percentage of households living in rural areas is much higher than in urban areas. The rate of multidimensionally poor households living in rural areas is twice as high as that of non-poor households. The percentage of households receiving production and business information from the commune/village radio system is 56.1%, in which the percentage in the non-poor group is 56.8% and the poor group is 41.5%. The percentage of female household heads is quite low and there is no significant difference in the two groups (23.2% in the non-poor group and 23.7% in the poor group). The average age of the household head in the non-poor group is higher than that of the poor group, 50.6 and 45.5 years, respectively. Most of the surveyed household heads are currently married, with 84.1% of the household heads in the non-poor group and 78.5% in the poor group.
The percentage of household heads with a high school diploma is the highest in the entire sample, accounting for 51.2% of the total sample. The percentage in the non-poor group is 52.3%, while that in the poor group is only 30.4%. The education level of household heads in the poor group is low. Specifically, 25.9% of household heads in the poor group are without a degree, which is twice as high as that of the non-poor group. The most common education level of household heads in the poor group is primary school, accounting for 43.7%. None of the household heads in that group graduated from college or university. Thus, the education level of the household heads in the multidimensionally poor group is much lower than in the non-poor groups. The percentage of households with land or other houses is quite low, accounting for only 9.9%, in which the percentage in the poor group is 1.5%.
4.2. Estimation results
We first perform the Rho test to check whether the models have endogenous problems. Table shows that all five models corresponding to five FI variables (BAC, SAV, ATM, CRE, STOCK) have p-values of less than 0.05. As such, there are endogenous problems in these models. Therefore, we use a regression model with an instrumental variable to estimate the impact of FI (BAC, SAV, ATM, CRE, STOCK) on multidimensional poverty (MPH).
Table 4. The impact of financial inclusion and control variables on multidimensional poverty in Vietnam
Next, we perform the Rho21 test to check the appropriateness of the research model. The results of Rho21 test reported in Table show that all five models have p-values of more than 0.05, indicating no endogenous problem in the Mvprobit models to estimate the influence of FI (BAC, SAV, ATM, CRE, STOCK) on MPH, and the selected instrumental variable is appropriate.
The results reported in Table show that the coefficients for BAC, SAV, ATM, CRE and STOCK are negative, implying that FI has a positive impact on multidimensional poverty reduction. Having bank accounts, savings, using ATM cards, credit cards, or investing in stocks or bonds reduce the possibility of households falling into multidimensional poverty.
Table also shows that households living in urban areas are less likely to fall into multidimensional poverty than those in rural areas; households with access to production and business information are less likely to fall into multidimensional poverty than those without access. Household size has a positive impact on multidimensional poverty. As the number of members in the household increases, the possibility of falling into multidimensional poverty also increases. As the age of the household head increases, the likelihood of the household falling into multidimensional poverty decreases; households with a spouse are less likely to fall into multidimensional poverty than other groups. The higher the education level of the household head, the lower the probability of falling into multidimensional poverty. On the other hand, there is no difference in the likelihood of falling into multidimensional poverty between men and women.
4.3. Discussions
The results of the study show that FI has contributed to reducing multidimensional poverty in Vietnam. In particular, usage of financial products and services reduces the probability of households falling into multidimensional poverty. These findings are consistent with our expectations and results from prior studies such as Demirgüç-Kunt and Singer (Citation2017), Churchill and Marisetty (Citation2020), Koomson et al. (Citation2020), Tran and Le (Citation2021), and Álvarez-Gamboa et al. (Citation2021). Access to and usage of bank accounts increases savings for farmers, leading to greater agricultural output and household spending (Demirgüç-Kunt & Singer, Citation2017), which is especially important for people living in the poorest households in rural areas. Greater access to formal financial services such as bank accounts and savings offers certain benefits such as security, spending control, and better cash management. Access to funds helps the poor in rural areas to apply new technology and processes to increase productivity in agricultural activities, thus increasing their income. The lack of access to formal financial services such as loans and savings causes individuals to use informal sources of funds, which then leads to cost burdens for them (Inoue, Citation2011). By providing access to financial services, FI increases the income of low-income groups and promotes poor households financial autonomy (Sarma & Pais, Citation2011; Sethi & Acharya, Citation2018). The provision of low-cost credit encourages the poor to take out loans and organize their own business activities, thus increasing the number of jobs and their income. The results also indicate that the efforts of the Government of Vietnam in promoting FI have contributed to reducing multidimensional poverty.
In addition, our study provides interesting evidence of the role of financial markets in multidimensional poverty reduction in Vietnam. Particularly, participating in financial markets, such as investing in stocks and bonds, reduces the probability of households falling into multidimensional poverty. The result implies that the ability to participate in financial markets creates an opportunity for poor households to invest their money in more lucrative assets than traditional savings products.
In recent years, Vietnam has made remarkable achievements in promoting FI and reducing poverty. FI is considered as an important poverty reduction measure in Vietnam. The Government of Vietnam has promulgated a number of policies to promote FI. As a result, access to financial services in Vietnam has achieved positive results. Vietnam’s credit institution system is increasingly developed with an extensive network. More favorable access to FI has contributed to an increase in households’ use of financial products and services. According to VHLSS in 2016, 2018, and 2020, the percentage of households using ATM cards is the highest among the use of financial services (31.45%, 36.71%, 45.81% in 2016, 2018, 2020, respectively) and is increasing at the highest rate (increased by 14.36% from 2016 to 2020). Next is the percentage of households with bank accounts, reaching 35.48% in 2020, increased by 14.21% compared to 2016. The percentage of households with savings at banks tends to increase, but the rate of increasing is still low, reaching 14.35% in 2020, up 2.88% compared to 2016. The percentage of households using credit cards is quite low and has not changed much over the past four years (2.49%, 3.04%, 3.8% in 2016, 2018, and 2020, respectively). Most of the households in the sample do not have assets such as stocks or securities. The percentage of households with investments in stocks and securities accounts for only 0.32% in 2020, remaining almost the same compared to 2016. Meanwhile, Table shows that using credit cards and having assets in stocks and securities reduces the probability of households falling into multidimensional poverty better than having bank accounts and ATM cards. In addition, Table also shows that most households in the multidimensional poverty group have very low percentages of using financial products and services. Thus, in order to further reduce multidimensional poverty, the Government of Vietnam needs to continue to make more efforts to increase the percentage of households using financial products and services, especially credit cards and participation in financial markets.
Regarding control variables, the living area (ARE), household size (HSIZE), age (AGE), marital status (MAR) and education of the household head (HEDU) have an impact on multidimensional poverty. On the contrary, the gender of the household head does not affect multidimensional poverty. These results are in line with findings from prior studies such as Betti et al. (Citation2002), Tran et al. (Citation2015), and Le and Nguyen (Citation2018). Households living in urban areas and have smaller size are less likely to be multidimensionally poor.
In Vietnam, access to finance is easier in cities than in rural and remote areas, causing difficulties for the poor and low-income people in the rural areas. According to the State Bank of Vietnam (Citation2021b), 34% of adults living in rural areas own an account, which is much lower than those living in urban areas (57%). While 90% of urban people take nearly 15 minutes to get to the nearest financial service point, that percentage in rural areas is only 40%. Despite a decreasing trend, the rate of multidimensionally poor households in rural areas of Vietnam is quite high at 11.8% in 2016, 9.6% in 2018 and 7.1% in 2020; this rate is 3.5%, 9.6%, 1.1%, respectively in urban areas. Besides, according to our calculation from the sample, there is a big difference in the proportion of households using financial products and services in urban and rural areas. Among households using credit cards, households living in urban areas account for 57.14%, those in rural areas account for only 42.86%. Of those who invested in stocks and securities, households living in urban areas account for 72.73%, and those in rural households account for only 27.7%. According to the State Bank of Vietnam (Citation2021b), the awareness of people, especially people living in rural, remote and isolated areas about financial services is still limited. The lack of financial literacy is one of the reasons hindering people’s effective access and usage of financial products and services. Therefore, in order to reduce the rate of multidimensional poverty, the Government needs to further promote financial education and propaganda to improve financial literacy for people, especially those living in rural and remote areas.
The results also imply that access to business information has an important influence on poverty reduction. Communication and information for poverty reduction is one of the five national targets on sustainable poverty reduction in the country. Communication of mass media agencies provides the poor with knowledge and information on production and business practices, so that they can determine how to set up their own business, and use the borrowed funds effectively.
On the other hand, the gender of the household head does not seem to impact poverty. The reason could be that both genders have equal opportunity to access financial services and are equally able to manage their family to overcome poverty. For example, there is no big difference in credit and debit card ownership rates between the two genders in the country. The credit card ownership percentage for men is 4.6%, for women is 3.7%; the debit card ownership percentage for men is 26.1%, for women is 27.3% (World Bank, Citation2021). In some other countries in the region, the disparity in debit card ownership rates between the two genders is quite high (India 42.8% and 22.3%; China 70.3% and 63.1%; Malaysia 79.4% and 67.5% for men and women, respectively).
In general, FI has been promoted over the recent years in Vietnam as a means to reduce poverty. However, access to and usage of financial services in Vietnam is still limited. Awareness of the people, especially those living in rural, remote and isolated areas about financial services is still low. Many people are not familiar with making transactions via ATMs or at point of sales (POSs). The causes come from both the suppliers and the users of financial products and services. Many people may not have sufficient knowledge and skills, causing them to be afraid of using financial products and services. Although the network of credit institutions covers all localities across the country, it is unevenly distributed and there is a big disparity between urban and rural areas. Financial products and services are still lacking in diversity, and unable to meet the needs of some customer segments. In rural areas, products are mainly related to credit, while savings, payments and insurance services are lacking and have not met the demand. The habit of using cash is still quite common even in urban areas. Recurring monthly payments such as paying utility bills, school fees, or receiving social benefits are still done mostly in cash in rural areas.
5. Conclusions
In this study, we use a multivariate probit model with a dataset obtained from Vietnam Household Living Standards Survey conducted by the General Statistics Office of Vietnam in 2016 and 2018 to estimate the effect of FI and other factors on multidimensional poverty. The results show that FI has a positive impact on multidimensional poverty reduction. Using financial products or services reduces the probability of households falling into multidimensional poverty. Specifically, households having bank accounts, bank savings, using debit cards, credit cards, or investing in stocks or bonds are less likely to fall into multidimensional poverty. Our study also provides interesting evidence that participating in financial markets such as investing in stocks or bonds reduces multidimensional poverty reduction in Vietnam. Regarding households and household heads’ characteristics, households living in urban areas and having access to production and business information are less likely to be multidimensionally poor. As the number of household members increases, the chance of falling into multidimensional poverty increases; As the age of the household head increases, the likelihood of the household falling into multidimensional poverty decreases; household heads with a spouse are less likely to be multidimensionally poor; The higher the education level of the household head, the lower the probability of falling into multidimensional poverty.
Our study has implications for the government in reducing poverty. To reduce the rate of poverty, the government’s policies should encourage households to use financial products and services. Policies should be implemented to promote FI, so that the poor and disadvantaged people in society have more opportunities to access financial products and services. In order to promote the household level of access to and use of financial products and services, it is necessary to focus on the following issues:
Firstly, the government should implement policies to support the expansion of banks’ branches, ATMs and POSs, especially in rural, remote and isolated areas; the development of various financial products and services, including payments, money transfers, savings, credit, and insurance; and the development of microfinance institutions.
Secondly, in addition to policies to promote access to FI, the government should implement policies to promote households’ use of financial products/services. Policies should be developed to improve financial literacy and capacity. Financial education curricula should be provided from primary school to colleges with the goal of forming systematic financial knowledge, helping the young generation to be financially literate. Finance distribution channels should be developed based on digital technology applications. Credit institutions and payment intermediaries should develop distribution channels using digital technology applications such as mobile banking, e-wallets, digital banking services to provide low cost services that customers can access safely and conveniently, especially customers in rural and remote areas.
Thirdly, besides measures to promote FI, the government should take measures to reduce multidimensional poverty such as promoting high-productivity jobs to increase people’s incomes; improving the effectiveness of poverty reduction programs and policies; closely monitoring support packages so that the support money reaches the people. The government should continue to invest in infrastructure in residential areas, especially in poor areas and poor communes in order to create favorable conditions for socio-economic development. The government should provide vocational training and create more jobs for people, especially workers in rural and remote areas.
In this study, we evaluate the effect of FI on multidimensional poverty. However, with the data collected from VHLSS, we have not yet been able to identify the challenges that prevent households from using financial products and services. Future studies can extend this topic by examining the determinants of households’ use of financial products and services, thus better policy recommendations can be made to develop the financial systems.
Disclosure statement
No potential conflict of interest was reported by the author(s).
Additional information
Funding
Notes on contributors
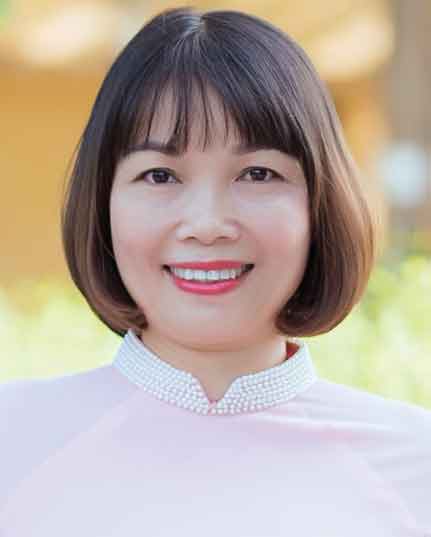
Huong Thi Thanh Tran
Huong Thi Thanh Tran is the Head of the Statistics Division, Faculty of Accounting and Auditing, Banking Academy, Vietnam. Before working as a lecturer at Banking Academy, she had 10 years working at the General Statistics Office of Vietnam. Her major fields of study include statistics, finance, economic development, and productivity.
Ha Thi Thu Le
Ha Thi Thu Le is the Deputy Dean of the Faculty of Accounting and Auditing, Banking Academy, Vietnam. She has prior working experience as an audit senior at KPMG Vietnam before becoming a lecturer at Banking Academy. Her major research interests include accounting and finance issues.
Nga Thanh Nguyen
Nga Thanh Nguyen is currently a lecturer at the Faculty of Mathematics, Banking Academy, Vietnam. Her major research interests include survival analysis and applied statistics.
Tue Thi Minh Pham
Tue Thi Minh Pham is currently the Deputy Head of Management Accounting Division, Faculty of Accounting and Auditing, Banking Academy, Vietnam. Her major fields of study include accounting and finance issues.
Huyen Thanh Hoang
Huyen Thanh Hoang is currently a lecturer at the Statistics Division, Faculty of Accounting and Auditing, Banking Academy, Vietnam. Her major fields of study include statistics and economics.
References
- Ajisafe, R. A., Anyakudo, I. O., Akinkuotu, F., & Okunade, S. O. (2018). Financial inclusion, monetary policy and poverty level in Nigeria. African Journal of Applied Research, 4(2), 253–22. http://doi.org/10.26437/ajar.04.02.2018.22
- Alimi, A. S., & Okunade, S. O. (2020). Financial inclusion, ICT diffusion and poverty reduction: Evidence from Sub-Sahara African Countries. Asian Journal of Economics and Business, 1(2), 139–152. https://arfjournals.com/image/32482_4_ahmed_s.pdf
- Alkire, S., Roche, J. M., Ballon, P., Foster, J., Santos, M. E., & Seth, S. (2015). Multidimensional poverty measurement and analysis. Oxford University Press.
- Alkire, S., & Santos, M. E. (2010). July 1, 2010 . United Nations Development Programme Human Development Report Office Background Paper No. 2010/11. Oxford Poverty & Human Development Initiative (OPHI) Working Paper No. 38. http://dx.doi.org/10.2139/ssrn.1815243
- Allen, F., Demirguc-Kunt, A., Klapper, L., & Peria, M. S. M. (2016). The foundations of financial inclusion: Understanding ownership and use of formal accounts. Journal of Financial Intermediation, 27, 1–30. https://doi.org/10.1016/j.jfi.2015.12.003
- Álvarez-Gamboa, J., Cabrera-Barona, P., & Jácome-Estrella, H. (2021). Financial inclusion and multidimensional poverty in Ecuador: A spatial approach. World Development Perspectives, 22, 100311. https://doi.org/10.1016/j.wdp.2021.100311
- Asante, S. A. (2018). Exploring multidimensional poverty and microfinance programmes impact: Poverty alleviation in rural Bangladesh. Master Essay I, Department of Economics, Lund University.
- Ashraf, N., Karlan, D., & Yin, W. (2010). Female empowerment: Impact of a commitment savings product in the Philippines. World Development, 38(3), 333–344. https://doi.org/10.1016/j.worlddev.2009.05.010
- Babajide, A. A., Adegboye, F. B., & Omankhanlen, A. E. (2015). Financial inclusion and economic growth in Nigeria. International Journal of Economics and Financial Issues, 5(3), 629–637. https://dergipark.org.tr/en/pub/ijefi/issue/31970/352165?publisher=http-www-cag-edu-tr-ilhan-ozturk
- Beck, T., Demirgüç-Kunt, A., & Levine, R. (2007). Finance, inequality and the poor. Journal of Economic Growth, 12(1), 27–49. https://doi.org/10.1007/s10887-007-9010-6
- Betti, G., D’Agostino, A., & Neri, L. (2002). Panel regression models for measuring multidimensional poverty dynamics. Statistical Methods and Applications, 11(3), 359–369. https://doi.org/10.1007/BF02509832
- Burgess, R., & Pande, R. (2005). Do rural banks matter? Evidence from the Indian social banking experiment. American Economic Review, 95(3), 780–795. https://doi.org/10.1257/0002828054201242
- Cámara, N., & Tuesta, D. (2014). Measuring financial inclusion: A multidimensional index. BBVA Research Paper, (14/26). https://papers.ssrn.com/sol3/papers.cfm?abstract_id=2634616
- Cappellari, L., & Jenkins, S. P. (2003). Multivariate probit regression using simulated maximum likelihood. The STATA Journal, 3(3), 278–294. https://doi.org/10.1177/1536867X0300300305
- Chhorn, D. (2021). Microfinance illusion, poverty and welfare in Cambodia. Journal of the Asia Pacific Economy, 26(4), 694–719. https://doi.org/10.1080/13547860.2020.1826074
- Chithra, N., & Selvam, M. (2013). Determinants of financial inclusion: An empirical study on the inter-state variations in India. SSRN Electronic Journal. Available at SSRN 2296096. https://doi.org/10.2139/ssrn.2296096
- Churchill, S. A., & Marisetty, V. B. (2020). Financial inclusion and poverty: A tale of forty-five thousand households. Applied Economics, 52(16), 1777–1788. https://doi.org/10.1080/00036846.2019.1678732
- Dartanto, T., & Nurkholis. (2013). The determinants of poverty dynamics in Indonesia: Evidence from panel data. Bulletin of Indonesian Economic Studies, 49(1), 61–84. https://doi.org/10.1080/00074918.2013.772939
- Demirgüç-Kunt, A., & Klapper, L. (2012). Measuring financial inclusion: The global findex database. World Bank Policy Research Working Paper, 6025(April), 1–61. The World Bank Washington, DC, USA. https://doi.org/10.1596/978-0-8213-9509-7.
- Demirgüç-Kunt, A., & Singer, D. (2017). Financial inclusion and inclusive growth: A review of recent empirical evidence. World Bank Policy Research Working Paper, 8040. https://openknowledge.worldbank.org/bitstream/handle/10986/26479/WPS8040.pdf?
- Dixit, R., & Ghosh, M. (2013). Financial inclusion for inclusive growth of India-A study of Indian states. International Journal of Business Management & Research, 3(1), 147–156. https://d1wqtxts1xzle7.cloudfront.net/48382483/2-32-1362664602-13.Financial.full-2-with-cover-page-v2.pdf?Expires=1665309329&Signature=baiuPQibiFlZjyu5t~ZmPP9dLGkxAAKTN3yEf2IcpjOlmN3FTEwqRcoaxmS4TPoWusQ6CVhGK2VemWW~t8BaJN3QJTKmJ1M0h2DOsbMvGlzq9inelSRbsrQu2sEFJHWGsGzwLbHCnuD0OoiuxxxptI0VzyJZKWoNIgHZir3y2tGcYBkP53MhLOdGUs1dsZ6a~LgvTMYLEyvynvSArRHrWr3W9W0mLnTLPdvLfBvP7qT6bvtAYC1wWkpZd1bOwJO69EZyfbVKA1s6EiPTsdnqVTt5ccYirpdHLnaGiDfHtAh-uXf5tGdxYeRcW~GlLlj3Ynkc9gFLgLUAqHMLiwGhbA__&Key-Pair-Id=APKAJLOHF5GGSLRBV4ZA
- Dogan, E., Madaleno, M., & Taskin, D. (2022). Financial inclusion and poverty: Evidence from Turkish household survey data. Applied Economics, 54(19), 2135–2147. https://doi.org/10.1080/00036846.2021.1985076
- Evans, O. L. A. N. I. Y. I., & Alenoghena, O. R. (2017). Financial inclusion and GDP per capita in Africa: A bayesian VAR model. Journal of Economics & Sustainable Development, 8(18), 44–57. https://core.ac.uk/download/pdf/234647999.pdf
- Geda, A., Shimeles, A., & Zerfu, D. (2008). Finance and poverty in Ethiopia: Ahousehold-level analysis. In B. Guha-Khasnobis, G. Mavrotas (Eds.), Financial development, institutions, growth and poverty reduction (pp. 61–86). Palgrave Macmillan.
- Inoue, T. (2011). Financial inclusion and poverty alleviation in India: An empirical analysis using state-wise data. In S. Hirashima, H. Oda, & Y. Tsujita (Eds.), Inclusiveness in India (pp. 88–108). Palgrave Macmillan.
- Kelkar, V. (2010). Financial Inclusion for Inclusive Growth. ASCI Journal of Management, 39(1), 55–68.
- Khan, I., Khan, I., Sayal, A. U., & Khan, M. Z. (2022). Does financial inclusion induce poverty, income inequality, and financial stability: Empirical evidence from the 54 African countries? Journal of Economic Studies, 49(2), 303–314. https://doi.org/10.1108/JES-07-2020-0317
- Kim, H., & DeVaney, S. A. (2001). The determinants of outstanding balances among credit card revolvers. Financial Counseling and Planning, 12(1), 67–77. https://citeseerx.ist.psu.edu/viewdoc/download?doi=10.1.1.587.8612&rep=rep1&type=pdf
- Koomson, I., Villano, R. A., & Hadley, D. (2020). Effect of financial inclusion on poverty and vulnerability to poverty: Evidence using a multidimensional measure of financial inclusion. Social Indicators Research, 149(2), 613–639. https://doi.org/10.1007/s11205-019-02263-0
- Kumar, N. (2013). Financial inclusion and its determinants: Evidence from India. Journal of Financial Economic Policy, 5(1), 4–19. https://doi.org/10.1108/17576381311317754
- Le, T. T. L., & Nguyen, T. B. (2018). Những nhân tố ảnh hưởng đến tình trạng nghèo đa chiều của Việt Nam [Determinants of multidimensional poverty in Vietnam]. Journal of Science of Ho Chi Minh City Open University, 61(4), 47–56. https://journalofscience.ou.edu.vn/index.php/econ-vi/article/view/1509
- Mohseni-Cheraghlou, A. (2015). Reducing poverty through promoting financial inclusion, shared prosperity and social solidarity: The role of Islamic finance and qard Hassan. In 4th International Conference on Inclusive Islamic Financial Sector Development ( Vol. 53, No. 9, pp. 1689–1699).
- Ningaye, P., Ndjanyou, L., & Saakou, G. M. (2011). Multidimensional poverty in Cameroon: Determinants and spatial distribution. AERC Research Paper 211. African Economic Research Consortium, Nairobo.
- Nuryitmawan, T. R. (2021). The impact of credit on multidimensional poverty in rural areas: A case study of the Indonesian Agricultural Sector. Agriecobis: Journal of Agricultural Socioeconomics and Business, 4(1), 32–45. https://doi.org/10.22219/agriecobis.v4i1.15515
- Park, C. Y., & Mercado, R., Jr. (2018). Financial inclusion, poverty, and income inequality. The Singapore Economic Review, 63(1), 185–206. https://doi.org/10.1142/S0217590818410059
- Sanya, O., & Olumide, F. F. (2017). Financial inclusion as an effective policy tool of poverty alleviation: A case of Ekiti State. IOSR Journal of Economics and Finance (IOSR-JEF), 8(4), 1–10. https://doi.org/10.9790/5933-0804020110
- Sarma, M. (2008). Index of financial inclusion. Working paper no. 215, Indian Council for Research on International Economic Relations (ICRIER). New Delhi.
- Sarma, M., & Pais, J. (2011). Financial inclusion and development. Journal of International Development, 23(5), 613–628. https://doi.org/10.1002/jid.1698
- Sethi, D., & Acharya, D. (2018). Financial inclusion and economic growth linkage: Some cross country evidence. Journal of Financial Economic Policy, 10(3), 369–385. https://doi.org/10.1108/JFEP-11-2016-0073
- State Bank of Vietnam. (2021a) . History of Vietnam Bank in 1951–2021. Labor Publishing House.
- State Bank of Vietnam. (2021b) . Inquiries about financial inclusion. Vietnamese Women’s Publishing House.
- Tran, V. Q., Alkire, S., & Klasen, S. (2015). Static and dynamic disparities between monetary and multidimensional poverty measurement: Evidence from Vietnam. In Research on Economic Inequality, in: Measurement of poverty, deprivation, and economic mobility (Vol. 23, pp. 249–281). Emerald Group Publishing Limited. https://doi.org/10.1108/S1049-258520150000023008
- Tran, H. T. T., & Le, H. T. T. (2021). The impact of financial inclusion on poverty reduction. Asian Journal of Law and Economics, 12(1), 95–119. https://doi.org/10.1515/ajle-2020-0055
- Vietnam Prime Minister (2015). Decision No. 59/2015/QD-TTg dated November 19, 2015 of the Prime Minister on the promulgation of the multidimensional poverty line for the period of 2016-2020.
- Vietnam Prime Minister (2016). Decision No. 1726/QD-TTg dated 5 September 2016 of the Prime Minister approving the project to improve accessibility to banking services for the economy
- Vietnam Prime Minister (2018). Decision No. 241/QD-TTg dated 23 February 2018 of the Prime Minister approving the scheme to promote payments through banks for public services.
- Vietnam Prime Minister. (2020). Decision 149/QD-TTg dated January 21, 2020 accepts the National Financial Inclusion Strategy to 2025, with a vision to 2030, signed by the Prime Minister of Vietnam.
- Walker, S. H., & Duncan, D. B. (1967). Estimation of the probability of an event as a function of several independent variables. Biometrika, 54(1–2), 167–179. https://doi.org/10.1093/biomet/54.1–2.167
- World Bank, 2021 The Global Findex Database 2021. https://www.worldbank.org/en/publication/globalfindex/Data
- World Bank (2021). The Global Findex Database 2021. https://www.worldbank.org/en/publication/globalfindex/Data
- Yang, Y., & Fu, C. (2019). Inclusive financial development and multidimensional poverty reduction: An empirical assessment from rural China. Sustainability, 11(7), 1900. https://doi.org/10.3390/su11071900
- Zhang, Q., & Posso, A. (2017). Thinking inside the box: A closer look at financial inclusion and household income. The Journal of Development Studies, 55(7), 1616–1631. https://doi.org/10.1080/00220388.2017.1380798
- Zhu, D., & De’Armond, D. (2005). The factors of consumer debt: A look at demographic, economic, and credit management variables among participants of the 2001 consumer expenditure survey. Association for Financial Counseling and Planning Education. Scottsdale, Arizona.