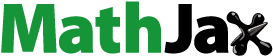
Abstract
Establishing a link between specific sustainable development goals (SDGs) and the outcomes of adopted agricultural technological products is lacking in developing countries. Therefore, this study aims to address food security SDG 02 by adopting crop protection products of multinational brands versus sub-standard crop protection products SDG 12 by smallholder farming households. We employ endogenous switching probit models using a survey of smallholder farming households in the cotton-wheat zone in Pakistan. Full information maximum likelihood estimates illustrate that comparative advantage guide the adoption of crop protection products of multinational brands (CMBs). Our findings suggest that adopting CMBs rather than sub-standard crop protection products may translate as a responsible farming practice if assuming the use of crop protection products is inevitable. In sum, CMBs enhance food security and can play a vital role in the current debate on responsible consumption and production, particularly for sustainable development. Our findings also indicate that promoting agricultural extension information via radio broadcasts has a significant and positive relationship with adoption. Hence, it stands out as the most promising policy option.
1. Introduction
Food insecurity is high for a substantial fraction of the Pakistani rural population, with 53% of rural households being food insecure (The Government of Pakistan, Citation2022). For a lower-middle-income country, food security is at alarmingly low levels in Pakistan: Pakistan only ranks 75th of the 113th countries covered by the Global Food Security Index (GFSI, Citation2021). Likewise, Pakistani rural communities have a rural poverty syndrome, deprived of factors endowments but the aggressive use of sub-standard crop protection products (hereafter SCPs; Bilal & Barkmann, Citation2019; Rehman et al., Citation2015).
In many low-income and middle-income countries, crop protection products are contaminated by hazardous substances or have insufficient declarations or safety and usage information. Pakistan has been a signatory of the Rotterdam Convention on prior informed consent procedures for certain hazardous chemicals and crop protection products since 1999 and the signatory of the Stockholm Convention on persistent organic pollutants since 2001 (Rotterdam convention, Citation2019; Stockholm convention, Citation2019). Despite the fact, existing literature indicates that to reduce production costs, the substantive smallholder farming households (SHFHs) in Pakistan purchase and use locally manufactured products with low-quality formulations and unpredictably variable concentrations of active agents (Khan et al., Citation2013; Nafees et al., Citation2008). In particular, the literature also indicates the usage of WHO hazardous category-I crop protection products (e.g., carbamates, organophosphates, and pyrethroid) by SHFHs (Khan et al., Citation2011). The excessive and irresponsible usage of SCPs also contributes to lower sustainable agricultural development; it induces pests and weeds infestation and increased reliance on crop protection products (Aga, Citation2019; Tariq et al., Citation2007; UNDP, Citation2001). The cautious use of crop protection products is vital in cotton to control yield losses from insect pest attacks. However, due to ignorance of vital inputs and weeds, potential yield losses in cotton account for 40% and 9% of total losses due to insects and viruses (Khan et al., Citation2015; Oerke, Citation2006). Wheat is not an exception from the potential yield losses; wheat accounts for 35%-40% of potential yield losses due to insects, pests, and viruses (Khan et al., Citation2012; Rehman et al., Citation2015). Unfortunately, higher-quality crop protection products’ higher prices and lack of accessibility may offset the agronomic advantages of higher quality products (Aga, Citation2019; Aktar et al., Citation2009; Antle & Pingali, Citation1994; Lee, Citation2005).
The crop protection products of multinational brands (hereafter CMBs) may translate as higher quality crop protection products because their product formulation generally follows high standards in terms of research and development (R&D; Alston, Citation2010; Naseem et al., Citation2006; Thirtle et al., Citation2005). About one-third of investment in agricultural R&D is globally direct towards crop protection products (Anandajayasekeram, Citation2011; Pray & Fuglie, Citation2001). Furthermore, the adoption of branded products shows an attraction for the consumer due to product information, products specification, and packaging attributes (Lewis et al., Citation2016). On the contrary, SCPs translate as sub-standard because SCPs firms generally lack R&D departments (Khan & Khattak, Citation2013; Piesse & Thirtle, Citation2010). Additionally, SCPs may include outdated ingredients or low-quality formulations, low and unpredictably variable concentrations of active agents, lacking and insufficient declarations, safety, and usage information (Khan et al., Citation2013; Hashmi, Citation2016).
The diversity of varying quality crop protection products goes from CMBs to SCPs in the agricultural heartland of Pakistan (Bilal et al., Citation2022; Khan et al., Citation2013). Against this diversity of crop protection products of varying quality, it is surprising that we could not identify any scientific study that—to our knowledge—specifically investigates the potential advantages of using CMBs for smallholder food security.
The paper is structured as follows: the proceeding section covers the literature regarding the theme of the study. In section 2, we introduce the study site, sampling methodology, details of HFIAS, and conceptual and methodological framework. Section 3 covers the results and discussion focusing more on the estimates of the endogenous switching probit models. Section 4 concludes with policy implications and prospects for future research on the agricultural administration of Pakistan.
1.1. Literature review
Technology adoption can be essential for achieving agricultural sustainability and food security (Vergragt, Citation2006). Empirical studies show that technologically improved products can enhance farm productivity and play a crucial role in improving the food security of SHFHs (Kassie et al., Citation2015; Nyyssola et al., Citation2014). For example, SHFHs are adopting improved verities, genetically modified (GM) crops, and improved storage technologies—with positive synergies on food security outcomes (Elias et al., Citation2013; Kabunga et al., Citation2014; Tesfaye & Tirivayi, Citation2018). Many issues on the adoption of improved technological products, particularly the quality of crop protection products (e.g., insecticides, fungicides, and herbicides) as well as adoption outcomes in terms of improving food security, still need to be explored (Carvalho, Citation2017; Muzari et al., Citation2012; Niles et al., Citation2018).
A substantial number of studies indicate a positive impact of adopting crop protection products in general on productivity. For example, Hameed et al. (Citation2017) show that adopting herbicides (e.g., glyphosate and paraquat formulations) use are positively associated with productivity in Pakistan, and Bakhsh et al. (Citation2016) include the quantity of crop protection products to estimate the productivity of Bt (Bacillus thuringiensis) cotton in Pakistan. However, such synergies may be misleading because the manufacturing and qualitative aspects of the applied crop protection products remain unaddressed. A highly relevant study by Bilal et al. (Citation2022) highlight the positive relationship between high-quality crop protection products and farm productivity. The technical inefficiency models’ estimates show that adopting high-quality crop protection products and specialization (if farmers cultivate only cotton and wheat crops) may positively contribute to agricultural sustainability.
Unfortunately, increasing agricultural productivity to meet the brisk pace of food demand comes with social and environmental costs. On the way toward a more sustainable and resilient world, the world faces substantial challenges (e.g., poverty and food insecurity). No country is an exception to excel in all assigned targets of achieving SDGs by 2030 (FAO, Citation2018). McCollum et al. (Citation2018) emphasize the dire need for interdisciplinary research with fresh perspectives to inter-link the SDGs. Using the global UN data, Ament et al. (Citation2020) demonstrate that 70% of SDG indicators are positively associated with GDP per capita. However, the study asserts to identify the major opportunities to achieve SDGs targets and indicates the scarcity of complementary empirical evidence at the country level (Saccone & Vallino, Citation2022).
We extend to the previous literature by focusing more explicitly on the adoption outcomes of higher quality crop protection products and establishing a link with particular SDGs. Specifically, to elucidate the quality impacts of crop protection products (SDG 12: responsible production and responsible consumption) on food security (SDG 02: zero hunger) of SHFHs in the cotton-wheat zone in Pakistan. Food security outcomes are assessed via the self-reported Household Food Insecurity Access Scale (HFIAS; Coates et al., Citation2007). HFIAS is widely applied in several countries, including developing countries of Africa and Asia (Sheikh et al., Citation2020).
We acknowledge the methodological challenges in ex-post impact assessments. Because the observed and unobserved heterogeneity may taint the outcomes of technology adoption (Heckman & Vytlacil, Citation2007), we consider this when comparing adopters (exclusive CMBs users) to non-adopters (SCPs/otherwise). Therefore, as we deal with non-experimental studies, farmers are not randomly assigned to adopters and non-adopters. Consequently, we include selection instruments that help identify an adoption decision’s effect but are exogenous to food security outcomes (Heckman & Vytlacil, Citation2007). We employ endogenous switching regression (endogenous switching probit model) by full information maximum likelihood (FIML) to account for the endogeneity.
In a nutshell, this study reduces the knowledge gap about food security triggered by the adoption of CMBs. We, therefore, attempt to answer the specific research question: Does the adoption of CMBs affect the food security of SHFHs?
2. Methodology
2.1. Study site and sampling methodology
The Cotton-wheat zone of the Punjab province is a substantial retail market for crop protection products (Pakistan Crop Protection Association, Citation2016). According to the Punjab Bureau of Statistics, farms with an area ≤ of 5 acres (~2.02 hectares) are considered small farms, and 58% are small farms. Punjab contributes 72% of cotton to the total cotton production in Pakistan (The Government of Punjab, Citation2015). The cotton-wheat zone comprises three important agricultural divisions (the highest administrative unit) of Punjab province: the Bahawalpur, the Multan, and the Sahiwal. We employed multistage random sampling with the probability of selecting a farming household being proportional to population, accounting for the availability and accessibility of crop protection product types.
Firstly, from each division, we randomly selected one district. Secondly, we randomly selected one tehsilFootnote1 from each district: Burewala, Pakpattan, and Sadiqabad (Figure ). Lastly, six villages from each subdistrict make up 18 villages in total. A total of N = 275 SHFHs were interviewed in 18 randomly selected villages. We sampled socio-demographic, farm-specific attributes, food security data (HFIAS) and the adoption status of CMBs and SCPs.
2.2. Household Food Insecurity Access Scale (HFIAS)
To compute the dependent variable for the outcomes equation, we use the HFIAS scale because of its proven applicability across cultures, low data requirement and successful application in South Asia and Africa (Chinnakali et al., Citation2014; Kabunga et al., Citation2014; Sheikh et al., Citation2020). HFIAS is a subjective assessment of food insecurity consisting of a household food access assessment (Coates et al., Citation2007). The HFIAS consists of nine questions about access to food. An affirmative answer to any question adds one to a total. Thus, the HFIAS scores range from 0 to 9.
However, the subjective assessment may induce response bias (Headey & Ecker, Citation2013). We asked SHFHs to outline their main agricultural activities during the agricultural calendar year before asking HFIAS questions to reduce response biases. Typical activities include land preparation, cultivation, harvesting, threshing, selling milk animals, and buying farm inputs (e.g., crop protection products). Moreover, we asked SHFHs to identify the months they expect grain or food shortages.
We employ principal component analysis for the robustness check about the response from HFIAS because of the high correlation among the responses. The analysis results retain two components because of the minimum advisable eigenvalue of the above unity (Kaiser, Citation1960). Component one loaded mainly on the first five questions that may reflect “food insecure”, while component two loaded primarily to the last three questions that may reflect “severely food insecure” (Table in Appendix A). The Cronbach alpha statistic tested the scale reliability coefficient, and the value for the scale was α = 0.95. We also observed the Kaiser-Meyer-Olkin (KMO) measure of sampling adequacy = 0.89, suggesting the data adequacy for component analysis.
The information extracted from these components could have been used as a continuousFootnote2 dependent variable for the outcomes equation. However, failing to find sufficient selection instruments, we prefer a binaryFootnote3 dependent variable for outcomes modelling. Therefore, we classify smallholders exclusively into two categories concerning their food security status: food secure and food-insecure households.
Recent past studies greatly support the construction of a binary dependent variable based on subjective assessment. For example, Tesfaye and Tirivayi (Citation2018) constructed a binary dependent variable for outcomes equation using HFIAS information. Moreover, closely following the existing literature on self-assessment and subjective food security assessment, Shiferaw et al. (Citation2014) and Khonje et al. (Citation2015) used a binary dependent variable for food security outcomes.
2.3. Conceptual and methodological framework
Ex-post impact assessment studies, where farmers are not randomly assigned into treatment and control groups, may result in self-selection bias and endogeneity problems (Heckman & Vytlacil, Citation2007). Sometimes unobserved endogenous variables (e.g., farm management, personal capabilities, and farming decisions) impact the treatment and outcomes variables and may cause endogeneity. One possible solution to account for endogeneity is to invoke a selection instrument to improve identification. The selection instrument explains the adoption decision but is exogenous to outcome variables (Bellemare, Citation2010). Finding a relevant selection instrument is difficult as it must simultaneously fulfil the conditions of relevance and exogeneity (Kassie et al., Citation2015).
We use the distance (i) from a smallholder farm to the farm of the village head and (ii) from a smallholder farm to the main road as selection instruments. There is substantial empirical evidence of the positive association of demographic attributes with technology adoption (Khonje et al., Citation2015; Tesfaye & Tirivayi, Citation2018). Recent impact assessment studies by Dedehouanou et al. (Citation2018) have used the household distance in kilometres (km) to the administrative subdivision as a selection instrument; Krishna et al. (Citation2017) have used the altitude of the place of residence to the sea level in meters as a selection instrument. Our selection instruments are similar to those used in these successful implementations of the method.
We employed proper econometrical procedures to validate the inclusion of selection instruments. Firstly, we constructed a correlation matrix between potential selection instruments, adoption status, and food security status. Secondly, we considered the relevance and exogeneity conditions for selection instruments and employed relevant diagnostic tests (e.g., Sargan’s and Anderson’s canonical correlations statistics). Finally, we follow the admissibility of exclusion restriction on considered selection instruments. We employ the falsification approach of Di Falco et al. (Citation2011). The selection instruments must be significantly correlated with adoption decisions but not with the interest outcomes among non-adopters farm households. A Wald test on selection instruments also confirms that these are potential instruments as the test statistics are significant with P <1% (Tables A2–A4 in Appendix A).
This study uses binary adoption and food security outcomes (Tesfaye & Tirivayi, Citation2018). Farmers who exclusively incorporate CMBs are termed adopters, and those who do not are non-adopters. However, adoption is potentially endogenous, and this gives no causal interpretation of outcomes of interest because of smallholders’ own choices for adoption. This condition may be affected by unobserved heterogeneity and possible self-selection due to farm level attributes and farming household attributes that may create problems in assessing food security gains by adopting technology (Kabunga et al., Citation2014). We tackle this situation and employ an endogenous switching probit model with FIML (Full Information Maximum Likelihood), which is considered an efficient method to estimate the binary selection equation and the binary outcomes equation simultaneously to yield consistent standard errors of the estimates. The model works under the assumption of joint normality of the error terms in the selection and outcomes equations (Lokshin & Glinskaya, Citation2009; Lokshin & Sajaia, Citation2011).
We assume that SHFHs can adopt CMBs, but the response to adoption may vary. We distinguish the response by introducing a criterion function . That determines which regime an SHFH is facing. As discussed above, here, the adoption (CMBs) and the outcomes (exclusive food secure) have one of two potential values (Aakvik et al., Citation2005).
The observed is defined as
In the present case,
and
are the latent variables (whether the SHFHs are exclusive food secure or not) that determine the observed binary outcomes,
and
(SHFHs response to adopting exclusive CMBs).
and
these are vectors of weakly exogenous variables, and for the model to specify the exogenous variables correctly in EquationEq. (2)
(2)
(2) and (Equation3
(3)
(3) ) should be the same.
J is a vector of variables that determine a switch between the regimes.
,
and α are vectors of parameters.
and
,
, and
these are the error terms under the assumption that
,
, and
are jointly normally distributed in the context of binary outcomes, with a mean-zero vector and correlation matrix (Heckman & Vytlacil, Citation2007; Lokshin & Sajaia, Citation2011).
Where
and
are the correlations between
,
and
,
and
is the correlation between
and
. We assume that
= 1 (α is estimable only up to a scalar factor).
We calculate the probabilities of adoption and adoption outcomes in the actual and counterfactual regimes. First, the probability of being treated and having positive outcomes is calculated (EquationEq. 4)(4)
(4) . Second, the probability of not being treated and having zero outcomes is calculated (EquationEq. 5)
(5)
(5) . Third, the probability of being treated and having zero outcomes (EquationEq. 6
(6)
(6) ), and fourth, not being treated and having positive outcomes (EquationEq. 7)
(7)
(7) is computed.
Following Aakvik et al. (Citation2005) and Lokshin and Sajaia (Citation2011), we calculate the effect of the treatment on the treated (TT) summarised in EquationEq. (8)(8)
(8) as the difference of EquationEq. (4)
(4)
(4) —(Equation6
(6)
(6) ). EquationEq. (8)
(8)
(8) represents the effect of exclusive adoption of CMBs on the food security outcomes of the adopted SHFHs.
Following the same procedure, we calculate the treatment effect on the untreated (TU) summarised in EquationEq. (9)(9)
(9) as the difference between EquationEq. (7)
(7)
(7) —(Equation5
(5)
(5) ). EquationEq. (9)
(9)
(9) represents the effect of exclusive adoption of CMBs on food security outcomes of the SHFHs who did not adopt.
3. Results and discussion
This section describes the estimates of the descriptive statistics of independent variables and endogenous switching probit model estimated by FIML with robust standard errors. Tables presents FIML estimates of the endogenous switching probit model.
Table 1. Adoption and food security status of sampled households
Table 2. Descriptive statistics of variables
Table 3. Estimates of the endogenous switching probit model
Table 4. Treatment effects of CMBs adoption on the food security status
3.1. Description of dependent variables
The dependent variable for the selection equation is adoption status: adopters (exclusive CMBs use, coded 1) and non-adopters (use of SCPs/otherwise, coded 0). Within the econometric framework, adopters are also referred to as the “treated” group and non-adopters as the “untreated” group. The dependent variable for the outcome’s equation is food security status: exclusive food-secure households (HFIAS total score = 0 “zero”, translates for exclusive food secure =1) and food-insecure households (HFIAS total score ≥ 1, translates to food insecure =0). Table presents the frequency of full sample distribution into adoption status and food security status.
3.2. Description of independent variables
Table presents the detailed descriptive statistics. Two-sample mean-comparison tests indicated statistically significant differences between adopters and non-adopters (e.g., cotton seed if GM (yes =1; no =0), off-farm income sources, farm machinery, seasonal labour, and agricultural extension information via radio). No substantial difference was observed, e.g., for the total number of males and females in a household, milk animals, and agricultural area.
Table includes socio-demographic and farming capital variables. We take guidance from empirical studies and economic theory to have socio-demographic and farming capital variables (Abdulai & Huffman, Citation2014; Kassie et al., Citation2011; Shiferaw et al., Citation2014; Tesfaye & Tirivayi, Citation2018). The source of agricultural information is significant to keep farmers up to date about the development in the agriculture sector. At first glance, our sample indicated that adopters listen to the radio more frequently to acquire agriculture extension service information, and they have more years of schooling than non-adopters (Asfaw et al., Citation2012; Khonje et al., Citation2015).
Since the adoption of GM cotton seed in Pakistan in 2002 (James, Citation2012), the SHFHs cultivate pest-resistant cultivars, such as GM cotton seed (e.g., Bt cotton). Despite the cultivation of Bt cotton, the initial adoption of crop protection products has been increasing swiftly because Bt cotton fails to resist detrimental sucking pests (e.g., Whitefly, Mealybug, Aphids, and Jassids; Abdullah, Citation2010; Spielman et al., Citation2017). The sample mean of average cultivation of GM cotton seed was significantly higher for adopters than for non-adopters. Similarly, the sample mean of average ownership of farm machinery is significantly higher for adopters than for non-adopters (Di Falco et al., Citation2011; Kabunga et al., Citation2014). From this perspective, the adoption of CMBs and the ownership of farm machinery complement each other (Bilal et al., Citation2022).
From the aspect of factor endowments—especially land and labour, the tendency to hire permanent labour, seasonal labour, and the area sown under cotton crop was higher for adopters than non-adopters. As we exclusively dealt with SHFHs, only 11% of the total sample hired permanent labour, and ~45% of the full sample had off-farm income sources. From a demographic point of view, the distance of SHFHs farms to the main road and the village head’s house was significantly smaller for adopters than for non-adopters (Tesfaye & Tirivayi, Citation2018).
3.3. Determinants of CMBs adoption
The first column of Table presents the estimated coefficients of the selection equation. Those farmers who had off-farm income generation means, employed seasonal labour for agricultural farming, and owned farm machinery was more likely to adopt. In particular, those farmers who acquired the agricultural extension information via radio were more likely to adopt it. This finding aligns with Rware et al. (Citation2021) confirm that agricultural extension services broadcast via radio promote the adoption of management practices for the application of pesticides. Likewise, Ashraf et al. (Citation2015) emphasize that underprivileged and uneducated farmers can benefit from extension field staff and farmer field schools.
From a demographic point of view, the SHFHs whose farms were closer to the main road and closer to the farm of the village head were more likely to adopt CMBs. Interestingly, those farming households who had received credit and those households with a higher number of females and those farming households who were in region 3 (Pakpattan) were less likely to adopt CMBs.. Table (Appendix A) presents the estimates of variables included in the main model by separate probit models of the selection equation without switching. The dependent variable equals one if the SHFHs exclusively decided to adopt CMBs and 0 for SCPs/otherwise. A comprehensive discussion on the factors determining the adoption of CMBs is beyond the scope of this study. However, Bilal and Barkmann (Citation2019) discuss the fundamental determinants of CMBs adoption in the agricultural mainland of Pakistan.
3.4. Estimates of the endogenous switching probit model: treatment effects
In the last row of Table , we report Wald tests statistics of the joint significance ( =
=0) of the error correlation coefficients in the selection and outcomes equations. The test rejected the null hypothesis that
=
because of the probability >
. Therefore, we were justified in employing an endogenous switching probit model to account for endogeneity (Dedehouanou et al., Citation2018).
The second last row of Table presents the correlation coefficients among error terms. The estimated correlation coefficient () for adopters and food secure SHFHs was significantly different from zero. This estimate may imply that the adoption of CMBs had a significant effect on food security, and the adopters would have gained more significant benefits from CMBs adoption than non-adopters had non-adopters decided to adopt CMBs. However, the estimated correlation coefficient (
) for non-adopters was not significantly different from zero. This estimate may mean that adopters and non-adopters have the same value of the outcomes provided by their observed characteristics.
Additionally, the signs of the correlation coefficients are essential to derive economic interpretations. From a fundamental economic point of view, we found comparative advantages for adoption and non-adoption of CMBs because the correlation coefficients are opposite in sign—considering the food security status of SHFHs (Fuglie & Bosch, Citation1995). This comparative advantage suggested that adopters have higher average values of food security outcomes than non-adopters, and non-adopters also have higher average values of food security outcomes than non-adoption.
The above interpretations of the correlation coefficients sign align with the estimates of the outcomes equation presented in the last two columns of Table —this indicated the presence of significant differences in both observed and unobserved characteristics among adopters and non-adopters. The positive effects of the off-farm income sources,Footnote4 seasonal labour, farm machinery ownership, and the region1 (if farmer located in Vehari =1, otherwise =0) on food security status were more prominent among the SHFHs who exclusively adopted CMBs. Surprisingly, the negative effect of credit receivedFootnote5 on food security status was also more pronounced among the SHFHs who exclusively adopt CMBs. The positive effect of the off-farm income sources on food security status among adopters and non-adopters was the same but varying magnitude. However, the negative effect of region1 on food security status was more apparent among the SHFHs who did not exclusively adopt CMBs.
Most importantly, Table presents the predicted food security outcomes of SHFHs under actual EquationEq. (4)(4)
(4) and (Equation5
(5)
(5) ) and counterfactual EquationEq. (6)
(6)
(6) and (Equation7
(7)
(7) ) regimes.
We report the treatment effects of adoption status on the food security status of SHFHs in the last column of Table . In the counterfactual regime EquationEq. (6(6)
(6) ), the SHFHs who actually adopted may have 22 percentage points less in food security if they had not adopted. This positive and significant value of TT suggests that adoption of CMBs would positively contribute to the food security of adopters, and their decision to opt for CMBs is presumably robust and rational. These findings corroborate the finding of recent studies on the food security impact of adoption (Negash & Swinnen, Citation2013; Shiferaw et al., Citation2014; Tesfaye & Tirivayi, Citation2018).
If the actual non-adopters had adopted, adoption would have reduced food security by 19 percentage points. This treatment effect for non-adopters on food security is consistent with Krishna et al. (Citation2017), who find that adopting oil palm expansion may reduce household welfare by 7% for actual non-adopters if they had adopted it. Similarly, Negash and Swinnen (Citation2013) find that non-adopter households of castor production decrease the food security probability if they had adopted it. Noltze et al. (Citation2013) also suggest that non-adopters would suffer from household income loss if they adapted to natural resource management technologies. This negative and significant value of TU indicates that adoption of CMBs would not positively contribute to the food security of non-adopters,Footnote6 and their decision not to opt for CMBs is presumably robust and rational. The present case may indicate heterogeneity because the transitional heterogeneity effect is positive and significant. Additionally, it may depict that base heterogeneity effects are significantly higher for those SHFHs that actually did adopt than those that did not (Krishna et al., Citation2017).
4. Conclusion and policy implications
This study mainly focused on the food security (SDG 02) effects of adopting CMBs (SDG 12). The Household Food Insecurity Access Scale (HFIAS) assessed the food security outcomes. This study was confined to SHFHs in the cotton-wheat zone of Punjab, Pakistan. We estimated an endogenous switching probit model with FIML to account for observed and unobserved heterogeneity using the survey data of the SHFHs in the cotton-wheat zone in Pakistan.
Among the variables tested, we found government extension service broadcast via radio is the most promising policy option to encourage the adoption of CMBs. This finding is in line with Rware et al. (Citation2021) and confirms that agricultural extension services broadcast via radio promote the adoption of management practices for the application of pesticides. To aid the diffusion of innovations among farmers, salient and practical information regarding improved agricultural technologies connotes the significance of institutional support for rural areas (Lee, Citation2005; Rogers, Citation1983). Consonant to that, this study and recent literature show the role of agricultural extension services in adoption, productivity enhancement, poverty alleviation, and food security in developing countries (Bilal & Barkmann, Citation2019; Simtowe et al., Citation2016). The lack of extension staff in developing countries hinders the overall response to adoption. A focus on improving government extension services broadcast via radio may also alleviate resource constraints of extension departments, such as the lack of extension staff (Aldosari et al., Citation2019). Our findings also indicated that promoting agricultural extension information via radio broadcasts has a significant and positive relationship with adoption. Hence, it stands out as the most promising policy option.
Based on FIML estimates, we propose a few vital policy implications because adoption is guided by comparative advantage. Our findings suggested that adopting CMBs may translate as a responsible farming practice if assuming crop protection products are inevitable. Hence, exclusive adoption of CMBs promotes food security among those SHFHs that actually adopted it. What about non-adopters whose food security would have decreased if they had adopted. Should they be encouraged to use the SCPs and practice irresponsible farming practices? Not necessarily. For example, off-farm income sources are more lucrative and help relieve capital constraints to adoption. Likewise, farm mechanization also encourages adoption. From this perspective, an exclusive adoption of CMBs appears complementary to other smallholder farming inputs. Therefore, a policy that helps non-adopters become mechanized and increases off-farm income may facilitate adoption—with positive synergies on food security outcomes and sustainable development.
correction
This article has been republished with minor changes. These changes do not impact the academic content of the article.
Authorship
This paper is co-authored by Bernhard Brümmer (BB) and Jan Barkmann (JB): Muhammad Bilal (MB) and JB conceptualized the design of the research. MB carried out survey and collected data. MB analysed data and interpreted. BB and JB assisted in interpretations, constructive comments, and important feedbacks at various stages of the research and drafting of this paper.
Acknowledgements
We gratefully thank the financial support of the fiat panis Foundation (project no: 29/2017) and scholarship from the Higher Education Commission of Pakistan (reference: 91591599). We also gratefully thank the consultation support of German Academic Exchange Service (DAAD). The suggestions by Dr Marwan Benali and Prof. Xiaohua of George August University, Goettingen, Germany are also gratefully acknowledged.
Disclosure statement
No potential conflict of interest was reported by the author(s).
Additional information
Funding
Notes on contributors
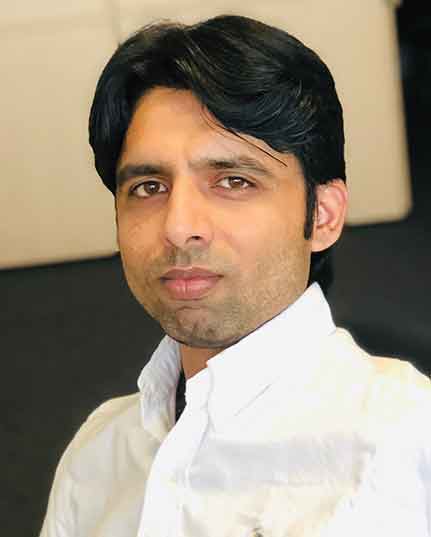
Muhammad Bilal
Dr Muhammad Bilal PhD, He is currently a lecturer at the School of Business & Economics, Westminster International University in Tashkent, Uzbekistan. He got PhD from Georg-August-University Göttingen, Germany. His research interest includes different aspects of food systems, sustainable development, innovation in agricultural technologies, smallholders, and multinational corporations in agriculture sector.
Notes
1. Tehsil is below the district administrative unit with a formal government in context to Pakistan.
2. The estimations with continuous dependent outcomes variable require movestay command (Lokshin & Sajaia, Citation2004) and also require fulfilling the assumption of exclusion restriction to validate selection instruments (Aakvik et al., Citation2005), regretfully, we failed to find the sufficient selection instruments for the continuous dependent variable for outcomes equation.
3. We used switch_probit command (Lokshin & Sajaia, Citation2011) that is flexible for binary dependent outcomes variable. The estimations were carried out using STATA version 15.
4. We acknowledged the potential endogeneity in the variables i.e., off-farm income sources and the ownership of farm machinery in outcomes equation, failure to find a sufficient instrument, we drop all potentially endogenous variables in outcomes equation from the main model, but interestingly, the magnitude and sign of TT and TU remains almost the same (see, Tables in Appendix A). Therefore, based on highly significant likelihood ratio test LR (12) = 35.99 at less than 1%, we include these variables in the main model presented above.
5. We also investigated potential endogeneity in the variable access to credit for the controlled models, therefore, we use the variable credit received in the main model presented above, which translates the number of respondents who actually received credit. The negative sign associated with credit received can be interpreted as respondents who actually received credit are those with low food security status (higher HFIAS score) than those who do not actually received credit (Di Falco et al., Citation2011).
6. For robustness, we separately executed a model for exclusive CMBs adopters versus exclusive SCPs adopters (N = 222). We have found similar evidence of the negative and the significant value of TU and the positive and significant value of TT on food security status but a slight difference in magnitude. That further validates and proved our hypothesis of positive food security effects of exclusive CMBs adoption (see, Table ).
References
- Aakvik, A., Heckman, J. J., & Vytlacil, E. J. (2005). Estimating treatment effects for discrete outcomes when responses to treatment vary: An application to Norwegian vocational rehabilitation programs. Journal of Econometrics, 125(1–2), 15–21. https://doi.org/10.1016/j.jeconom.2004.04.002
- Abdulai, A., & Huffman, W. (2014). The adoption and impact of soil and water conservation technology: An endogenous switching regression application. Land Economics, 90(1), 26–43. https://doi.org/10.3368/le.90.1.26
- Abdullah, A. (2010). An analysis of Bt cotton cultivation in Punjab, Pakistan using the agriculture decision support system (ADSS). AgBioForum, 13(3), 274–287. http://hdl.handle.net/10355/8975
- Aga, A. (2019). The marketing of corporate agrichemicals in Western India: Theorizing graded informality. The Journal of Peasant Studies, 46(7), 1458–1476. https://doi.org/10.1080/03066150.2018.1534833
- Aktar, W., Sengupta, D., & Chowdhury, A. (2009). Impact of pesticides use in agriculture: Their benefits and hazards. Interdisciplinary Toxicology, 2(1), 1–12. https://doi.org/10.2478/v10102-009-0001-7
- Aldosari, F., Al-Shunaifi, M. S., Ullah, M. A., Muddassir, M., & Noor, M. A. (2019). Farmers’ perceptions regarding the use of Information and Communication Technology (ICT) in Khyber Pakhtunkhwa, Northern Pakistan. Journal of the Saudi Society of Agricultural Sciences, 18(2), 211–217. https://doi.org/10.1016/j.jssas.2017.05.004
- Alston, J. M. (2010). The benefits from agricultural research and development, innovation, and productivity growth. Food, Agriculture And Fisheries Papers, No. 31, OECD Publishing, Paris. https://doi.org/10.1787/5km91nfsnkwg-en
- Ament, J. M., Freeman, R., Carbone, C., Vassall, A., & Watts, C. (2020). An empirical analysis of synergies and tradeoffs between sustainable development goals. Sustainability, 12(20), 1–12. https://doi.org/10.3390/su12208424
- Anandajayasekeram, P. (2011). The role of agricultural R&D within the agricultural innovation systems framework. Agricultural R&D – Investing in Africa’s future: Analyzing Trends, Challenges, and Opportunities, Accra, Ghana: ASTI, FARA. Conference Working Paper 6 (pp. 33).
- Antle, J. M., & Pingali, P. L. (1994). Pesticides, productivity, and farmer health: A Philippine case study. American Journal of Agricultural Economics, 76(3), 418–430. https://doi.org/10.2307/1243654
- Asfaw, S., Shiferaw, B., Simtowe, F., & Lipper, L. (2012). Impact of modern agricultural technologies on smallholder welfare: Evidence from Tanzania and Ethiopia. Food Policy, 37(3), 283–295. https://doi.org/10.1016/j.foodpol.2012.02.013
- Ashraf, S., Khan, G. A., Ali, S., & Iftikhar, M. (2015). Socio-economic determinants of the awareness and adoption of citrus production practices in Pakistan. Ciência Rural, 45(9), 1701–1706. https://doi.org/10.1590/0103-8478cr20131227
- Bakhsh, K., Akram, W., Jahanzeb, A., & Khan, M. (2016). Estimating productivity of bt cotton and its impact on pesticide use in Punjab (Pakistan). Pakistan Economic and Social Review, 54(1), 15–24. https://pesr.econpu.edu.pk/website/journal/article/607437ead88ed/page
- Bellemare, M. F. (2010). As you sow, so shall you reap: The welfare impacts of contract farming. Munich Personal RePEc Archive (MPRA) Paper No. 23638. https://mpra.ub.uni-muenchen.de/id/eprint/23638
- Bilal, M., & Barkmann, J. (2019). Farm and farmer capital foster adoption of improved quality agro-chemical inputs in the cotton-wheat zone of the Punjab, Pakistan. Journal of Agriculture and Rural Development in Tropics and Subtropics, 120(2), 229–240. https://doi.org/10.17170/kobra-20191217881
- Bilal, M., Barkmann, J., & Brummer, B. (2022). The role of crop protection products of multinational brands for agricultural sustainability in the cotton-growing zone in Pakistan. Journal of Agriculture and Rural Development in Tropics and Subtropics, 123(1), 1–11. https://doi.org/10.17170/kobra-202201195568
- Carvalho, F. P. (2017). Pesticides, environment, and food safety. Food and Energy Security, 6(2), 48–60. https://doi.org/10.1002/fes3.108
- Chinnakali, P., Upadhyay, R. P., Shokeen, D., Singh, K., Kaur, M., Singh, A. K., Goswami, A., Yadav, K., & Pandav, C. S. (2014). Prevalence of household-level food insecurity and its determinants in an urban resettlement colony in North India. Journal of Health, Population, and Nutrition, 32(2), 227–236. https://europepmc.org/article/MED/25076660
- Coates, J., Swindale, A., & Bilinsky, P. (2007). Household Food Insecurity Access Scale (HFIAS) for measurement of food access: Indicator guide, version 3. Food and Nutrition Technical Assistance Project (FANTA), managed by FHI360. https://doi.org/10.1037/e576842013-001
- Dedehouanou, S. F. A., Araar, A., Ousseini, A., Harouna, A. L., & Jabir, M. (2018). Spillovers from off-farm self-employment opportunities in rural Niger. World Development, 105(2018), 428–442. https://doi.org/10.1016/j.worlddev.2017.12.005
- Di Falco, S., Veronesi, M., & Yesuf, M. (2011). Does adaptation to climate change provide food security? A micro-perspective from Ethiopia. American Journal of Agricultural Economics, 93(3), 829–846. https://doi.org/10.1093/ajae/aar006
- Elias, A., Nohmi, M., Yasunobu, K., & Ishida, A. (2013). Effect of agricultural extension program on smallholders’ farm productivity: Evidence from three peasant associations in the highlands of Ethiopia. Journal of Agricultural Science, 5(8), 163–181. https://doi.org/10.5539/jas.v5n8p163
- FAO. (2018). Transforming food and agriculture to achieve the SDGs. Food and Agriculture Organization of the United Nations.
- Fuglie, K. O., & Bosch, D. J. (1995). Economic and environmental implications of soil nitrogen testing: A switching-regression analysis. American Journal of Agricultural Economics, 77(4), 891–900. https://doi.org/10.2307/1243812
- GFSI. (2021). Global food security index. http://foodsecurityindex.eiu.com/Index
- The Government of Pakistan. (2022). Pakistan economic survey 2021-20. https://www.finance.gov.pk/survey/chapters_21/02-Agriculture.pdf
- The Government of Punjab. (2015). Punjab development statistics. http://bos.gop.pk/developmentstat
- Hameed, R. A., Ajum, S., & Afzal, M. N. (2017). Effect of glyphosat and paraquat herbicides on weed control and productivity of cotton. Cercetari Agronomice in Moldova, 50(2), 51–56. https://doi.org/10.1515/cerce-2017-0014
- Hashmi, M. S. (2016). Agrochemical and agricultural sustainability: A case study of Pakistan. Agricultural Sciences, 9, 1–9. http://www.agropublishers.com/files/JEAS%209%201.PDF
- Headey, D., & Ecker, O. (2013). Rethinking the measurement of food security: From first principles to best practice. Food Security, 5(3), 327–343. https://doi.org/10.1007/s12571-013-0253-0
- Heckman, J. J., & Vytlacil, E. J. (2007). Handbook of Econometrics (6th ed.). Elsevier BV.
- James, C. (2012). Global status of commercialized biotech/GM crops: 2012. ISAAA Briefs, Brief, 44, 329. https://www.isaaa.org/resources/publications/briefs/44/download/isaaa-brief-44-2012.pdf
- Kabunga, N. S., Dubois, T., & Qaim, M. (2014). Impact of tissue culture banana technology on farm household income and food security in Kenya. Food Policy, 45, 25–34. https://doi.org/10.1016/j.foodpol.2013.12.009
- Kaiser, H. F. (1960). The application of electronic computers to factor analysis. Educational and Psychological Measurement, 20(1), 141–151. https://doi.org/10.1177/001316446002000116
- Kassie, M., Shiferaw, B., & Muricho, G. (2011). Agricultural technology, crop income, and poverty alleviation in Uganda. World Development, 39(10), 1784–1795. https://doi.org/10.1016/j.worlddev.2011.04.023
- Kassie, M., Teklewold, H., Marenya, P., Jaleta, M., & Erenstein, O. (2015). Production risks and food security under alternative technology choices in Malawi: Application of a multinomial endogenous switching regression. Journal of Agricultural Economics, 66(3), 640–659. https://doi.org/10.1111/1477-9552.12099
- Khan, M., Akram, N., Husnain, M. I., Haq Padda, I. U., & Qureshi, S. A. (2011). Poverty environment nexus: Use of pesticide in cotton zone of Punjab, Pakistan. Journal of Sustainable Development, 4(3), 163–173. https://doi.org/10.5539/jsd.v4n3p163
- Khan, A. M., Khan, A. A., Afzal, M., & Iqbal, M. S. (2012). Wheat crop yield losses caused by the aphids infestation. Journal of Biofertilizers & Biopesticides, 03(4), 1–3. https://doi.org/10.4172/2155-6202.1000122
- Khan, J., & Khattak, N. U. R. (2013). The significance of research and development for economic growth: The case of Pakistan. City University Research Journal, 3(2). https://mpra.ub.uni-muenchen.de/id/eprint/56005
- Khan, M., Mahmood, H. Z., & Damalas, C. A. (2015). Pesticide use and risk perceptions among farmers in the cotton belt of Punjab, Pakistan. Crop Protection, 67, 184–190. https://doi.org/10.1016/j.cropro.2014.10.013
- Khan, F. Z. A., Sagheer, M., Hasan, M. U., Tahira Gul, H., Hassan, F., Manzoor, A., & Wahid, A. (2013). Agricultural dynamics in Pakistan: Current issues and solutions. Russian Journal of Agricultural and Socio-Economic Sciences, 20(8), 20–26. https://doi.org/10.18551/rjoas.2013-08.03
- Khonje, M., Manda, J., Alene, A. D., & Kassie, M. (2015). Analysis of adoption and impacts of improved maize varieties in Eastern Zambia. World Development, 66, 695–706. https://doi.org/10.1016/j.worlddev.2014.09.008
- Krishna, V., Euler, M., Siregar, H., & Qaim, M. (2017). Differential livelihood impacts of oil palm expansion in Indonesia. Agricultural Economics, 48(5), 639–653. https://doi.org/10.1111/agec.12363
- Lee, D. R. (2005). Agricultural sustainability and technology adoption: Issues and policies for developing countries. American Journal of Agricultural Economics, 87(5), 1325–1334. https://doi.org/10.1111/j.1467-8276.2005.00826.x
- Lewis, K. E., Grebitus, C., & Nayga, R. M., Jr. (2016). The impact of brand and attention on consumers’ willingness to pay: Evidence from an eye tracking experiment. Canadian Journal of Agricultural Economics/Revue Canadienne D’agroeconomie, 64(4), 753–777. https://doi.org/10.1111/cjag.12118
- Lokshin, M., & Glinskaya, E. (2009). The effect of male migration on employment patterns of women in Nepal. The World Bank Economic Review, 23(3), 481–507. https://doi.org/10.1093/wber/lhp011
- Lokshin, M., & Sajaia, Z. (2004). Maximum likelihood estimation of endogenous switching regression models. The Stata Journal, 4(3), 282–289. https://doi.org/10.1177/1536867X0400400306
- Lokshin, M., & Sajaia, Z. (2011). Impact of interventions on discrete outcomes: Maximum likelihood estimation of the binary choice models with binary endogenous regressors. The Stata Journal, 11(3), 368–385. https://doi.org/10.1177/1536867X1101100303
- McCollum, D. L., Echeverri, L. G., Busch, S., Pachauri, S., Parkinson, S., Rogelj, J., Krey, V., Minx, J. C., Nilsson, M., Stevance, A.-S., & Riahi, K. (2018). Connecting the sustainable development goals by their energy inter-linkages. Environmental Research Letters, 13(3), 033006. https://iopscience.iop.org/article/10.1088/1748-9326/aaafe3
- Muzari, W., Gatsi, W., & Muvhunzi, S. (2012). The impacts of technology adoption on smallholder agricultural productivity in Sub-Saharan Africa: A review. Journal of Sustainable Development, 5(8), 69. https://doi.org/10.5539/jsd.v5n8p69
- Nafees, M., Jan, M. R., & Khan, H. (2008). Pesticide use in Swat valley, Pakistan: Exploring remedial measures to mitigate environmental and socioeconomic impacts. Mountain Research and Development, 28(3/4), 201–204. https://doi.org/10.1659/mrd.1042
- Naseem, A., Omamo, S. W., & Spielman, D. J. (2006). The private sector in agricultural R&D: Policies and institutions to foster its growth in developing countries. ISNAR Discussion Paper 6. International service for national Agricultural research (ISNAR) division.
- Negash, M., & Swinnen, J. F. M. (2013). Biofuels and food security: Micro-evidence from Ethiopia. Energy Policy, 61, 963–976. https://doi.org/10.1016/j.enpol.2013.06.031
- Niles, M. T., Salerno, J. D., & Holland, M. (2018). A cross-country analysis of climate shocks and smallholder food insecurity. PLOS ONE, 13(2), e0192928. https://doi.org/10.1371/journal.pone.0192928
- Noltze, M., Schwarze, S., & Qaim, M. (2013). Impacts of natural resource management technologies on agricultural yield and household income: The system of rice intensification in Timor Leste. Ecological Economics, 85, 59–68. https://doi.org/10.1016/j.ecolecon.2012.10.009
- Nyyssola, M., Pirttilä, J., & Sandström, S. (2014). Technology adoption and food security in subsistence agriculture – Evidence from a group-based aid project in Mozambique. Finnish Economic Papers, 27(1), 1–33. http://www.taloustieteellinenyhdistys.fi/wp-content/uploads/2014/11/fep12014_Nyyssola_pirttila_sandstrom.pdf
- Oerke, E.-C. (2006). Centenary review crop losses to pests. Journal of Agricultural Science, 144(1), 31–43. https://doi.org/10.1017/S0021859605005708
- Pakistan Crop Protection Association. (2016). Pakistan current crop scenario and future opportunities.
- Piesse, J., & Thirtle, C. (2010). Agricultural R&D, technology and productivity. Philosophical Transactions of the Royal Society B: Biological Sciences, 365(1554), 3035–3047. https://doi.org/10.1098/rstb.2010.0140
- Pray, C. E., & Fuglie, K. O. (2001). Private investment in agricultural research and international technology transfer in Asia (Agricultural Economics Report 805). Economic Research Service, US Department of Agriculture.
- Rehman, A., Jingdong, L., Shahzad, B., Chandio, A. A., Hussain, I., Nabi, G., & Iqbal, M. S. (2015). Economic perspectives of major field crops of Pakistan: An empirical study. Pacific Science Review B: Humanities and Social Sciences, 1(3), 145–158. https://doi.org/10.1016/j.psrb.2016.09.002
- Rogers, E. M. (1983). Diffusion of innovations (3rd ed.). Free Press; Collier Macmillan.
- Rotterdam convention. (2019). Rotterdam convention. http://www.pic.int/Countries/CountryProfiles/tabid/1087/language/en-US/Default.aspx
- Rware, H., Kansiime, M. K., & Mugambi, I. (2021). Is radio an effective method for delivering actionable information for responding to emerging pest threats? A case study of fall armyworm campaign in Zambia. CABI Agric Biosci, 2(32), 1–11. https://doi.org/10.1186/s43170-021-00053-8
- Saccone, D., & Vallino, E. (2022). Food security in the age of sustainable development: Exploring the synergies between the SDGs. World Development, 152, 105815. https://doi.org/10.1016/j.worlddev.2022.105815
- Sheikh, S., Iqbal, R., Qureshi, R., Azam, I., & Barolia, R. (2020). Adolescent food insecurity in rural Sindh, Pakistan: A cross-sectional survey. BMC Nutrition, 6(1), 17. https://doi.org/10.1186/s40795-020-00343-w
- Shiferaw, B., Kassie, M., Jaleta, M., & Yirga, C. (2014). Adoption of improved wheat varieties and impacts on household food security in Ethiopia. Food Policy, 44, 272–284. https://doi.org/10.1016/j.foodpol.2013.09.012
- Simtowe, F., Asfaw, S., & Abate, T. (2016). Determinants of agricultural technology adoption under partial population awareness: The case of pigeonpea in Malawi. Agricultural and Food Economics, 4(1), 7. https://doi.org/10.1186/s40100-016-0051-z
- Spielman, D. J., Zaidi, F., Zambrano, P., Khan, A. A., Ali, S., Cheema, H. M. N., Nazli, H., Khan, R. S. A., Iqbal, A., Zia, M. A., Ali, G. M., & Luthe, D. S. (2017). What are farmers really planting? Measuring the presence and effectiveness of Bt cotton in Pakistan. PLOS ONE, 12(5), e0176592. https://doi.org/10.1371/journal.pone.0176592
- Stockholm convention. (2019). Stockholm convention. http://www.pops.int/Countries/StatusofRatifications/PartiesandSignatoires/tabid/4500/Default.aspx
- Tariq, M. I., Afzal, S., Hussain, I., & Sultana, N. (2007). Pesticides exposure in Pakistan: A review. Environment International, 33(8), 1107–1122. https://doi.org/10.1016/j.envint.2007.07.012
- Tesfaye, W., & Tirivayi, N. (2018). The impacts of postharvest storage innovations on food security and welfare in Ethiopia. Food Policy, 75, 52–67. https://doi.org/10.1016/j.foodpol.2018.01.004
- Thirtle, C., Piesse, J., & Gouse, M. (2005). Agricultural technology, productivity and employment: Policies for poverty reduction. Agrekon, 44(1), 37–59. https://doi.org/10.1080/03031853.2005.9523702
- UNDP. (2001). Policy and strategy for the rational use of pesticides in Pakistan, building consensus for action, UNDP/FAO Paper, Rome, Italy.
- Vergragt, P. J. (2006). How technology could contribute to a sustainable world. GTI Paper Series. Tellus Institute.
APPENDIX A
Table A1. Rotated component loading from PCA
Table A2. Correlation of selection instruments with adoption and food security status
Table A3. The relevance and exogeneity conditions for selection instruments
Table A4. Falsification approach to validate selection instrument
Table A5. Regression estimates of adoption of CMBs from a probit model (main model)
Table A6. Treatment effects of CMBs adoption on food security status (reduced model excluding all potentially endogenous variables)
Table A7. Estimates of the endogenous switching probit model (reduced model excluding all potentially endogenous variables)
Table A8. Treatment effects of exclusive CMBs adoption versus exclusive SCPs adoption on food security status (N = 222)