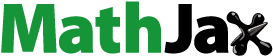
Abstract
Post-harvest losses (PHL) reported in maize production put Sub-Saharan African countries at higher risks of food insecurity. Recent studies reported that higher percentage of PHLs occur during the production stage when farmers are in full control of the crop, suggesting that farmers are not equipped with PHL management skills. This study therefore aimed at assessing the determinants of access to trainings on PHL management among maize farmers in Uganda. Primary data were drawn from 246 randomly sampled farmers in Alebtong District followed by Binary logit analysis. The results depicted that majority of the farmers (58%) did not have access to PHL management trainings. However, those who had access sourced it from extension workers (40.65%), farmers’ groups (22.76%) and farmer-to-farmer trainings (12.20%). The main barriers limiting access to the trainings were unawareness of the PHL trainings and inaccessibility of the training centers. Farm size, group membership, maize output and marital status had positive effect on farmers’ access to PHL management trainings while farm location, and distance to the training centers had a negative effect on access to PHL management trainings. Based on the findings, there is need for public sensitization on the benefits of the PHL trainings, farmers should also be motivated to join farmer-based groups and association where they would learn more about the PHL trainings. In addition, the government should open more training centers and employ more training agents so that many farmers can be reached and trained on how to handle and mitigate PHLs in maize.
Public Interest Statement
Sub-Saharan African countries depend on agriculture as a source of their food. However, due to poor handling of the farm produce by the smallholder farmers, there have been cases of post-harvest losses in crops. This not only reduces both the quality and quantity of crop produce but also renders farm products unsafe for human consumption. The author is therefore interested in how farmers can be trained on PHL management in order to reduce post-harvest losses. In addition, my interest lies on research targeting agricultural productivity such as crop diversification, optimum resource allocation among farming enterprises, technical, economic and allocative efficiency, cost–benefit analysis, adoption of agricultural technologies so as to improve the living standards of smallholder farmers.
1. Background
The growing population in Uganda, a low-income country in East Africa, calls for advanced investment in agriculture not only provide to food for the population but also to boost the economic development of this country in order to propel it to a middle-income country (MAAIF, Citation2016; Shively & Hao, Citation2012). Having sustainable climatic conditions and fertile soils in different areas, this country has the potential, which has not been fully utilized, to produce both food and cash crops (MAAIF, Citation2017; Mwaura & Adong, Citation2016). While food crops play a major role in ensuring that her citizens have adequate food, cash crops on the other hand are tasked with income generation and economic development roles (Shively & Hao, Citation2012).
The government of Uganda encourages smallholder farmers to produce maize since it is a multipurpose crop which serves as both food security and income generation (MAAIF, Citation2020). Many Ugandans depend on maize as one of their staple foods among other crops such as cassava, sweet potatoes, beans and matoke (Midamba, Citation2022). Being a staple food, this crop dominates almost all the regions in Uganda and grown by more than 55% of the households (MAAIF, Citation2017, Citation2020). Annually, Uganda produces approximately 3.4 million metric tons of maize (FAO, Citation2018; UBOS, Citation2018a).
Despite the significant benefits maize to Ugandan economy in terms of household food security and economic development, this crop suffers from post-harvest losses (PHLs). According to Shee et al. (Citation2019), PHLs in maize occurs during harvesting, dehusking transporting to their homes, drying, shelling, storage, milling and selling. Shee et al. (Citation2019) further noted that a higher percentage of PHLs occur from harvesting to shelling when the farmer is in full control of maize. Abass et al. (Citation2014) confirmed that indeed PHLs in maize production occur in the field, during processing and during maize storage. Moreover, Yeshiwas and Tadele (Citation2021) on the other hand illustrated that transportation and storage of crops are the major loopholes to PHLs due to the invasion by storage pests such as weevils. Debebe (Citation2022) noted that 64% of the farmers in Ethiopia suffered the problems of PHLs among different crops with a higher loss when the crops are in the fields. Sugri et al. (Citation2021) reported that PHLs occur mostly in cow peas, groundnuts and maize in Ghana with groundnuts recording a higher percentage of PHL during the production stage. Kumar and Underhill (Citation2019) present a PHL ranging between 26.1 to 27.6% among tomato farmers in Fiji Islands, also when the crops are with the farmers. Arah et al. (Citation2015) present that PHLs in tomatoes occurs during the pre-harvesting activities when the crops are in full control of the smallholder farmers. The above studies clearly reveal that PHLs is not only evident in maize but also in other crops.
The findings of these studies suggest that farmers may not be handling their crops appropriately and thus contributing to PHLs among different crops. The poor handling of crops by the farmers may be attributed to lack of PHL management skills among the farmers. This is evident in the study of Abass et al. (Citation2014), who confirmed that majority of smallholder farmers lack the skills to reduce PHLs for their crops and thus a reason for the crop loss when it is in the hands of the farmers. However, one of the major proposed solutions to reducing PHLs is training farmers on the ways of controlling, managing and adoption strategies that help in reducing PHLs. Furthermore, from Abass et al. (Citation2014) findings’ that farmers lack inadequate skills to reduce PHLs, it is clearly evident that the government of Sub-Saharan African countries should invest in farmers’ trainings and demonstrations aiming at equipping them with practical skills for PHL management. Similarly, Shee et al. (Citation2019) noted that formal trainings and field demonstrations on PHL are highly desirable in order to reduce PHLs. Moreover, Dzanku and Osei (Citation2018) observed that farmers’ trainings and follow ups on PHLs are the significant ways of reducing PHLs in Sub-Saharan Africa.
However, trainings on PHL management require empirical studies that explain the characteristics of the farmers, level of access to the PHL trainings, barriers limiting access to the trainings and the determinants of access to PHL management trainings. Existing literature such as Abass et al. (Citation2014), Amanullah et al. (Citation2020), Arah et al. (Citation2015), Debebe (Citation2022), Shee et al. (Citation2019), and Yeshiwas and Tadele (Citation2021) focus on the socio-economic factors affecting PHLs but fail to clearly point out the determinants of access to trainings on PHL management among farmers as one of the solutions to curbing PHLs in crops. Therefore, the contribution and novelty of this study is that, unlike existing literature which only focus on the determinants of PHLs such as (Abass et al., Citation2014; Amanullah et al., Citation2020; Arah et al., Citation2015; Debebe, Citation2022; Shee et al., Citation2019; Yeshiwas & Tadele, Citation2021), the study provides results on the frequency of farmers with access to PHL management trainings, barriers of access to PHL trainings as well as the socio-economic determinants of access to trainings on PHL management as a way of mitigating PHLs in maize production. In addition, the paper contributes to the policy measures to reduce PHL in maize production by providing policy recommendations to be implemented by the various institutions.
2. Literature review
2.1. Sources and impacts of post-harvest losses in crops
The negative effects of loss of part of or the whole crop reduces both the quality and quantity of the crop as well as rendering it unsafe for both human and animal consumption. In order to deeply understand the origin of PHLs in crops, past studies have tried to research on the sources of PHLs among different crops.
A study done by Shee et al. (Citation2019) on the determinants of PHLs in maize and sweet potatoes reported that PHLs mainly occur during the harvesting, dehusking, transportation and storage, resulting into reduction in both quality and quantity of the crop. Similarly, Arah et al. (Citation2015) reported that PHLs are associated with the farm activities conducted by the farmers prior to and after harvesting. They presented that pre-harvesting factors such as fertilizer application, pruning, maturity stage, irrigation, cultivation types are associated with the PHLs in tomato production. On the other hand, post-harvesting factors such as temperature, relative humidity, combination gases, physical handling and post-harvest chemical application contributes to PHLs in tomatoes.
Similarly, Yahaya and Mardiyya (Citation2019) conducted a review study to determine the sources of PHLs in fruits and vegetables. Their findings indicated that PHLs are caused by mechanical damage which occurs when the crops are not well handled; microbial factors which are attributed to bacterial and fungal attack resulting into rotting, reducing the quality and quantity of the crop; and environmental factors such as climatic change resulting into high or low temperature and humidity.
On the impacts of PHLs in crops, Ismail and Changalima (Citation2019) noted that if PHLs in maize are controlled, farmers would increase their income, implying that PHLs reduces the level of income to the farmers. A review study conducted by Bekele (Citation2021) indicates that one of the catastrophic impacts of PHLs in crops is food insecurity. They observed that excess PHLs puts Sub-Saharan Africa into dire levels of food insecurity. Similarly, Affognon et al. (Citation2015) reported that household food security in African can be boosted by controlling PHLs in crop produce.
In conclusion, the above studies reported that the impacts of PHLs include food and nutritional insecurity as well and reducing income among farmers. They also noted that PHLs in crops is attributed to both human and climatic factors. While climatic factors cannot be easily dealt with especially in East Africa, human factors can be mitigated through training farmers on how to handle PHLs. Thus, the study seeks to understand the determinants of access to trainings on PHLs among maize farmers in Uganda in order to inform policy makers on how to control PHLs.
2.2. Determinants of access to trainings among farmers
Food security and economic development in Sub-Saharan African depend on agricultural production (FAO, Citation2002). When farmers intensively invest in agriculture, they help in reducing food crisis as well as helping the government in boosting economic development. While the world is still healing from the impacts of Covid-19 as well as the Russia -Ukraine war on agriculture (Ouko et al., Citation2020), especially on SSA countries, there is need to preserve what is already produced by the farmers to boost food security. This is achieved when farmers reduce the rate of PHLs in crops. Yang et al. (Citation2021) noted that in order to reduce the rate of PHLs in crops, farmer-based trainings on how to mitigate PHLs is highly desirable. A study by Manzoor et al. (Citation2020) on the training needs and gaps among the farmers revealed that majority of the farmers are in dire need of PHL trainings in order to preserve their produce. However, to achieve this, having an understanding of the determinants of access to such trainings among the farmers is highly recommended.
A study by Alemu (Citation2021), which mainly aimed at unfolding the socio-economic determinants of trainings participation by farmers, reported that education increases the rate of farmers’ access and participation in training programs. They attributed this to the fact that as farmers spend more years in school, they increase their level of awareness on the benefits of training program son agricultural productivity. As such, they recommended strengthening of adult literacy programs to increase the literacy levels among the farmers.
Adeyanju et al. (Citation2021) conducted a study to determine the factors affecting post-harvest management strategies which includes trainings in Northern Ghana. Their findings clearly depicted that variables such as education and volume of farm output were positively related to management of PHLs. They also reported a negative effect of family size, age, distance to the capital city on PHL management strategies.
In order to formulate policies that improve access to trainings on agro-processing, Mthombeni et al. (Citation2022) carried out a study on 307 smallholder crop farmers in South Africa. Their findings indicated that as the level of farming experience increases, the rate of access to the trainings also increases. Specifically, they reported that highly experienced farmers had 2.7% higher chances of access to trainings than their fellows who had less years of experience. This was attributed to the increased knowledge, skills and awareness available to the experienced farmers than the non—experienced counterparts.
A recent study by Adeyanju et al. (Citation2021) reported that age, years spent in school, extension contact and location were the major factors which positively contributed to the participation in training program among the youths. They also noted that gender and household size significantly reduced the level of youth participation in agricultural training programs.
In conclusion, the reviewed literature depicts that different socio-economic factors affect access to trainings among the farmers. The reviewed literature also revealed that the studies were done in different areas. These areas have difference in governance, policies, farming methods, climatic conditions among others. As such a policy which works in Africa may not necessarily apply to other continents. This, therefore, calls for more empirical studies on determinants of farmers’ access to PHL management trainings in order to formulate specific policies for specific regions. In addition, this study was propelled by the varied results presented in the related literature. Finally, PHLs have been widely researched on especially on the determinants of PHLs in crops. However, there are limited studies on the determinants of access to trainings on PHL management as a strategy of mitigating PHLs. In order to bridge the gap in the literature, the study determined the frequency of farmers who could access the trainings, characterized those with and without access to the PHL trainings and finally finalized by presenting the determinants of access to PHL trainings among maize farmers in Uganda.
2.3. Methods for measuring access to trainings on PHL management
Researchers such as Abdallah and Abdul-Rahaman (Citation2019 Ainab (Citation2019), Atsbeha and Gebre (Citation2021), Saqib et al. (Citation2018), Midamba et al. (Citation2022), Mohamed and Temu (Citation2008), and Ojo et al. (Citation2012) have categorized the term “Access” under binary variables. They have also explained that binary variables normally take the values of 0 and 1 for the farmers who have access and their counterparts who do not have access to agricultural trainings.
In cases where the dependent variables are binary or dichotomous in nature, two methods are available in the literature to explain the relationship and magnitude between the binary dependent variable and the hypothesized socio-economic variables or determinants such as age, gender, household size, farm size, market distance, education, farming experience, among others. These methods include Binary logistic regression model (Adeyanju et al., Citation2021; Alemu, Citation2021; Atube et al., Citation2021; Nahayo et al., Citation2017; Njenga et al., Citation2021; Ojo et al., Citation2012; Okeyo et al., Citation2020) and Probit regression models (Asante et al., Citation2011; Baird et al., Citation2003; Bonabana-Wabbi et al., Citation2016; Martey et al., Citation2014; Tolno et al., Citation2015). While the two models are similar in many ways, Binary logistic regression model has an advantage over the Probit model since it predicts the outcome much better than the Probit model (Jose et al., Citation2020). Similarly, Greene and Hensher (Citation2010), the other advantage of Binary logit over Probit model due to the computational complexity which arises when there is lack of a closed form for the normal cumulative density function of which the probit model is based.
2.4. Conceptual framework
The conceptual framework presented in Figure below describes the causal relationship between the explained and the explanatory variables. It depicts that a farmer would choose to attend or access trainings on PHL management if he perceives such trainings as beneficial to him. From the conceptual framework below, the study considers farmers’ related factors such as family size, education, farming experience, marital status, gender of the household head and distance to the training centers (Adeyanju et al., Citation2021; Mthombeni et al., Citation2022). Similarly, the considered farm related factors include farm size, farm location and maize output (Adeyanju et al., Citation2021). Finally, the only institutional factor considered in the study is group membership (Weber, Citation2020). From the literature review, these factors are hypothesized to be having either a positive or negative relationship with access to PHL management trainings.
3. Methodology
3.1. Study area
Due to the high number of maize farmers (UBOS, Citation2018a), Alebtong District was considered for the study. It is located in the central part of Uganda (Figure ) and approximately 285 kilometers away from Kampala, the largest city in Uganda (UBOS, Citation2017). Akura and Abia sub-counties were specifically considered for data collection due to the high maize volumes of production (MAAIF, Citation2017). Majority of the population in this district source their livelihood from agriculture, hence agriculture is their main economic activity. It has 5 sub-counties and 35 parishes. This district has a total population of 225, 327 persons. Its coordinates are 02 18 N, 33 18E (UBOS, Citation2017).
3.2. Data sources, sampling and sample size
Simple random sampling was adopted to collect data from the farmers from the two counties; Abia and Akura counties. The sampling frame consisted on 246 active maize farmers. 123 farmers were randomly considered in Akura while the rest 123 were also randomly selected from Abia. Prior to the actual data collection, we took care of the reliability, relevance and validity of the study tool by pretesting it on randomly selected 20 farmers in Jinja district as suggested by (Chune et al., Citation2022). Consequently, primary data were then collected from the farmers using the pretested tool. Farmers’ socio-demographics, access to PHL trainings and the sources of such trainings, and constraints to access to PHL trainings were all captured in the tool. The total number of study participants were arrived at using the formula below.
Where, N represents the sample size, Z represents the z scores at 95% confidence level (1.96), P represents the population proportion of maize farmers in Alebtong district which is approximate to be at 0.80 according to UBOS (Citation2018b), while M is the margin of error at 5%. In total N had a value of 246 as the sample size. Collected data were then subjected to analysis using Stata version 13 software.
4. Methods of analysis
4.1. Binary logistic model
Farmers who had access to trainings on PHL management were compared to their counterparts who did not have access to trainings on PHL management. This was done using t-test (continuous variables) and Chi2 test (categorical variables) as recommended by Ahmed et al. (Citation2022). Descriptive statistics were also used to determine the number of PHL management practices adopted by the farmers.
The explained variable in this study was dichotomous (binary) with 1 and 0 values for the farmers with and without access to PHL management trainings, respectively. This is based on the fact that a farmer considers such trainings as beneficial to him and therefore he attends them. Previous studies have presented Binary logistic and Probit models as suitable in explaining the relationship between the binary dependent variable and set of hypothesized independent variables (Ahmed et al., Citation2022; Bilaliib Udimal et al., Citation2017; Mugwe et al., Citation2009) . However, according to Ahmed et al. (Citation2022), Binary logistic model is preferred over Probit model because it is computationally easier to use. In addition, Ahmed et al. (Citation2022) noted that Binary logit lends itself to a meaningful interpretation than other models. Based the relative advantages of Binary logit over Probit model, Binary logit was adopted in this study. Other past studies which have used Binary logit in agriculture include (Akudugu et al., Citation2012; Assogba et al., Citation2017; Bilaliib Udimal et al., Citation2017; Kemboi et al., Citation2020; Kurdyś-Kujawska et al., Citation2021; Odendo et al., Citation2009). EquationEquation 1(1)
(1) illustrates the probability of a farmer to have access to PHLs management trainings as noted by Jaza et al. (Citation2018).
Aryal et al. (Citation2020) presented that the probability of having no access to PHLs trainings is given in Equationequation 2(2)
(2) ;
Endalew and Yenewa (Citation2021) and Jegede (Citation2020), presented that Binary logistic regression is then specified in Equationequation 3(3)
(3) as a result of combining Equationequation 1
(1)
(1) and Equation2
(2)
(2) as;
In Equationequation 3(3)
(3) above,
is the probability of having access to PHL management training,
is the constant term,
represents the regression coefficients, which will be obtained from the regression result,
represents the explanatory variables. These are the hypothesized explanatory variables with their apriori sign expectations as presented in Table . Finally,
is the error term. EquationEquation 4
(4)
(4) presents binary logit marginal effects obtained by differentiating Equationequation 2
(2)
(2) with respect to Xk
Table 1. Explained and explanatory variables
5. Results and discussion
5.1. Number of farmers with access to training on PHLs management
Presented in Figure are the results for the number of farmers who had access to training on PHLs management, only 103 (42.00%) farmers out of the 246 sampled farmers reported that they indeed received trainings. The rest 143 (58.00%) farmers did not receive any training and were dependent on their own trial and error methods. This confirms that indeed farmers need to be trained on modern agriculture in order to boost their farm yields.
Figure 3. Frequency of farmers with access to PHL management trainings.
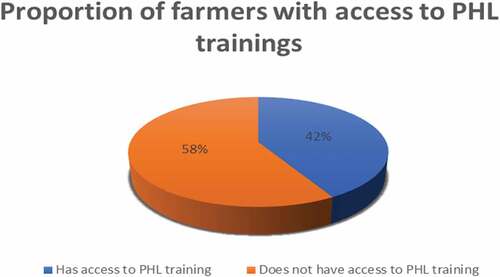
The results presented in Table below show that farmers received PHL management trainings from three major sources. These included farmers’ groups, extension workers and from their fellow farmers through demonstrations. Majority of the farmers (40.65%) sourced the trainings from extension agents while only 12.20% of the farmers received their trainings from their fellow farmers. Farmers’ group trainings attracted only 22.76% of the farmers. This implies that farmers trainings should also be intensified through farmers’ groups.
Table 2. Sources of PHL trainings
5.2. Comparison of farmers with and without access to PHL management trainings
The results presented in Table show that farmers who had access to PHL management trainings had significantly (P < 0.01) higher yields than their counterparts who never received the training. Similarly, more acres of land were significantly (P < 0.01) owned by farmers who had access to trainings than their fellows who had no access to the trainings. Based on the study results, it is clearly evident that farmers with access to PHL management trainings significantly (P < 001) spent more years in school than their counterparts. As farmers’ age increases, assuming that they continuously produce, their farming experience also increases. Farmers who had access to PHL management trainings had significantly (P < 0.01) more years of farming and were also significantly (P < 0.01) older than their fellows who did not receive any training. Institutionally, we observed that there were significantly (P < 0.05) many farmers who had access to credit as well as PHL management trainings than those who did not have access to the trainings.
Table 3. Comparison of access to PHL trainings across farmers’ socio-demographics
In terms of household food security, the study found out that there were significantly (P < 0.05) many farmers who received PHL management trainings and were food secure than those who did not receive the trainings on PHLs. Thus, PHL management trainings may be helping in increasing food availability. Finally, the findings show that there was significantly (P < 0.01) higher rates of PHLs among farmers who never attended the trainings than their counterparts.
5.3. PHL management practices adopted by the farmers
Amanullah et al. (Citation2020) reported that harvesting, drying, sorting, packaging, transportation and marketing are the major PHL management practices which should be adopted by maize farmers. In this study, as presented in Table , we considered the above practices plus addition of application of chemicals to control pests and diseases before storing maize. Harvesting maize in time helps to prevent rotting and destruction by the rains, hence was considered in this study. Fortunately, the results showed that majority of the farmers (75.60%) harvested their maize from the farms in time. Drying the grains helps to reduce moisture content so that the grains may not rot during the storage periods. Strikingly, the results showed the only 44.72% of farmers dried their grains. The results further showed that only 20.33% of the farmers sorted their grains to remove the spoilt and other materials so as to remain with the clean maize, implying that majority of the farmers did not adopt this practice. Packaging was done by only 12.20% which is below average. Application of chemicals before storing the grains is a recommended practice to control PHL due to weevils and other pests’ attack. Unfortunately, this practice attracted only 27.64% of the farmers. Fortunately, all the farmers shipped their produce to their homes. However, on transportation to the markets, only 65.05% shipped their produce to the markets since some millers purchased the grains from their homes directly. Finally, the results presented a total of only 18.70% of the farmers as the only ones who marketed their produce. In conclusion, it is clearly shown that majority of these practices attracted below average number of farmers, thus putting their maize at a higher risk of PHLs.
Table 4. PHL management practices adopted by the farmers
5.4. Barriers impeding access to trainings on PHL management
Farmers presented two major concerns on why they were not able to access the trainings on PHLs (Figure ). They reported that lack of knowledge on the trainings was the major reason why they did not attend such trainings (Fahad & Wang, Citation2018). In addition, the farmers also reported that they were located too far from the training centers where the PHL trainings were conducted. As such, accessing the training centers was a challenge to them since they had to spend money on transport to reach the trainings venues in time.
5.5. Determinants of access to trainings on PHLs management
5.5.1. Summary of the binary logit model fit
The model was generally significant at 1% level of significance based on the results shown in Table . Mbachu et al. (Citation2012) clearly elaborates that the “extremely good” range of pseudo-R squared ranges from 20% to 40%. Our model, having a pseudo-R squared of 38.50, was therefore extremely good. The log likelihood ratio stood at −101.60. It is therefore right to conclude that the results of the model fit were adequate enough for this study.
Table 5. Binary logit estimates of the determinants of access to PHL trainings
The results from binary logit for the determinants of access to training on PHL management are presented in Table . From the results, it is shown that 70% of the explanatory variables considered in this study had significant influence on the farmers’ access to PHL management trainings. The number of people living in a household showed a negative and significant (P < 0.10) association with access to farm trainings. Large families had 9.1% lower probability of accessing trainings on PHL management. The inverse relationship between family size and access to trainings is attributed to the fact that large families are much concerned with family issues such as food provision, school fees, among others than trainings on PHL management. This is consistent with the findings reported by Eticha (Citation2021); Ojo et al. (Citation2012).
Farmers with large portions of land had a 55.4% significantly (P < 0.10) higher probability of accessing PHL management trainings. This depicts that indeed farm size is positively related to access to farm trainings on PHL management. This can be linked to the fact that majority of the farmers who had large portions of land were producing maize mainly for income generation. This, therefore, implies that the farmers were able to access trainings and workshops on PHL management so that they could increase their income from maize, unlike those who had small portions of land for domestic maize production. This is in agreement with the findings of Abdallah and Abdul-Rahaman (Citation2019); Alemu (Citation2021).
Farmers’ groups have increased significantly over the past years. These groups serve as communication, sales and training platforms for smallholder farmers. Farmer to farmer trainings are easily coordinated when farmers come together to join groups. In this study, group membership was considered as one of the positively hypothesized variables for the determinants of access to PHL management trainings. Indeed, the results confirmed this by depicting a positive effect of group membership on access to PHL management trainings among maize farmers. It is observed that group members had 12.8% significantly (P < 0.05) higher probability of accessing the trainings than their fellow non-group members as shown in the marginal effects’ results. This is similar to the findings reported by Abdallah and Abdul-Rahaman (Citation2019), Banik et al. (Citation2013), Pratiwi and Suzuki (Citation2017), and Vu et al. (Citation2020).
Similarly, the results from marginal effects depicted that a unit increase in maize output was associated with 1.2% significantly (P < 0.10) higher probability of accessing PHL management trainings. The positive effect of maize output on access to PHL management trainings was fueled by fact that as farmers obtain more yields, they are motivated to prevent the losses through accessing farm trainings targeting the prevention of such losses so as to increase farm income. This is in agreement with the findings of Nakano et al. (Citation2018); Pratiwi and Suzuki (Citation2017).
Married farmers had 11.5% significantly (P < 0.05) higher probability of accessing the trainings than their fellow unmarried farmers. This is due to the encouragements and, roles, tasks and responsibilities sharing which increase the level of access to the PHL management trainings among married farmers than the unmarried. This agrees to the related reports presented by Badstue et al. (Citation2020); Ngeywo et al. (Citation2015).
Majority of the smallholder farmers were constrained by communication barriers (Awili et al., Citation2016) since they were located deep in the rural areas. In extreme cases, there are no formal roads leading to where these farmers reside, resulting into low access to agricultural services. As such, farmers located near the training centers have a relative advantage of accessing PHL trainings easily and timely than their fellows who had to travel long distance to reach the training venues as shown by the positive and significant (P < 0.05) association between distance to the training centers and access to PHL trainings. This is similar to the findings by Abdallah and Abdul-Rahaman (Citation2019); Alemu (Citation2021).
Farmers located in Abia had 55.4% significantly (P < 0.01) lower probability of accessing PHL trainings than their fellows in Akura sub-county. This was attributed the fact that Abia farmers were located deep in the villages hence accessing the trainings areas was a challenge to them. Furthermore, those who were able to access the trainings spent money on transport to reach the training venues. Akura farmers on the other hand enjoyed the easy access to the trainings due to being located near the trainings centers. The negative influence of farm location on access to PHL trainings were also noted by ; Zangeneh et al. (Citation2015).
6. Conclusion
Past studies indicate that the high PHLs occur mostly when maize is in full control of the farmers. This suggests that farmers may be exposing their maize produce to the PHLs. In addition, existing literature confirm that majority of the smallholder farmers lack the necessary skills to manage PHLs, a situation which calls for extensive farmer-based trainings and demonstrations on strategies for eradicating the PHLs. In order to achieve this, having a knowledge on the determinants of access to PHLs management trainings among the smallholder farmers is highly beneficial.
This study therefore presents the determinants of farmers’ access to trainings on PHL management among maize farmers. Primary data were drawn from 246 randomly selected maize farmers followed by binary logit analysis. The results depicted that minority of the farmers had access to trainings on PHL management. Furthermore, farmers who had access to the trainings were better off in terms of household food security and farm output than their counterparts. Binary logit estimates showed that family size, farm size, access to credit, group membership, maize yield, marital status and distance to the training centers were the significant determinants of access to trainings on PHL management trainings in maize production. The study therefore concluded that the level of access to PHL management trainings is low among maize farmers in Uganda. The major barriers to accessing the trainings include lack of knowledge about the existence of such trainings and inaccessibility of the training venues. Lastly, the study concluded that the farm size, group membership, maize output and marital status increases access to trainings on PHLs while family size, farm location and distance to the training centers reduces access to the PHL management trainings.
6.1. Policy recommendations
It is clearly evident that majority of the farmers could not access trainings on PHL management due to the unawareness of the existence of such trainings. As such, public sensitization by the extension agents is highly desirable. The agents should disseminate information on the areas where such trainings are offered and how farmers can access them.
From the positive association between access to trainings on PHL management and group membership, it is clearly evident that farmers who joined groups could access the trainings easily than their counterparts who did not join the groups. It is therefore recommended that the government through extension officers should educate the farmers on the benefits of such groups while also encouraging them to join the groups so as to increase the rate of access to trainings on PHL management.
The negative effect of distance to the training areas on access to trainings implies that farmers who were near the training areas could access the trainings easily than their counterparts located far away from the training areas. This calls for establishment of more training centers so that those farmers who are disadvantaged due as a result of long distances can benefit from the newly established training centers.
There is also need to increase the number of training or extension agents so that many farmers can be reached and trained on how to mitigate PHLs in maize. This will also ensure efficiency of training delivery to the smallholder farmers.
From the inverse relationship between family size and access to trainings on PHL management, it is observed that families with many household members could hardly access trainings on how to manage PHLs in their maize. In order to increase access to trainings on PHLs, the farmers need to be encouraged and motivated to use family planning so as to moderate the population. This will ensure that they do not only concentrate on their children but also on how to reduce PHLs in order to feed their families.
Acknowledgments
The authors acknowledge the support of the Regional Universities Forum for Capacity Building in Agriculture (RUFORUM) for funding the study through the Transforming African Agricultural Universities to meaningfully contribute to Africa’s growth and development (TAGDev) funded by MasterCard Foundation.
Disclosure statement
No potential conflict of interest was reported by the author(s).
Additional information
Funding
Notes on contributors
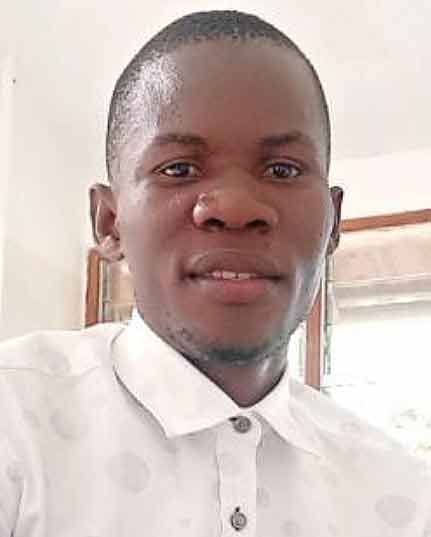
Dick Chune Midamba
Dick Chune Midamba is a Kenyan, currently 27 years old. He started his school at Kakiimba secondary school, where he sat for his secondary education certificate in 2013. He then joined Laikipia University in 2014 for his undergraduate degree in Agricultural Economics, graduated in 2018. In 2019, he was awarded MasterCard Foundation scholarship through TagDev project to further his studies in Gulu University, Uganda. He finished his Masters degree in Agri – Enterprises Development in 2022 from Gulu University, Uganda. Midamba is currently doing his internship at International Centre of Insects Physiology and Ecology (ICIPE) under Push – Pull program, where he learns more about agricultural research. In addition, he is a trainer at Sauti Kuu Foundation, tasked with training young people on how to become agripreneur rather than job seekers.
References
- Abass, A. B., Ndunguru, G., Mamiro, P., Alenkhe, B., Mlingi, N., & Bekunda, M. (2014). Post-harvest food losses in a maize-based farming system of semi-arid Savannah area of Tanzania. Journal of Stored Products Research, 57, 49–17. https://doi.org/10.1016/j.jspr.2013.12.004
- Abdallah, A.-H., & Abdul-Rahaman, A. (2019). Determinants of access to agricultural extension services: Evidence from smallholder rural women in Northern Ghana. Asian Journal of Agricultural Extension, Economics & Sociology, 9(3), 1–8. https://doi.org/10.9734/AJAEES/2016/23478
- Adeyanju, D., Mburu, J., Mignouna, D., & Akomolafe, K. J. (2021). Determinants of youth participation in agricultural training programs: The case of Fadama program in Nigeria. International Journal of Training Research, 19(2), 142–166. https://doi.org/10.1080/14480220.2021.1905685
- Affognon, H., Mutungi, C., Sanginga, P., & Borgemeister, C. (2015). Unpacking postharvest losses in sub-Saharan Africa: A meta-analysis. World Development, 66, 49–68. https://doi.org/10.1016/j.worlddev.2014.08.002
- Ahmed, Y., Ebrahim, S., & Ahmed, M. (2022). Determinants of solar technology adoption in rural households: The case of Belesa districts, Amhara region of Ethiopia Determinants of solar technology adoption in rural households: The case of Belesa districts, Amhara region of Ethiopia. Cogent Economics & Finance, 10(1). https://doi.org/10.1080/23322039.2022.2087644
- Ainab, A. A. (2019). Determinants of access to extension service among cashew nut farmers in Kilifi County – Kenya. International Journals of Academics and Research, 1(2), 177–184. https://doi.org/10.32898/ihssj.02/1.4article14
- Akudugu, M. A., Guo, E., & Dadzie, S. K. (2012). Adoption of Modern Agricultural Production Technologies by farm households in Ghana: What factors influence their decisions? Journal of Biology, Agriculture and Healthcare, 2(3), 1–13. https://www.researchgate.net/profile/Samuel_Dadzie/publication/235751741_Adoption_of_modern_agricultural_production_technologies_by_farm_households_in_Ghana_What_factors_influence_their_decisions/links/00463533b1249ebdf1000000.pdf
- Alemu, A. (2021). Determinants of participation in farmers training centre based extension training in Ethiopia. Journal of Agricultural Extension, 25(2), 86–95. https://doi.org/10.4314/jae.v25i2.8
- Amanullah, A., Nawi, N., Kamarulzaman, N., & Shamsudin, M. (2020). Factors influencing post-harvest losses of apples among growers in Paktia, Afghanistan. Food Research, 4(6), 2313–2321. https://doi.org/10.26656/fr.2017.4(6).302
- Arah, I. K., Amaglo, H., Kumah, E. K., & Ofori, H. (2015). Preharvest and postharvest factors affecting the quality and shelf life of harvested tomatoes: A mini review. International Journal of Agronomy, 2015. https://doi.org/10.1155/2015/478041
- Aryal, J. P., Sapkota, T. B., Rahut, D. B., Krupnik, T. J., Shahrin, S., Jat, M. L., & Stirling, C. M. (2020). Major climate risks and adaptation strategies of smallholder farmers in coastal Bangladesh. Environmental Management, 66(1), 105–120. https://doi.org/10.1007/s00267-020-01291-8
- Asante, B. O., Afari-Sefa, V., & Sarpong, D. B. (2011). Determinants of small scale farmers’ decision to join farmer based organizations in Ghana. African Journal of Agricultural Research, 6(10), 2273–2279. https://doi.org/10.5897/AJAR10.979
- Assogba, P. N., Haroll Kokoye, S. E., Yegbemey, R. N., Djenontin, J. A., Tassou, Z., Pardoe, J., & Yabi, J. A. (2017). Determinants of credit access by smallholder farmers in North-East Benin. Journal of Development and Agricultural Economics, 9(8), 210–216. https://doi.org/10.5897/JDAE2017.0814
- Atsbeha, A. T., & Gebre, G. G. (2021). Factors affecting women access to agricultural extension services: Evidence from poultry producer women’s in Northwestern Tigray, Ethiopia. Cogent Social Sciences, 7(1). https://doi.org/10.1080/23311886.2021.1975413
- Atube, F., Malinga, G. M., Nyeko, M., Okello, D. M., Alarakol, S. P., & Okello-Uma, I. (2021). Determinants of smallholder farmers’ adaptation strategies to the effects of climate change: Evidence from northern Uganda. Agriculture and Food Security, 10(1), 1–14. https://doi.org/10.1186/s40066-020-00279-1
- Awili, M. A., White, P., & Kimotho, S. (2016). Factors that influence effective communication of agricultural information among farmers – The case of farmers in South West Kisumu Ward, Kisumu County. Journal of Developing Country Studies, 1(1), 1–20. https://www.iprjb.org/journals/index.php/JDCS/article/view/21
- Badstue, L., Petesch, P., Farnworth, C. R., Roeven, L., & Hailemariam, M. (2020). Women farmers and agricultural innovation: Marital status and normative expectations in rural Ethiopia. Sustainability (Switzerland), 12(23), 1–22. https://doi.org/10.3390/su12239847
- Baird, S., Maonga, B. B., Assa, M. M., Haraman, E. M. K., Mildred, B., Jackline, B.-W., Pearce, J., Kumar, G. D. S., Popat, M. N., Finlayson, J. D., Lawes, R. A., Metcalf, T., Robertson, M. J., Ferris, D., Ewing, M. A., Moser, C. M., Barrett, C. B., Heilman, P., Malone, R. W., & Division, N. (2003). Assessing factors affecting adoption of agricultural technologies: The case of Integrated Pest Management (IPM) in Kumi District, Eastern Uganda. Journal of Sustainable Development in Africa, 22(3), 146. https://hdl.handle.net/10919/36266
- Banik, N., Koesoemadinata, A., Wagner, C., Inyang, C., & Bui, H. (2013). The impact of group based training approaches on crop yield, household income and adoption of pest management practices in the smallholder horticultural subsector of Kenya. Journal of Sustainable Development in Africa, 15(1), 117–140. https://doi.org/10.1190/segam2013-0137.1
- Bekele, D. (2021). Role of postharvest management for food security: A review. Advances in Crop Science and Technology, 9(7), 1–6. https://www.omicsonline.org/open-access/role-of-postharvest-management-for-food-security-a-review-116715.html%0Ahttps://www.omicsonline.org/peer-reviewed/role-of-postharvest-management-for-food-security-a-review-116715.html
- Bilaliib Udimal, T., Jincai, Z., Mensah, O. S., & Caesar, A. E. (2017). Factors influencing the agricultural technology adoption: The case of improved rice varieties (Nerica) in the Northern Region, Ghana. Journal of Economics and Sustainable Development, 8(8), 1700–2222. www.iiste.org
- Bonabana-Wabbi, J., Hezron, M., Onesmus, S., Julian, K., & Basil, M. (2016). Adoption of integrated soil fertility management by groundnut farmers in Eastern Uganda. Journal of Development and Agricultural Economics, 8(4), 86–94. https://doi.org/10.5897/JDAE2014-0627
- Chune, M. D., Beatrice, A., Obrine, A. B., & Kwesiga, M. (2022). Measuring technical efficiency and its determinants among sweet potatoes farmers in Western Uganda. African Journal of Agricultural Research, 18(2), 117–126. https://doi.org/10.5897/AJAR2021.15878
- Debebe, S. (2022). Post ‑ harvest losses of crops and its determinants in Ethiopia : Tobit model analysis. Agriculture & Food Security, 1–8. https://doi.org/10.1186/s40066-022-00357-6
- Dzanku, F. M., & Osei, R. D. (2018). Impact of pre – And post-harvest training reminders on crop losses and food poverty in Mali. 30th International Conference of Agricultural Economists, 0–28.
- Endalew, B., & Yenewa, W. (2021). Determinants of farm households ’ participation in fish production in Southwest Ethiopia. Cogent Food & Agriculture. https://doi.org/10.1080/23311932.2020.1728107
- Eticha, D. (2021). Analysis of stallholder farmers’ participation in agricultural extension services in Yayo and Hurumu Districts of Oromia, South-West Ethiopia. Journal of Economics and Sustainable Development, 12(21), 10–21. https://doi.org/10.7176/jesd/12-21-02
- Fahad, S., & Wang, J. (2018). Farmers’ risk perception, vulnerability, and adaptation to climate change in rural Pakistan. Land Use Policy, 79(April), 301–309. https://doi.org/10.1016/j.landusepol.2018.08.018
- FAO. (2002). The role of agriculture in the development of least-developed countries and their integration into the world economy. Fao, 7. http://www.fao.org/3/a-y3997e.pdf
- FAO. (2018). AGRISurvey programme in Uganda key findings of the annual agricultural survey 2018. Food and Agriculture Organization of the United Nations.
- Greene, W. H., & Hensher, D. A. (2010). Ordered choices and heterogeneity in attribute processing. Journal of Transport Economics and Policy, 44(3), 331–364. https://www.jstor.org/stable/25801404
- Ismail, I. J., & Changalima, I. A. (2019). Postharvest losses in maize : Determinants and effects on profitability of processing agribusiness enterprises in Tanzania department of business administration and management department of business administration and management. East African Journal of Social and Applied Sciences (EAJ-SAS), 1(2), 203–211. https://www.mocu.ac.tz
- Jaza, F. A. J., Tsafack, P. P., & Kamajou, F. (2018). Logit model of analysing the factors affecting the adoption of goat raising activity by farmers in the non-pastoral centre region of Cameroon. Tropicultura, 36(1), 54–62. https://doi.org/10.25518/2295-8010.976
- Jegede, O. O. (2020). Node between firm’s knowledge-intensive activities and their propensity to innovate: Insights from Nigeria’s mining industry. African Journal of Science, Technology, Innovation and Development, 12(7), 873–881. https://doi.org/10.1080/20421338.2020.1746043
- Jose, A., Philip, M., Prasanna, L. T., & Manjula, M. (2020). Comparison of probit and logistic regression models in the analysis of dichotomous outcomes. Current Research in Biostatistics, 10(1), 1–19. https://doi.org/10.3844/amjbsp.2020.1.19
- Kemboi, E., Muendo, K., & Kiprotich, C. (2020). Crop diversification analysis amongst smallholder farmers in Kenya(empirical evidence from Kamariny ward, Elgeyo Marakwet County). Cogent Food and Agriculture, 6(1), 1834669. https://doi.org/10.1080/23311932.2020.1834669
- Kumar, S., & Underhill, S. (2019). Smallholder farmer perceptions of postharvest loss and its determinants in Fijian tomato value chains. Horticulturae, 5(4), 1–14. https://doi.org/10.3390/horticulturae5040074
- Kurdyś-Kujawska, A., Strzelecka, A., & Zawadzka, D. (2021). The impact of crop diversification on the economic efficiency of small farms in Poland. Agriculture (Switzerland), 11(3). https://doi.org/10.3390/agriculture11030250
- MAAIF. (2016). The republic of Uganda, agriculture sector strategic plan 2015/2016-2019/2020. 1–100. http://npa.go.ug/wp-content/uploads/2016/08/ASSP-Final-Draft.pdf
- MAAIF. (2017). The Republic of Uganda Ministry of Agriculture, Animal Industries and Fisheries: Performance Review Report. 150. https://www.agriculture.go.ug/
- MAAIF. (2020). The Republic of Uganda Ministry of Agriculture, Animal Industry and Fisheries,Draft Annual Report. 1– 150(October), 150.
- Manzoor, A., Tanwir, F., Akhtar, S., & Shahbaz, B. (2020). An assessment of knowledge level and training gap of the farmers regarding post-harvest losses of wheat in Punjab, Pakistan. Journal of Pure and Applied Agriculture, 5(1), 2617–8680. http://jpaa.aiou.edu.pk/
- Martey, E., Etwire, P. M., Wiredu, A. N., & Dogbe, W. (2014). Factors influencing willingness to participate in multi-stakeholder platform by smallholder farmers in Northern Ghana: Implication for research and development. Agricultural and Food Economics, 2(1), 1–15. https://doi.org/10.1186/s40100-014-0011-4
- Mbachu, H. I., Nduka, E. C., & Nja, M. E. (2012). Designing a Pseudo R-Squared Goodness-of-Fit Measure in Generalized Linear Models. Journal of Mathematics Research, 4(2). https://doi.org/10.5539/jmr.v4n2p148
- Midamba, D. C. (2022). Determinants of maize production income in Western Uganda. East African Journal of Agriculture and Biotechnology, 5(1), 1–13. https://doi.org/10.37284/eajab.5.1.532
- Midamba, D. C., Obrine, A. B., Kwesiga, M., Beatrice, A., & Kizito, O. (2022). Drivers of access to credit among smallholder farmers in Uganda: Application of binary logistic model. East African Journal of Business and Economics, 5(1), 154–163. https://doi.org/10.37284/eajbe.5.1.622
- Mohamed, K. S., & Temu, A. E. (2008). Access to credit and its effect on the adoption of agricultural technologies: The case of Zanzibar. Savings and Development, 32(SUPPL), 45–89. https://doi.org/10.2307/41410533
- Mthombeni, D. L., Antwi, M. A., & Oduniyi, O. S. (2022). Factors influencing access to agro-processing training for small-scale crop farmers in Gauteng province of South Africa. Agriculture and Food Security, 11(1), 1–7. https://doi.org/10.1186/s40066-022-00370-9
- Mugwe, J., Mugendi, D., Mucheru-Muna, M., Merckx, R., Chianu, J., & Vanlauwe, B. (2009). Determinants of the decision to adopt integrated soil fertility management practices by smallholder farmers in the central highlands of Kenya. Experimental Agriculture, 45(1), 61–75. https://doi.org/10.1017/S0014479708007072
- Mwaura, F. M., & Adong, A. (2016). Determinants of households’ land allocation for crop production in Uganda. Journal of Sustainable Development, 9(5), 229. https://doi.org/10.5539/jsd.v9n5p229
- Nahayo, A., Omondi, M. O., Zhang, X. H., LI, L. Q., PAN, G. X., & Joseph, S. (2017). Factors influencing farmers’ participation in crop intensification program in Rwanda. Journal of Integrative Agriculture, 16(6), 1406–1416. https://doi.org/10.1016/S2095-3119(16)61555-1
- Nakano, Y., Tsusaka, T. W., Aida, T., & Pede, V. O. (2018). Is farmer-to-farmer extension effective? The impact of training on technology adoption and rice farming productivity in Tanzania. World Development, 105, 336–351. https://doi.org/10.1016/j.worlddev.2017.12.013
- Ngeywo, J., Basweti, E., & Shitandi, A. (2015). Influence of Gender, Age, Marital Status and Farm Size on Coffee Production: A Case of Kisii County, Kenya. Asian Journal of Agricultural Extension, Economics & Sociology, 5(3), 117–125. https://doi.org/10.9734/ajaees/2015/15702
- Njenga, M. W., Mugwe, J. N., Mogaka, H., Nyabuga, G., Kiboi, M., Ngetich, F., Mucheru-Muna, M., Sijali, I., & Mugendi, D. (2021). Communication factors influencing adoption of soil and water conservation technologies in the dry zones of Tharaka-Nithi County, Kenya. Heliyon, 7(10), e08236. https://doi.org/10.1016/j.heliyon.2021.e08236
- Odendo, M., Obare, G., & Salasya, B. (2009). Factors responsible for differences in uptake of integrated soil fertility management practices amongst smallholders in western Kenya. African Journal of Agricultural Research, 4(11), 1303–1311. https://www.academicjournals.org/AJAR
- Ojo, C. O., Bila, Y., & Iheanacho, A. C. (2012). Agricultural resource access and the influence of socioeconomic characteristics among rural women in borno state, Nigeria. Agris On-Line Papers in Economics and Informatics, 4(2), 27–34. https://doi.org/10.22004/ag.econ.131360
- Okeyo, S. O., Ndirangu, S. N., Isaboke, H. N., Njeru, L. K., & Omenda, J. A. (2020). Analysis of the determinants of farmer participation in sorghum farming among small-scale farmers in Siaya County, Kenya. Scientific African, 10. https://doi.org/10.1016/j.sciaf.2020.e00559
- Ouko, K. O., Robert, O. G., Alworah, G. O., Zephaniah, M., & Sharon, V. O. (2020). Effects of covid-19 pandemic on food security and household livelihoods in Kenya. Review of Agricultural and Applied Economics, 23(2), 72–80. https://doi.org/10.22004/ag.econ.308403
- Pratiwi, A., & Suzuki, A. (2017). Effects of farmers’ social networks on knowledge acquisition: Lessons from agricultural training in rural Indonesia. Journal of Economic Structures, 6(1). https://doi.org/10.1186/s40008-017-0069-8
- Saqib, S. E., Kuwornu, J. K. M., Panezia, S., & Ali, U. (2018). Factors determining subsistence farmers’ access to agricultural credit in flood-prone areas of Pakistan. Kasetsart Journal of Social Sciences, 39(2), 262–268. https://doi.org/10.1016/j.kjss.2017.06.001
- Shee, A., Mayanja, S., Simba, E., Stathers, T., Bechoff, A., & Bennett, B. (2019). Determinants of postharvest losses along smallholder producers maize and Sweet potato value chains: An ordered Probit analysis. Food Security, 11(5), 1101–1120. https://doi.org/10.1007/s12571-019-00949-4
- Shively, G., & Hao, J. (2012). A review of agriculture, food security and human nutrition issues in Uganda. University Department of Agricultural …, September, 1–42. http://ageconsearch.umn.edu/bitstream/135134/2/12-3Shively.Hao.pdf
- Sugri, I., Abubakari, M., Owusu, R. K., & Bidzakin, J. K. (2021). Postharvest losses and mitigating technologies: Evidence from Upper East Region of Ghana. Sustainable Futures, 3(December 2020), 100048. https://doi.org/10.1016/j.sftr.2021.100048
- Tolno, E., Kobayashi, H., Ichizen, M., Esham, M., & Balde, B. S. (2015). Economic analysis of the role of farmer organizations in enhancing smallholder potato farmers’ income in Middle Guinea. Journal of Agricultural Science, 7(3), 123–137. https://doi.org/10.5539/jas.v7n3p123
- UBOS. (2017). Area specific profiles Nwoya District. The National Population and Housing Census 2014
- UBOS. (2018a). Annual agricultural survey 2018 statistical release (p. 4).
- UBOS. (2018b). Uganda bureau of statistics. The 2018 statistical report. 345.
- Vu, H., Van, Ho, H., & Le, Q. H. (2020). Impact of farmers’ associations on household income: Evidence from tea farms in Vietnam. Economies, 8(4), 92. https://doi.org/10.3390/economies8040092
- Weber, N. C. (2020). Field and post-harvest factors affecting the quality and shelf life of soft fruits. Modern Concepts & Developments in Agronomy, 6(2), 631–634. https://doi.org/10.31031/mcda.2020.06.000634
- Yahaya, S., & Mardiyya, A. (2019). Review of post-harvest losses of fruits and vegetables. Biomedical Journal of Scientific & Technical Research, 13(4), 10192–10200. https://doi.org/10.26717/bjstr.2019.13.002448
- Yang, Q., Zhu, Y., & Wang, F. (2021). Exploring mediating factors between agricultural training and farmers ’ adoption of drip fertigation system : Evidence from banana farmers in China. Water (Switzerland), 1–18. https://doi.org/10.3390/w13101364
- Yeshiwas, Y., & Tadele, E. (2021). An and their handling investigation into major causes for postharvest losses of horticultural crops practice in Debre Markos, North-Western Ethiopia. Advances in Agriculture, 2021. https://doi.org/10.1155/2021/1985303
- Zangeneh, M., Akram, A., Nielsen, P., & Keyhani, A. (2015). Developing location indicators for agricultural service center: A Delphi–TOPSIS–FAHP approach. Production and Manufacturing Research, 3(1), 124–148. https://doi.org/10.1080/21693277.2015.1013582