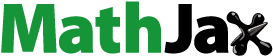
Abstract
Nowadays, poverty is one of the most important issues that needs due attention in many developing countries like Ethiopia. Nonetheless, poverty in Ethiopia remains widespread in both rural and urban areas. Therefore, this study aimed to examine the determinants and status of poverty in Berehet Woreda. The study was conducted using a cross-sectional survey. To conduct the study, a sample of 384 households was selected using a stratified simple random sampling technique. Foster Greer Thorbecke’s Poverty Index was used to examine the extent and severity of poverty in the Woreda. Accordingly, about 36% of households in Woreda live below the poverty line, with an average poverty gap of 12% and poverty severity of about 7%. The binary logit model showed that household education status, dependency ratio, residential area, and access to credit were statistically significant in determining household poverty status. Since the poverty situation in Woreda was worse than the national average, the regional government should prioritize this Woreda and develop a special type of projects especially in rural areas that can lift the majority of the poor out of poverty.
JEL classification:
PUBLIC INTEREST STATEMENT
Poverty is a major problem across the world and reducing poverty is not simple task. Some advocates of antipoverty programs claim that fighting poverty is a public good. This might be provided in the form of rigors research conducted by researcher on the level of poverty, sources of poverty and on the determinant of poverty. This would also leads to government intervention through designed policy are important. Societies also need a reduced poverty level accompanied by a number of constructive social impacts. Therefore, the research conducted on poverty would help the government or policy makers to meet the interest of the people by using recommended policy options.
1. Introduction
The concept of poverty refers to individuals or families who do not have enough resources to meet their current needs. The poor are vulnerable groups who lack access to adequate food, shelter, education, health and other services. However, the task of reducing poverty is quite challenging, as poverty means not only low levels of income/consumption and low levels of human development in terms of education and health care, but also feelings of powerlessness, vulnerability and fear because the poor are at greater risk of living on the brink of subsistence. Therefore, different policies and poverty eradication strategies should be interwoven to eradicate extreme hunger and poverty as propagated in the Sustainable Development Goals (SDGs) under Goal 1. Poverty can have many consequences, for instance, (Neville, Citation2011; Ratcliffe & Mckernan, Citation2010) pointed out that children growing up in poverty have much worse mental and cognitive development than children growing up in non-poor areas, and this is repeated in the same group. In extreme situations, poverty has always been seen as a curse on humanity (Kotler et al., Citation2006), particularly in less developed countries such as Ethiopia.
As in every African country, poverty is widespread in Ethiopia, which is why poverty reduction becomes one of the main goals of the development efforts of all underdeveloped countries including Ethiopia (Bigsten et al., Citation2003), which in turn helps to maintain and promote social cohesion (Sharma & D, Citation2014; Teka et al., Citation2019). For example, in Ethiopia, where most people live below the poverty line, poverty is pervasive and persistent (Alemu et al., Citation2011). Along with severe poverty, Ethiopia is also threatened by high population growth associated with high unemployment rates, high dependency ratios and larger family sizes (Deressa & Sharma, Citation2014).
As for Ethiopia’s poverty status, in 2000 45.5% of the population lived below the national poverty line, which fell to 30% in 2011. The same report also notes that the poverty rate fell from 45.5% in 2000 to 23.5% in 2016 (UNDP, Citation2018). In the same report, the poverty status based on the area of residence using the national poverty line in the year 2000 was 39.9% in urban and 45.4% in rural areas. Furthermore, the poverty status of the Amhara regional state where the study area is found was 41.8% in 2011 and 30.5% in 2014 (World Bank Group, Citation2015). Even if poverty gap and poverty severity indices in the year 2000 were declined from 10.1% and 3.9% to 3.7% and 1.4% in 2016 respectively (UNDP, Citation2018), the level of poverty remains widespread in Ethiopia, both in rural and urban areas. However, the rate at which poverty decline in urban areas is not the same as that of rural areas (Enquobahrie, Citation2004). In this regard, in urban areas poverty has declined from 26% in 2011 to 15% in 2016. Conversely, progress in rural areas has been more modest, with poverty falling from 30% to 26% over the same period (UNDP, Citation2018).
Despite some improvements in recent years, poverty, along with other issues, is still becoming the most serious problem which affects the life of million in Ethiopia. For instance, a study conducted by (Hartline Grafton & Dean, Citation2017) points to a direct link between food insecurity and poverty, in which food-insecure households are poor. Additionally, (Teka et al., Citation2019) found a close link between poverty and income inequality in pastoral and agro-pastoral communities of Afar Regional state, Ethiopia. Berehet Woreda, where the study was conducted, is one of the most food-insecure woreda in the northern Shewa Zone of Amhara Regional State which share a boarder with Afar Regional state. In addition, the poverty status in the study area is expected to be above the national and regional averages. Therefore, the incidence of poverty varies by social group, season, location, and region. In this sense, most studies of poverty have focused on poverty at the national or regional level (Bogale et al., Citation2005; Deressa & Sharma, Citation2014; Ermiyas et al., Citation2013). However, as far as the problem of poverty and its determinants is concerned, it is highly crucial to study the matter along specific area case in order to arrive at concrete results or solution. This is because the existing situations in one area may not be compatible with those in the other. For this reason, some of the policy implications that are proposed by pooling a group of people at national or regional level that are structurally diverse may not be effective in addressing the issue of poverty. Moreover, by its very nature, poverty is multifaceted, complex and pervasive. In this regard, poverty in the study area was further complicated by poor access to infrastructure, lack of government support, limited access to employment opportunities, and poor living conditions. Therefore, the main objective of the study is to determine the poverty status in Berehet Woreda, investigate rural–urban difference of poverty status and identify the determinant of poverty in the study area.
Moreover, poverty alleviation has been an important policy debate in the international development literature in recent years and comprehensive development occurs when people have political, social, and economic power (Muhdin, Citation2015). However, as (Kotler et al., Citation2006) explained, the poor have often been viewed as a homogeneous mass to be addressed with standardized aid programs at the national level, which inevitably leads to discrepancies between local needs and solutions. For this reason the contribution of our study comes in two pervasive ways. First, most of the studies conducted on poverty at national and regional levels may not reflect the actual status of poverty in all parts of the country and fail to account poverty at grass root level. Second, to the best of the researcher’s knowledge, no study has been conducted on poverty in Berehet Woreda; therefore, our study adds to the available literature and sheds light for further research. As a result, potential stakeholders such as the government and organizations working on poverty alleviation will clearly understand and make evidence-based decisions.
2. Theoretical literature review
Classical economists believed that poverty is highly associated with individual characteristics and a behavioral problem passes from generation to generation as a culture because of genetic factors, and they thought that the contribution of the government to combat poverty is minimal. On the other hand, the neo-classical theory assumed poverty results from unequal endowments in talents, skills, and capital. This theory considers poverty is a due to lack of capital in different forms, including human, physical, social, and institutional. Therefore, the empirical analysis of the determinant of poverty was conducted based on individual factors, institutional factors, and socio-economic factors that determine the likelihood of the household to be poor or non-poor (Davis & Sanchez-martinez, Citation2014).
2.1. Empirical literature review on determinants of poverty
2.1.1. Household specific factors
Different literature indicated that household head age (Beyene & Muche, Citation2010; Muleta & Deressa, Citation2014; Tesfaye & Getachew, Citation2018) has a positive and significant effect, that means as the age of the household increase, it increases the probability of being poor, which implies that older households are less likely to participate in productive activities. According to the life cycle theory of income, poverty will be higher for households headed by young and by old people. This is because productivity is low at a relatively young age, increases at middle age and then decreases again at old age. Contradictory to the above research finding, the research conducted by (Muhammedhussen, Citation2016) indicated that the age of the household has a negative and significant effect on reducing poverty.
The sex of the household is another substantial determinant of poverty and has a negative impact on reducing poverty in the male-headed household (Bekele & Silshi Merid, Citation2020). This is because most of the time, females were engaged and occupied by non-productive activities, and they were deprived of vital and productive resources like land and other economic resources (Neway et al., Citation2022). Research conducted on determinants of rural poverty in Ethiopia the case of Dejen Woreda and Hong Kong proved that male headed households have low probability of being poor (Ermiyas et al., Citation2019; Peng et al., Citation2019).
Furthermore, the study results on the determinant of poverty indicate that dependency ratio have a positive and significant effect in aggravating poverty (Ermiyas et al., Citation2019; Girma & Temesgen, Citation2018; Kassahun et al., Citation2022; Muleta & Deressa, Citation2014; Sinnathurai, Citation2013; Tesfaye & Getachew, Citation2018). Bringing a more unproductive household member into the family could exacerbate poverty as the household would struggle to meet food and other needs due to limited income. Therefore, households with more non-working members earning less income are at risk of falling into poverty (Eyasu & Yildiz, Citation2020).
The other important household-specific variable that can influence household poverty status is the educational status of the household head. Education is considered as the most important determinant of household’s poverty in many studies (Awel & Brar, Citation2019; Bogale et al., Citation2005; Kassahun et al., Citation2022; Muleta & Deressa, Citation2014). Given that the main asset of the poor is their labour, and since the returns to labour are highly correlated with education, Garza–Rodríguez (Citation2015) found an inverse relationship between education and poverty. In addition, (Eyasu & Yildiz, Citation2020) also found a positive correlation between education and poverty in both urban and rural areas. This means that improvement in education status increases earning potential and improve the occupational and geographic mobility of labour. The study from Hong Kong on determinant of poverty affirmed that household who have lower educational attainment have high probability of being poor (Peng et al., Citation2019).
2.1.2. Socio-economic factors
The livestock sector also makes an important contribution to the economy and environmental protection: it restores income and other sources of crop production, absorbs income shocks caused by crop failures, generates a continuous income stream and employment opportunities, and reduces the seasonality of income, especially among the rural. Tropical Livestock unit as a measure of livestock owned by households found to have a negative and statistically significant association with poverty status of households in a number of studies (Alemaw et al., Citation2021; Awel & Brar, Citation2019; Girma & Temesgen, Citation2018; Kassahun et al., Citation2022; Muhammedhussen, Citation2016; Tesfaye & Getachew, Citation2018). Livestock owned as an essential asset of the household, can help households by bridging income and sometimes food gap by absorbs income shocks caused by crop failures, generates a continuous income stream and employment opportunities and it is also sources of wealth (Alemaw et al., Citation2021; Beyene & Muche, Citation2010; Ermiyas et al., Citation2019; Rehman et al., Citation2017).
The religion of the head of household is also a major factor in poverty (Mberu et al., Citation2014). The Kenya study on patterns and determinants of poverty transitions in urban poor households showed that Muslims are less likely to escape poverty than Christians. The other study conducted in America (Ranjith & Rupasingha, Citation2012) showed the same result, and which indicating that Muslim households have a large family size, and this large family size contributed for poverty.
The marital status of the household is another determinant of poverty. Married households has a better probability than none married one to get out of poverty (Heshmati et al., Citation2019). The phenomenon that married men earns higher average wages as compared to unmarried men, the so-called marriage premium.
2.1.3. Institutional factors
Access to credit has been considered as one of the important variables of interest in poverty reduction. Access to credit services (Tesfaye & Getachew, Citation2018) has a negative and significant effect in reducing the probability of being poor. Access to credit services is the main source of financial capital that sustains rural livelihood. Therefore, enhancing and expanding rural credit services are important ingredient for farmers to fulfill their demand for modern farm inputs and enhance technology adoption. These directly increase the income of the household and help to escape out of poverty trap.
2.1.4. Spatial factors
Residential area (urban-rural) differential is one of the determinants of poverty. Rural residents are relatively worse off socio-economically than urban residents (Mberu et al., Citation2014). On the other hand, urban residents have higher consumption expenditure (income) than rural residents (Heshmati et al., Citation2019). Poverty alleviation also has been better achieved along with urban residents due to access to better education, infrastructure, and job opportunity result in a higher income than rural residents.
2.2. Measurement and decomposition of poverty
The Foster-Greer-Thorbecke (FGT) index is a generalized poverty measure developed by Erik Thorbecke, Joel Greer, and James Foster (Foster et al., Citation1984). They also identify three categories of FGT contributions in poverty analysis to the measurement, to the axiomatic, and to the application. It also contributed to the design, implementation, and evaluation of prominent development programs (Foster et al., Citation2010). Even though there are competing measurements of poverty, researcher commonly used Foster Greer and Thorbecke poverty measure that has been found suitable for presenting information on poverty in an operationally convenient manner as compared to other unidimensional poverty measure such as Watts poverty index, Sen-Shorrocks-Thon index and Time taken to exit. On the other, Foster Greer and Thorbecke (FGT) poverty measure have desirable characteristics which are understandable and straightforward for policymakers (Foster & Greer Thorbecke, Citation2010). The FGT index has proven to be very useful for evaluating the extent of poverty across space and time in many studies. In this regard, many empirical studies focusing on the issue of poverty were bound to use FGT index since the measure is suitable to analyze inequality (average squared normalized poverty gap using P2 squared coefficient of variation, renormalization of income gap (average poverty normalized gap) using P1 and headcount ratio using P0 (Foster et al., Citation1984). Most of the applications use the decomposability property of FGT measure to analyze the significant correlates of the incidence or headcount, depth, and severity of poverty and laid the ground for informed policy discussion to confront poverty (Foster et al., Citation2010). The FGT index as poverty measure is formulated as;
Where
P is a measure of absolute poverty
is the FGT parameter which may be interpreted as a measure of poverty α = 0,1,2 headcount, poverty gap and severity, respectively
Y is total consumption expenditure per adult equivalent (i = 1,2, …,n)
n is the total number of households in the sample
q is the total number of poor households below the poverty line
3. Research methods
3.1. Sampling
A two-stage stratified sampling method was used to select the sample respondents. In the first stage, one rural kebele from the nine Kebeles of the Woreda were randomly selected because the population in the Woreda is homogenous regarding religion, geographic location, and mode of living and one urban kebele it was selected. In the second stage, a total of 384 representative samples were selected from the two strata by using proportional random sampling i.e. 154 rural kebele and 250 from urban kebele.
3.2. Data type and collection method
For this study, the following key data was extracted from primary sources for the period of 2017/18. In order to generate the information required for this study, a structured questionnaire was developed. The questionnaires were used as a significant source of information to collect data on different aspects of the household and individual characteristics related to determinants of household poverty.
3.3. Methods of data analysis
3.3.1. The setting of poverty line
Poverty comparisons involve three main decisions: choosing a welfare measure; choice of a poverty line, and choosing a poverty index for aggregation (Appleton et al., Citation1999). Regarding the choice of welfare measure, the study chose consumption rather than income because consumption would be a better indicator of poverty measurement than income. Even if there is a great deal of literature on how to assess poverty the question remains unanswered to draw the line of poverty (Thorbecke, Citation2004). Currently, there are two main methods to set the poverty line: Cost of basic Needs Value (CBN) and Food-Energy-Intake (FEI). The cost of basic need approach is important for ensuring consistency or treating individuals of the same standard of living equally (World Bank Organization, Citation2001). The poverty line is also estimated by using the cost of basic need approach both food and non-food. In poverty analysis, there is no fixed poverty line instead we used the food poverty line of meeting 2300 kcal per person per day. W because an individual who has the same income shortfall does not’ mean that they have the same calorie shortfall. Any normal person needs a minimum energy requirement to perform regular physical life, estimated by Ethiopia nutrition and health research institute was used for this study. The study adopts the cost of basic need approach, which is the widely used method of setting the poverty line. The Monetary poverty line is constructed by using the cost of basic need approach, including the cost of basic food and non-food needs. A household is deemed as living in poverty if the daily per capita household food energy intake falls below 2,300 kcal and non-poor if the daily per capita household food energy intake falls above 2,300 kcal. This is done through estimation of the cost of bundle of good the average household consumed that give 2300 a daily kilo calorie to perform a good physical function. The consumption data from the household was collected to reflect the general pattern of food consumption to estimate the quantities of food items consumed by the average households and converted to monetary value in order to set the poverty line.
Where
Si = food share to total expenditure
Yi = total expenditure
Zi = food poverty line
For households whose total expenditure is approximately equal to the food poverty line (Yi = Zi), the food share is α, and consequently, the non-food share of expenditure is (1—α). Thus, the poverty line is
Where
Zf food poverty line
Znf non-food poverty line
All the above mathematical expressions would help identify the households as poor and non-poor.
3.3.2. The logistic regression model
In order to capture the determinants of poverty, a logistic regression model is employed. This model is selected for the following reasons. First, it is easy to manipulate and simple to comprehend. Second, the dependent variable, the probability of being poor and non-poor, is dichotomous. Given the dependent variable of main interest that a household may be classified as poor or non-poor, a binary logit model could be used for the analysis of the data. Based on the cost of basic need approach, the food poverty line and non-food expenditure is estimated 3961 and 4476 respectively Consider that a household is poor (Y = 1) if per capita household food and non-food expenditure is less than or equal 8437 Birr per year or non-poor (Y = 0) if the per capita household food and non-food expenditure is greater than 8437 Birr.
Where Is the underlying latent variable that index the agricultural technology adoption
is the stochastic error term, and β is a column vector of parameters that has to be estimated.
OR
If we let Xik be the kth element of the vector independent variable Xi, and βk be the kth element of β, then the marginal effect of a particular independent variable, Xi, on the probability of the occurrence of the response is given by (Maddala, Citation1983):
Where,
Yi = Probability of being adopter in relation with the explanatory variables
Ai = A function of n explanatory variables
βs = Unknown Parameters
= Error/Stochastic term
i = Individuals/Respondents in the study in which i =1, 2, 3, …, n =384
3.3.3. Variable measurement and specification
Concerning the determinants of poverty status of the household after reviewing different theories and empirical evidence, variables are identified based on household-specific factors (sex of the household head, age of the household head, education status of the household head, and dependency ratio), socio-economic factors (marital status of the household head, religion of the household and livestock owned by the household) institutional factors (access to credit) and Spatial factors (residential area). The list of variables, measurements and expected relationship with household poverty is also determined based on the literature review, and it is presented as follows in Table .
Table 1. Summary of variable specification
4. Result and Discussion
4.1. Descriptive analysis
Table shows that 281 (73.18%) of the respondents were male headed household of which 138 (35.94) are poor and 143 (37.24%) are non-poor and 103 (26.82%) of the respondents were female headed households of which 32 (8.33%) are poor and the remaining 71 (18.49%) are non-poor. In analyzing the association between sex of the household head with poverty status a Pearson chi2 test was undertaken to check whether there exists a significant association or not. The test result shows that as there is a statistically significant association between sex of the household head and poverty status at 1% level of significance since p-value = 0.002. On the basis of the test result majority of the female headed households are poor as compared to their counterpart. This might be explained by the fact that male-headed households have alternative income sources and resource entitlements than female-headed households.
Table 2. Descriptive statistics on categorical variables
Regarding area of residence of the household head Table infers, 230 (59.90%) of the household heads are urban dwellers of which 67 (17.45%) of them are poor and the remaining 163 (42.45%) of them are non-poor. On the other hand, rural dwelling households accounts 154 (40.10%) of the respondents of which 103 (26.82%) are poor and the remaining 51 (13.28%) of them are non-poor. The chi2 test undertaken to check the association between residential area of the household head and poverty status asserted as there is a statistically significant association between them at 1% level of significance since p-value = 0.000. The summary statistics showed that majority of the urban dwelling households fall under poor poverty status. This might be due to fact that urban dwelling households shoulder much economic burden than rural dwelling households since cost of living in urban areas is pretty much higher than rural areas. In addition, unlike urban dwelling households, rural dwelling households mostly engage in productive activity which can support them at least to cover some portion of their food expenditure.
Concerning marital status of the household head as presented in Table , almost one third i.e. 127 (33.07%) of the married respondents are poor and the remaining 129 (33.59%) of married respondents and 85 (22.14%) of the non-married sampled households are non-poor. The test result also indicates the existence of significant difference between households with married and non-married marital status in terms of their poverty status at 1% level of significance since p-value = 0.003. Here, households in which their marital status is married are more prone to poverty as compared to the non-married one since the married one faces greater level of dependency burden.
The other variable capable of influencing poverty is education, particularly when poverty is defined in terms of deprivation in human capabilities such as knowledge, longevity and living standards. This means that literate household heads are less likely to be poor than are illiterate households. Having a scholarly household head modestly reduces the likelihood of a family he being poor since education might increase earning potential and improve the occupational and geographic mobility of labor. In this regard, Table , entails that as we move from illiterate to >12 grade education status the proportion of poor households decreases. The test result also signifies the existence of a statistically significant association between education status and poverty status at 1% level of significance since p-value = 0.000.
Access to credit is the other important variable associated with poverty status. Ease of access to credit helps poor households develop to improve their welfare and be able to get out of poverty. Financial institutions that are targeting the poor in providing credit should create a suitable environment for the poor. Having easy access to credit can motivate households’ productivity to develop and engage in new businesses and strengthen the already existing one. Accordingly, among 267 (69.53%) of the households who do not have access to credit 105 (27.34%) of them are poor and the remaining 162 (42.19%) are non-poor. On the other hand among households having access to credit 65 (16.93%) and 52 (13.54%) of the sampled respondents fall under poor and non-poor poverty status.
According to Table , the average age for the sampled household heads is almost 38 years. The statistical test showed that there is a statistically significant difference among households with poor and non-poor poverty status in terms of age of the household head at 1% level of significance since p-value = 0.0000. This signifies age of the samples household head can lead to difference in poverty status. On the other hand, the average household size is 5.12 for poor households and 2.89 for non-poor households. Like the age of the household head household size also signifies the existence of a statistically significant difference among poor and non-poor households in terms of family size at 1% level of significance since p-value = 0.0000. Total expenditure on food and non-food items is another factor that is assumed to have a greater effect on poverty status and it showed that on average the sampled households spend birr 21,867.17 per annum on average. The mean comparison test showed that there is a significant difference between poor and non-poor households in terms of total expenditure at 1% level of significance since p-value = 0.0000. In relation to the total expenditure, per capital expenditure of the sampled households also entails the existence of significance difference among poor and non-poor households at 1% level of significance since p-value = 0.0000. This may be due to the existence of significant difference among poor and non-poor household’s in terms of family size. The number of livestock owned measured using tropical livestock unit (TLU), have also statistical difference among poor and non-poor households. In this regard, non-poor households have larger livestock ownership (5.55 TLU) than poor households (2.20 TLU). Dependency ratio which is measured as a ration of the number of economically active family members on active one found to be 1.47 on average. On average poor households faces relatively higher dependency ratio (2.34) as compared to non-poor households (0.79) and the difference is significant 1% level of significance since p-value = 0.0000. This indicates as dependency ratio increases the likelihood of being poor also increases.
Table 3. Descriptive statistics on continuous variables
4.2. Poverty decomposition
Estimating the poverty index was the first step in the study of poverty. So, we try to examine the level poverty in the study area by estimating the three most common Foster-Greer-Thorbecke poverty indexes. Namely headcount ratio (proportion of the poor) FGT (0) 44.27% of the people are poor, FGT(1) average normalized poverty gap 12.24%, and FGT(2) average squared normalized poverty gap 4.6%. Based on subgroup or area of residence analysis of FGT index estimates in a rural area is FGT (0) headcount ratio (proportion poor) 66.88%, FGT (1) average normalized poverty gap 21.91%, and FGT (2) average squared normalized poverty gap 8.71 while the subgroup FGT index estimates of an urban area are FGT (0) headcount ratio (proportion poor) 29.13% FGT (1) average normalized poverty gap 5.77% and FGT (2) average squared normalized poverty gap 1.81%.
FGT (0): headcount ratio (proportion poor)FGT (1): average normalized poverty gapFGT (2): average squared normalized poverty gap
To investigate rural-urban difference in terms of poverty status in the Woreda, we observe a significance difference in the level of poverty based on the headcount ratio. Out of the total population who live in rural areas, 66.88% are poor, while only 29.13% of the sampled respondents living in urban areas are poor. The poverty gap in the study area is about 5.77% in urban areas and 21.91% in rural areas, and the figure clearly showed that the poverty gap in rural areas is higher than in urban areas. Regarding the severity level, it is higher in rural areas than urban areas as we see, in Table . From the literature review, it was proved that the rate at which poverty declined in urban areas was not the same as that of rural areas, and we found the same result in this study that the level of poverty in rural areas is by far greater than urban areas.
Table 4. Foster-Greer-Thorbecke poverty indices, FGT (a)
4.3. Econometric analysis
In identifying factors that determine households’ poverty status, a set of 11 explanatory variables are included in the binary logit regression analysis is preesented in Table below. These variables are selected based on theoretical explanations and the results of various empirical studies. From the logit model, household head level of education, dependency ratio; livestock ownership and area of residence are statically significant at 1% significant level. While education at primary level is statistically significant at 5% significant level and access to credit is statistically significant at 10% of significant level.
Table 5. Result from the logit model
(*) dy/dx is for discrete change of dummy variable from 0 to 1
The variable dependency ratio is a demographic measure of the ratio of the number of dependents to the total working-age in the household. It was found that positive and statistically significant at 1% level of significance, implying that households with a high dependency ratio are poorer than households with lower dependency. The marginal effect indicates that as the dependency ratio increases by one, the probability of households falling into poverty increases by 16%, ceteris paribus. This is obvious because dependents, due to age and physical problems, contribute less to family labour and income. In addition, it also increases the consumption of the household and requires additional money for their livelihood reducing the freedom to use the money for other purposes. A high dependency ratio indicated that the economically active population and the overall economy face a greater burden to support and provide the social services needed by children and by older persons who are often economically dependent. Therefore, household with high dependency ratio has the chance of falling in poverty, Similar finding is obtained by (Ermiyas et al., Citation2019; Girma & Temesgen, Citation2018; Kassahun et al., Citation2022; Muleta & Deressa, Citation2014; Sinnathurai, Citation2013; Tesfaye & Getachew, Citation2018).
Another critical factor influencing the household poverty is education status of the household head. Household education status, which is a categorical variable, it has a negative and statistically significant impact on the extent of household poverty at the 1% level of significance. Both formal and informal education has a negative effect on poverty; the marginal effect implies for those households with primary level of education the probability of being poor decreases with 14.8% as compared to the illiterate households. On the other hand, for households with secondary school education status, the probability of being poor decreased by 24.7% as compared to illiterate households. For households with tertiary education level also the probability of being poor decreases by 29.6% as compared to household heads who are illiterate. This implies as the household moving to the higher education ladder he/she has the chance to be employed on the formal sectors and earn a higher income, resulting in improved living conditions and low probability of falling into poverty. It is also an important determinant of poverty because it influences households decision in a different way, such as the adoption of innovative technology and facilitates entry into highly profitable farm and non-farm activities (Hagos et al., Citation2012). Education has been declared a primary weapon to prevent the spread of poverty (Awan et al., Citation2011). The findings of this study is consistent with (Awel & Brar, Citation2019; Bogale et al., Citation2005; Ijaiya, Marikan et al., Citation2018; Kassahun et al., Citation2022). Therefore, education is the basis for poverty eradication through economic growth and increased income (Liu et al., Citation2021).
Livestock ownership as a proxy for measuring household asset possession measured using TLU (tropical livestock unit) which have negative and statistically significant effect on household level of poverty at 1% level of significance. The marginal effect shows that tropical livestock unit of the household increases by one unit, the probability of the household getting out of poverty increased or the probability of being poor would be reduced by 12.8%. Livestock is a prominent source of wealth to farm households (Beyene & Muche, Citation2010), a household with large livestock size is expected to be less vulnerable to poverty. Specially in times of drought when crops fail the likelihood of the household getting cash through sale of livestock become high (Bogale & Shimelis, Citation2009; Muhdin, Citation2015). Livestock provides organic fertilizer to the crop sector it restores income and other sources of crop production, absorbs income shocks caused by crop failures, generates a continuous income stream and employment opportunities, and reduces the seasonality of income, especially among the rural poor households (Rehman et al., Citation2017). The research finding also consistent with (Alemaw et al., Citation2021; Awel & Brar, Citation2019; Girma & Temesgen, Citation2018; Kassahun et al., Citation2022; Muhdin, Citation2015; Tesfaye & Getachew, Citation2018).
Access to credit has a positive and statistically significant effect on the household poverty status at the 5% level of significance. It is believed that access to credit services is the primary source of financial capital to sustain a rural livelihood, and it is important in urban areas to reduce unemployment and poverty. Rural credit services can help farm households solve credit constraints faced to buy farm oxen, modern farm inputs, and enhancement of technology adoption such as water pump (Hagos et al., Citation2012). However, in the study area, which found that credit access increases the probability of being poor. The very reason might be due to poor management of credit management among households having access to credit specially using credit for unproductive activities. The 8marginal effect indicated that for those household who have got access to credit the probability being poor increases by 15.41% as compared to households who do not have access to credit. The result indicate that making credit available is not the only solution to reduce poverty rather creating awareness about credit management for households who have access to credit should be part of the credit service process. In addition to the above reason, research findings indicated that in the short-term, preferential loans do not have a substantial impact in improving the income of borrowing households compared to non-borrowing ones. Income and poverty have a strong relationship that means any variable that has a negative effect on income have a positive effect on poverty or increases poverty (Linh et al., Citation2019; Tesfaye & Getachew, Citation2018).
The residential area of the household is statistically significant in influencing the household’s poverty status at 1% level of significance. The marginal effect infers that for households who are living in rural area the probability of being poor increased by 34.3% as compared to urban dwelling households. The previous discussion on poverty decomposition entails that both urban and rural dwellers experience poverty with different intensity. In this regard, rural poverty is very high in the study area and the finding of this study is consistent with (Heshmati et al., Citation2019; Macours & Swinnen, Citation2008; Mberu et al., Citation2014) that show in Ethiopia rural poverty is generally higher than urban poverty, and rural and urban poverty differ strongly across country. The possible reason as to why rural poverty is higher than urban could be access to market, access to infrastructure, access to education and access to health facilities, an important factor that makes urban poverty lower than rural poverty (Gibson & Rozelle, Citation2005).
5. Conclusion and recommendation
The first objective of the study is to look into the rural–urban disparity in poverty status. In the study area, rural poverty is pervasive and persistent, with the majority of sample households desperately poor and living below the poverty line. The level of poverty in the study area exceeds both the regional and national poverty levels. Even within the same Woreda, rural poverty is worse than urban poverty. In terms of headcount ratio, rural poverty is more than double that of urban poverty. At the same time, the poverty gap and severity in rural areas are nearly quadrupled compared to urban areas.
The second objective of this study is to identify the factors that contribute to poverty in the study area. The Binary logistic model was used to identify the primary cause of poverty in Berehet woreda. The results show that the household’s primary, secondary, and tertiary education status, credit access, livestock ownership, dependency ratio, and residential area all have a significant impact on the household’s poverty status. As a result, the aforementioned variable is regarded as a major predictor of poverty in the Woreda. Furthermore, of all significant variables, access to credit and dependency ratio have the greatest influence on the likelihood of a household falling into poverty.
As a result, the regional government should prioritize this Woreda and design a special type of project that can help alleviate poverty, such as irrigation (irrigation technologies such as micro dams, deep wells, river diversions, and ponds have significant poverty-reducing effects that can help to alleviate poverty, particularly in rural areas). In urban areas, the government should focus on increasing productive employment, which can help the poor increase their income through social protection programs such as an urban safety net.
Disclosure statement
No potential conflict of interest was reported by the authors.
Data availability statement
The data supporting this study’s findings are available from the corresponding author upon reasonable request.
Additional information
Funding
Notes on contributors

Markew Mengiste Neway
Markew Mengiste Neway joined Berehet woreda Administrative office and working as the head of the office up to vice administrator of the woreda from 2006 up to 2015 after he holds his bachelor degree in Economics from College of business and economics Bahir Dar University, Ethiopia. After 9-year work experience, he got an in-country scholarship in Bahir Dar University. Now, he holds his master degree in Applied Development Economics on June 2017. After the accomplishment of Master degree he was engaged in teaching, research, and community service in Debre Markos University. Currently he is working in Debre Berhan University engaged in teaching, research, and community service. Specially, measurement and modeling of willingness to pay, food security, value chain analysis, income diversification, livelihood analysis, and poverty analysis are the author’s interest area of research.
References
- Alemaw, A., Kalayu, D., Kahsu, K., & Redae, H. (2021). Poverty status and its determinants in rural households of Enda-mohoni woreda, Northern Ethiopia. Ekonomika Poljoprivrede, 68(3), 729–16. https://doi.org/10.5937/ekopolj2103729t
- Alemu, D., Bewket, W., Zeleke, G., Assefa, Y., & Trutmann, P. (2011). Extent and determinants of household poverty in rural Ethiopia: A study of six villages. Eastern Africa Social Science Research Review, 27(2), https://doi.org/10.1353/eas.2011.0005
- Appleton, S., Emwanu, T., Kagugube, J., & Muwonge, J. (1999). Changes in poverty in Uganda. Issue Wps/99.22. Centre for the Study of African Economies, University of Oxford.
- Awan, M., Malik, N., Sarwar, H., & Waqas, M. (2011). Impact of education on poverty reduction. International Journal of Academic Research, 3(1). https://mpra.ub.uni-muenchen.de/id/eprint/31826https://mpra.ub.uni-muenchen.de/id/eprint/31826
- Awel, M. Y., & Brar, J. S. (2019). Analysis of the determinants of poverty in tigrai and afar national regional state, Ethiopia. Asian Journal of Multidimensional Research, AJMR, 8(2), https://doi.org/10.5958/2278-4853.2019.00028.4
- Bekele, D., & Silshi Merid, A. (2020). Determinants of poverty in rural Ethiopia: Evidence from tenta woreda, Amhara Region. Journal of Sustainable Rural Development. https://doi.org/10.32598/JSRD.02.02.40
- Beyene, F., & Muche, M. (2010). Determinants of food security among rural households of Central Ethiopia: An empirical analysis. Quarterly Journal of International Agriculture, 49(4). https://doi.org/10.22004/ag.econ.155555
- Bigsten, A., Kebede, B., Shimeles, A., & Taddesse, M. (2003). Growth and poverty reduction in Ethiopa: Evidence from household panel surveys. World Development, 31(1), https://doi.org/10.1016/S0305-750X(02)00175-4
- Bogale, A., Hagedorn, K., & Korf, B. (2005) Determinants of poverty in rural Ethiopia. Quarterly Journal of International Agriculture, https://doi.org/10.5167/uzh-64170.
- Bogale, A., & Shimelis, A. (2009). Household level determinants of food insecurity in rural areas of dire dawa, eastern Ethiopia. African Journal of Food, Agriculture, Nutrition and Development = Journal Africain de l’Alimentation, l’Agriculture, Nutrition Et Le, 9(30), 1914–1926. Developpement. https://doi.org/10.4314/ajfand.v9i9.50072
- Davis, E. P., & Sanchez-martinez, M. (2014). A review of the economic theories of poverty. National Institute of Economic and Social Research, 435, 1–65. https://bura.brunel.ac.uk/handle/2438/10008
- Deressa, T. K., & Sharma, M. K. (2014). Determinant of poverty in Ethiopia. Ethiopian Journal of Economics, 23(1), 113–130. https://www.ajol.info/index.php/eje/article/view/123798
- Enquobahrie, A. (2004). Understanding poverty: The Ethiopian context. The Gambia AAPAM Roundtable Conference, Banjul, The Gambia. UN. April 19 - 23, 2004.
- Ermiyas, A., Batu, M., & Teka, E. (2013). Determinants of poverty in Rural Ethiopia: A household level analysis. Arts and Social Sciences Journal, 10(2). https://doi.org/10.4172/2151-6200.1000436
- Ermiyas, A., Batu, M., & Teka, E. (2019). Determinants of rural poverty in Ethiopia : A household level analysis in the case of dejen woreda. Arts and Social Sciences Journal, 10(2). https://doi.org/10.4172/2151-6200.1000436
- Eyasu, A. M., & Yildiz, F. (2020). Determinants of poverty in rural households: Evidence from North-Western Ethiopia. Cogent Food and Agriculture, 6(1), 1823652. https://doi.org/10.1080/23311932.2020.1823652
- Foster, J., Greer, J., & Thorbecke, E. (1984). A class of decomposable poverty measures. Econometrica, 52(3), 761. https://doi.org/10.2307/1913475
- Foster, & Greer Thorbecke, E. (2010). The foster-greer-thorbecke (FGT) poverty measures: twenty-five years later. IIEP-Working Paper, 2(9).
- Foster, J., Greer, J., & Thorbecke, E. (2010). The foster-greer-thorbecke (FGT) poverty measures: 25 years later. Journal of Economic Inequality, 8(4), 491–524. https://doi.org/10.1007/s10888-010-9136-1
- Garza–Rodríguez J. (2015). The determinants of poverty in the Mexican states of the US–Mexico border. REF, 17(33), 141–167. 10.21670/ref.2016.33.a06
- Gibson, J., & Rozelle, S. (2005). Poverty and access to infrastructure in Papua New Guinea. SSRN Electronic Journal. https://doi.org/10.2139/ssrn.334140
- Girma, M., & Temesgen, Y. (2018). Determinants and its extent of rural poverty in Ethiopia: Evidence from doyogena district, Southern part of Ethiopia. Journal of Economics and International Finance, 10(3), 22–29. https://doi.org/10.5897/jeif2017.0837
- Hagos, F., Jayasinghe, G., Awulachew, S. B., Loulseged, M., & Yilma, A. D. (2012). Agricultural water management and poverty in Ethiopia. Agricultural Economics (United Kingdom), 43(1), 99–111. https://doi.org/10.1111/j.1574-0862.2012.00623.x
- Hartline Grafton, H., & Dean, O. (2017). The impact of poverty, food insecurity. In David , F. (Ed.) Poor Nutrition on Health and Well-Being (pp. 7). Food Research & Action Center.
- Heshmati, A., Maasoumi, E., & Wan, G. (2019). An analysis of the determinants of household consumption expenditure and poverty in India. Economies, 7(4), 96. https://doi.org/10.3390/economies7040096
- Ijaiya, Marikan, D. A. A., Abu Bakar, N. A., & Roszopor, M. A. (2018). Socio-demographic factors as a determinant of household poverty in niger State, Nigeria. https://repository.futminna.edu.ng:8080/jspui/handle/123456789/7555
- Kassahun, T., Tessema, A., & Adbib, K. (2022). Analysis of rural household food and non-food poverty status in Ethiopia: The case study from meskan district. Food and Energy Security, 11(2). https://doi.org/10.1002/fes3.363
- Kotler, P., Roberto, N., & Leisner, T. (2006). Alleviating poverty: A macro/micro marketing perspective. Journal of Macromarketing, 26(2), 233–239. https://doi.org/10.1177/0276146706291039
- Linh, T. N., Long, H. T., Chi, L. V., Tam, L. T., & Lebailly, P. (2019). Access to rural credit markets in developing countries, the case of Vietnam: A literature review. Sustainability (Switzerland), 11(5). https://doi.org/10.3390/su11051468
- Liu, F., Li, L., Zhang, Y. Q., Ngo, Q. T., & Iqbal, W. (2021). Role of education in poverty reduction: Macroeconomic and social determinants form developing economies. Environmental Science and Pollution Research. https://doi.org/10.1007/s11356-021-15252-z
- Macours, K., & Swinnen, J. F. M. (2008). Rural-urban poverty differences in transition countries. World Development, 36(11), 2170–2187. https://doi.org/10.1016/j.worlddev.2007.11.003
- Maddala, G. S. (1983). Limited-dependent and qualitative variables in econometrics. https://doi.org/10.1017/CBO9780511810176
- Mberu, B. U., Ciera, J. M., Elungata, P., & Ezeh, A. C. (2014). Patterns and determinants of poverty transitions among poor urban households in Nairobi, Kenya. African Development Review, 26(1. https://doi.org/10.1111/1467-8268.12073
- Muhammedhussen. (2016). Determinants of rural income poverty in Ethiopia: Case study of villages in dodola district. version 1.0. Online 2249-4588 & Print 0975-5853. Global Journal of Management and Business Research: (B) Economics and Commerce, 15(11). https://journalofbusiness.org/index.php/GJMBR/article/download/1857/1759
- Muhdin, M. (2015). Determinants of rural income poverty in Ethiopia: Case study of villages in dodola district. Type: Double Blind Peer Reviewed International Research Journal Publisher: Global Journals Inc, 15(11).
- Muleta, A., & Deressa, D. (2014). Female headed households and poverty in rural Ethiopia. Science, Technology and Arts Research Journal, 3(1), 152. https://doi.org/10.4314/star.v3i1.25
- Neville, H. (2011). Comment: Neuroscience exposes pernicious effects of poverty. Science News, 179(3), 32. https://doi.org/10.1002/scin.5591790326
- Neway, M. M., Zegeye, M. B., & Serpa, S. (2022). Gender differences in the adoption of agricultural technology in North Shewa Zone, Amhara Regional State, Ethiopia. Cogent Social Sciences, 8(1). https://doi.org/10.1080/23311886.2022.2069209
- Peng, C., Fang, L., Wang, J. S. H., Law, Y. W., Zhang, Y., & Yip, P. S. F. (2019). Determinants of poverty and their variation across the poverty spectrum: Evidence from Hong Kong, a High-Income Society with a High Poverty Level. Social Indicators Research, 144(1), 219–250. https://doi.org/10.1007/s11205-018-2038-5
- Ranjith, S., & Rupasingha, A. (2012). Social and cultural determinants of child poverty in the United States. Journal of Economic Issues, 46(1), 119–141. https://doi.org/10.2753/JEI0021-3624460105
- Ratcliffe, C., & Mckernan, S.-M. (2010). Childhood poverty persistence: Facts and consequences. In (Brief 14).
- Rehman, A., Jingdong, L., Chandio, A. A., & Hussain, I. (2017). Livestock production and population census in Pakistan: Determining their relationship with agricultural GDP using econometric analysis. Information Processing in Agriculture, 4(2). https://doi.org/10.1016/j.inpa.2017.03.002
- Sharma, M. K., & D, T. K. (2014). Determinant of poverty in Ethiopia. Ethiopian Journal of Economics, 23(1), 113–130. https://www.ajol.info/index.php/eje/article/view/123798
- Sinnathurai, V. (2013). An empirical study on the nexus of poverty, GDP growth, dependency ratio and employment in developing countries. Journal of Competitiveness, 5(2), 67–82. https://doi.org/10.7441/joc.2013.02.05
- Teka, A. M., Temesgen Woldu, G., & Fre, Z. (2019). Status and determinants of poverty and income inequality in pastoral and agro-pastoral communities: household-based evidence from afar regional State, Ethiopia. World Development Perspectives, 15. https://doi.org/10.1016/j.wdp.2019.100123
- Tesfaye, D., & Getachew, T. (2018). Determinants of rural households’ poverty with selected link functions: The case of soro district, hadiya zone, SNNPR States, Ethiopia. International Journal of Economics & Management Sciences, 7(1). https://doi.org/10.4172/2162-6359.1000490
- Thorbecke, E. (2004). Conceptual and Measurement Issues in Poverty Analysis. World Institute for Development Economics Research (WIDER).
- UNDP. (2018). Ethiopia’s progress towards eradicating poverty. Implementation of the Third United Nations Decade for the Eradication of Poverty, 2018–2027.
- World Bank Group. (2015). Ethiopia poverty assessment 2014. World Bank.
- World Bank Organization. (2001). world development report 2000/2001: Attacking poverty. In Oxford University Press.