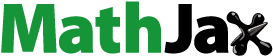
Abstract
Labour engagement, underutilization and unemployment has dominated discourse in development literature in developing economies. It tangentially dictates the direction of migration, gross domestic output and in some cases, youth restiveness. This study investigated the unique relationship between investment in human capital proxied by spending in education and health and its effects on youth employment outcomes in Sub-Saharan Africa (SSA). Annual data spanning 1995–2017 were obtained from 40 SSA countries comprising 920 macro panel observations. The bootstrap-based bias correction for the panel fixed effects estimation technique was employed to improve on the analytical corrections. Findings suggest that human capital investment comprising private and government health expenditures, primary, secondary and tertiary education expenditures were found to have varying significant impact on youth employment in SSA. The policy implication is that to reverse the perennial problem of youth unemployment in SSA would require serial consistent disproportionate investment more in education than in health.
1. Introduction
Youth employment is widely considered as a veritable tool for social stability and economic growth in the global discourse; hence job opportunities for youths are held as priority in nations across the globe (International Labour Organization (ILO), Citation2019; Mueller, Citation2019). Youth employment as conceptualized by International Labour Organisation (ILO) and United Nations Educational, Scientific and Cultural Organization (UNESCO) is the engagement of persons between 15 and 24 age cohorts in productive activities. In other words, it is operational when persons within this age bracket (15–24) are gainfully working for pay. Albeit, the UN Habitat and the African Youth Charter (AYC) define youths as persons between 15 and 35 years; this study adopts the former’s conceptualization of youth as comprising people (youths or young people) between the ages of 15 and 24 as its operational definition. Expectedly, the potentials, opportunities and implications of youths’ activities in the labour market have attracted the interest of labour specialists in the literature (Baah-Boateng, Citation2015; International Labour Organisation, Citation2010; Kwon, Citation2009; Tacoli et al., Citation2015).
Available statistics from International Labour Organization (ILO; Citation2020) shows that about 1.15 billion (590 million male and 560 million female) of the world’s population are youth, and more than half of them live in developing countries located in Africa, Southern Asia and Latin America. Incidentally, about 60% of African population is below 25 years, making it the world’s continent with most youth. Sub-Saharan Africa (SSA) is the region with most youth accounting for approximately 20% of the world’s youth population numbering above 230 million. The projection is that this figure for Sub-Saharan African youth will upsurge by 42% by the end of 2030. In addition, SSA holds the world’s highest population growth rate (2.7%). Inadmissibly, it has become awful that roughly half of these statistics which could be leveraged on as enormous asset for SSA are not gainfully engaged in productive economic activities. This provokes the question, why is youth unemployment this high in SSA? Respective economies in SSA have been struggling with the challenges of youth unemployment with some ruinous consequences, among which are ever increasing restiveness, violence and crime rate (Anowor et al., Citation2019; Barford & Cieslik, Citation2019; Barford et al., Citation2021; Onodugo et al., Citation2017). Additionally, SSA recorded the highest rate of global working-poor youth at nearly 70% (Atif, Citation2020).
More so, the young workers and women (as observed by the 2016 report of ILO) in the region risk the highest probabilities of employment instability, job loss and of living below the poverty line than the adult and male workers. This is evident when considering the successive increasing number of dependants within the region. Besides the working-poor youth in SSA, most young people in SSA appear to lack sufficient skills, competence, health, proficiencies and qualifications to competitively fit into the modern labour market. The general lack of experience on the part of the youth as noted in Baah-Boateng (Citation2015) in the labour market throw up exclusive obstacles to securing reasonable engagements. This obviously aggravates their chances of securing employments subsequently exacerbating the already high youth unemployment faced in the region. The barriers facing these people in securing quality jobs after school increases their vulnerability in society and makes them liable to social vices and source of conflicts and civil disorders (Baah-Boateng, Citation2015). To be exact, the employability of the young people in SSA ought to transcend beyond economic growth analysis and political rhetoric of providing employment. Nonetheless, this study proposes the need to also be worried about the youth possessing the right human capital to absorb and attract employment opportunities. Obviously, the foregoing highlights considerable human capital issues in attending to the labour market challenges of the youth in SSA, which draws attention to the education, skills, knowledge, experiences, health, vigor and vitality possessed by youth cohorts. The aforementioned brings to spotlight the research question, what are the chances of youth employment across SSA countries with precise investment in human capital?
The strategic position of human capital in economic development began to gain relevance with the emergence of the endogenous growth theory which submits that human capital investment, innovation, knowledge, and sound health are key predictors of economic growth. This implies that such growth rate of per capita income/output depends on the growth rate of human capital (Anowor et al., Citation2020; Danquah & Ouattara, Citation2014; Engelbrecht, Citation2002; Onodugo et al., Citation2013). Arguments around growth determinants like those of Becker (Citation1962), G. Becker (Citation1964a), Romer (Citation1986, Citation1990), Lucas (Citation1988), Rebelo (Citation1991), Barro (Citation1991, Citation2001), Aghion and Howitt (Citation1997), have always been that knowledge, skills, health as well as education are inputs to economic production represented in labour which inevitably raises output and intensifies an economy’s capacity to advance and to embrace modern technologies. Incidentally, the youths are the most critical segment of the population concerned with acquisitions of human capital resources given the fact that they are better favoured to create more wealth in the society if fully engaged. This is so because they stand to be more productive on the account that they are more energetic and have more years to put into productive activities than the adults. Hence, SSA could improve her productivity and output by engaging (employing) her surplus youthful population.
Therefore, this study is particularly concerned, as its objective, if the required investments needed to ensure the realization of the desired proportion of youth employment in SSA are attainable. This gives the credibility to worry about the extent human capital investment has influenced youth employment across the region, and to ask question like: what is the effect of human capital investment on youth employment across SSA countries? This study is also worried about the growing number, as reported in International Labour Organization (ILO; Citation2020), of unemployed persons in the region which accounts for increasing tendencies of youth migrating out of Africa in recent time, poverty, youth restiveness, crime and criminality and persisting underdevelopment. Moreover, the fact that there has been a paucity of empirical studies on human capital investment and youth employment in SSA is also another motivation behind this study. A number of studies (Agbarakwe et al., Citation2018; Agrawal et al., Citation2015; Aleksynska & Schindler, Citation2011; Danquah & Ouattara, Citation2014; Fashoyin & Tiraboschi, Citation2011) attempted to explain the missing links between education and labour market in some areas of the globe but with limited focus on Africa or SSA. What is more, there is limited attention on youth employment or on partial analysis of how investment in human capital impacts youth employment in SSA. However, an earlier work (Anowor et al., Citation2020) on healthcare financing in ECOWAS Sub-region paid much attention on output per capita but did not bother on its effects on youth employment. Some other studies on human capital, even as they are country specific, like Olaniyan and Okemakinde (Citation2008), Oluwatobi and Ogunrinola (Citation2011), and Eme et al. (Citation2014), Odior (Citation2014) directed attentions to productivity and growth but not a bit on youth employment. Others like Kwon (Citation2009), International Labour Organisation (Citation2010), Baah-Boateng (Citation2015), Tacoli et al. (Citation2015) investigated, though not across SSA countries, the opportunities of young people at the labour market. The empirical studies above adopted different econometric methods, data, periods, countries and region. Consequently, this opens the significance and necessity for further research which also motivates this study to employ the bootstrap-based bias correction for the panel fixed effects estimation technique to make certain the effects of human capital investment on youth employment in SSA region.
Obviously, this study is designed to identify a perspective for tackling the issues of human capital investment and youth employment in SSA. The data used span 1995 to 2017, 23 macro panel series from 40 SSA countries. The countries included were: Angola, Benin, Botswana, Burkina Faso, Burundi, Cameroon, Central African Republic, Chad, Comoros, Congo Democratic Republic, Congo Republic, Cote d’Ivoire, Equatorial Guinea, Ethiopia, Gabon, Gambia, Ghana, Guinea, Guinea-Bissau, Kenya, Lesotho, Liberia, Madagascar, Malawi, Mali, Mauritania, Mauritius, Mozambique, Namibia, Niger, Nigeria, Rwanda, Senegal, Sierra Leone, South Africa, Sudan, Tanzania, Togo, Uganda and Zambia. The choice of these countries stems from the challenges of accessing information for all the variables in the model for some of the countries not included. Besides, it becomes pertinent to exclude some countries from the analysis because they had too few observations per annum or they had observations per year quite far apart to be useful. The eight countries not included in the list are: Cabo Verde, Eritrea, Eswatini (Formerly known as Swaziland), Sao Tome and Principe, Seychelldees, Somalia, South Sudan and Zimbabwe. The period (1995–2017) for the time series was chosen based on availability of data and also economies in sub-Sahara Africa within this period engaged in more openness following the advent of structural adjustment programmes (SAP).
2. Literature
The basic assumptions of human capital of a person or group of persons consist theoretically and practically of dexterities, experiences, proficiencies, deftness, verve and abilities in addition to the health conditions of the person(s) or population in the production process of commodities. A plethora of empirical scholarly work evidence that human capital investments could press forward productivity and accelerate economic development (Mincer, Citation1996; Aleksynska & Schindler, Citation2011; Anowor et al., Citation2020; Danquah & Ouattara, Citation2014; Hammed et al., Citation2019; McDonald & Roberts, Citation2002; Onodugo et al., Citation2013). The grounds above necessitated this investigation on how investment in human capital can influence youth employment in SSA. The neoclassical growth model of Solow (Citation1956) and Swan (Citation1956) shows that the effect of diminishing returns would finally cause economic growth to come to a halt in the absence of technological progress. Aghion and Howitt (Citation1992, Citation1997) establish that technological progress with its labour-saving nature could displace workers from employment in the short-run; however, it bestows greater opportunities that could enrich the population because productivity growth will spur demand and therefore prompt the creation of new jobs. The foregoing therefore seems to be submitting that youth employment in SSA will increase and that the SSA youth with the right amount of human capital can be efficiently employed into the productive process as new jobs are created. The pro-Schumpeterian endogenous growth models as theorize by Segerstrom et al. (Citation1990), Aghion and Howitt (Citation1992), Caballero and Hammour (Citation1996), and Bartelsman et al. (Citation2004) have brought to light the consequence of the contribution of human capital by exploitation of knowledge in new ways, which thereby positively affect productivity.
Health and education as demonstrate by Schultz (Citation1999) are not only valuable on their own but they can also be considered as human capital investment which navigates the economy to an advanced state in the future. Hence, the summation of investments in education, health, on-the-job training and other spending which improves the productivity of labour and the value of labour in the labour market is referred to as investment in human capital (Becker, Citation1964a; Schultz, Citation1961). Soares (Citation2014) also posits that spending on education and health has positive impact on productivity as a result improve human capital. The endogenous growth models of Romer (Citation1986, Citation1990), Lucas (Citation1988), Rebelo (Citation1991) and Barro (Citation1991, Citation2001) propose that an economy can grow unimpeded as long as it does not run out of technological advancement driven by newly improved human capital acquired through investments in education, health, research and development (R&D), on-the-job training, among others. Apparently, the more-educated, more-skilled and healthier workers are preconditioned to be more employable than their less-educated, less-skilled and weaker contemporaries in a labour market where wages reveal the marginal product of workers.
The results from the study for eight SSA countries by Assaad and Levison (Citation2013) indicate that approximately between 1.5% and 28.8% of young people never had formal education. The result further reveals that approximately one out of three young people in Benin, Madagascar and Zambia have some schooling but left (dropped out) before completion; while more than 50% of the youth in Malawi, Togo and Uganda still remain disadvantaged quality access to education with no stable job. The low probability of finding decent employment for youth in these countries was attributed to lack of required training, basic education and knowledge. Wagner (Citation1890) postulates that the expansion of cultural and welfare expenditures is based on the conjecture that society would demand more education, more equitable distribution of wealth, and more public services as income rises. At this postulation, Wagner (Citation1890) saw expenditures in education and culture as required investments for any society. Sweeten et al. (Citation2009), Rees and Mocan (Citation1997) both used panel data analysis to investigate respective relationship between unemployment and high school dropouts, and community college enrolment. They established a positive effect of unemployment on high school dropouts and a negative effect of unemployment on college enrolment. Micklewright et al. (Citation1990) found a positive effect of unemployment on early school leaving while the time series analysis of Whitefield and Wilson (Citation1991) yields the opposite answer to the same question. Also, Fredrickson (1997) obtained a very small effect of unemployment clearly inferior to the role played by wages in explaining the demand for higher education.
In establishing the relationship between economic growth and change in unemployment, Okun (Citation1962) empirically found 3:1 relationship between gross national product and rate of unemployment. Explicitly, Okun’s law advanced a bi-directional relationship between unemployment rate and output. This implies that more labour is required to produce more output and as employment of labour rises during recovery stage of business cycle, personal income increases which in turn increases the aggregate demand as well as the national output. An empirical study based on modified Solow growth accounting model by Lee (Citation2003) revealed that human capital accumulation in Korea made significant contribution to economic growth. The study emphasizes the position of human capital in the total factor productivity and economic growth. It also indicated that total factor productivity growth may come as a result of technological advancement. Specifically, enhancement of human capital of the youth through investments could impact on employment and productivity through technological progress. Keji (2021) employed vector autoregressive and Johansen techniques to examine the functional relationship between human capital and economic growth, the study concludes that human capital has long-run and significant impact on economic growth. Alawamleh et al (Citation2019) carried out a study on the relationship between innovation and economic development in Jordan and establishes that innovation is a product of human capital investment and that human capital investment is highly relevant in economic development.
From the foregoing, it can be ascertained that human capital investment is relevant in enriching the basic indicators of economic development, however, the previous studies are yet to worry about the extent human capital investment has touched employment most notably youth employment. Remarkably, not any has investigated the disaggregated expenditure on education (primary, secondary and tertiary) and health (private and public) data in relation to its effect on youth employment. Consequently, this study prominently stands out, as its contribution to the extant literature and as a move to fill the existing literature gap, to ascertain the extent human capital investment has influenced youth employment across SSA.
3. Method and data
Based on the methodological lapses observed from the previous studies, this study employed different panel data approaches to estimate the human capital investment youth-employment nexus in SSA to ensure robustness of the results across various econometric techniques. In order to achieve this, the study employed, first, the Westerlund ECM panel co-integration technique. Then because of potential endogeneity problem and potential presence of root mean square error that emanates from this technique, the System Generalized Method of Moments (Sys-GMM) would have been used (as were applicable to most dynamic panel data studies). However, it has come to light that Sys-GMM suffers from the weak instrument problem particularly when the time series is large and substantial unobserved heterogeneity and cross-sectional dependence exists (Bun & Windmeijer, Citation2010; Hayakawa, Citation2009), hence the bootstrap-based bias correction for the panel fixed effects (FE) estimator was employed to improve on the analytical correction when the variance of the individual effects increases. In addition, SYS-GMM estimator placed emphasize on time series effects (Ashley & Sun, Citation2016), whereas cross-sectional effects are dominant in the present study. With the purpose of dispelling this problem and thereby checking for the sensitivity of the results, this study complements the internally generated set of instruments with the iterative bootstrap-based bias correction for the panel fixed effects (FE) estimator
The framework of the New Endogenous Growth Theory arising from the modification by Romer (Citation1986) and Lucas (Citation1988) of the old neoclassical growth theory formed the base of this study. The endogenous growth theory recognizes the vital importance of the endogeneity of human capital and research & development (R&D) activities in the growth process (Mallick & Moore, Citation2006).
From the model by Romer (Citation1986), the production function of a firm is in the following form:
whereA (R) = public stock of knowledge from research and development;Ri = stock of results from the stock of expenditure on research and development;Ki = capital stock of firm i;Li = labour stock of firm i;Ri = the technology prevalent at the time in firm i.
A dynamic panel model is specified in line with Mankiw et al. (Citation1992), as adopted in Islam (Citation1995) and Armah and Nelson (Citation2008) to estimate the effect of human capital investment on the youth employment across SSA countries.
Here, the ratio of youth employment (aged between 15 and 24 years) to the total employment (YEit/EMit) is specified as a function of different levels of education expenditures (primary, PE, secondary, SE, and tertiary education, TE respectively), private health expenditure (PHE) and public health expenditure (GHE). To capture the responsiveness of youth employment to the different components of human capital expenditures: PE is a measure of total expenditure on primary education as percentage of total expenditure on education, SE is a measure of total expenditure on secondary education as percentage of total expenditure on education, TE is a measure of total expenditure on tertiary education as percentage of total expenditure on education, PHE is a measure of private health expenditure as percentage of total expenditure on health, and GHE is a measure of public health expenditure as percentage of total expenditure on health. This is controlled by the population growth rate (PGR) which measures the demographic tendency, per capital growth rate (GDPpc) that captures the economic environment or the aggregated economic performance in country i at time t.
The general dynamic of the above function is presented in the following format:
where(YE/EM)it = the ratio of youth employment (aged between 15 and 24 years) to the total employment, in country i, at time t;
Xit = vector of human capital investment variables as defined earlier such as levels of education expenditure (primary, PE, secondary, SE, and tertiary education, TE, respectively), private health expenditure (PHX) and public health expenditure (GHX);
Zit = a number of controlled which is population growth rate (PGR), that captures the demographic tendency, per capital growth rate (GDPpc) that captures the economic environment or the aggregated economic performance;α0 = intercept;νit = stochastic error terms.
It is argued in the literature that economic performance increases the stock of human capital investment, which in turn increases labour productivity and wages via rise in youth employment (Anyanwu, Citation1998, 2005). The dynamic panel model of Equationequation 2(2)
(2) can be explicitly expanded in the following format:
where
(YE/EM)it = the ratio of youth employment to the total employment, in country i, at time t;
= the initial level of youth employment to the total employment in i, at time t;
PEit = expenditure on primary education as percentage of total expenditure on education, in country i, at time t;
SEit = expenditure on secondary education as percentage of total expenditure on education, in country i, at time t;
TEit = expenditure on tertiary education as percentage of total expenditure on education, in country i, at time t;
PHXit = private health expenditure as percentage of total expenditure on health, in country i, at time t;
GHXit = public health expenditure as percentage of total expenditure on health, in country i, at time t;
GDPpcit = per capital growth rate, a measure of aggregate economic performance;
PGRit = the population growth rate, that captures the demographic tendencies;
ρit = country and period specific effects;
λ0 = the intercept;
νit = the idiosyncratic error or stochastic error terms.
The inclusion of these variables can be justified as follow:
Education and health have been identified by scholars like Becker (Citation1962, Citation1964b), Romer (Citation1986, Citation1990), Lucas (Citation1988), Rebelo (Citation1991), Barro (Citation1991, Citation2001), and Aghion and Howitt (Citation1997) as key human capital variable. We refer to education expenditures as spending on primary, secondary, tertiary education, and public and private educational institutions, which include payment for services provided by educational institutions. Health (public and out of pocket) expenditure involves all expenditures for the provision of health services, nutritional activities and other actions designated for health. Spending on education and health has positive impact on productivity as a result improve human capital (Soares, Citation2014). Based on the above claim, this study assumes that investment in education and health should improve human capital of the youth and by extension increase youth employment.
Per capital growth rate in this study is a measure of aggregate economic performance. Per capital growth rate provide a basic measure of the value of output per capita (Anowor et al., Citation2020). Per capital growth rate is a broad measure of economic growth. The assumption of this study is that youth employment improves as per capital growth rate increases.
Population growth rate in this study captures the demographic tendencies. Population growth rate is the change in the number of individuals over a specific period of time. This is the expression of the ratio between the annual increase in the population size and the total population. This study aligns with the theoretical position that a growing population of the labour force provides opportunities for economic growth while at the same time creating challenges for job creation and integration of new labour entrants (youth) among the employed population.
The study used panel datasets from the UNESCO Institute of Statistics (2018 update), ILOSTAT database of International Labour Organization, World Bank Edstats/World Development Indicators 2018 and Penn World data 9.0. The data on GDP growth and human capital investment, including expenditures on education and health were extracted from the database of the World Bank Edstats/World Development Indicators 2018 and Penn World data 9.0. Data on the demographic tendencies were retrieved from Penn World data 9.0. The measure of youth employment used in this study was derived from ILO definition (modeled ILO estimate) of youth employment which defined youth employment as the share of the employed youth in the total labor force. Stata 14 was employed for the estimation and analyses.
4. Results and discussion
4.1. Cross-sectional dependence (Cd) analysis
Pesaran cross-sectional dependence (CD) test was employed for this analysis, having N > T, (N = 40 and T = 23). Pesaran Cross-sectional Dependence (CD) test is a normally distributed test with zero mean and constant variance, done under the null hypothesis that suggests cross-section independence CD, (CD ~ N(0,1)). Table presents the estimated results of the Pesaran CD test for the study.
Table 1. Pesaran cross-sectional dependence test result
The result in Table showed that all the macro panel variables, with the exception of the ratio of youth employment to the total employment (YE_EM) and the population growth rate (PGR), exhibits cross-sectional independence. Judging with 5% level of significance, the CD-test and P-values associating to the variables showed an acceptance of above stated null hypothesis of cross-sectional independence of the macro panel data.
4.2. Panel unit root analysis
Testing for unit roots and co-integration in macro panel data is faced with twin problems of structural breaks and cross-sectional dependence. Pesaran (Citation2007) proposed panel unit root that deals with both structural break and cross-sectional dependency issues. Hence the Pesaran Panel Unit Root test is adopted for this analysis. The preference of Pesaran panel unit root test is also because of its ability to handle unit root in a dynamic heterogeneous panel. Pesaran method was based on augmenting the usual ADF regression with the lagged cross-sectional mean and its first difference to capture the cross-sectional dependence that arises through a single factor model. This is called the Cross-sectional Augmented Dickey-Fuller (CADF) test. The method requires a simple test of disturbance cross-section dependence (CD) for unit root dynamic heterogeneous panels with short T and large N (N > T) like the present study. The null hypothesis for CADF testing is that the panel series contains unit root against the alternative that the panel series are stationary (at least one is different from zero). Table presents the level and first difference results of Pesaran’s CADF panel unit root test of the study.
Table 2. Result for Pesaran’s cross-sectional augmented dickey-Fuller (CADF) test
In level form, Table result reveals that four (4) of the macro panel variables are not integrated (I(0)). These variables are Government Health Expenditure as % of Total Health Expenditure (GHX); expenditure on primary education as a percentage of total education expenditure (PE); expenditure on secondary education as a percentage of total education expenditure (SE); and expenditure on tertiary education as a percentage of total education expenditure (TE). While the four (4) integrated variables in level form are the ratios of youth employment to the total employment (YE_EM), GDP per capita, a measure of aggregate economic performance (GDPpc), the population growth rate (PGR), and Private Health Expenditure as % of total health expenditure (PHX). In the first difference operator, the four (4) variables that were integrated was made stationary, I(1), making all the variables to be stationary in order one.
4.3. Panel co-integration analysis
This study employed Error Correction-based panel cointegration tests introduced by Westerlund (Citation2007) and modified by Persyn and Westerlund (Citation2008). The method estimates four statistics, Ga, Gt, Pa and Pt respectively. The Ga and Gt are panel statistics and the other two Pa and Pt are group statistics. These panel co-integration tests allow for a large degree of heterogeneity, both in the long-term co-integrating relationship and in the short-term dynamic, and dependence within as well as across the cross-sectional units (countries). The advantage of Westerlund ECM panel co-integration tests over other panel co-integration test methods is its flexibility. The test provides an estimate of the speed of error-correction towards the long-term equilibrium, and at the same time allow for an almost completely heterogeneous specification of both the long and short-term parts of the ECM, even in unequal length macro panel settings. Also, in a situation where the cross-sectional units are found to be correlated or cross-sectional dependence are found in the model, like the case of the present study, robust critical values can be obtained through bootstrapping. Table presents the results of the Westerlund ECM panel co-integration tests with two p-values, one based on asymptotic normal distribution and the other based on bootstrap method.
Table 3. Westerlund ECM panel co-integration test results
Table shows that cointegration relationship exists in the ratio of youth employment to the total employment (YE_EM) model. In the panel result, at least one of the four statistics the Ga, Gt, Pa and Pt value lead to the rejection of the null hypothesis of no evidence of co-integration for the aggregate panel series.
4.4. Random and fixed effects analysis (Hausman test)
The Hausman specification test was conducted to compare the consistent Fixed-Effects (FE) model with the efficient Random-Effects (RE) model. Table presents the Hausman FE and RE test result for the models.
Table 4. Results of the hausman test
Table result indicates rejection of the null hypothesis of none systematic difference in coefficients between FE and RE models for the ratio of youth employment to the total employment. The judgment was reached from the Chi-Square statistics value for the model. The YE_EM model ChiSq (7) = 38.61(0.0000), implying rejection of random effect orthogonality assumption in favour of fixed effect model. Based on this, the study concludes that the difference in coefficient between FE and RE is systematic; thus, RE estimator is inconsistent for the models, and the FE estimator is both consistent and efficient and best fit for model’s analyses.
4.5. Model result
Based on the outcome of the pre-estimation tests especially the inherent Hausman tests outcome which favours Fixed Effect (FE) and Westerlund ECM panel co-integration tests which show that cointegration relationship exist, the results of the model are presented in Table . In the table, column 1 (Long Run, LR) and column 2 (Short Run, SR) presented the result.
Table 5. Results of YE_EM model
5. Discussion
Table shows that youth employment measured in this study as the ratio of youth employment to the total employment (YE_EM) across SSA countries exhibits positive linear functions of education investment, such as expenditure on primary, secondary and tertiary educations in both the short-run and long-run. It indicates positive significant association with primary and tertiary education expenditure and insignificant positive association with secondary education expenditure in the short-run and long-run. Specifically, the result indicates that a 10%-point increase in primary education expenditure would in the subsequent year increase youth employment by approximately 0.184% and 0.145% in short-run and long-run respectively, others factor remaining fixed. Also, a 10%-point increase in tertiary education expenditure would in the preceding year increase youth employment by 0.0045% and 0.082% respectively in the short-run and long-run, holding other factors constant. The results above support the view of Fashoyin and Tiraboschi (Citation2011) which affirm that educational spending enhances employment opportunities. However, secondary education expenditure did not exert any statistically significant impact on youth employment within the period of study, in both the short-run and long-run.
On the health sector expenditures, result on Table shows that youth employment exhibits negative linear functions of private as well as government health expenditure in SSA countries in the short-run. Quantitatively, the result indicates that a 10%-point increase in private health expenditure, measured as percentage of total health expenditure would reduce youth employment by 0.18% in SSA, other factors remaining fixed. While a 10%-point increase in government health expenditure, measured as percentage of total health expenditure, ceteris paribus, would decrease youth employment by approximately 0.28%. Intuitively, this outcome suggests a trade-off between investment in health sector and youth employment in SSA, a situation where youth employment never improved in response to increased health expenditure. This however, does not conform to the earlier findings by Eme et al. (Citation2014) which assert that the more spending on healthcare the higher the chances of being employed.
By implication, the response of youth employment to changes in human capital investment varies alongside investment types, as increases in the health sector investment seem not to increase youth employment, while in education sector, increase in investment increase youth employment. Even within the education sector, the results in Table suggests that certain levels of education expenditure (primary and tertiary) impact more significantly to youth employment compared to others like secondary education in SSA countries. On the contrary, health expenditures (private and government) exhibit a positive linear relationship with youth unemployment in the long-run. The results indicate that a 10% increase in health expenditure (private and government) raises youth employment by 0.23% and 0.56% respectively.
Another key finding from Table result is that youth employment in SSA has a positive linear function of GDP per capita (GDPpc) and the population growth rate (PGR) in the long-run and short-run. It indicates that a 10%-point increase in GDPpc would increase youth employment in the subsequent year by 0.53% and 0.74% in the short-run and long-run respectively, while a 10%-point increase in the population growth rate increases youth employment by approximately 1.1% and 5.34% respectively in the short-run and long-run, other factors constant. These findings are in line with literature, as evidences have shown that increase in employment of youth led to improvement of the national economy by the increasing formation of capital and aggregated demand. Study has shown that the young employees tend to spend a reasonable percentage of their earnings for purchasing goods and services, which contributes positively to the aggregate demand (International Labour Organization, Citation2011). The young employees, also, tend to save more money from their realized income, which directly increases the disposable capital for investments in the economy (Gabriela & Mircea, Citation2014). Thus, there is a direct and positive association between the rate of youth employment and economic performance of a nation (Levine, Citation2011).
In the long-term, the result of the speed of adjustment or the ECM parameter indicates that 31.7% disequilibrium in the youth employment equation would in the preceding year adjust back to equilibrium state. It shows relatively low speed of adjustment by the youth employment to shocks in human capital investment, demographical change and economic performances in the long-term. This implies that it takes about 4 years for youth employment to adjust back to equilibrium from shocks induced by the distortions in the explained variables included in the model, especially the human capital investment variables.
6. Conclusion and recommendation
The consequential effect of youth employment in emerging economies has accentuated the interest of policy makers and scholars on the subject matter. SSA has youthful population in abundance and thus has generated a lot of debate as to the prospects of improving the development fortunes of SSA through investments in human capital. There is a plethora of studies that assessed the relationship between human capital and employment/unemployment in various countries and to some extent, SSA. There is however, paucity of studies that examined the disaggregated expenditure on education (primary, secondary and tertiary) and health (private and public) data vis-à-vis its effect on youth employment. This was the gap that this study filled.
This study found that human capital investment as measured by private and government health expenditures, and primary, secondary and tertiary education spending were found to have varying significant impact on youth employment in SSA. Specifically, health expenditures were found to have negative impact, whereas education expenditure was found to have positive impact. Therefore, the study concludes that human capital investment (especially investment in education) has indeed significant effect on youth employment across SSA countries.
The policy implication of the findings is that nations that desire to improve youth employment should target increasing certain levels of education investment especially primary and tertiary levels that were found to significantly impact youth employment than on the secondary education segment. The plausible reason for the inverse relationship between health expenditure and youth employment could be that these youths are the stage of their physiological development where health burden is light and by extension reduced health spending. The health investments made at the stage of infancy seem to support robust health status during the youthful period. The implication however is not to discourage health spending at this stage, but to increase health spending at the earlier stages of human development (prenatal, neonatal and infancy levels) since it yields health standing that will attract reduced spending at the youthful stage.
Disclosure statement
No potential conflict of interest was reported by the authors.
Additional information
Funding
Notes on contributors
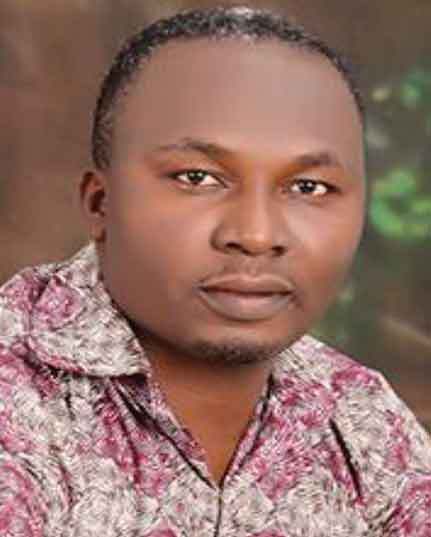
Oluchukwu F Anowor
Oluchukwu ANOWOR is a professional Economist; highly-motivated, service oriented, proactive and enterprising with excellent team skills. He is interested in sustainable future with expertise in Development Economics, Microeconomics and Macroeconomics. Oluchukwu Anowor is knowledgeable in the current industry trends as well as proficiency in analytical skills with proven ability to meet tight deadlines. He is a consultant, policy analyst, and has been enjoying a sound progressive profile in research.
Hyacinth E Ichoku
Hyacinth ICHOKU is a Professor of Economics and currently the Vice Chancellor of Veritas University Abuja, Nigeria. He is a Development Finance Economist with special interest in Health Financing.
Vincent A Onodugo
Vincent ONODUGO is a Professor at Department of Management, University of Nigeria with special interest in Human and Organization Development. He has been consulting and researching in the areas of human capital sourcing, social and public institutions among others
Chinedu Ochinanwata
Chinedu OCHINANWATA holds a Ph.D in Digital Economy and has been consulting and researching for various international organizations. He is also a Research Fellow at University of Lincoln, UK.
Peter Chika Uzomba
Peter UZOMBA is an expert and consultant in the field of Economics, lecturing at Department of Economics, Federal University Lokoja, Nigeria.
References
- Agbarakwe, H. U., & Anowor, O. F., & Ikue John. (2018). Foreign resources and economic growth in English speaking ECOWAS countries. Opción (Universidad del Zulia, Venezuela), 34 (14), 117–16. http://produccioncientificaluz.org/index.php/opcion/article/view/23928
- Aghion, P., & Howitt, P. (1992). A model of growth through creative destruction. Econometrica, 60(2), 323–351. https://doi.org/10.2307/2951599
- Aghion, P., & Howitt, P. (1997). Endogenous Growth Theory. MIT press. https://mitpress.mit.edu/books/endogenous-growth-theory
- Agrawal, M., Khandelwal, E., Tiwari, T., Parvez, Z., Jain, A., Kaur, M., Dhir, R., Gour, A., Sharma, R., & Kumar, S. (2015). Global Human Capital Trends 2015, Leading in the new world of work.Deloltte. University Press.
- Alawamleh, M., et al, Bani Ismail, L., & Aqeel, D., et al. (2019). The bilateral relationship between human capital investment and innovation in Jordan. J Innov Entrep, 8(6). https://doi.org/10.1186/s13731-019-0101-3.
- Aleksynska, M., & Schindler, M. (2011). Labor market institutions in advanced and developing countries: A New Panel Database. IMF Working Paper No. 11/154. International Monetary Fund.
- Anowor, O. F., Ichoku, H. E., Onodugo, V. A., & McMillan, D. (2020). Nexus between healthcare financing and output per capita: Analysis of countries in ECOWAS sub-region. Cogent Economics & Finance, 8(1), 1832729. https://doi.org/10.1080/23322039.2020.1832729
- Anowor, O. F., Uwakwe, Q. C., & Chikwendu, N. F. (2019). How investment does affect unemployment in a developing economy. Sumerianz Journal of Economics and Finance, 2(7), 82–88. https://www.sumerianz.com/pdf-files/sjef2(7)82-88.pdf
- Anyanwu, S. O. (1998). The human factor and economic development in Africa. In V. G. Chivaura & C. G. Mararike (Eds.), The human factor approach to development in Africa (pp. 66–76). Harare: UZ Publications.
- Armah, S., & Nelson, C., (2008). Is foreign aid beneficial for Sub-Saharan Africa? A panel data analysis. American Agricultural Economics Association Annual Meeting. July 27-29. 1–37. llinois: University of Illinois at Urbana-Champaign.
- Ashley, R., & Sun, X. (2016). Subset-Continuous-Updating GMM Estimators for Dynamic Panel Data Models. Econometrics, 4. 47. https://doi.org/10.3390/econometrics4040047
- Assaad, R., & Levison, D. (2013). Employment for Youth – A Growing Challenge for the Global Community. In Commissioned Paper for the High-Level Panel on Post-2015. UN MDG Development Agenda Employment and Economic Growth. Working Paper No. 2013-07 https://doi.org/10.18128/MPC2013-07
- Atif, A. (2020). From school to employment; the dilemma of youth in Sub–Saharan Africa. International Journal of Adolescence and Youth, 25(1), 945–964. https://doi.org/10.1080/02673843.2020.1778492
- Baah-Boateng, W. (2015). Unemployment in Africa: How appropriate is the global definition and measurement for policy purpose. International Journal of Manpower, 36(5), 650–667. https://doi.org/10.1108/IJM-02-2014-0047
- Barford, A., & Cieslik, K. 2019. Making A Life: A Youth Employment Agenda. https://www.repository.cam.ac.uk/handle/1810/294839
- Barford, A., Coombe, R., & Proefke, R. (2021). Against the Odds: Young people’s high aspirations and societal contributions amid a decent work shortage. Geoforum, 121, 162–172. https://doi.org/10.1016/j.geoforum.2021.02.011
- Barro, R. J. (1991). Economic growth in a cross section of countries. The Quarterly Journal of Economics, 106(2), 407–443. https://doi.org/10.2307/2937943
- Barro, R. J. (2001). Human Capital and Growth. The American Economic Review, 91(2), 12–17. https://doi.org/10.1257/aer.91.2.12
- Bartelsman, E., Haltiwanger, J., & Scarpetta, S. (2004). Microeconomic evidence of creative destruction in industrial and developing countries. Mimeo, University of Maryland.
- Becker, G. S. (1962). Investment in Human Capital: A Theoretical Analysis. Journal of Political Economy, 70(5), 9–49. https://doi.org/10.1086/258724
- Becker, G. (1964a). Human capital. Colombia University Press for the National Bureau of Economic Research.
- Becker, G. S. (1964b). Human Capital: A theoretical and empirical analysis, with Special Reference to Education. University of Chicago Press. https://ssrn.com/abstract=1496221
- Bun, M. J. G., & Windmeijer, F. (2010). The weak instrument problem of the system GMM estimator in dynamic panel data models. Econometrics Journal, 13(1), 95–126. https://doi.org/10.1111/j.1368-423X.2009.00299.x
- Caballero, R., & Hammour, M. (1996). On the timing and efficiency of creative destruction. Quarterly Journal of Economics, 111(3), 805–852. https://doi.org/10.2307/2946673
- Danquah, M., & Ouattara, B. (2014). Productivity growth, human capital and distance to frontier in Sub-Saharan Africa. Journal of Economic Development, 39(4), 4–24. https://doi.org/10.35866/caujed.2014.39.4.002
- Eme, O. I., Uche, O. A., & Uche, I. B. (2014). Building a solid health care system in Nigeria: Challenges and prospects. Academic Journal of Interdisciplinary Studies, 3(6), 501–510.
- Engelbrecht, H.-J. (2002). Human capital and international knowledge spillovers in TFP growth of a sample of developing countries: An exploration of alternative approaches. Applied Economics, 34(7), 831–841. https://doi.org/10.1080/00036840110061947
- Fashoyin, T., & Tiraboschi, M. (2011). Productivity, Investment in Human Capital and the Challenge of Youth Employment. In P. Manzella & L. Rustico (Eds.), Newcastle upon Tyne, NE6 2XX, UK. Cambridge Scholars Publishing, 12 Back Chapman Street.
- Gabriela, P., & Mircea, B. (2014). Stochastic dynamic model on the consumption – saving decision for adjusting products and services supply according with consumers` Attainability. Academy of Economic Studies - Bucharest, Romania. The Amfiteatru Economic Journal. 16. 35.
- Hammed, O. M., Agboola, H. Y., & Kafilah, L. G. (2019). Endogenous specification of foreign capital inflows, human capital development and economic growth: A study of pool mean group. International Journal of Social Economics. https://doi.org/10.1108/IJSE-04-2018-0168
- Hayakawa, K. (2009). On the effect of mean-nonstationarity in dynamic panel data models. Journal of Econometrics, 153(2), 133–135. https://doi.org/10.1016/j.jeconom.2009.04.008
- International Labour Organisation. (2010). Global Employment Trends for Youth. August Special Issue on the Impact of the Global Economic Crisis on Youth. International Labour Organisation (ILO).
- International Labour Organization. (2011). Global Employment Trends for Youth. International Labour Organisation.
- International Labour Organization (ILO). (2019). World Employment and Social Outlook: Trends 2019. International Labour Organisation.
- International Labour Organization (ILO). 2020. Report on employment in Africa (Re-Africa) – Tackling the youth employment challenge – International Labour Office –.
- Islam, N. (1995). Growth empirics: a panel data approach. The Quarterly Journal of Economics, 110(4), 1127–1170. https://doi.org/10.2307/2946651
- Kwon, D.-B. Human capital and its measurement. The 3rd OECD world forum on “Statistics, Knowledge and Policy” Charting Progress, Building Visions, Improving Life. 1–36. 27-30October. (2009) OECD.
- Lee, J.-W. (2003), “Economic Growth and Human Development in the Republic of Korea, 1945-1992”, Occasional Paper 24
- Levine, L. (2011). Implications of the Anti-Poverty Program for Education and Employment, Vocational. Guidance Quarterly, 14(1), 8–18. https://doi.org/10.1002/j.2164-585X.1965.tb00763.x
- Lucas, R. (1988). On the mechanics of economic development. Journal of Monetary Economics, 22(1), 3–42. https://doi.org/10.1016/0304-3932(88)90168-7
- Mallick, S., & Moore, T. (2006). Foreign Capital in a Growth Model. In European Economics and Finance Society5th Annual Meeting of the European Economics and and Finance Society (EEFS) (pp. 1–3). European Economics and Finance Society (EEFS).
- Mankiw, G., Romer, P., & Weil, D. (1992). A contribution to the empirics of economic growth. Quarterly Journal of Economics, 107(2), 407–437. https://doi.org/10.2307/2118477
- McDonald, S., & Roberts, J. (2002). Growth and multiple forms of human capital in an augmented Solow model: A panel data investigation. Economics Letters, 74(2), 271–276. https://doi.org/10.1016/S0165-1765(01)00539-0
- Micklewright, J., Pearson, M., & Smith, S. (1990). Unemployment and Early Leaving. Economic Journal, 100(400), 163. https://doi.org/10.2307/2234193
- Mincer, J. (1996). Economic development, growth of human capital, and the dynamics of the wage structure. Journal of Economic Growth, 1(1), 29–48. https://doi.org/10.1007/BF00163341
- Mueller, B. (2019). Rural youth employment in sub-Saharan Africa: Moving away from urban myths and towards structural policy solutions, mimeo.
- Odior, E. S. O. (2014). Government expenditure on education and poverty reduction: Implications for achieving the MDGs in Nigeria, a computable general equilibrium micro-simulation analysis. Asian Economic and Financial Review, 4(2), 150–172.
- Okun, A. M. (1962). Potential GNP, its measurement and significance. Cowles Foundation, Yale University.
- Olaniyan, D., & Okemakinde, T. (2008). Human Capital Theory: Implications for Educational Development. European Journal of Scientific Research, 24(2), 157–162. https://doi.org/pjssci.2008.479.483
- Oluwatobi, S. O., & Ogunrinola, O. (2011). Government expenditure on human capital development: implications for economic growth in Nigeria. Journal of Sustainable Development, 4(3), 72–80. https://doi.org/10.5539/jsd.v4n3p72
- Onodugo, V. A., Kalu, I. E., & Anowor, O. F. (2013). An empirical analysis of the impact of investment in human capital on Nigerian economy. PARIPEX– Indian Journal of Research, 2(4), 336–339. https://www.worldwidejournals.com/paripex/file.php?val=April_2013_1366124026_945cb_114.pdf
- Onodugo, V. A., Obi, K. O., Anowor, O. F., Nwonye, N. G., & Ofoegbu, G. N. (2017). Does public spending affect unemployment in an emerging market? Risk Governance & Control: Financial Markets & Institutions, 7(1), 32–40. http://dx.doi.org/10.22495/rgcv7i1art4
- Persyn, D., & Westerlund, J. (2008). Error correction based cointegration tests for panel data. Stata Journal, 8(2), 232–241. https://doi.org/10.1177/1536867X080080
- Pesaran, M. H. (2007). A pair-wise approach to testing for output and growth convergence. Journal of Econometrics, 138(1), 312–355. https://doi.org/10.1016/j.jeconom.2006.05.024
- Rebelo, S. T. (1991). Long-run policy analysis and long-run growth. Journal of Political Economy, 99(3), 500–521. https://doi.org/10.1086/261764
- Rees, D. I., & Mocan, N. H. (1997). Labour market conditions and the high school dropout: Evidence from New York State. Economics of Education Review, 16(2), 103–109. https://doi.org/10.1016/S0272-7757(96)00037-4
- Romer, P. (1986). Increasing returns and long-run growth. Journal of Political Economy, 94(5), 1002–1037. https://doi.org/10.1086/261420
- Romer, P. (1990). Endogenous technological change. Journal of Political Economy, 98(5), 71–102. https://doi.org/10.1086/261725
- Schultz, T. W. (1961). Education and Economic Growth. In N. Henry (Ed.), Social Forces Influencing American Education (pp. 1–12). University of Chicago Press.
- Schultz, T. W. (1999). Health and school investment in Africa. Journal of Economic Growth, 13(1), 67–88. https://doi.org/10.1257/jep.13.3.67
- Segerstrom, P., Anant, T. C., & Dinopoulos, E. (1990). A schumpeterian model of the product life cycle. American Economic Review, 80, 1077–1091.
- Soares, R. R. (2014). Gary Becker’s contributions in health economics. In IZA Discussion Paper No. 8586. The Institute for the Study of Labor.
- Solow, R. M. (1956). A contribution to the theory of economic growth. The Quarterly Journal of Economics, 70(1), 65–94. https://doi.org/10.2307/1884513
- Swan, T. W. (1956). Economic growth and capital accumulation. Economic Record, 32(2), 334–361. https://doi.org/10.1111/j.1475-4932.1956.tb00434.x
- Sweeten, G., Bushway, S. D., & Paternoster, R. (2009). Does dropping out of school mean dropping into delinquency? American Society of Criminology, 47(1), 47–91. https://doi.org/10.1111/j.1745-9125.2009.00139.x
- Tacoli, C., McGranahan, G., & Satterthwaite, D. (2015). Urbanization, Rural–urban Migration and Urban Poverty. Human Settlements Group International Institute for Environment and Development_WORLD MIGRATION REPORT 2015.
- Wagner, A. (1890). Finanzwissenschaft. C. F. Winter.
- Westerlund, J. (2007). Testing for error correction in panel data. Oxford Bulletin of Economics and Statistics, 69(6), 709–748. https://doi.org/10.1111/j.1468-0084.2007.00477.x
- Whitefield, A., Wilson, F., & Dowell, J. (1991). A framework for human factors evaluation. Behaviour & Information Technology, 10(1), 65–79. https://doi.org/10.1080/01449299108924272