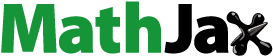
Abstract
The United Nations Sustainable Development Goals (SDGs) 7, 11, and 12 are all aimed at advancing green energy consumption in the fight against the three planetary crises facing the world today: climate change, biodiversity loss, and pollution. Besides, Africa has an abundance of natural resources, yet, the continent continues to witness slow growth and development compared to its counterparts. Therefore, this study, the first of its kind, simultaneously assesses the impact of green energy consumption and natural resources rents on economic growth by applying the dynamic panel ARDL and the Feasible Generalized Least Square (FGLS) estimators on data from 1990 to 2020 for 24 selected African countries. The results show that green energy consumption has a short-run growth-limiting and a long-run growth-enhancing effect in Africa. Also, CO2 emissions have both short- and long-run significant positive impacts on economic growth, while fossil fuel combustion negatively impacts growth both in the short and long run, albeit the effect is not significant in the long run. Similarly, regarding Africa’s natural resource rents’ impact on growth, the results show that total natural resource rents are growth-enhancing in the short run and growth-limiting in the long run. Additionally, both forest and mineral rents have a significant short-run negative impact on growth in Africa. However, in the long run, only the effect of mineral rent is growth-enhancing although generally not statistically significant. These findings provide relevant implications for policy shifts to enhance environmental sustainability, achieve sustainable economic growth, and ensure proper natural resources management in Africa.
1. Introduction
The continuous increase in atmospheric greenhouse gas emissions due to nonrenewable energy consumption has resulted in three planetary crises: climate change, biodiversity loss, and pollution. Non-renewable energy consumption has further resulted in about 99% of the world’s urban population inhaling polluted air (United Nations, Citation2022). Additionally, Aydoğan and Vardar (Citation2020) contend that the burning of fossil fuels (usually coal and natural gas) stimulates economic growth, but at the same time poses serious consequences to our planet and the reduction of its use is vital for the planet’s welfare. Consequently, this study argues that switching from the usage of non-renewable energy sources to renewable energy in developing countries may most likely lead to lower growth and development in the short run. However, in the medium-to-the long-term, its impact on growth and development will become positive and more sustainable as greenhouse gas emissions will diminish.
Moreover, according to the (United Nations, Citation2022) Sustainable Development Goals report, renewable energy consumption has increased by a quarter between 2010 and 2019. However, the total share of renewable energy consumption is only 17.7% of the total final energy consumption (United Nations, Citation2022). Similarly, the British Petroleum (Citation2022) projected that the global share of renewable energy is expected to increase from 35% in 2019 to about 65% by 2050. The significance being placed on renewable energy consumption as a means to an end and not an end in itself is a result of the tripartite planetary crises faced by the world today. Renewable energy enhances environmental sustainability by lowering CO2 emissions and other greenhouse gases in the atmosphere (Alola et al., Citation2022; Ayanlade et al., Citation2022; Ibrahim et al., Citation2022; Khan et al., Citation2022; Kirikkaleli & Adebayo, Citation2022), and this will by far counteract extreme heating, global warming, and other serious climatic conditions threatening human survival and labor productivity in general.
Besides, issues regarding Africa’s natural resource curse have caused a stir in the growth and development literature for some time now and there is no sign that it will die out. Arguably, Africa is estimated to house the largest amount of the earth’s natural resources. For instance, the African continent has an aquaculture sector approximated at USD 24 billion and is home to the second-largest tropical forest. Additionally, 64% of Africa’s land size as represented is allocated to its 63 international rivers, which contain 93% of the total surface water resources. In the mineral sector, the region accounts for roughly 30% of all global minerals reserves while oil reserves and natural gas stand at 8% and 7% of the world’s reserves, respectively (AfDB, Citation2015). Paradoxically, the growth and employment elasticities of such resource abundance are nothing to write home about and most of the continent’s economies continue to experience high levels of poverty, rising income inequality, low levels of growth, and underdevelopment.
The abundant natural resource and development paradox in Africa is widely referred to as the curse of natural resources (Ari & Auty, Citation2016). The most common explanation given for this quagmire originates from the Dutch disease in which it is stated that national integration of commodity rents, creates vulnerabilities in the economy such as negative trade shocks and dwindling composition of non-mining commodities tradable in GDP (Sachs & Warner, Citation1995). For example, minerals alone account for about 70% of the total African exports but their contribution to the Gross Domestic Product of the African economies is only 28% (AfDB, Citation2015). This is an indication of poor natural resource management, from exploitation, extraction, marketing, and rents. Against this backdrop, this study explores the real impact of Africa’s natural resource rents on the growth of their economies.
Furthermore, previous empirical studies conducted to examine the nexus between renewable energy and economic growth show mixed and inconclusive results. A few studies found that renewable energy contributes positively and significantly to economic growth (see, for example, Kahia et al., Citation2019; Magazzino et al., Citation2022; Pao & Fu, Citation2013). On the other hand, Abbasi et al. (Citation2020) found a negative relationship between renewable energy and economic growth. Likewise, Apergis et al. (Citation2010) found no link between economic growth and renewable energy consumption. In the same vein, the relationship between natural resource rent and economic growth remained mixed and inclusive. For example, empirical studies (Awosusi et al., Citation2022; Mehar et al., Citation2018) reported a positive and statistically significant relationship between natural resource rents and economic growth. On the contrary, Adabor et al. (Citation2020) found a negative relationship between natural resource rents and economic growth.
This study contributes to the existing literature by jointly analyzing the impact of green energy and/or renewable energy consumption and Africa’s natural resource rents on the economic growth of African economies. The study also contributes to the existing literature by checking for robustness and accounting for cross-sectional (and temporal) dependence among the residuals through the adoption of the Feasible Generalized Least Square (FGLS) estimator. In addition, to ascertain the long-run impact of green energy consumption and natural resources rents on economic growth in Africa, the study further utilizes the Dynamic panel Autoregressive Distributed Lag (ARDL) estimators of the Pooled Mean Group (PMG) and the Mean Group (MG) estimators. Pesaran and Smith (Citation1995) posited that in panel data estimation, aggregation can lead to biased estimates especially when the slope coefficients vary across the cross-sectional units. Besides, Hoechle (Citation2007) postulated that intentionally ignoring cross-sectional (and temporal) dependence which most likely exists in panel data estimations can lead to spurious regression results.
The rest of the paper is organized as follows: the second section provides a review of the related empirical background in the green energy-vis-à-vis-natural resource rents and economic growth nexus. The third section discusses the methods, variables, and data sources. In addition, the fourth Section discusses the estimation techniques, while the fifth section presents the results. Similarly, section six outlines the discussion of results, and the last and final section seven highlights the conclusion and policy directions.
2. Literature review
This section presents the theoretical foundation of the study and further highlights previous empirical discussions on green energy, natural resource rents, and economic growth nexus both within and outside Africa.
2.1. Theoretical review
The theoretical foundation underpinning this study rest within the ambit of the environmental Kuznets curve, and the Jevons paradox.
2.1.1. Environmental kuznets curve hypothesis
The environmental Kuznets curve (EKF) hypothesis was popularized by Simon Kuznets in the 1950s. Dasgupta et al. (Citation2002) opined that initially, Kuznets used it to explain the link between income inequality and development until Grossman and Krueger modified it to explain the link between environmental pollution and development in 1993. The relationship between environmental degradation and per-capita income is explained by the shape of an Inverted “U” (Pasten & Figueroa, Citation2012). EKF links the consumption of renewable energy to economic growth in the sense that structural changes in the economy’s early stages of development permit the emission of greenhouse gases into the atmosphere. However, after a certain level of GDP per capita, the economy will adopt environmental-friendly technologies and this will result in a significant reduction of greenhouse gas emissions in the economy. Critics of the environmental Kuznets curve argue that it just gives a small picture of a multidimensional process. Over time, the curve will go beyond the maximum points of pollution, as the earth will become more polluted due to the ever-increasing integration among countries around the world (Dasgupta et al., Citation2002).
2.1.2. The Jevons paradox
The Jevons paradox was propounded by William Stanley Jevons in the 19th century in his book titled the Coal Question. Jevons explicitly states that there is a positive relationship between coal consumption, fossil energy resources, and increased efficiency (Polimeni, Citation2007). The Jevons paradox presupposes that technological improvements will lead to improved efficiency of the resource being used and more and more units of the resource will be used. Furthermore, environmental impact, population, and technical progress are directly proportional to the consumption of natural resources. This theory links the consumption of energy and natural resources to economic growth in the sense that, increased efficiency paradoxically leads to increased overall energy consumption and in turn creates an economic boom (Jeff Dardozzi, Citation2020). Therefore, the Jevons paradox has important implications, not only for energy consumption but also for the consumption of natural resources.
2.2. Empirical review
In this section, we present a review of past empirical literature in the domain of green energy consumption, natural resource rents, and economic growth nexus.
2.2.1. Renewable energy consumption and economic growth
The relationship between renewable energy consumption and economic growth comes with mixed results and submissions. For instance, Pearson (Citation2021) examines the impact of renewable energy consumption on economic growth in Croatia using time series data from 1996 to 2011. The study employed the Autoregressive Distributed Lag (ARDL) model and demonstrated that renewable energy consumption positively impacts economic growth in both the short and long run. In a related study, Qudrat-Ullah and Nevo (Citation2021) using panel data, for 37 African countries, and employing the system Generalized Method of Moments (GMM) found that renewable energy adoption and development will lead to an increase in economic growth in Africa, both in the long and short run. On the contrary, Dogan and Ozturk (Citation2017) examined the impact of real income (GDP), renewable energy consumption, and non-renewable energy consumption on carbon dioxide (CO2) emissions for the United States of America (USA) in the environmental Kuznets curve (EKC) model for the period 1980 to 2014 employing Autoregressive Distributed Lag (ARDL) model. The findings reveal that in the presence of a structural break, CO2 emissions, real income, quadratic real income, and renewable and non-renewable energy consumption are integrated. Further, results from long-run estimates indicate that increases in renewable energy consumption mitigate environmental degradation, whereas increases in non-renewable energy consumption contribute to CO2 emissions. In addition, the EKC hypothesis was found not to be valid for the USA.
Moreover, Maji et al. (Citation2019) conducted a study using a sample of 15 West African countries and utilizing the panel Dynamic Ordinary Least Squares (DOLS) on data between 1995 and 2014. The findings show that renewable energy consumption reduces economic growth in West African countries. Similarly, Maji (Citation2015) using the Autoregressive Distributed Lag (ARDL) model on data from 1971 to 2011 in Nigeria reported a significant negative relationship between two indicators of clean energy (alternative nuclear energy and electric power consumption) and economic growth. On the contrary, Riti et al. (Citation2022) carried out a similar study in a panel setting in sub-Saharan Africa by applying the Autoregressive Distributed Lag (ARDL) model to data from 1990 to 2018. The study reveals that there exists a positive and statistically significant relationship between renewable energy consumption and economic growth in the long run. In the same vein, Riti et al. (Citation2022) further show that the environmental pillar of sustainability results show that real GDP and real gross-fixed capital formation exert positive and significant impacts on emissions of greenhouse gasses (GHG) while renewable energy exerts a negative and significant impact on emissions of GHG.
Last but not least, Apergis and Payne (Citation2012) documented the electricity consumption nexus in Central America within a panel error correction model framework. The findings indicate a long-run equilibrium relationship between real gross domestic product, renewable electricity consumption, non-renewable electricity consumption, real gross-fixed capital formation, and the labor force. Additionally, except for renewable electricity consumption, the respective long-run coefficient estimates were found to be positive and statistically significant. Also, the panel error correction model results show a unidirectional causality from renewable electricity consumption to economic growth in the short-run, but bidirectional causality in the long run. The results further indicate bidirectional causality between non-renewable electricity consumption and economic growth in both the short and long run.
2.3. Non-renewable energy consumption and economic growth
The nexus between non-renewable energy consumption and economic growth comes as mixed and remains inconclusive. For example, Borhan et al. (Citation2012) investigate the impact of Co2 on economic growth in 8 ASEAN countries between 1965 and 2010. The study used three equations simultaneous models with the pollution indicator as emissions CO2. The findings reveal that the Environmental Kuznets Curve relationship was found. Ivanovski et al. (Citation2021) also examined the link between renewable energy consumption, non-renewable energy consumption, and economic growth by applying the Least Squares Dummy Variable estimation (LSDV) method on data from 1990 to 2015 across a panel comprising OECD and non-OECD countries. The findings show that non-renewable energy consumption exerts a positive and statistically significant impact on economic growth across OECD nations. However, the contribution of renewable energy consumption to economic growth is statistically not significant in these countries for most of the study.
In addition to the above, Brooks (Citation2011) analyzes the nexus between economic development and carbon dioxide emissions using global panel data. The findings indicated that there is a diminishing marginal propensity to emit (MPE) carbon dioxide as GDP per capita rises. The study contended that global carbon dioxide emissions growth is not sensitive to average output growth. However, the study found that output and population growth stimulate CO2 emissions in lower-income nations and the propensity to emit is high (high MPEs).
Similarly, Mikayilov et al. (Citation2018) examined the link between economic growth and CO2 emissions in Azerbaijan. The study employed the cointegration analysis of data over the period 1992–2013. The results from the different cointegration methods were consistent with each other and show that economic growth has a positive and statistically significant impact on CO2 emissions, in the long run, implying that the Environmental Kuznets curve hypothesis does not hold for Azerbaijan.
In a related study, Sannassee, (Citation2015) investigated the nexus between Mauritius’s carbon emissions and economic growth by estimating the Environmental Kuznets Curve (EKC) for the period 1975–2009. The results of the study demonstrated that the carbon dioxide emissions trajectory is closely related to the GDP time path. Furthermore, the results show that emissions elasticity to income growth has been increasing over time and failed to prove the existence of a reasonable turning point. Thus, no EKC “U” shape was obtained. Lastly, Chen and Huang (Citation2013) conducted a study to assess the relationship between carbon dioxide (CO2) emission per capita and economic growth for N-11 countries between 1981 and 2009. The study adopted the cointegration approach in a heterogeneous panel. Results from the study verified that there is a positive long-run relationship between CO2 emissions, Electric power consumption, Energy use, and GDP. Likewise, they show that there exists bi-directional causality between CO2 emission and electric power consumption.
2.4. Natural resource rents and economic growth
The relationship between renewable energy and economic growth in the empirical literature also revealed mixed and inconclusive results. Adabor et al. (Citation2020) examined the effect of oil and gas resource rent on the economic growth of Ghana between 2007 and 2019 by using the Autoregressive Distributed Lag (ARDL) time series econometric model. The findings show that oil resource rent had a negative and statistically significant relationship with the economic growth of Ghana. However, gas resource rent was found to have a positive impact on the economic growth of Ghana.
In a similar study, Mohamed (Citation2020) analyzed the link between natural resource rents, human development, and economic growth in Sudan by applying the cointegration and vector error correction modeling (VECM) to data between 1970 and 2015. The results confirm the existence of a long-run equilibrium relationship between natural resource rents, human development, and economic growth in Sudan. Additionally, the results from the VECM show that economic growth is positively affected by natural resource rents and development expenditure. However, it was found to be negatively affected by life expectancy at birth in the short run. Also, natural resource rents, school enrolment, life expectancy, and financial development were observed to be inversely related to economic growth.
Furthermore, Mehar et al. (Citation2018) examined the nexus between total natural resources rent and economic growth in Pakistan and India between 1970 and 2017. The study employed cointegration and VECM for the estimations. The study observed that total natural resources rents have a positive and statistically significant effect on Pakistan’s and India’s GDP per capita. The results from the cointegration analysis depicted that there are two co-integrated equations of the relationship between the variables.
Moreover, Ofori et al. (Citation2021) established a link between oil rent fluctuations and remittances received in the economy for a sample of 43 sub-Saharan African (SSA) countries between 1990 and 2017. They used the Pooled Ordinary Least Squares, the Fixed Effects, the Random Effects, and the Generalized Method of Moments. The study found that forest rent exerts a positive impact on economic growth whilst oil rent and natural gas rent hurt economic growth. Likewise, a positive marginal and net effect on economic growth from the interaction between remittances and oil rent was observed.
Besides, Elbadawi and Soto (Citation2016) analyzed the impact of oil rents and political governance on economic growth in oil-rich countries. The study uses the cointegration and vector error-correction mechanisms and found that oil-rich countries with low levels of governance scores are likely to experience the curse, while those with high enough levels may turn resource rents into a driver of growth. The study further indicated that countries with high scores on only one dimension may avoid the curse but are not likely to effectively use resource rents to promote growth.
Ousama et al. (Citation2021) examined the causal relationship between total natural resource rents and economic growth in a sample of top resource-abundant countries over the period 1970–2013. The study used the Pooled Mean Group (PMG) estimator for the analysis. Evidence from the study supports the existence of the natural resource blessed hypothesis in the long run, while no such evidence is confirmed in the short run. Similarly, Dramani et al. (Citation2022), investigated the threshold effects of natural resource dependence on economic growth in sub-Saharan Africa (SSA) using both aggregate and disaggregate data from 1990 to 2019 by employing a threshold effect model. Their findings show a double threshold effect of natural resource rent on economic growth. Thus, below 6% of GDP, aggregate natural resource rent exerts a significant negative effect on economic growth. However, as the rents increase above 6% to about 15% of GDP its negative effect on economic growth significantly reduces. In addition, beyond 15% of GDP, total natural resource rent exhibits a substantial significant positive impact on economic growth. Furthermore, the results indicate that forest rents exhibit a strong weighty adverse effect on economic growth at all levels of thresholds, but the negative effects of mineral rents become positive beyond 15% of GDP.
In a related study, Tang, Ma, et al (Citation2022) examined the financial resource curse hypothesis by scrutinizing the linkages between financial development and natural resources rent from 1984 to 2018. Empirical results validate the financial resource curse hypothesis. Moreover, the results suggest that business regulations stimulate financial development and neutralize the negative effects of natural resources on financial development. Similarly, Muhamed et al. (Citation2021) employed the quantile-on-quantile regression in the top five selected Asian economies which have the most natural resources in the region. The findings reveal that natural resources have a positive and significant impact on economic growth in all countries, except India. The findings, thus, confirm that the effect of natural resources on economic growth is negative and significant in the Indian economy.
Moreover, Christa (Citation2008) utilizes both OLS and 2SLS regressions to investigate the effects of natural resource abundance on economic growth using new measures of resource endowment and considering the role of institutional quality. The findings indicate a direct positive relationship between natural resource abundance and economic growth the positive resource effects are particularly strong for sub-soil wealth. Furthermore, no evidence of negative indirect effects of natural resources through the institutional channel is found. Finally, Sharma and Paramati (Citation2022), investigated the association between natural capital and economic development using panel data comprising a large number of countries across the world for the period 1995–2018 utilizing the GMM estimation technique. The study rejects the resource curse hypothesis and supports the resource blessing hypothesis.
Given the review of the existing literature on green energy consumption, and natural resource rents vis-à-vis economic growth nexus, the current study contributes to the literature by simultaneously analyzing the impacts of the two important concepts on economic growth by using disaggregated data on both concepts to check for the robustness of the results for relevant policy perspectives. The study also contributes to the existing literature by accounting for cross-section (and temporal) dependence through the application of the Feasible Generalized Least Squares (FGLS) estimator.
3. Methods, variables, and data sources
This study employs the Dynamic Autoregressive Distributed Lag (ARDL) panel models of the Pooled Mean Group (PMG) and the Mean Group (MG) estimators together with the Feasible Generalized Least Square (FGLS) estimator on data spanning the period 1990–2020 for 24 selected African countries to investigate the impact of green energy (renewable energy) usage and Africa’s natural resource rents on economic growth. The dependent variable in this study is the Gross Domestic Product of the various countries. The primary independent variables on the other hand include green energy consumption and Africa’s natural resource rents.
The gross domestic product (GDP) has been a standard measure of economic growth, and higher GDP can be achieved through increasing production, industrialization, and manufacturing sectors. This presupposes that increased economic activities are associated with greater usage of energy and eventually higher pollution of the environment through CO2 emissions resulting from the consumption of nonrenewable energy sources (Borhan et al., Citation2012). Therefore, we used CO2 emissions and fossil fuel combustions as proxy variables for nonrenewable energy consumption, and we expect their effects on growth to either be positive or negative since they are harmful to human health and survival. Renewable energy sources such as solar, biomass, wind, geothermal, and hydropower are essential to environmental sustainability as they are capable of reducing the amount of CO2 emissions and other greenhouse gasses which are responsible for global warming, sea level rise, and other extreme climatic conditions (N. Apergis & Payne, Citation2012; Riti et al., Citation2022). Hence, we used renewable energy proxy for green energy and we expect it to have a short-run negative and a long-run positive impact on growth.
Additionally, natural resource rents such as mineral rents, forest rents, and total natural resource rents make significant contributions to the gross domestic product (Mehar et al., Citation2018). A priori, we expect the impact of natural resource rents on growth to be positive. The control variables used in this study are gross fixed capital formation, inflation, and trade openness. These variables are included to take care of their confounding effects on growth in Africa. We expect trade openness and gross fixed capital formation to have a positive impact on growth while inflation is expected to either stimulate or hurt economic growth.
Table gives the summary of all the variables, their data sources, and their expected signs with economic growth in Africa. Similarly, all the countries selected for this study are listed in Table .
Table 1. Variables description, data sources, and expected signs
4. Techniques of estimations
The primary objectives of this study are to investigate the impact of green energy usage and Africa’s natural resource rents on economic growth. As such, the current study carefully selects the three estimation techniques based on their relevance to the study’s objectives and their peculiar characteristics. The Dynamic Autoregressive Distributed Lag (ARDL) panel models of the Pooled Mean Group (PMG) and the Mean Group (MG) estimators allows us to estimate both the short and long-run impacts of the key variables of interest on growth in Africa. In panel data estimation, aggregation can lead to biased estimates especially when the slope coefficients vary across the cross-sectional units (Pesaran & Smith, Citation1995). One plausible way to resolve this problem is to use an estimator based on the so-called “Mean Group” method which estimates the short-run coefficients for each country during the period and then calculates the average of the estimated coefficients. This model assumes long-run homogeneity of the slope coefficients across panels. However, the MG method only gives consistent results if the dimension of the panel tends toward infinity.
Another option is to use an estimator based on the so-called “Pooled Mean Group” method. In this case, the long-term coefficients are uniform but the short-term coefficients are allowed to be heterogenous across panels. This method makes it possible to establish a distinction between short-term dynamics and long-term dynamics and takes into account the heterogeneity of the countries. The method also resolves the endogeneity problem in a dynamic specification (Pesaran et al., Citation1999). Moreover, the validity, consistency, and efficiency of the estimates from the MG and the PMG depend on the following conditions: i) The existence of the long-term relationship between the dependent and the independent variables requires that the coefficient of the error correction term be negative and not lower than −2; ii) The residual resulting from the error correction model is serially uncorrelated and the explanatory variables can be treated as exogenous. This condition can be satisfied by including the lags p and q (p for the dependent variable and q for the independent variable) in the error correction model; and finally, iii) The time dimension (T) should be greater than the panel dimension (N), which makes it possible to avoid the bias in the average of the estimators and solve the problem of heterogeneity.
In the words of (Johansen, Citation1995; Phillips & Hansen, Citation1990), the existence of a long-run relationship among the variables is only valid when the variables are integrated in the same order. However, Pesaran et al. (Citation1999) argued that panel ARDL can be used even if the variables are integrated with different orders. Thus, irrespective of whether they are integrated of I (0) or I (1) or a mixture of both. The generic specification of the panel ARDL model is given in model 1.
Where yit is the dependent variable for country i at time t, Xit is the vector of independent variables for country i at time t.
The operational form of the Dynamic panel ARDL estimations of the MG and the PMG model in Equationequation 1(1)
(1) are reparametrize in models 2 and 3 where Equationequation 2
(2)
(2) denotes the causal relationship between green energy including other control variables and growth and Equationequation 3
(3)
(3) shows the causal relationship between Africa’s natural resource rents including other control variables and growth.
Where is the log of GDP for each country at time t and
,
,
,
,
, and
are the independent variables involving renewable energy consumption, carbon dioxide emissions, fossil fuel energy consumption, gross fixed capital formation, inflation, and trade openness for country i at time t. Additionally, p and q are the optimal lag lengths for the dependent and the independent variables, respectively.
is the cross-country heterogeneity and
is the panel idiosyncratic error term.
Where is the log of GDP for each country at time t and
,
,
,
,
, and
are the independent variables involving forest rents, mineral rents, total natural resource rents, gross fixed capital formation, inflation, and trade openness for country i at time t. Moreover, p and q are the optimal lag lengths for the dependent and the independent variables, respectively.
is the cross-country heterogeneity and
is the panel idiosyncratic error term.
It is important to state that the Dynamic panel ARDL models as specified in Equationequations 2(2)
(2) and Equation3
(3)
(3) do not account for cross-sectional (and temporal) dependence among the variables across the cross-sectional units. Deliberately ignoring cross-sectional (and temporal) dependence in panel data settings can have dire consequences and may lead to spurious regression results (Hoechle, Citation2007). Therefore, after checking for cross-sectional dependence, this study further adopts the Feasible Generalized Least Square (FGLS) estimator to counteract the effect of cross-sectional (and temporal) dependence in the residuals and to check for the robustness of the estimates from the PMG and the MG estimations. The FGLS estimator was first developed by Parks (Citation1967) and later popularized by Kmenta (Citation1988). As such, it is often called the Parks or Parks-Kmenta.
The FGLS estimator could be used to estimate panels with heteroskedasticity and contemporaneously correlated error matrix (Beck, Citation2001; Hoechle, Citation2007). However, this estimator only produces efficient, consistent, and valid estimates when the time dimension (T) is larger or greater than the cross-sectional dimension (N), and therefore, the estimator is inappropriate to use with medium—and large-scale micro-econometric panels (Beck, Citation2001; Hoechle, Citation2007). Besides, the Parks-Kmenta estimator is also criticized for underestimating standard errors since it assumes that the parameters of are known, not estimated (Beck, Citation2001). Despite these criticisms, the Parks-Kmenta is still relevant in estimating panels with heteroskedasticity and contemporaneously correlated error matrix especially when the time dimension is larger, as in the case of this study (T = 30 > N = 24).
The generic form of the econometric specification of the FGLS method is given as:
Where is the dependent variable;
is a
vector of the explanatory variable;
is a
vector of coefficients of the explanatory variables;
is the intercept term; and
is the idiosyncratic error term.
The FGLS assumes that
Thus, with this assumption, the data provides T sets of residuals to estimate the contemporaneous covariance, [having typical elements
] along the block diagonal.
The operational form of the FGLS equation specified in model 4 is reparametrize to estimate the causal relationship between green energy including other control variables and growth (see model 5) and also the causal relationship between Africa’s natural resource rents including other control variables and growth (see model 6).
It should be noted that the results of the FGLS method are only based on short-run analysis and therefore, do not give any long-run implications. This study only adopted the FGLS estimator to account for cross-sectional (and temporal) dependence and to conduct a robustness check on the estimates from the PMG and MG estimators. depicts the econometric flowchart of this study.
5. Empirical results
We begin the empirical results by presenting the Pesaran test for cross-sectional dependence; the Im-Pesaran-Shin and Levin-Lin-Chu tests for unit root of all the variables; the Hausman test and finally display the results of both the FGLS and the Dynamic panel ARDL models of the PMG and the MG, respectively, based on the decision of the Hausman Test.
5.1. Test for cross-sectional dependence
The study carries out the Pesaran test for cross-sectional dependence on models 1 and 2 to ascertain whether cross-sectional dependence exists among the residuals. Table presents the results of the estimation. The results show that the null hypothesis of the Pesaran test for cross-sectional independence cannot be accepted for both models 1 and 2 respectively, at the 1% significance level. In all, we conclude that models 1, and 2 are cross-sectionally dependent. This necessitates the possible adoption of the FGLS on the models to account for problem cross-sectional (and temporal) dependence and check for robustness.
Table 2. Pesaran’s test for cross-sectional dependence
5.2. Unit root test
Since most economic variables contain a unit root (Darné & Diebolt, Citation2005), the current study relies on the lm-Pesaran-Shin (IPS) and Levin-Lin-Chu (LLC) first-generation tests of a unit root in heterogeneous panels developed by Pesaran et al. (Citation1997) and Levin et al. (Citation2002), respectively, to determine the unit root properties of the panel. Johansen (Citation1995), and Phillips and Hansen (Citation1990) argued that the existence of a long-run relationship among the variables can only happen when the variables are integrated in the same order. However, Pesaran et al. (Citation1999) show that panel ARDL can be used even if the variables are integrated with different orders. Thus, irrespective of whether they are integrated of I (0) or I (1) or a mixture of both. This is one of the essential features of the panel ARDL estimation techniques as it makes the test for unit root useless. Table reports the results of the IPS and the LLC tests for unit root.
Table 3. Results of the Im-Pesaran-Shin and Levin-Lin-Chu tests for unit root
The results from Table show that the null hypothesis of the presence of unit root is rejected for all the variables at their first difference forms for both IPS and LLC. The general implication is that all the variables are at most integrated of order one, I (1). Hence, estimating the underlying models with the panel ARDL method(s) will not produce spurious regression results.
5.3. Hausman test for selecting an appropriate model (s)
Table presents the results of the Hausman Test for models 1 and 2, respectively. From the table, the results show that for model 1, the Hausman test favors the PMG model as opposed to the MG model since the probability value of the chi-square statistic is insignificant even at the 10% level of significance. On the other hand, the Hausman test favors the MG model, as opposed to the PMG model for model 2 since the probability value of the chi-square statistic is significant at the 1% level of significance. Besides, since the Hausman test favors the MG model for model 2, the study further tests to decide between the MG model and the DFE model. However, the Hausman test results still favor the MG model over the DFE model as the probability value of the chi-square statistic is now insignificant even at the 10% level of significance. Therefore, the study concluded that the PMG model best suits model 1, while the MG model best fits model 2 for the estimation.
Table 4. Hausman test results
5.4. Impact of green energy on economic growth
Table presents the results of both the PMG and the FGLS estimations establishing the relationship between green energy consumption, and nonrenewable energy consumption, including other control variables and economic growth. The results show that green energy (renewable energy) consumption has a short-run negative impact on the economic growth of African countries, albeit the effect is only significant at the 10% level of significance under the FGLS estimation. On the contrary, carbon dioxide (CO2) emissions exert a short-run positive impact on economic growth in Africa under both estimations, although the effect is only significant under the FGLS. Additionally, fossil fuel energy combustion (FEC) exhibits a short-run mixed effect on the economic growth of African countries. Thus, a positive and statistically significant effect under the FGLS estimation and a negative but statistically insignificant effect under the PMG estimation technique. Moreover, the estimates from both estimations show that inflation (INFL) and trade openness (Trade) have short-run significant negative effects on the economic growth of African countries, while gross fixed capital formation (GFCF) has a short-run positive but statistically insignificant effect on the economic growth of African countries.
Table 5. PMG and FGLS results of green energy and economic growth
In terms of the long-run dynamics, the findings indicate that both green energy (renewable energy) consumption and carbon dioxide (CO2) emissions have statistically significant positive impacts on economic growth in Africa. On the other hand, fossil fuel energy combustion (FEC) negatively influences economic growth in Africa over the long run, albeit the effect is generally not significant. Furthermore, trade openness (Trade) and gross fixed capital formation (GFCF) have long-run statistically significant positive effects on economic growth in Africa, while the effect of inflation (INFL) is negative, though it is generally insignificant.
5.5. Impact of natural resource rents on economic growth
Table reports the estimates of the PMG and the FGLS estimations depicting the nexus between Africa’s natural resource rents together with other control variables and economic growth. In the short run, holding other factors constant, the results show that forest rents (FR) have a statistically significant negative impact on economic growth, while total natural resource rents (TNR) have a statistically significant positive impact on economic growth in Africa under both estimation strategies. On the other hand, mineral rents (MR) appear to have a mixed effect on economic growth in Africa. Thus, a negative and statistically significant effect under the FGLS estimation and a positive but statistically insignificant effect under the PMG estimation. Besides, inflation (INFL) and trade openness (Trade) are found to have a short-run statistically significant negative impact on economic growth in Africa under both estimations. Likewise, gross fixed capital formation (GFCF) has short-run positive effects on economic growth in Africa, even though the effect is generally not statistically significant.
Table 6. PMG and FGLS results of natural resource rents and economic growth
Table 7. List of countries selected for this study
Presenting the results from the long-run perspectives, we found evidence of long-run relationships among the variables as the ETC (−0.1041457) is greater than −2. However, the results indicate that forest rents (FR) and total natural resource rents (TNR) impact negatively on the economic growth of African countries over the long run, albeit the effects are generally not statistically significant. Also, the effects of mineral rents (MR) on economic growth in Africa are positive, though it is generally not significant. Moving further, trade openness (Trade) and gross fixed capital formation (GFCF) are found to have a long-run positive effect on economic growth in Africa, while the effect of inflation (INFL) is negative, though these effects are not statistically significant when estimated with Africa’s natural resource rents.
6. Discussion of results
The advancement and advocacy for the usage of green energy occupy the heart of growth and development scholarship today. This is mainly because of the three planetary crises faced by the world today: climate change, biodiversity loss, and pollution. Likewise, what is so-called “Africa’s resource curse” has also sparked severe controversies in the growth and development literature. This study, the first of its kind, jointly assesses the impacts of green energy and Africa’s natural resource rents on economic growth in Africa.
The results acquiescently show that renewable energy consumption has a statistically significant short-run negative and long-run positive impact on economic growth in Africa. The negative short-run relationship between renewable energy and economic growth in Africa comes as no surprise as shifting to the usage of renewable energy sources for industrial production and other economic activities will take quite a long time for these countries to cover the losses in production. It is not surprising, however, that it positively and statistically significantly impacted economic growth in the long run in Africa. The long-run positive impact of renewable energy consumption on economic growth is more sustainable as the emissions of greenhouse gases into the atmosphere will be reduced. The reduction in greenhouse gas emissions will go a long way to help combat the current three planetary crises as already mentioned above and their disastrous effects on both plants and animals. The results of this current study corroborate with previous studies (Pearson, Citation2021; Qudrat-Ullah & Nevo, Citation2021; Riti et al., Citation2022) in which renewable energy consumption was found to have a long-run positive impact on economic growth in Croatia, Africa, and sub-Saharan Africa, respectively. However, their short-run results contradict the current study. Likewise, the short-run negative impact of renewable energy on growth in this study is in agreement with the results of the study by Maji (Citation2015) on the effects of renewable on economic growth in West Africa.
Furthermore, carbon dioxide (CO2) emissions exert both short and long-run positive and statistically significant impacts on economic growth in Africa. These findings are reasonable in that higher production correlates with higher emissions of greenhouse gases into the atmosphere as most of the energy sources in Africa come from nonrenewable sources. However, the positive short and long-run impacts of CO2 emissions on economic growth in Africa are not sustainable as it worsens the three planetary crises already demonstrated in this study. Aydoğan and Vardar (Citation2020) argued that the burning of fossil fuels (usually coal and natural gas) stimulates economic growth but it poses serious consequences to our planet and the reduction of its use is vital for the planet’s welfare. Similarly, the findings indicate that fossil fuel energy combustion (FEC) has a short-run significant positive impact and a long-run insignificant negative impact on economic growth in Africa. The results of this study concord with the findings of previous studies (Borhan et al., Citation2012; Chen & Huang, Citation2013; Ivanovski et al., Citation2021; Sannassee, Citation2015). However, Mikayilov et al. (Citation2018) found no long-run relationship between CO2 emissions and economic growth in Azerbaijan. The findings of this study further revealed that the Environmental Kuznets Hypothesis does not hold for Africa as higher emissions stimulate growth in Africa over the long run.
In terms of Africa’s natural resource rents’ impact on growth, we found evidence of the so-called “natural resource curse” in Africa. The findings from Table convincingly show that forest rents (FR) and mineral rents (MR) have a short-run statistically significant negative impact and long-run statistically insignificant effects forest rents on growth, while mineral rents positively impact economic growth in Africa, albeit the effect is generally not statistically significant. However, total natural resource rents (TNR) have statistically significant short-run positive growth effects and statistically insignificant negative long-run growth effects in Africa. This could arguably be due to poor natural resource governance in Africa in terms of the entire value chain of natural resources: exploitation, extraction, marketing, and rents. Due to the poor natural resource governance in Africa, the potential growth and employment elasticities of the luxurious natural resources are lost. Africa’s resources are being controlled and governed by foreign companies who extract and repatriate trillions of dollars in tax havens, trade misinvoicing, unfair tax deals, and other illicit financial flows. According to a report by War on Want (Citation2016) about 101 companies listed on the London Stock Exchange (LSE)—most of them British, have mining operations in 37 sub-Saharan African countries. The report indicated that these companies collectively control over $1 trillion worth of Africa’s most valuable resources. In the same vein, Honest Accounts (Citation2017), and War on Want (Citation2016) demonstrated that an approximated amount of $41 billion is extracted each year out of Africa, mainly in the form of repatriation of profits by foreign companies, tax dodging, and the costs of adapting to climate change. How can any continent, country, or region be prosperous with such statistics? Africa needs to properly manage and owns its resources should the continent want to reap the growth and employment elasticities of its abundant resources. The long-run negative relationship between Africa’s natural resource rents and economic growth confirms the findings of past empirical studies (Dramani et al., Citation2022; Mohamed, Citation2020; S. Tang et al., Citation2022) in which total natural resource rent was found to have a significant negative impact on economic growth and financial development. On the contrary, studies by (Christa, Citation2008; Muhamed et al., Citation2021; Sharma & Paramati, Citation2022) found no evidence of the resource curse hypothesis; however, their studies were more general and limited to those regions in which the studies were conducted. In the same vein, Ousama et al. (Citation2021) using top-natural resource-abundant countries found evidence of the resource curse hypothesis in the short run, while no such hypothesis was found in the long run. Similarly, the negative impact of forest and mineral rents on economic growth found in this study reaffirms the findings of the study by Dramani et al. (Citation2022) in which it was observed that forest rents exhibit a negative and statistically significant effect on economic growth in sub-Saharan Africa at all threshold levels, while the negative effects of mineral rents vanish beyond a threshold of 15% of GDP.
Apart from green energy and Africa’s natural resource vis-a-vis economic growth nexus, the current study also investigates the impact of trade openness (Trade), gross fixed capital formation (GFCF), and inflation (INFL) on economic growth in Africa. The results reasonably show that trade openness and inflation have short-run statistically significant negative impacts on economic growth in Africa. However, the impact of trade openness on growth is positive and statistically significant over the long run, while the effect of inflation is still negative though not statistically significant. The negative short- and long-run relationship between inflation and economic growth in Africa indicates that higher inflation rates are detrimental to the growth of the African economies as it distorts the price system and raises the costs of living for the average consumer. This finding is in tandem with the studies by (Burdekin et al., Citation2004; Gylfason & Herbertsson, Citation2001; López-Villavicencio & Mignon, Citation2011; Sarel, Citation1996) in which inflation is found to hurt economic growth beyond a certain threshold in developing countries. Besides, the findings imply that trade openness initially worsens economic growth in Africa due to significant tax cuts and subsidies, however, it later acts as a stimulant for growth and development in Africa over the long run. This finding provides empirical evidence that the African Continental Free Trade Area (AfCFTA) may be a good step toward revitalizing the growth and employment prospect of the African continent over the long run. These findings corroborate the results of previous studies (Dollar & Kraay, Citation2001; Mumuni & Braimah Abille, Citation2022; World Bank, Citation2005) in which it is found that trade openness catalyzed long-run economic growth and income inequality reduction. Lastly, the results depict that gross fixed capital formation has a short-run insignificant positive and long-run significant positive impact on economic growth in Africa. The implication is that domestic investment is necessary for the overhaul growth and development of the African continent. The results, therefore, conform with the findings of past empirical studies (Etokakpan et al., Citation2020; Li et al., Citation2022; Suwandaru et al., Citation2021; Zaman et al., Citation2021).
7. Conclusion and policy recommendations
This study employs two different estimation techniques (the FGLS and the dynamic panel ARDL models of the PMG and MG) estimators to assess the impact of green energy and Africa’s natural resource rents on economic growth in the continent. The findings revealed that green energy initially worsens economic growth in Africa and later acts as a precursor of growth over the long run. On the other hand, carbon dioxide (CO2) emissions stimulate economic growth in Africa over the short and long run, while fossil fuel energy combustion stimulates economic growth in the short run and has negative long-run effects on economic growth in Africa. Additionally, the study found evidence of Africa’s natural resource curse as the findings reveal that mineral and forest rents have significant short-run negative impacts on economic growth, albeit the effects are generally not significant in the long run. Similarly, total natural resource rents in Africa have statistically significant short-run positive and long-run statistically insignificant negative impacts on economic growth.
Based on these findings, we proposed the following recommendations for a better policy perspective in Africa toward achieving the United Nations Sustainable Development Goals.
The study proposes that African governments need to encourage the production and usage of renewable energies such as geothermal, hydropower, wind, and solar, among others for both domestic and industrial production. This will help to reduce the amount of CO2 emissions, a key greenhouse gas responsible for more than 70% of global emissions causing global warming and other climatic uncertainties. This policy initiative will also help to ensure cleaner and greener air and enhance environmental sustainability and economic growth in general;
Export diversification and green technology industrialization should be the hallmark of African governments. Green technology industrialization is necessary to foster export diversification while maintaining ecological sustainability. This will help African governments to get the most out of their innumerable natural resources, mostly exported in their raw forms and command lower prices at the world market. Africa’s resources are usually exported unprocessed and as such exposed to price instabilities and unfavorable terms of trade;
The study further recommends that African governments need to collaboratively create independent and strong institutions to monitor and effectively manage their abundant and luxurious natural resources. Transparency and Accountability are paramount in the entire value chain of natural resources and to ensure that Africa’s natural resources rents are linked to their growth and employment elasticities. Independent and strong institutions across the globe need to also support African governments in imposing tax obligations and royalty rates on the resources extracted by foreign companies;
The global shadow banking system which promotes illicit financial activities and corporate tax evasion via secrecy laws, tax havens, and shell corporations must be destroyed. Thus, there should be a revamp of the global tax rules;
Lastly, taming inflation, strengthening governments’ commitments to the AfCFTA, encouraging domestic investment, and/or developing more physical infrastructure are effective ways to promote growth and development in Africa over the long run.
8. Limitations of the study
The findings of this study are limited to the selected African countries and/or Africa as a whole. The results may not apply to other parts of the globe. Therefore, care should be taken when using these findings for policy directions in other regions of the world.
9. Suggested areas for further research
Future researchers can explore the modulation or mediation effect of governance, institutional quality, or human capital development in the causal relationship between green energy consumption, natural resource rents, and economic growth dynamics.
Data Availability
Data used for this study can be found online at https://data.worldbank.org/indicator (accessed on 1 September 2022).
Disclosure statement
No potential conflict of interest was reported by the author(s).
Additional information
Funding
References
- Abbasi, K., Jiao, Z., Shahbaz, M., & Khan, A. (2020). Asymmetric impact of renewable and non-renewable energy on economic growth in Pakistan: New evidence from the nonlinear analysis. Energy Exploration and Exploitation, 38(5), 1946–22. https://doi.org/10.1177/0144598720946496
- Adabor, O., Buabeng, E., & Annobil-Yawson, G. (2020). The impact of natural resource rent on economic growth of Ghana. Asian Journal of Economics, Business, and Accounting, January, 1–17. https://doi.org/10.9734/ajeba/2020/v19i130293
- AfDB. (2015). African Natural Resources Center (ANRC) strategy (2015-2020): African development bank group. Revised Version. https://www.google.com/url?sa=t&rct=j&q=&esrc=s&source=web&cd=&cad=rja&uact=8&ved=2ahUKEwjPip2iwI38AhULNOwKHRxRD9AQFnoECAMQAQ&url=https%3A%2F%2Fwww.afdb.org%2Fen%2Fdocuments%2Fdocument%2Fafrican-natural-resources-centres-strategy-for-2015-2020-54305&usg=AOvVaw3Zz2JNHrS8hLcca9pvXmc7
- Alola, A. A., Tomiwa, I., & Adebayo, S. (2022). Are green resource productivity and environmental technologies the face of environmental sustainability in the Nordic region? Sustainable Development. https://doi.org/10.1002/SD.2417
- Apergis, N., & Payne, J. E. (2012). The electricity consumption-growth nexus: Renewable versus non-renewable electricity in Central America. Energy Sources, Part B: Economics, Planning, and Policy, 7(4), 423–431. https://doi.org/10.1080/15567249.2011.639336
- Apergis, N., Payne, J. E., Menyah, K., & Wolde-Rufael, Y. (2010). On the causal dynamics between emissions, nuclear energy, renewable energy, and economic growth. Ecological Economics, 69(11), 2255–2260. https://doi.org/10.1016/j.ecolecon.2010.06.014
- Ari, E., & Auty, R. (2016). Links between resource extraction, governance, and development : African links between resource extraction, governance, and development : African Experience (ARI). October .
- Awosusi, A. A., Mata, M. N., Ahmed, Z., Coelho, M. F., Altuntaş, M., Martins, J. M., Martins, J. N., & Onifade, S. T. (2022). How do renewable energy, economic growth, and natural resources rent affect environmental sustainability in a globalized economy? Evidence from Colombia based on the gradual shift causality approach. Frontiers in Energy Research, 9(January), 1–13. https://dx.doi.org/10.3389/fenrg.2021.739721
- Ayanlade, A., Oladimeji, A. A., Okegbola, O. M., Eludoyin, A. O., Eslamian, S., Ayinde, A. F. O., & Perkins, P. E. (2022). Effect of climate change on water availability and quality: An assessment of socio-resilience in Nigeria. Disaster Risk Reduction for Resilience, 245–262. https://doi.org/10.1007/978-3-030-99063-3_11
- Aydoğan, B., & Vardar, G. (2020). Evaluating the role of renewable energy, economic growth, and agriculture on CO2 emission in E7 countries. International Journal of Sustainable Energy, 39(4), 335–348. https://doi.org/10.1080/14786451.2019.1686380
- Beck, N. (2001). Time series - cross-sectional data: What have we learned in the past few years? Annual Review of Political Science, 4(1), 271–293. http://search.ebscohost.com/login.aspx?direct=true&db=buh&AN=5367194&site=ehost-live%0Ahttp://www.annualreviews.org/doi/10.1146/annurev.polisci.4.1.271
- Borhan, H., Ahmed, E. M., & Hitam, M. (2012). The impact of Co2 on economic growth in ASEAN 8. Procedia - Social and Behavioral Sciences, 35(December 2011), 389–397. https://doi.org/10.1016/j.sbspro.2012.02.103
- British Petroleum. (2022). Energy Outlook 2022 Edition: Report by British Petroleum.
- Brooks, R. (2011). CO2 emissions and economic growth. The International Journal of Climate Change: Impacts and Responses, 2(3), 25–36. https://doi.org/10.18848/1835-7156/cgp/v02i03/37070
- Burdekin, R. C. K., Denzau, A. T., Keil, M. W., Sitthiyot, T., & Willett, T. D. (2004). When does inflation hurt economic growth? Different nonlinearities for different economies. Journal of Macroeconomics, 26(3), 519–532. https://doi.org/10.1016/j.jmacro.2003.03.005
- Chen, J.-H., & Huang, Y.-F. (2013). The study of the relationship between carbon dioxide (CO 2) emission and economic growth. Journal of International and Global Economic Studies, 6(2), 45–61.
- Christa, N. B. (2008). Cursing the blessings? Natural resource abundance, institutions, and economic growth. World Development, 36(3). https://doi.org/10.1016/j.worlddev.2007.03.004
- Dardozzi, J., (2020). Jeff Dardozzi: Carbon dioxide – the effluent of wealth. https://www.google.com/url?sa=t&rct=j&q=&esrc=s&source=web&cd=&cad=rja&uact=8&ved=2ahUKEwi1kO75xY38AhUtu6QKHal9AtAQFnoECA8QAQ&url=https%3A%2F%2Fvtdigger.org%2F2020%2F09%2F11%2Fjeff-dardozzi-carbon-dioxide-the-effluent-of-wealth%2F&usg=AOvVaw3iqJyu41QfDnWwbrJLc4bV
- Darné, O., & Diebolt, C. (2005). Non-stationarity tests in macroeconomic time series BT - new trends in macroeconomics. C. Diebolt & C. Kyrtsou eds. 173194. 173194. Springer:. https://doi.org/10.1007/3-540-28556-3_9
- Dasgupta, S., Laplante, B., Wang, H., & Wheeler, D. (2002). Confronting the environmental Kuznets curve. Journal of Economic Perspectives, 16(1), 147–168. https://doi.org/10.1257/0895330027157
- Dogan, E., & Ozturk, I. (2017). The influence of renewable and non-renewable energy consumption and real income on CO2 emissions in the USA: Evidence from structural break tests. Environmental Science and Pollution Research, 24(11), 10846–10854. https://doi.org/10.1007/S11356-017-8786-Y
- Dollar, D., & Kraay, A. (2001). Trade, growth, and poverty. Policy Research Working Paper. No. 2615. https://hdl.handle.net/10986/19597
- Dramani, J. B., Abdul Rahman, Y., Sulemana, M., & Owusu-Takyi, P. (2022). Natural resource dependence and economic growth in SSA: Are there threshold effects? Development Studies Research, 9(1), 230–245. https://doi.org/10.1080/21665095.2022.2112728
- Elbadawi, I., & Soto, R. (2016). Resource rents, political institutions, and economic growth. Understanding and Avoiding the Oil Curse in Resource-Rich Arab Economies, 187–224. https://doi.org/10.1017/CBO9781316493854.008
- Etokakpan, M. U., Solarin, S. A., Yorucu, V., Bekun, F. V., & Sarkodie, S. A. (2020). Modeling natural gas consumption, capital formation, globalization, CO2 emissions and economic growth nexus in Malaysia: Fresh evidence from combined cointegration and causality analysis. Energy Strategy Reviews, 31, 100526. https://doi.org/10.1016/J.ESR.2020.100526
- Gylfason, T., & Herbertsson, T. T. (2001). Does inflation matter for growth? Japan and the World Economy, 13(4), 405–428. https://doi.org/10.1016/S0922-1425(01)00073-1
- Hoechle, D. (2007). Robust standard errors for panel regressions with cross-sectional dependence. Stata Journal, 7(3), 281–312. https://doi.org/10.1177/1536867X0700700301
- Ibrahim, R. L., Adebayo, T. S., Awosusi, A. A., Ajide, K. B., Adewuyi, A. O., & Bolarinwa, F. O. (2022). Investigating the asymmetric effects of renewable energy-carbon neutrality nexus: Can technological innovation, trade openness, and transport services deliver the target for Germany? 0958305X2211270. https://doi.org/10.1177/0958305X221127020
- Ivanovski, K., Hailemariam, A., & Smyth, R. (2021). The effect of renewable and non-renewable energy consumption on economic growth: Non-parametric evidence. Journal of Cleaner Production, 286. https://doi.org/10.1016/j.jclepro.2020.124956
- Johansen, S. (1995). Likelihood-based inference in cointegrated vector autoregressive models. Likelihood-Based Inference in Cointegrated Vector Autoregressive Models. https://doi.org/10.1093/0198774508.001.0001
- Kahia, M., Ben Jebli, M., & Belloumi, M. (2019). Analysis of the impact of renewable energy consumption and economic growth on carbon dioxide emissions in 12 MENA countries. Clean Technologies and Environmental Policy, 21(4), 871–885. https://doi.org/10.1007/S10098-019-01676-2
- Khan, S. A. R., Ibrahim, R. L., Al-Amin, A. Q., & Yu, Z. (2022). An ideology of sustainability under technological revolution: Striving towards sustainable development. Sustainability (Switzerland), 14(8). https://doi.org/10.3390/SU14084415
- Kirikkaleli, D., & Adebayo, T. S. (2022). Political risk and environmental quality in Brazil: Role of green finance and green innovation. International Journal of Finance & Economics. https://doi.org/10.1002/ijfe.2732
- Kmenta, J. (1988). Elements of Econometrics (2nd). Macmillan Publishing Co., 1986, xii + 786 pp. In American journal of agricultural economics. Vol. 70(1). 210–211. https://doi.org/10.2307/1242003
- Levin, A., Lin, C.-F., & James Chu, C.-S. (2002). Unit root tests in panel data: Asymptotic and finite-sample properties. Journal of Econometrics, 108(1), 1–24. https://doi.org/10.1016/S0304-4076(01)00098-7
- Li, Y., Tariq, M., Khan, S., Rjoub, H., & Azhar, A. (2022). Natural resources rents, capital formation and economic performance: Evaluating the role of globalization. Resources Policy, 78, 102817. https://doi.org/10.1016/J.RESOURPOL.2022.102817
- López-Villavicencio, A., & Mignon, V. (2011). On the impact of inflation on output growth: Does the level of inflation matter? Journal of Macroeconomics, 33(3), 455–464. https://doi.org/10.1016/J.JMACRO.2011.02.003
- Magazzino, C., Toma, P., Fusco, G., Valente, D., & Petrosillo, I. (2022). Renewable energy consumption, environmental degradation, and economic growth: The greener the richer? Ecological Indicators, 139, 108912. https://doi.org/10.1016/j.ecolind.2022.108912
- Maji, I. K. (2015). Does clean energy contribute to economic growth? Evidence from Nigeria. Energy Reports, 1, 145–150. https://doi.org/10.1016/j.egyr.2015.06.001
- Maji, I. K., Sulaiman, C., & Abdul-Rahim, A. S. (2019). Renewable energy consumption and economic growth nexus: A fresh evidence from West Africa. Energy Reports, 5, 384–392. https://doi.org/10.1016/J.EGYR.2019.03.005
- Mark, Curtis , Tim, Jones Honest Accounts, Global Justice Now. (2017). How the world profits from Africa’s wealth (pp. 1–12). https://www.google.com/url?sa=t&rct=j&q=&esrc=s&source=web&cd=&cad=rja&uact=8&ved=2ahUKEwjjwI-Eyo38AhWBCuwKHbhiAc4QFnoECBAQAQ&url=https%3A%2F%2Fwww.cadtm.org%2FHonest-Accounts-2017-How-the-world&usg=AOvVaw1CLIYoB-IxU0L-iIT-IyZ2
- Mehar, M., Hasan, A., Sheikh, M., & Adeeb, B. (2018). Total natural resources rent relation with economic growth: The case of Pakistan and India. European Journal of Economics and Business, 03(2350–2282), 14–22.
- Mikayilov, J. I., Galeotti, M., & Hasanov, F. J. (2018). The impact of economic growth on CO2 emissions in Azerbaijan. Journal of Cleaner Production, 197(2018), 1558–1572. https://doi.org/10.1016/j.jclepro.2018.06.269
- Mohamed, E. S. E. (2020). Resource rents, human development and economic growth in Sudan. Economies, 8(4), 99. https://doi.org/10.3390/ECONOMIES8040099
- Muhamed, H., Sebastian, K., Hafezali, I. M., & Fakarudin, K. (2021). The natural resources curse-economic growth hypotheses: quantile–on–quantile evidence from top Asian economies. Journal of Cleaner Production. https://doi.org/10.1016/j.jclepro.2020.123596
- Mumuni, S., & Braimah Abille, A. (2022). Do trade liberalization and external debt offset income inequality? New evidence from selected African countries. https://doi.org/10.21203/RS.3.RS-1929320/V1
- Ofori, P. E., Grechyna, D., & Shafiullah, M. (2021). Remittances, natural resource rent, and economic growth in Sub-Saharan Africa. Cogent Economics and Finance, 9(1). https://doi.org/10.1080/23322039.2021.1979305
- Ousama, B. S., Hajer, D., & Maamar, S. (2021). Natural resource rents and economic growth in the top resource-abundant countries: A PMG estimation. Resources Policy, 74(6). https://doi.org/10.1016/j.resourpol.2018.07.005
- Pao, H. T., & Fu, H. C. (2013). Renewable energy, non-renewable energy, and economic growth in Brazil. Renewable and Sustainable Energy Reviews, 25, 381–392. https://doi.org/10.1016/j.rser.2013.05.004
- Parks, R. W. (1967). Efficient estimation of a system of regression equations when disturbances are both serially and contemporaneously correlated. Journal of the American Statistical Association, 62(318), 500–509. https://doi.org/10.1080/01621459.1967.10482923
- Pasten, R., & Figueroa, E. B. (2012). The environmental Kuznets curve: A survey of the theoretical literature. International Review of Environmental and Resource Economics, 6(3), 195–224. https://dx.doi.org/10.1561/101.00000051
- Pearson, S. (2021). The effect of renewable energy consumption on economic growth in Croatia. Zagreb International Review of Economics and Business, 24(1), 113–126. https://doi.org/10.2478/zireb-2021-0006
- Pesaran, M. H., Shin, Y., & Smith, R. P. (1997). Pooled estimation of long-run relationships in dynamic heterogeneous panels. https://econpapers.repec.org/RePEc:cam:camdae:9721
- Pesaran, M. H., Shin, Y., & Smith, R. P. (1999). Pooled mean group estimation of dynamic heterogeneous panels. Journal of the American Statistical Association, 94(446), 621. https://doi.org/10.2307/2670182
- Pesaran, M. H., & Smithb, R. (1995). Estimating long-run relationships from dynamic heterogeneous panels. Journal of Econometrics, 68(1995), 79–113. https://doi.org/10.1016/0304-4076(94)01644-F
- Phillips, P. C. B., & Hansen, B. E. (1990). Statistical inference in instrumental variables regression with I(1) processes. The Review of Economic Studies, 57(1), 99–125. https://doi.org/10.2307/2297545
- Polimeni, J. M. (2007). Jevons ‟ paradox and the economic implications for Europe. International Business & Economics Research Journal (IBER), 6(10), 109–120. . https://doi.org/10.19030/iber.v6i10.3422
- Qudrat-Ullah, H., & Nevo, C. M. (2021). The impact of renewable energy consumption and environmental sustainability on economic growth in Africa. Energy Reports, 7, 3877–3886. https://doi.org/10.1016/j.egyr.2021.05.083
- Riti, J. S., Riti, M. K. J., & Oji-Okoro, I. (2022). Renewable energy consumption in sub-Saharan Africa (SSA): Implications on economic and environmental sustainability. Current Research in Environmental Sustainability, 4(March. https://doi.org/10.1016/j.crsust.2022.100129
- Sachs, D., & Warner, M. (1995). Natural resource abundance and economic growth. NBER Working Paper, 3, 47. https://doi.org/10.7916/D86T0TC1
- Sannassee, R. V. (2015). On the relationship between CO2 emissions and economic growth: The mauritian experience. January, 1–25.
- Sarel, M. (1996). Nonlinear effects of inflation on economic growth. IMF Staff Papers, https://www.elibrary.imf.org/view/journals/024/1996/001/024.1996.issue-001-en.xml(1), 199–215. . https://doi.org/10.5089/9781451957099.024
- Sharma, C., & Paramati, R. (2022). Resource curse versus resource blessing: New evidence from resource capital data. Energy Economics, 115. https://doi.org/10.1016/j.eneco.2022.106350
- Suwandaru, A., Alghamdi, T., & Nurwanto, N. (2021). Empirical analysis on public expenditure for education and economic growth : Evidence from Indonesia. 1–13. https://doi.org/10.3390/economies9040146
- Tang, C., Irfan, M., Razzaq, A., & Dagar, V. (2022). Natural resources and financial development: Role of business regulations in testing the resource-curse hypothesis in ASEAN countries. 10, 2. https://doi.org/10.1016/j.resourpol.2022.102612
- Tang, S., Ma, Y., & Altuntaş, M. (2022). Natural resources volatility, political risk and economic performance: Evidence from quantile-on-quantile regression. Resources Policy, 78. https://doi.org/10.1016/j.resourpol.2022.102842
- United Nations. (2022). The sustainable development goals report 2022. In United Nations publication issued by the department of economic and social affairs. https://unstats.un.org/sdgs/report/2022/The-Sustainable-Development-Goals-Report-2022.pdf
- War on Want. (2016). The new colonialism: Britain’s scramble for Africa’s energy and mineral resources. 629916. http://media.waronwant.org/sites/default/files/TheNewColonialism.pdf?_ga=1.163857279.1562019882.1468355472
- World Bank. (2005). World development report 2005. World Bank Group. https://doi.org/10.1596/0-8213-5682-8
- Zaman, M., Pinglu, C., Hussain, S. I., Ullah, A., & Qian, N. (2021). Does regional integration matter for sustainable economic growth? Fostering the role of FDI, trade openness, IT exports, and capital formation in BRI countries. Heliyon, 7(12), e08559. https://doi.org/10.1016/J.HELIYON.2021.E08559