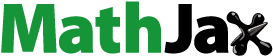
Abstract
The main objective of this paper is to examine the determinants of portfolio at risk in Palestine by analyzing the impact of macroeconomic and micro-level factors on credit risk for microfinance institutions during the period of 2010–2020. This study includes five regulated MFIs using generalized method of moment estimator. The findings indicate that portfolio at risk is more sensitive to the institutional factors and economic cycle. The operating efficiency and number of loan officers have positive and significant effect on credit risk. However, growth in GDP and number of active borrowers have negative and significant effect on credit risk. But, no evidence supports the impact of inflation and interest rate on vulnerability of credit risk. The conclusion presented may be useful for MFI directors and regulators in timely repayments and credit risk control. Overall, our ample evidence suggests that MFIs directors should consider the influence of institutional factors on their investment and credits decisions.
PUBLIC INTEREST STATEMENT
This study concludes an interesting results to the public and non-specialist readers of this research in different ways. First, the issue is microfinance, which is backbone for poor households and poverty alleviation. Second, this conclusion could be benefit for serving economic sectors in Palestine: agriculture, handcraft, and women projects. Third, the existing study will provide guidelines to the MFIs management and policy makers in order to redesign the credit policy and strategy to further improve loans quality and hedging from credit risk against any potential crisis.
1. Introduction
During the last two decades, microfinance institutions (MFI) grew faster and served large numbers of poor households (Robert & Jonathan, Citation2017). This body promises to fight poverty alleviation and supports borrowers with lower incomes beside their social role toward societies (Khan et al., Citation2020). The economic conditions put MFI under pressure in case of social and financial self-sufficiency. Therefore, MFI in global has been posing many obstacles and threats over the last decade due to increase in economic fluctuations and financial instability (Weber & Musshoff, Citation2012). Active borrowers are unable to repay their obligations and outstanding loans and MFIs have faced potential loan losses. In this case, portfolio at risk is considered the source of MFIs crisis. Lately, portfolio at risk (PAR30) has been defined in different ways. Necesito (Citation2016) considered PAR30 as proportion of gross loan portfolio that overdue by more than 30 days. Alternatively, PAR30 is an effective instrument that used to measure credit risk across MFIs. Therefore, the repayments and arrear rates provide information about quality of loans portfolio (Crabb & Keller, Citation2006). In this regard, recent studies mentioned that PAR30 ruins Lloans quality of MFIs (Durango-Gutiérrez et al., Citation2021; Sifrain, Citation2022; Teferi, Citation2019).
Despite of the notable developing to the Palestinian microfinance sector, the necessity of risk control has gotten little attention. This sector stands a critical juncture due to the political and economic challenges. In this sense, Morrar et al. (Citation2021) argued that microfinance sector has great opportunities of growth and has vital role in promoting comprehensive and sustainable development in Palestine. However, the quality of the portfolio seems to be an issue, where MFIs present weak credit history and that can be worrisome (Migdad, Citation2022). Moreover, World bank (Citation2020) reported that the economic uncertainty and the protracted crises in Palestine have gradually deteriorated quality of loans portfolio across the microfinance sector. Such schemes effect on credit of livelihoods as they stopping repayment loans. This casts doubt the credit policy of MFIs and hampers credits collection.
The related literature to the portfolio at risk is mainly focused on quality of loan portfolio. Some previous studies have reported the importance of studying the factors of loan default in supporting decision makers regarding the provision of credits and financial availability of MFIs (Durango-Gutiérrez et al., Citation2021; Ngonyani & Mapesa, Citation2019). Nevertheless, a limited number of studies examined the impact of macroeconomic and micro-level factors on portfolio at risk (Ayayi, Citation2012; Inekwe, Citation2019; Lassoued, Citation2017; Silva et al., Citation2015). Thus, this study differs from other previous literature in the scrutiny of research variables and the methodological approach that taken therein.
In Palestinian context, few prior literature have been primarily studied the microfinance industry (Al-Bitawi, Citation2016; Martino & Sarsour, Citation2009; Tabbaa, Citation2019). To our knowledge, no study has yet undertake the modeling factors of portfolio at risk in MFIs from emerging country like Palestine. Therefore, this study aims to fill this knowledge gap and to extend the existing literature through extensively analyze the relationship between economic, institutional factors, and portfolio at risk in context of MFIs in Palestine. This article comes to the massive effect of these factors on portfolio in MFIs. Moreover, it is remarkable to examine the sudden change in economic cycle resulted in a significant change in PAR30 last decade.
This study contributes to the existing literature into three-folds. First. This paper aims to provide a good knowledge in financial self-sufficiency and risk control for MFIs in Palestine. Second, this study uses different methodological approaches in predicting portfolio at risk relied on panel data estimates and generalized of method moment (GMM). In the best of researcher knowledge, it is considered the kindest conducted research in such relationship. Third, this study contributes in helping MFI directors to evaluate their investment portfolio and hedging their assets from credit risk. Therefore, the results might have important implications in risk control for MFI directors, policymakers and monetary authorities in Palestine.
The rest of the paper is organized into five sections. The next part deals with a review of relevant literature and formulates the hypotheses based on theoretical foundation. The third part describes the research methodology. The fourth part presents empirical results and discussion. The final part concludes results and research implications as well as further future research directions.
2. Literature review
The theoretical literature and hypotheses development underpinning this research are explored in this section. This part presents the theoretical foundation of portfolio at risk and formulates the research hypotheses of portfolio at risk, macroeconomic variables and micro-level factors.
2.1. Theoretical foundation of Portfolio at risk
Theoretically, when borrowers unable to repay their loans over than 30 days, it becomes default. This practice indicates microfinance firm is more vulnerable to credit risk (Agasha et al., Citation2020). However, Safiullah and Shamsuddin (Citation2018) considered credit risk as a late payment of more than 90 days by borrowers to the contractual term. Therefore, De Oliveira (Citation2017) argued MFI with lower level of portfolio risk has a good quality of loans.
In banking literature, many research scholars ascertained that Nnon-Performing Loans (NPLs) ratio was used to measure credit risk (Abusharbeh, Citation2020; Chaibi & Ftitit, Citation2015; Kabir et al., Citation2022; Koju et al., Citation2020; Naili & Lahrichi, Citation2022). However, some researchers uses portfolio at risk (PAR30) as a proxy of credit risk in MFIs (Chikalipah, Citation2018; Durango-Gutiérrez et al., Citation2021; Inekwe, Citation2019; Lassoued, Citation2017; Silva et al., Citation2015). There were two group of factors that theoretically account for predicting credit risk in finance literature. First, the perspective of economic uncertainty including macroeconomic indicators, such as inflation, nominal interest, growth in GDP. Second, micro-level factors (personnel, operating costs, active borrowers) that significantly effect on MFIs credit risk and incentive to provide higher quality of loans. In this sense, the majority of empirical studies have shown different results between these factors and portfolio at risk. Therefore, this study fills the knowledge gap in pervious literature that only examined the effect of some economic variables on portfolio at risk (Silva et al., Citation2015) and other previous studies that used micro-level factors of PAR30 (Durango-Gutiérrez et al., Citation2021; Lassoued, Citation2017). For this reason, this study modeling the factors of portfolio at risk in MFIs from developing country perspective like Palestine.
2.2. Macroeconomic variables and portfolio at risk
Inflation measures the economic stability in the research model. Consumer Price Index (CPI) was used as proxy of inflation rate to predict credit risk in the banking system (Wiryono & Effendi, Citation2018). CPI is a major consideration when it comes to the performance of investment portfolio, because it can be challenge for investors to mitigate the risk to their portfolios. Thus, there were a mixed results from previous studies on the connection between inflation and portfolio at risk. Lassoued (Citation2017) argued that inflation can weaken the ability of borrowers to repay their debts by reducing their real income. In contrast, Crabb and Keller (Citation2006) mentioned that inflation rate negatively influences portfolio at risk. Similarly, Bohachova (Citation2008) provided evidence that higher inflation rates lead to decrease the credit risk of MFIs. Based on earlier mentioned, this study puts forward the following research hypothesis:
H01: inflation has a significant and negative effect on portfolio at risk in Palestinian context.
Another factor used in predicting credit risk of MFIs is interest rate. It reflects the monetary policy in our conceptual model and considered the central source of risk for the conventional bank. In the light of asymmetric information theory, interest rate is the core of adverse selection problem, that is higher interest rate will deter the potential borrowers with riskless projects (Stiglitz & Weiss, Citation1981). In this regard, Ngonyani and Mapesa (Citation2019) found a positive connection between interest rate charged and portfolio at risk in Tanzania. Similarly, other prior studies (Cerqueiro et al., Citation2014; Loannidou et al., Citation2022) confirmed the positive connection between interest and credit risk. Hence, the research hypothesis can be formulated as follows:
H02: Interest rate has significant and positive effect on portfolio at risk in Palestinian context.
Regarding to the previous studies (Chaibi & Ftitit, Citation2015; Kjosevski & Petkovski, Citation2017; Koju et al., Citation2020), growth in GDP has a negative influence on credit risk. An increased in GDP indicates an expansion of economic cycle and increased in the capacity of borrower to borrow additional funds. GDP leads to increase the purchasing power and the borrower’s ability to repay their loans without any default. Therefore, it has negative association with credit risk of MFIs. However, Necesito (Citation2016) argued that growth GDP leads to deteriorate the repayment of borrowers. In contrast, Lassoued (Citation2017) provided that no evidence for the impact of GDP growth on MFI’s portfolio at risk. Hence, drawing from earlier discussion, it is evident that previous literatures are debated on relationship between growth in GDP and credit risk. Thus, the research hypothesis can be formulated as follows:
H03: GDP has a significant and negative effect on portfolio at risk in Palestinian context.
2.3. Micro-level factors and portfolio at risk
As for institutional factors of MFIs, employee staff is considered an influential factor of credit risk for MFIs. Personnel is defined as the number of individuals who are actively employed by MFI. Regarding this factor, it is logical to assume that an increase in number of staff in MFIs should have positive effect on credit management, which would ultimately improve capability of people to repay their debts. Thus, prior studies confirm this conclusion. Inekwe (Citation2019) found that the number of loans officers is positively related to portfolio at risk. Further, Yimga (Citation2016) argued that more staff of MFI devotes to risk control, and the better quality loans of portfolio. Similarly, Wydick (Citation1999) argued that the number of loan officers leads to increase ability of monitoring borrower’s repayment and thus MFIs with higher number of employees may lower credit risk. Nevertheless, Ayayi (Citation2012) argued that recruitment policy could increase the running costs which leads to increase the probability of loans losses. Therefore, the research hypothesis is formulated as follows:
H04: The number of employee staff has a significant and positive effect on portfolio at risk.
Operational efficiency has become an important element in the operational activities of MFI due to variability in running costs. Therefore, operating expenses ratio could lead to increase the degree of credit risk in MFIs. MFIs efficiency can increase the costs for cross pounding to increase their profit. Berger and De Young (Citation1997) stated that financial institution skimps to ensure high quality of loans. They found that operational costs have negative impact on credit risk. Similarly, Ayayi (Citation2012) confirmed that operational inefficiency has a negative influence of loan quality loans of portfolio for MFIs in Vietnam and East Asia. Teferi (Citation2019) revealed that operating expenses ratio is positively related to portfolio at risk in Ethiopia. Thus, the research hypothesis is formulated as follows:
H05: Operating expenses ratio has significant and positive effect on portfolio at risk.
In respect to micro-level institutional factors, Khan et al. (Citation2020) argued that number of active borrowers is main driver of poverty alleviation. This implies that more active borrowers tend to increase participation of poor households in financial activities of MFI. Thus, large number of borrowers offers a better breadth of outreach for MFI and serve more poor people (Schreiner, Citation2002). Therefore, Durango-Gutiérrez et al. (Citation2021) demonstrated the significant positive effect of number of loan applicant on portfolio at risk in Latin American countries. Yimga (Citation2016) argued MFI with more borrowers can effectively achieve the economics of scale and become more efficient in loan recovery. Silva et al. (Citation2015) revealed that active borrower is positively effect on portfolio at risk in Mexican MFIs. Similarly, Gonzalez (Citation2010) mentioned that growth in number of borrower has a positive influence on quality of loans in MFIs. Thus, the following research hypothesis can be formulated as follows:
H06: Number of borrowers has a significant and positive effect on portfolio at risk.
3. Methodology
Quantitative approach was used to examine the modeling the factors that effect on portfolio at risk for MFIs in Palestine. Therefore, this section presents the data collection, variable measurement and model specification.
3.1. Data and sample
This research utilizes data from MFIs that officially registered in Palestinian monetary authority (PMA) for the period 2010 to 2020. Thus, our sample consisted of only five MFIs (ASALA, VITAS, FATEN, ACAD, and REEF) of 8 MFIs that officially regulated by PMA. The chosen of MFIs and time period for this study are due to data availability. Moreover, data were collected from annual reports of MFIs and the macroeconomic data were collected from PMA database. As result, the total number of year firm’s observation is 55 of balanced panel data estimates.
3.2. Variables measurement
With regard to the dependent variable, the empirical literature always suggests portfolio at risk (Durango-Gutiérrez et al., Citation2021; De Oliveira, Citation2017; Silva et al., Citation2015). This variable measures MFI default loans overdue than 30 days and represents the credit risk of MFI (Crabb & Keller, Citation2006). As for independent variables, the current study uses macroeconomic and institutional factors. Hence, macroeconomic variables include; inflation (Bohachova, Citation2008), interest rate (Durango-Gutiérrez et al., Citation2021), and gross domestic product (Loannidou et al., Citation2022). For micro-level factors, we use number of employees in MFI (Wydick, Citation1999), operational efficiency (Ayayi, Citation2012) and number of active borrowers (Yimga, Citation2016). As illustrated in Table , the symbols, description, and expected directional relationship are listed.
Table 1. Variables measurement
3.3. Model specification
The research panel regressions were developed for examining the effect of some economic and institutional factors on PAR30 of MFIs in Palestine for the years 2010 to 2020. Hence, panel data estimates were deemed the most appropriate models to predict such relationship (Arellano, Citation2003). Thus, we employed ordinary least square (OLS), fixed effects, and random effects model to test the research hypotheses. In this sense, Lagrange multiplier test was used to determine the best out of OLS and fixed effects (Bjorken & Drell, Citation1965). However, Hausman statistic discerns the best out of the fixed effects and random effects regression results (Hausman, Citation1978).
The endogeneity is considered one of the most serious problems in panel data estimates, such as omitted variable bias and measurement errors. To overcome this problem, the researcher uses diagnostic tests to check normality, autocorrelation, and heteroscadisity among explanatory variables and to validate the panel data model. In this research, we use generalized method of moment (GMM) proposed by Blundell and Bond (Citation1998) to detect the endogeneity problem and to provide robust and realistic results (Alnabulsi et al., Citation2022; Hakimi et al., Citation2021; Khan et al., Citation2020).
For analyze panel data, various types of econometric models are available such as ordinary least square (OLS), fixed effects model (FEM), and random effect model (RFM). In this regard, OLS ignores the impact of individual MFIs and time with assumption that all individual MFIs are homogeneous (Gujarati & Dawan, Citation2009). Thus, this model as the same constant and coefficients in cross sectional and time series data. It can be simply formulated in Equationequation (1)(1)
(1) :
Fixed effects model allows different constants for individual MFIs with constant coefficients in Equationequation (2)(2)
(2) :
As for random effects model. It allows time variation to individual MFIs. Thus, this model has constant coefficients with random effect and the constant includes random component and formulated by Equationequation (3)(3)
(3) :
In this study, we examined the impact of various independent variables on portfolio at risk (PAR30) for panel data estimates. Hence, the residuals are correlated with time and individual MFIs and takes into account the heterogeneity among Palestinian MFIs. Therefore, we proposed following model specification in predicting portfolio at risk (PAR30):
where denotes the credit risk for MFI i (5 MFIs) in period t (11 years). t is the period from 2010 to 2020. I indicates the MFIs.
indicates the constant.
represents the inflation rate in Palestinian country in time t.
indicates the nominal interest rate of conventional banks in time t.
denotes the growth of gross domestic product for the time period t.
implies the number of employees staff for MFI in time t.
denotes the operational efficiency for MFI in time period t.
shows the number of active borrowers for MFI in time t. Meanwhile,
,
,
,
,
,
are coefficients of explanatory variables.
denotes the error term.
denotes the individual effect which is constant over time.
4. Results and discussion
4.1. Descriptive statistics and correlation
Table illustrates the average portfolio at risk over the last decade. The mean value of PAR30 is 7.2% and deviated by 5.5%. Moreover, inflation rate is at approximately average of 1% over last 10 years. The Palestinian commercial banks have provided loans at an average nominal interest rate of 6.1%. The average number of staffs in Palestinian MFIs is 80 employees and the mean value of operating expenses from assets is 13.3%, indicating that operational expenses are relatively low compared with total assets leads to higher level of operational efficiency. Meanwhile, the average number of active clients over the last 10 years is approximately 9270 borrowers with average gross loan portfolio of $26.3 million.
Table 2. Descriptive statistics and Pearson correlation
Table presents the correlation between each pair of variables. Thus, the results showed that inflation was positively correlated with the variables; interest rate (0.605), GDP (0.563), and expenses ratio (0.219). However, inflation was negatively related to the variables; personnel (−0.317) and active borrowers (−0.272). Nominal interest rate was positively correlated with GDP (0.607) and negatively with number of staff (−0.280). Number of staff was negatively correlated with the variables; GDP (−0.214) and expenses ratio (−0.481). Number of active borrowers was positively related to number of staff (0.588) and negatively with expenses ratio (−0.416). The highest correlated relationship was between GDP and interest rate (0.605). In this sense, all coefficients were below the absolute value of 0.8 (Walker & Madden, Citation2009). Therefore, This finding provides evident to the absence of multi-co-linearity situation.
As for portfolio at risk, the result showed that portfolio at risk was negatively correlated with the variables; GDP (−0.229) and number of active borrowers (−0.407) at significant of level 0.01. Nevertheless, portfolio at risk was positively related to the variables; number of staff (0.236) and expenses ratio (0.519) at significant level of 0.05.
Figure shows the average portfolio at risk over the last 10 years. This ratio has yearly fluctuated from 2010 to 2020. Therefore, there is an evidence of upward on mean value of PAR30 to 11.5% in 2020 compared with 9.67% of the base year 2010. However, the highest variability in PAR30 is in year of 2020. Hence, it observes that substantial increase in the level of credit risk in MFIs due to the pandemic of covid-19 that causes lockdown of all economic activities in worldwide as well as in Palestine. This ample that MFIs are unable to cushion the influence of corona crisis on their financial activities, especially in hedging from portfolio at risk (PAR30).
4.2. Panel unit roots and stationary
Table shows the results from testing the panel unit roots among data variables using Levin Lin Chu test (Chien-Fu Lin et al., Citation2002). Accordingly, the result of LLC shows that five variables including INF, I, GDP, Personnel, and OE/TA have stationary without explosive behavior (unit root) at the first difference. However, the variable PAR30 became stationary at 2nd difference with intercept. This result may be due to the lockdown of economic activities because of COVID-19 pandemic outreach. COVID-19 plays out in reality will vary by economy and rely on risk management strategy taken by each MFI. However, this indicates that COVID-19 is driven the loan quality of microfinance in Palestine. This may be provide a different level of stationary among explanatory variables. Thus, we proceeded to analysis the time series data based on 1st difference.
Table 3. Results of panel unit roots test (Levin Lin Chu test)
4.3. Optimal panel data model
Breusch-Pagan LM test was carried out to determine the appropriateness of the fixed effects model over than ordinary least square (Breusch & Pagan, Citation1979). The result (see, Table ) implies that p-value was less than significant level of 0.05, concluding that fixed effect is more appropriate than ordinary least square. Furthermore, Hausman test was used to ascertain the appropriateness of random effect or fixed effect in the estimated model (Hausman, Citation1978). Table shows that p-value is greater than 0.05, concluding that null hypothesis is accepted (H0: β1 = 0). As result, random effects model is more superior than fixed effects in conducting panel data estimates.
Table 4. Optimal panel data estimates
4.4. Residual diagnostic tests
Diagnostic tests were used to achieve the basic assumptions of panel data estimates (random effects model) and to ascertain whether panel data model could provide best unbiased estimated model. Therefore, normality, autocorrelation, and heteroskedasticity were tested to check the residual diagnostic among the explanatory variables (Pagan & Hall, Citation1983). Table also shows that a significant Jarque–Bera value of 9.24 and less than significant level of 0.05, which indicates panel data were not normally distributed. Wooldridge test was performed to check the autocorrelation in panel data (Wooldridge, Citation2002). The result indicates that p-value was greater than significant level of 0.05, concluding that error term of coefficients was smaller than actual values. This result implies that the residuals had no serial autocorrelation. Furthermore, Breusch Pagan test was tested to detect the randomness of the disturbance term (Breusch & Pagan, Citation1979). It indicates that p-value was less than significant level of 0.05. Thus, null hypothesis was accepted and disturbance term was not constant. Hence, the result confirmed the existence of heteroskedasticity errors among explanatory variables in random effect model. Thus, Generalized Method of Moments (GMM) was used to detect asymptotic normality and heteroskedasticity problems in panel data set (Hansen, Citation1982).
Table 5. Residual diagnostic tests of panel data estimates
4.5. Results of panel data estimates
Random effect model was deemed the best out model in conducting panel data regression. Nevertheless, the result found that data variables were not normally distributed and to the existence of heteroskedasticity. Therefore, robust option was adopted using panel generalized method of moments (GMM).
The result in Table shows that the dependent variable PAR30 is positively related to inflation rate but in significant effect. This means that the level of credit risk is effected by the change of inflation rate. This finding not support H01. This result is contrary with the finding of Crabb and Keller (Citation2006) who stated that higher inflation rate has negative effect on credit risk of MFIs.
Table 6. Panel data regression results
Similarly, the coefficient of interest rate is negative and statically in significant with PAR30. This argument not support H02, concluding that nominal interest on conventional loans will not spillover effect on credit risk of MFIs in Palestine. Thus, higher interest rates are not considered the initial symptom of portfolio at risk. In this sense, conventional banks that charge high interest rate on debts are not likely effect on quality of loans in MFIs. The reason is that poor people are not considered bank as alternative choice when they deciding to borrow from MFI. This finding is conflicted with previous studies (Loannidou et al., Citation2022; Cerqueiro et al., Citation2014) affirmed that conventional bank interest rate will increase the portfolio at risk in MFIs.
The level of portfolio at risk is found to be more sensitive to change in economic cycle. The coefficient of GDP is negative and significant with PAR30 as expected. Therefore, this finding supports hypothesis H03. This indicates that growth in GDP leads to decline the credit risk of MFIs in Palestine. A result, this study discloses that in stable economic cycle, active borrowers are able to repay their credits and MFIs are able to lower their portfolio at risk. This argument is consistent with finding be Koju et al. (Citation2020), who examines the macroeconomic factors on credit risk of high income countries. Nevertheless, This result is contrary with Kjosevski and Petkovski (Citation2017) who find a positive relationship between GDP growth and credit risk in Baltics states.
The effects of micro-level factors are in line the expectations. Regarding the factor Personnel, we observe that it was statically significant and displayed a positive sign in GMM model. This result supports H04, suggesting that the higher number of employees will expose MFI to high level of portfolio at risk. The higher number of staffs may actually make it more costly for MFI to operate in full capacity and negatively affect operational efficiency. As result, the policy of recruitment and higher level of employment erode the quality of loans. This conclusion was confirmed by Ayayi (Citation2012) who argues that the number of staffs is positively related to credit risk of Chinese MFIs. The current study provides evidence that personnel is considered an essential predictor of credit risk for MFIs in Palestine.
The coefficient of expenses ratio indicated that operational efficiency has a significant effect on PAR30. The positive relationship confirmed the hypothesis that MFI with higher expenses ratio takes higher level of credit risk. The result supports H05, which is consistent with empirical result of Teferi (Citation2019) who argued that operational inefficiency leads to deteriorate the loan quality of portfolio in Ethiopia. Favorable explanation is that microcredits with higher costs of fund, MFIs provide funds with higher interest charges. This action would increase the level of portfolio at risk.
As for number of borrowers, the result shows a negative and significant effect on PAR30 which supports H06. The negative relationship affirmed that the hypothesis with higher number of active clients takes low level of portfolio at risk. This means that higher demand on credits from poor people lead to decrease the level of PAR30. The favored interpretation is that providing more fund to poor households leads to lower poverty and achieve economic of scale which, in turn lower the level of portfolio at risk. This indicates that MFIs have selective criteria in choosing the high quality of active borrower in money market. This result is in line with the finding by Gonzalez (Citation2010) who finds that higher borrowers growth rate decreases the portfolio deterioration and improves quality of loan for MFIs. In the same manner. Yimga (Citation2016) argues that more clients from poor household can effectively achieve economic of scale and become more efficient in reducing none performing loans.
5. Conclusion and implications
Using GMM, this paper analyzes the impact of macroeconomic and micro-level variables of PAR30 for panel data from five regulated MFIs in Palestine over the period 2010 to 2020. Our results obtained highlight the influence of institutional factors on portfolio at risk and guide Palestinian MFIs to point out the importance of controlling risk for their quality loans of portfolio. We found that, from micro-level factors of our model, the number of staff, operational efficiency, and the number of active borrower have strongest effect of PAR30. The number of active borrowers is inversely related to portfolio at risk when collateral is pledged, credit risk is relatively lower when growth of active borrowers rate rises. MFIs directors should be oriented their loans quality toward proper credit policy to achieve lower written off ratio because the growth of funding poor households long thought to be major factor of loan delinquency. In addition, the results reveals that higher running costs could deteriorate loan portfolio quality and increase portfolio at risk. Moreover, the growth of GDP has negative effect on PAR30 in Palestine. The finding indicates PAR30 responds to macroeconomic conditions, such as GDP growth and there is a feedback effect from PAR30 on Palestinian economy. This concludes that the sound and resilience of microfinance system cannot be achieved without economic stability.
Our findings provides several implications in terms of MFIs credit policy and operational efficiency. First, MFIs directors should seek to hedge from credit risk on their loans and thus improve credit quality should pay attention to the institutional variables itself, specially the collateral provided, borrower creditworthiness, recruitment policy, and running costs. Second, operating costs reduction is greatly benefit for MFIs decisions during period of any potential credit crisis. These results have a diverse benefits for MFIs including; avoiding aggression in recruitment of loan officers and achieving sustainability in serving economic sectors. Third, Palestinian MFIs should use sold credit policy through types of collaterals provided in order to monitoring their credit risks. Fourth, operational efficiency is effective instrument for credit risk management, thus more effort and time should be spent on how MFIs effectively use their financial resources and which important sectors are served by MFIs. Fifth, it’s essential to for regulator establish credit rating system to mitigate credit risk and safeguard financial stability of MFIs.
Our crucial fact, one matter should be addressed to the Palestinian economy. This country faces high percentage of poverty and high unemployment rates in different economic sectors. Thus, MFIs in Palestine are not serving all poor households because most of poor people cannot recognize the living standards for obtaining financial support. Therefore, financial authorities in Palestine should develop a solid strategic plan for MFIs to determine the binding priorities of MFI in serving their programs and poor people. Two important parallel programs of families are necessary to develop their capabilities and manage their small projects. First program focuses on relief assistance for supporting health and education sectors. The second program focuses on economic empowerment projects for poor households in order to be survive (Migdad, Citation2022).
This study may motivate other researchers to further investigate the impact of corona various (COVID-19) on credit risk of microfinance institutions. Further research could extend the study objectives and include moderating factors, such as MFI size and age that influence on credit policy of MFIs. Further research may assess the social and financing efficiency of microfinance industry during the global crisis.
Disclosure statement
No potential conflict of interest was reported by the author.
Additional information
Funding
Notes on contributors
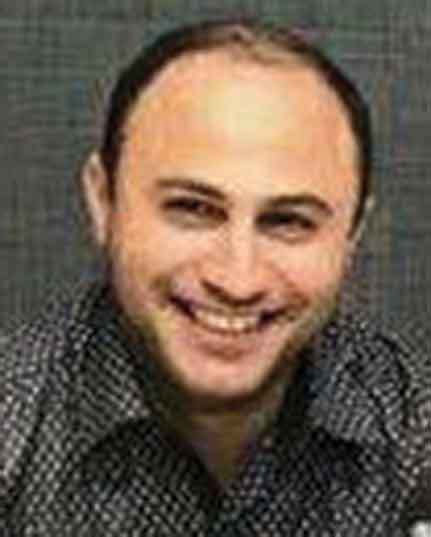
Mohammed T. Abusharbeh
Mohammed T. Abusharbeh is Associate professor of finance at Arab American university in Palestine. The author has more than 16 years teaching and research experience. He has published more than 20 full research article demonstrating on finance and banking fields. This study is conducted in augmenting the portfolio at risk in microfinance from emerging country perspective.
References
- Abusharbeh, M. (2020). Determinants of credit risk in Palestine: Panel data estimation. International Journal of Finance and Economics, 25(4), 1–14. https://doi.org/10.1002/ijfe.2329
- Agasha, E., Monametsi, G., & Feela, T. (2020). Loan portfolio quality of microfinance institutions in Uganda: A qualitative assessment. Journal of Financial Risk Management, 9(2), 155–177. https://doi.org/10.4236/jfrm.2020.92009
- Al-Bitawi, W. (2016). Outreach and efficiency of the microfinance institutions in the Palestinian economy. Master’s thesis, Faculty of graduate studies. Birzeit University.
- Alnabulsi, K., Kozarević, E., & Hakimi, A. (2022). Assessing the determinants of non-performing loans under financial crisis and health crisis: Evidence from the MENA banks. Cogent Economics & Finance, 10(1), 1–23. https://doi.org/10.1080/23322039.2022.2124665
- Arellano, M. (2003). Panel Data Econometrics. Oxford University Press.
- Ayayi, A. G. (2012). Credit risk assessment in the microfinance industry. Economics of Transition, 20(1), 37–72. https://doi.org/10.1111/j.1468-0351.2011.00429.x
- Berger, A., & De Young, R. (1997). Problem loans and cost efficiency in commercial banks. Journal of Banking & Finance, 21(6), 849–870. https://doi.org/10.1016/S0378-4266(97)00003-4
- Bjorken, J. D., & Drell, S. D. (1965). Relativistic Quantum Fields. McGraw-Hill.
- Blundell, R., & Bond, S. (1998). Initial conditions and moment restrictions in dynamic panel data models. Journal of Econometrics, 87(1), 115–143. https://doi.org/10.1016/S0304-4076(98)00009-8
- Bohachova, O. (2008). The impact of macroeconomic factors on risks in the banking sector: A cross-country empirical assessment. AW Diskussionspapiere, 44, Institut für Angewandte Wirtschaftsforschung (IAW).
- Breusch, T. S., & Pagan, A. R. (1979). A simple test for heteroskedasticity and random coefficient variation. Econometrica, 47(5), 1287–1294. https://doi.org/10.2307/1911963
- Cerqueiro, G., Ongena, S., & Roszbach, K. (2014). Collateralization, bank loan rates, and monitoring. The Journal of Finance, 71(3), 1295–1322. https://doi.org/10.1111/jofi.12214
- Chaibi, H., & Ftitit, Z. (2015). Credit risk determinants: Evidence from a cross-country study. Research in International Business and Finance, 33, 1–16. https://doi.org/10.1016/j.ribaf.2014.06.001
- Chien-Fu Lin, L., Lin, James Chu, Chia-shang, C.-F., & James Chu, C.-S. (2002). Unit root tests in panel data: Asymptotic and finite-sample properties. Journal of Econometrics, 108(1), 1–24. https://doi.org/10.1016/S0304-4076(01)00098-7
- Chikalipah, S. (2018). Credit risk in microfinance industry: Evidence from Sub-Saharan Africa. Review of Development Finance, 8(1), 38–48. https://doi.org/10.1016/j.rdf.2018.05.004
- Crabb, P., & Keller, T. (2006). A test of portfolio risk in microfinance institutions. Faith and Economics, 47, 25–39.
- De Oliveira, H. E. (2017). A different perspective on the debate between nonprofit and for-profit microfinance organizations. Honors Thesis Utah State University. Merrill-Cazier Library.
- Durango-Gutiérrez, M., Lara-Rubio, J., & Navarro-Galera, A. (2021). Analysis of default risk in microfinance institutions under the Basel III framework. International Journal of Finance and Economics, 1–18. https://doi.org/10.1002/ijfe.2475
- Gonzalez, A. (2010). Is Microfinance growing too fast?. MIX Data Brief No. 5. http://dx.doi.org/10.2139/ssrn.1644948
- Gujarati, D., & Poter‘s, D. (2009). Basic Economatrics (5th. ed) (pp. 944). McGraw-Hill Education. 0073375772.
- Hakimi, A., Boussaada, R., & Karmani, M. (2021). Are Financial Inclusion and Bank Stability Friends or Enemies? Evidence from MENA Banks. Applied Economics, 54(21), 2473–2489. https://doi.org/10.1080/00036846.2021.1992342
- Hansen, L. P. (1982). Large sample properties of Generalized method of moments estimators. Econometric, 50(4), 1029–1054. https://doi.org/10.2307/1912775
- Hausman, J. A. (1978). Specification tests in econometrics. Econometrica, 46(6), 1251–1271. https://doi.org/10.2307/1913827
- Inekwe, J. (2019). Lending risk in MFIs: The extreme bounds of microeconomic and macroeconomic factors. Journal of Small Business Management, 57(2), 538–558. https://doi.org/10.1111/jsbm.12401
- Kabir, M., Miah, M., & Rubaiya, H. (2022). Determinants of credit risk: A comparative analysis between Islamic and conventional banks in Bangladesh. The Singapore Economic Review, 67(1), 349–379. https://doi.org/10.1142/S0217590820420011
- Khan, A. A., Khan, S. U., Fahad, S., Ali, M. A. S., Khan, A., & Luo, J. (2020). Microfinance and poverty reduction: New evidence from Pakistan. International Journal of Finance and Economics, 1–11. https://doi.org/10.1002/ijfe.2038
- Kjosevski, M., & Petkovski, M. (2017). Non-performing loans in Baltic states: Determinants and macroeconomic effects. Baltic Journal of Economics, 17(1), 25–44. https://doi.org/10.1080/1406099X.2016.1246234
- Koju, L., Koju, R., & Wang, S. (2020). Macroeconomic determinants of credit risks: Evidence from high-income countries. European Journal of Management and Business Economics, 29(1), 41–53. https://doi.org/10.1108/EJMBE-02-2018-0032
- Lassoued, N. (2017). What drives credit risk of microfinance institutions? International evidence. International Journal of Managerial Finance, 13(5), 541–559. https://doi.org/10.1108/IJMF-03-2017-0042
- Loannidou, V., Pavanini, N., & Peng, Y. (2022). Collateral and asymmetric information in lending markets. Journal of Financial Economics, 144(1), 93–121. https://doi.org/10.1016/j.jfineco.2021.12.010
- Martino, P., & Sarsour, S. (2009). Microfinance in Palestine: Issues, performance, and trajectories, department of political economy and statistics. University of Siena.
- Migdad, A. (2022) Examining Islamic microfinance as a mechanism of Takaful in high-risk countries: Case study of Palestine. International Journal of Ethics and Systems. ahead-of-print No. ahead-of-print. https://doi.org/10.1108/IJOES-11-2021-0214.
- Morrar, R., Abdelrazq, O., Ghandour, R., Abu-Rmeileh, N. M., Forgione, D. A., & Younis, M. (2021). Financing development in Palestine through microfinance institutions and development banks: Options and challenges. Working paper. The Palestine Economic Policy Research Institute (MAS). Rammallah, Palestine.
- Naili, M., & Lahrichi, Y. 2022. The determinants of banks’ credit risk: Review of the literature and future research agenda. International Journal of Finance & Economics John Wiley & Sons, Ltd, 27(1), 334–360. https://doi.org/10.1002/ijfe.2156.
- Necesito, N. T. (2016). Testing the portfolio risk in Philippine microfinance institutions. Asia-Pacific Social Science Review, 16, 32–45.
- Ngonyani, D., & Mapesa, H. (2019). The effects of credit collection policy on portfolio at risk of microfinance institutions in Tanzania. Journal of Accounting, Finance and Auditing Studies, 5(1), 241–253. https://doi.org/10.32602/jafas.2019.12
- Nigmonoy, A., & Shams, S. (2021). COVID-19 pandemic risk and probability of loan default: Evidence from marketplace lending market. Financial Innovation, 7(83), 1–28. https://doi.org/10.1186/s40854-021-00300-x
- Pagan, A., & Hall, A. (1983). Diagnostic tests as residual analysis. Econometric Reviews, 2(2), 159–218. https://doi.org/10.1080/07311768308800039
- Robert, C., & Jonathan, M. (2017 doi:). Microfinance and economic development. World Bank Policy Research, Working Paper No. 8252.
- Safiullah, M., & Shamsuddin, A. (2018). Risk in Islamic banking and corporate governance. Pacific-Basin Finance Journal, 47(C), 129–149. https://doi.org/10.1016/j.pacfin.2017.12.008
- Schreiner, M. (2002). Aspects of outreach: A framework for the discussion of the social benefits of microfinance. Journal of International Development, 14(5), 591–603. https://doi.org/10.1002/jid.908
- Sifrain, R. (2022). Factors influencing loan portfolio quality of microfinance institutions in Haiti. Journal of Financial Risk Management, 11(1), 95–115. https://doi.org/10.4236/jfrm.2022.111005
- Silva, R., Cruz Aké, S., & Martínez, F. (2015). Differentiated determinants of risk in portfolio at risk of the microfinance institutions in Mexico (2007-2012). Contaduría y Administración, 60(1), 175–194. https://doi.org/10.1016/j.cya.2015.08.007
- Stiglitz, J., & Weiss, A. (1981). Credit rationing in markets with imperfect information. American Economic Review, 71(3), 393–410. http://www.jstor.org/stable/1802787
- Tabbaa, M. (2019). Micro-financing Palestine under a restricted regional economy. Master’s thesis, Harvard Extension School.
- Teferi, O. (2019). Determinants of microfinance institutions loan portfolios quality: Empirical evidence from Ethiopia. European Journal of Business and Management, 11(25), 15–25.
- Walker, J. T., & Madden, S. (2009). Statistics in criminology and criminal Justice, 3rd edition. Jones and Bartlett Publishers.
- Weber, R., & Musshoff, O. (2012). Is agricultural microcredit really more risky? Evidence from Tanzania. Agricultural Finance Review, 72(3), 416–435. https://doi.org/10.1108/00021461211277268
- Wiryono, S. K., & Effendi, K. A. (2018). Islamic bank credit risk: Macroeconomic and bank specific factors. European Research Studies Journal, 21(3), 53–62.
- Wooldridge, J. M. (2002). Econometric Analysis of Cross Section and Panel Data. MIT Press.
- World bank. (2020). Economic developments in the Palestinian territories. Publications.
- Wydick, B. (1999). Can social cohesion be harnessed to repair market failures? Evidence from group-based lending in Guatemala. Economic Journal, 109(457), 463–475. https://doi.org/10.1111/1468-0297.00457
- Yimga, J. (2016). The impact of high microfinance growth on loan portfolio. Journal of International Development, 28(5), 679–714. https://doi.org/10.1002/jid.3144