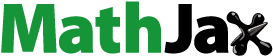
Abstract
The study investigates macroeconomic and bank-specific determinants of credit for commercial banks from 2010Q1–2022.Q4 in Indonesia. The banking credit is divided into an investment, working capital, and consumption credits. The study aims to address a gap in the literature since most prior studies are concentrated on developed markets. A system generalized method of moment (GMM) estimator is employed to investigate the impact of central bank rates and credit risk on credit. Dynamic-GMM estimations find that the central bank rate has a negative impact on the three types of credits. Meanwhile, non-performing loans positively impact investment and working capital credits but negatively affect consumption credit. The interaction between central bank rate and non-performing loans negatively impacts investment and working capital credits but positively affects consumption credit. This study provides managers and policymakers with timely information regarding bank credit drivers, encouraging management to take necessary measures, and policymakers may consider the importance of macroeconomic conditions while creating a bank lending policy. Similarly, it informs potential investors on how to evaluate the data when choosing a better investment option.
1. Introduction
Credit markets play a significant role in conveying the effects of monetary policy on the banking sector and the real economy. Monetary policy aims to maintain exchange and interest rate stability to achieve price stability, the balance of payments, and economic growth (Anwar & Suhendra, Citation2020; Dell’ariccia et al., Citation2018). According to Bernanke (Citation2020), in achieving the monetary policy objectives, there are several policy strategies, one of which is targeting carried out by determining bank credit. The central bank can manage the money supply through bank credit by setting the policy interest rate. This increased the interest rate, which greatly affected the distribution of credit (Arteta et al., Citation2018). Meanwhile, economists and policymakers argue that monetary policy works through interest rates (Bernanke, Citation2020). A tight monetary policy increases credit interest rates (Ranaldo et al., Citation2021) and reduces bank credit.
The credit channel illustrates that Central Bank policy changes affect the credit issued by commercial banks and influence the real economy (Anwar, Citation2022). According to Ivashina et al. (Citation2022), there are bank lending and company balance sheet credit channels. Theoretically, bank lending channels operate when the central bank takes tight monetary policy, reducing credit growth. In contrast, when the central bank loosens the monetary policy, bank loan growth can be increased (Naiborhu, Citation2020; Suhendra & Anwar, Citation2021). According to Jiménez et al. (Citation2020) and Albrizio et al. (Citation2020), bank credit channels are a mechanism that the central bank takes expansive monetary policy by lowering the interest rate. A decrease in central bank interest decreases loan rates and increases lending (Anwar, Citation2021; Naiborhu, Citation2020). According to the use of credit, bank credit is divided into investment, working capital, and consumption credits.
Until the global financial crisis of 2007–2008, the credit quality of lending portfolios in Indonesia was reasonably constant throughout the last decade. Average bank asset quality has dropped dramatically because of the worldwide economic downturn. However, the banks’ loan performance decline was unequal. The global financial crisis in 2007 is an example of a period that the banking system ignored the interaction between credit growth and risk (Allen & Gu, Citation2018; Chen et al., Citation2022; Kim et al., Citation2020). The expansion of subprime mortgage lending during this crisis, spurred by low-interest rates, growing property markets, and credit securitization, has resulted in enormous credit losses and significant implications for the global economy. This highlights the importance of the relationship between credit risk and bank loan growth (Saleh & Abu Afifa, Citation2020; Abdelaziz et al., Citation2022). Before the financial crisis, banks were more eager to extend loans, and since the crisis, they have curtailed loans in a credit crunch.
According to Hassan et al. (Citation2019) and Saleh and Abu Afifa (Citation2020), credit risk is the primary source of financial instability in the banking industry. The global financial crisis is the most recent illustration of inadequate credit risk management’s catastrophic economic implications. It is a significant cause of financial instability in the banking industry, according to Allen and Gu (Citation2018), Kim et al. (Citation2020), and Chen et al. (Citation2022). Furthermore, it has an effective management system, and a bank should effectively assess, monitor, and regulate credit risk. Appropriate credit risk measures provide a foundation for developing prudent supervision and control mechanisms. Several previous studies, such as Fahlenbrach et al. (Citation2018), Dang (Citation2019), Miyajima (Citation2020), and Jiménez (2020), showed that bigger loan losses result from quicker loan growth. Banks are more willing to repay debtors with poor credit records during a healthy business cycle with little collateral. Therefore, there is evidence that risk is linked to loan losses. A rise in non-performing loans (NPL) is predicted to reduce bank credit lines, leading to a negative link between NPL and loan growth rates.
The motivation of this study is an anomaly concerning the credit channel theory in the Indonesia case. It shows that the interest rates of the central bank are directly related to the supply of loans, and this is an anomaly between the real conditions and the existing theory. Furthermore, the previous study used total bank loans, which include consumer and real estate. The findings may be relevant to policymakers for two reasons, first, assessing overall asset quality and credit risk in the financial sector is a critical component of macroprudential monitoring. A solid knowledge of its causes allows the identification of important financial sector risks. Second, to provide standard scenarios for all financial institutions participating in such an exercise, regular stress tests of loan quality are increasingly based on macroeconomic assumptions. Loan quality stress testing was also an essential component of previously completed tests to rebuild trust in financial institutions.
In Indonesia, the credit situation appears to indicate monetary policy instrument imbalances, necessitating first determining the influence of these instruments and establishing the ideal monetary policy for the Central Bank of Indonesia to strive for when adopting its monetary policy stance. As a result, the purpose of this study is to investigate the Central Bank of Indonesia’s current monetary policy mix to see if it has an effect on the three forms of bank credit in Indonesia. There has been no prior research on the impact of monetary policy rates on three forms of bank loan in Indonesia.
As the researcher has highlighted and bridged the gaps existent in previous study, such as the absence of empirical evidence of the consequences of monetary policy on three forms of bank loan in Indonesia, this research is expected to make many additions to the body of literature. Very no empirical references or evidence were available to explain the association between monetary policy instruments and poor bank lending performance.
Monetary policy has different effects on bank loans in developed and developing nations. The monetary policy credit channel is extremely distinct and restrictive for developing nations such as Indonesia. This is mostly due to undeveloped financial systems, financial frictions, and structural rigidities in the system. The presence of sophisticated financial infrastructure in developed economies improves the efficacy of monetary policy transmission mechanisms to bank lending. Institutional restrictions impede financial intermediation and public policy efficacy in developing nations (Balogun, 2009; Modugu & Dempere, Citation2022). In addition to concentrated financial systems, a poor legal framework impedes monetary policy transmission (Mishra et al., Citation2012; Dang, Citation2019).
While more empirical attention has been paid to the relationship between monetary policies and bank credit in nation level data, the connection between monetary policy and bank credit at a country level data is still sparse. There is a notable shortage of empirical evidence in Indonesia. This study seeks to fill this gap by empirically investigating the monetary policy-bank lending nexus using evidence from Indonesia, where the impact of monetary policies on credit intermediation is critical to effective policy management, given the country’s desire to diversify its economic base and support growth through a private enterprise-led growth initiative. As a result, the study is useful in terms of providing empirical data that might influence strategies to improve monetary policy efficacy for growth.
2. Literature review
Models that relate monetary policy, credit risk, and bank credit are not new. Theoretical studies and monetary policy models that include the financial sector discover a relationship between central bank policy and lending. The traditional literature on the connections dates back to Bernanke, Gertler, and Gilchrist’s (1998) models. Anwar (Citation2022) proposed a framework that related the value changes of a credit portfolio to a dynamic global macro-econometric model and concluded that the relationship between monetary policy and credit risk is the primary driver of bank lending.
Theoretically, bank credit channel is a monetary policy transmission mechanism which illustrates that changes in Central Bank policies can affect the amount of credit issued by banks, which affect the real economy (Bernanke & Gertler, Citation1995). Bernanke & Gertler Citation1995 claims that the basically, theory of credit channels is divided into two, namely through bank lending channels and company balance sheet channels. Theoretically, bank lending channels can operate when the monetary authority is tightening, which will reduce loan growth. An easing monetary policy increases the growth of bank loans (Dlamini & Mashau, 2023). Bank lending channels are based on the assumption that banks play a special role in solving asymmetric information problems. Because of the special role of banks, certain borrowers will not have access to credit markets unless they borrow from banks. As long as there is no perfect substitution of retail bank deposits with other sources of funds, the bank’s lending channels of monetary transmission operate as follows. Expansionary monetary policy, which increases bank reserves and bank deposits, increases the amount of bank loans available. Since many borrowers depend on bank loans to finance their activities, an increase in these loans will cause investment and consumption spending to increase.
The empirical research on the interplay between monetary policy and credit risk is extensive and varied. The negative link between monetary policy and bank credit is a consistent result. However, the asset quality measures examined the differences in these articles. Most research relating credit risk to bank loans has used NPLs to measure asset quality. Ivanović (Citation2016) studied the determinants of credit growth in 11 banks in Montenegro from 2004 to 2014 using the Fixed Effect Model. It was shown that a higher central bank rate (CB Rate) reduces credit demand and decreases credit growth. Banks’ deposits and capital strength are directly related to credit growth. Meanwhile, the NPL and the credit growth are inversely proportional to one another. Al-Shammari and El-Sakka (Citation2018) investigated the determinants of credit growth in the private sector in organization for economic cooperation and development (OECD) countries, using Panel vector auto regressive (VAR) estimation for 24 countries and quarterly data from 2011 to 2013. The results showed that the central bank policy rate has a negative impact on bank credit. Other variables contributing to loans are exchange rates, consumer price index, deposit rates, non-resident liabilities, gross domestic bruto (GDP), and money supply.
The determinants of bank lending in Vietnam were analyzed using the fixed effect model and panel GMM estimation for the 37 commercial banks from 2006 to 2015. The central bank rate, size, expenditure costs, and inflation negatively affected credit. Meanwhile, capital adequacy ratio (CAR) and GDP have a significant positive effect on credit banks. Maiti et al. (Citation2020) investigated the impact of central bank interest rates on credit demand in Ghana after a period of financial liberalization, using the autoregressive distribution lag (ARDL) estimation from 1984 to 2017. They showed that central bank interest rates did not significantly affect credit, both in the short and long term. Therefore, the amount of funds borrowed does not depend on interest rates as long as the market is competitive and the cost of borrowing reflects the current state of the economy.
Naiborhu (Citation2020) evaluated the path of bank lending from monetary policy using a panel data estimation for quarterly datasets from 2005 to 2016 for 117 conventional commercial banks. The capital buffer and liquidity ratio have an important role in the impact of changes in monetary policy on credit growth in the large bank category. Meanwhile, the macroprudential policy has a positive influence on credit growth. It is different from the reference interest rate, exchange rate, GDP, bank asset ratios, and NPL, which negatively influence credit growth. Oyebowale (Citation2020) empirically proved the determinants of bank loans in Nigeria using ARDL estimation from 1961 to 2016. The results show that central bank interest rates, loan to deposit (LDR), and money increment negatively and positively affect credit growth in the short and long term.
Shokr (Citation2020) reviewed the monetary policy on bank loans in Egypt, using GMM estimation for 34 commercial banks from 1996 to 2014. The results of this study are that the monetary policy reference rate negatively affects bank loans in Egypt. Meanwhile, net bank income, interest rates, total assets, and bank size significantly affect bank loans. Miyajima (Citation2020) investigated the determinants of credit growth in Saudi Arabia using ten banks during the period 2000 to 2015 by using the fixed effect and panel GMM estimations. Capital ratios, deposit growth, oil price growth, GDP, and excess liquidity significantly positively affect credit growth in Saudi Arabia. In contrast, central bank interest rates, NPL, and the FED interest rate have a significant negative effect.
Sabri (Citation2021) studied how monetary policy influenced the promotion of bank credit for economic development by creating jobs and overcoming unemployment. The ARDL estimation was used for the period 2003 to 2019. The results show that central bank interest and inflation rates have a negative impact on credit. However, the money supply and the exchange rate have no effect on total credit in Iraq. Nguyen and Dang (Citation2021) used the general least squares (GLS) method for 27 commercial banks in Vietnam from 2008 to 2018. They found that central bank interest rates, size, capital, NPL, inflation, and foreign direct investment (FDI) significantly negatively affect credit growth, while liquidity, NPL, and return on asset (ROA) have a positive effect.
Modugu and Dempere (Citation2022) examined monetary policy and bank lending channels in emerging markets using GMM estimation for 80 banks in the Sub-Saharan Africa area from 2010 to 2019. They found that money supply, CAR, and GDP positively affect bank loans. Meanwhile, central bank interest rates and NPL have a significant negative effect. Suhendra and Anwar (Citation2021) examined the effect of the central bank policy rate on credit growth for quarterly data for the period 1976 Q1 to 2019 Q4 using a smooth transition regression approach. They proved the non-linear relationship between the central bank rate and credit growth. According to the findings, the Central Bank policy rate threshold level is 2.1036. A fall in the Central Bank rate reduces the credit gap above or below the threshold level. The exchange rate significantly influences the credit gap, with positive and negative directions for the high and low regimes. However, in the high regime, the impacts of economic growth, inflation, and money per GDP on the credit gap are negative but positive in the low regime.
This adds to the existing research on the empirical determinants of loan behavior by utilizing commercial banks’ data samples. Utilizing cross-firm variance in bank credit patterns is likely to give more robust results than an investigation of individual banks because time series for credit are often short, comprising at most 10 years of yearly data. At the same time, while studies based on national-level data are highly informative in a macro-level setting, they are only accessible to a few economies. Therefore, it is impossible to study the influence of monetary policy on individual commercial bank disparities in structural features on bank loans.
3. Data dan methodology
3.1. Data
The dependent variable is bank credit, divided into investment, working capital, and consumption. Investment credit is a facility provided to finance the need for capital goods in rehabilitation, modernization, expansion, the establishment of new projects, and special needs related to firm investment. Working capital credit is short-term and can increase production in its operations. Meanwhile, consumption credit includes mortgage and motor vehicle loans.
The independent variables are the central bank policy rate as a proxy from Bank Indonesia, and NPL is the ratio of unpaid credit. Control variables can be added from macro and bank-level data, including inflation, exchange rate, CAR, and LDR. Furthermore, 41 commercial banks are listed on the Indonesia stock exchange from 2010Q1 to 2022Q4. The definition of variables and the descriptive statistic are presented in Tables .
Table 1. Variable Definitions
Table 2. Descriptive Statistic
The investment credit, working capital credit, consumption credit, and exchange rate are in term of logarithm. While, the central bank rate, NPL, inflation, CAR, and LDR are in term of percentage.
Figure shows the trend of central bank policy rate from 2010 to 2022. It is showed clearly that the trend of central bank policy rate was fluctuation. From 2010 to 2012, the trend decreased since Bank Indonesia applied loosening monetary policy. However, from 2015 to 2015, Bank Indonesia set a tight monetary policy by increasing the policy rate and reached the highest at 2014. In 2017, the policy rate experienced the lowest, but then increased again in 2018. The COVID-19 pandemic pushed the central bank to reduces the policy rate to around 4%. However, the policy rate experienced an increased in the end 2022.
Figure shows the trend of three bank credits, investment, working capital, and consumption credits from the period 2010 to 2022. It can be seen that the trend of investment credit, showed very volatile trend, which increased in the period from 2010 to 2014 but decreased significantly in 2016. In the following period the investment credit continued to show fluctuating until the final period, the highest peak of investment credit was in the period 2018 to 2020. Furthermore, working capital credit experienced an increase from 2010 to 2020, in which there has not been a significant decline. However, from 2020 to 2022, there is a drastic reduction in working capital credit. The trend in the consumption credit showed a decline in the initial period but showed an increase from 2012 to 2020. There was a decrease in the consumption credit in 2021, but it increased at the end of the 2022.
3.2. Econometrics methodology
Macro and micro-level data are considered when estimating the effect of monetary policy and credit risk on bank credit. Panel regression is applied with data for 2010.Q1–2022.Q4 to test the effect of monetary policy and credit risk on bank credit. Furthermore, inflation, the exchange rate, capital adequacy ratio, and credit-to-deposit ratio are control variables affecting bank credit as a determinant of financial stability. Models 1 and 2 show the effect of monetary policy and credit risk on bank credit. Meanwhile, model 3 estimates the individual effects of monetary policy and credit risk on bank credit. The interaction between monetary policy and credit risk is included in Model 4.
Maiti et al. (Citation2020), Naiborhu (Citation2020), Shokr (Citation2020) showed that CAR and LDR are the determining factors in lending banks. According to Oyebowale (Citation2020) and Sabri (Citation2021), inflation is another factor that can affect credit distribution from a macro perspective. Finally, Naiborhu (Citation2020) stated that the exchange rate influences lending.
For investment credit, since period t investment credit is also influenced by t-1, the rate becomes dynamic:
Where INV credit is an investment credit, CB is the central bank policy rate as a proxy of monetary policy. NPL is a non-performing loan as a proxy of credit risk. Inf, ER, CAR, and LDR are inflation, exchange rate, capital adequacy ratio, and loan-to-deposit ratio. i is bank cross section. t is period (quarterly). The same process is applied for working capital and consumption credits.
An endogeneity test was performed to check the consistency of the panel ordinary least square (POLS) models’ findings. Individual regressor endogeneity is detected using the Durbin-Wu-Hausman test. In theory, the explanatory variable should be uncorrelated with the error term, and this test examines when the residuals are associated with the explanatory variable. To detect endogeneity in the ordinary least square (OLS) regression, a Durbin-Wu-Hausman test is performed.
Estimation using the POLS method can produce an inconsistent and well-correlated estimator. Therefore, the transformation through this difference can use an instrumental variable approach as an instrument. The First different GMM (FD-GMM) estimator can be constrained by limited sample bias (Blundell & Bond, Citation1998). The existence of restricted sample bias can be detected by comparing the FD-GMM results with alternative estimators of the autoregressive parameters. In AR(1), pooled least squares can give an estimate with an upward bias in the presence of individual-specific effects. Meanwhile, the fixed effect provides predictions with a downward bias, and consistent estimators can be expected between pooled least square or fixed effect. The FD-GMM estimator will be biased downward, which can be caused by a weak instrument when close to or below the fixed effect.
Endogeneity problems can produce biased and inconsistent estimators when there is a lag in the dependent variable. Arellano and Bond (Citation1991) opined that additional instruments could be obtained in the dynamic panel data model when using the orthogonality condition, which exists between the lag value and disturbance
. Blundell and Bond (Citation1998) stated the importance of utilizing initial conditions in producing an efficient estimator from a dynamic panel data model when T is small. Meanwhile, SYS-GMM can overcome the problem of weak instruments in the FD-GMM estimator. Blundell and Bond (Citation1998) attributed the bias and poor precision of the estimator to a weak instrument characterized by its concentration of parameters. The additional light stationarity restrictions on the initial condition process allow the estimator to be extended using the difference lag as the instrument for the equations. The GMM model has criteria for the best model, namely the sargan and the Arellano-bond tests.
4. Results
4.1. Unit root test
Table represents the result of the panel unit root test at level. The result illustrated that the null hypothesis is rejected for all variables at the 5% level of significance. Rejecting the null hypothesis means that those variables are I (0).
Table 3. Panel Unit Root Tests
4.2. Basic OLS estimation
Simple POLS and fixed effect estimations are utilized, but it is assumed that the findings may be biased due to endogeneity. For instance, NPLs may increase in banks during periods of stagnant economic activity and low credit demand, leading to a negative correlation that may be insignificant. Additionally, bank credit may increase NPL when market discipline is undermined during a financial crisis, allowing banks to be supervised less and decreasing lending standards. Omitted variable bias is another potential cause of endogeneity, as all factors of bank loans cannot be controlled. To address endogeneity issues, independent variables in OLS and fixed effects estimates are lagged by one period.
Table presents the results of the POLS Estimation, showing that a lag of 1 in investment credit has a positive significance on investment credit. The CB Rate and NPL have no significant negative effect on investment, working capital, and consumption credits. Furthermore, the CAR variable negatively affects the three types of credit, while inflation has no significant impact. The exchange rate positively affects investment and working capital credits, while the consumption credit is positive but insignificant. For investment credit, working capital, and consumption credits, LDR has no significant positive effect, no significant negative effect, and a significant negative effect, respectively.
Table 4. OLS Estimation
4.3. Fixed effect estimation
The Fixed Effect Estimation results are presented in Table , where lag 1 of investment, working capital, and consumption credits has a positive significance on the variables. The CB Rate and NPL partially and negatively affect the three variables. However, the CB rate and NPL significantly and positively affect investment, working capital, and consumption credits. The Exchange Rate and LDR significantly and positively affect the three variables. Meanwhile, CAR negatively affects investment, working capital, and consumption credits. The Inflation variable has no positive effect on the investment, working capital credits, and consumption credits.
Table 5. Endogeneity Test for Investment Credit
4.4. Endogeneity test
Under the presumptions of exogeneity, this study conducted an endogeneity test, as presented in Table The Durbin Wu- Hausman test was performed on models 1 through 4, where the results showed the probability for each group is 0.000, and the null hypothesis was rejected. Therefore, it was concluded that the panel data have an endogeneity problem.
4.5. GMM estimation
4.5.1. First different GMM
Tables present the results of the FD-GMM estimation. These results indicate that the CB rate negatively affects investment, working capital, and consumption credits. The NPL has a significant positive effect on investment and working capital credits but a negative effect on consumption. However, the interaction effect between CB Rate and NPL significantly positively affects investment and consumption. Inflation has a significant positive effect on investment and working capital credits but a significant negative effect on consumption credit. Furthermore, the exchange rate and CAR positively and negatively affect the three types of credits. The last variable, LDR, has a significant positive effect on working capital credit but a negative effect on investment and consumption credits.
Table 6. Fixed Effect Estimation
Table 7. First Difference GMM Estimation
4.5.2. System GMM
Table shows the results of the Panel System GMM Estimation. CB Rate negatively affects investment, working capital, and consumption credits. NPL has a significant positive effect on investment and working capital credits but a negative effect on working capital credit. A significant positive effect was reported for the interaction between CB Rate and NPL on investment and consumption credits. Furthermore, inflation and LDR have a significant positive effect on the three types of credits. The exchange rate has a significant negative effect on investment credit but a positive effect on working capital and consumption. CAR has a significant positive effect on investment and consumption credits but a negative effect on working capital.
Table 8. System GMM Estimation
5. Discussion
The CB Rate as a reference for commercial banks in distributing credit has a negative and significant effect on the three types of credit. The results are supported by Suhendra and Anwar (Citation2021), Naiborhu (Citation2020), Shork (2020), Oyebowale (Citation2020), Al-Shammari and El-Sakka (Citation2018), Vo (Citation2018), and Ivanovic (2016), where the central bank’s interest rate is directly proportional to the loan growth. The results from Naiborhu (Citation2020) stated that the CB Rate negatively affects bank credit due to tightening monetary policy limiting banking activities. This indicates that the influence of monetary policy transmission on lending channels functions well in responding to changes.
The monetary policy affects commercial banks’ ability to channel credit (Iddrisu & Alagidede, Citation2020). The adoption of expansive monetary policies, such as lowering the CB Rate, prompts commercial banks to adjust their balance sheets by reducing lending rates and increasing credit.
The biggest effect of the CB Rate on three types of credit is for investment loans compared to working capital and consumption. Investment credit is used for expanding business or building new projects, where the usage period is longer. High investment also causes increased employment absorption. The magnitude of the impact on investment credits shows Indonesia has large prospects. This is reflected in many new investments because investors are still optimistic about the economy in the medium and long term. Furthermore, banks obtain low-risk and high returns in extending credit in the investment sector.
NPL has a negative effect on consumption and investment credits. This result is under the credit theory of money (Innes, Citation2004) and is also supported by a study by Ivanovic (2016) and Naiborhu (Citation2020), meaning that an increase in NPL decreases distribution credit. NPL is the ratio of the quality of bank lending, describing the bank’s credit risk. The higher the ratio, the less credit will be disbursed, and the lower the ratio, the better the loan disbursement (Alessi & Detken, Citation2018). The rationing theory developed by (Jafee & Modigliani, 1969) argued that credit risk can occur in the banking sector. Therefore, it is crucial to maintain quality by preventing the occurrence of bad credit at the bank to mitigate risk. Banks’ pursuit of greater profits through raising lending rates poses a risk to non-performing loans (NPL) as it may become difficult for borrowers to repay the principal loan and the increased interest. The NPL is inversely related to the bank’s profits and can reduce the amount of credit extended.
However, the impact of NPL on working capital credit is positive. This finding is inconsistent with the theory, where NPL during the study does not exceed the maximum limit set by Bank Indonesia, namely 5%. The results are similar to those of Nguyen and Dang (Citation2021) and Modugu and Dempere (Citation2022), who showed that NPL positively affects bank lending. The positive effect on working capital credit might be caused by the relatively low NPL of commercial banks below 2%.
The above results found a contrasting effect of CB Rate and NPL on investment and working capital credits, which supported its substitution. This outcome implies that a higher CB Rate with a lower NPL can reduce investment and working capital credits. This result was in line with Samba and Mbassi (2016) and Karim et al. (2021), where the effect of CB Rate and NPL on bank credit was a substitute.
The interaction coefficient between CB Rate and NPL reported in Model (4) indicated that the interaction term was statistically significant and adversely influenced investment and working capital credits. The negative coefficient estimates implied that CB Rate and NPL are substitutes for lowering investment and working capital credits. Therefore, when CB Rate is high, lowering NPL has a bigger impact on investment and working capital credits. This finding was in line with the result to support the substitution effect of CB Rate and NPL on bank credit.
It is critical to evaluate whether the negative impact of central bank rates seen is the result of a shift in supply or demand of credit. In this study, we follow Modugu and Dempere (Citation2022), Dlamini and Mashau (2023), and Naqvi and Pungaliya (2023) to see if the impact of monetary policy on three types of bank credit varies by bank. Table shows the heterogeneous impact on various banks of interacting with monetary policy on three types of bank credit characteristics using system GMM estimation. The results show that the interaction term between the central bank policy rate and credit risk is positively significant for consumption credit, but it is negatively significant for investment and working capital credit. This implies that the negative impact of central bank rates on bank lending via credit risk is less pronounced in investment and working capital credits. This result suggests that when monetary policy is tight, investment and working capital credits are involved in high-risk credit activities, resulting in less bank lending. A tight monetary policy with a high credit risk, on the other hand, leads to more bank lending for consumption credit.
However, previous research on the influence of credit risk in central bank policy rates on bank loans yielded inconsistent results. According to Samba and Mbassi (2016) and Karim et al. (2021), credit risk supports the negative influence of central bank policy rates on bank loans. While Nguyen and Dang (Citation2021) and Modugu and Dempere (Citation2022) find the reverse, they hypothesise that the presence of credit risk enhances the favourable effect of central bank policy rates on bank loans.
The results found a similar effect of CB Rate and NPL on consumption credit, which supported its complementary. This outcome implies that a higher CB Rate and NPL would increase consumption credit. The coefficients on the interaction terms are positive and statistically significant, and the magnitudes of the coefficients are sufficiently large to suggest that most or all of the adverse effect of a change in CB rate is offset.
Inflation positively affects investment, working capital, and consumption credits. This means that an increase in inflation directly affects bank credits. The results are supported by prior research such as Ivanovic (2016), De Leon (Citation2020), and Molyneux et al. (Citation2020). The positive effect of inflation on bank credit is that the amount of money influences the variable in the economy. The exchange rate positively affects the disbursement of consumption and investment credits, meaning that any increase or weakening of the exchange rate can increase the distribution. However, this finding is in line with Wei and Han (Citation2021) and Suhendra and Anwar (Citation2021) regarding the positive effect of the exchange rate on bank credit. CAR positively affects investment, working capital, and consumption credits. This means that an increase in CAR increases bank credit. This finding is in line with the result of Saleh and Abu Afifa Citation2020 and De Marco et al. (Citation2021) for the positive effect of CAR on bank credit. Finally, the LDR positively affects investment, working capital, and consumption credits. This means that every increase in LDR directly affects bank lending, which is in line with the prior study of Boďa, M., & Zimková, E. (Citation2021) and Karamoy and Tulung (Citation2020).
6. Conclusion
The study assesses the impact of central bank policy rates and credit risk on investment, working capital, and consumption credits. It fills the research gap by attempting to answer the effects of the central bank policy rate, credit risk, and the interaction between variables on bank credit behavior in Indonesia. Furthermore, four models were created and a panel data estimation was performed to achieve the objective. A POLS estimation was run for the four models and found that the CB Rate has no significant negative effect on investment, working capital, and consumption. It was determined that NPL negatively and significantly impacts the three variables, while CB Rate and NPL had no significant negative effect. Subsequently, a Durbin Wu-Hausman test was performed to verify the endogeneity problem of the models. A solution for this issue was proposed, which is performing an instrumental variable estimation.
Using dynamic-GMM estimations, CB Rate has a negative impact on the three types of bank credits. Meanwhile, NPL has a positive impact on investment and working capital but a negative on consumption. The interaction between CB Rate and NPL negatively impacts investment and working capital credits but positively impacts consumption.
Based on the conclusion, the following recommendations are suggested. This study helps management take corrective actions, and policymakers may consider the significance of macroeconomic conditions while formulating policies regarding bank credit. Second, there is evidence that an expansionary monetary policy characterised by a decrease in the central bank policy rate encourages three forms of bank lending. Monetary authorities in Indonesia can thus rely on the monetary transmission mechanisms of changes in central bank policy rates to impact bank credit and real sector economic activity. Third, the data indicate that a high credit risk inhibits banks from lending for consumer credit rather than investment or working capital credit. As a result, banks in Indonesia should check credit risk by only lending to low-risk clients.
Fourth, empirical evidence suggests that inflation and the currency rate are important stimuli for bank lending. In order to produce more stable real business activity, Indonesian policymakers should regulate inflation and the currency rate. Fifth, the empirical findings indicate that a high capital adequacy ratio and loan to deposit ratio are major determinants of bank lending in Indonesia. As a result, effective policy regulation and supervision of banks to achieve the minimum capital adequacy ratio and loan to deposit ratio are critical to ensuring banks’ safety and viability to carry out efficient financial intermediation.
Disclosure statement
No potential conflict of interest was reported by the authors.
References
- Abdelaziz, H., Rim, B., & Helmi, H. (2022). The interactional relationships between credit risk, liquidity risk, and bank profitability in MENA region. Global Business Review, 23(3), 561–18. https://doi.org/10.1177/0972150919879304
- Albrizio, S., Choi, S., Furceri, D., & Yoon, C. (2020). International bank lending channel of monetary policy. Journal of International Money & Finance, 102, 102124. https://doi.org/10.1016/j.jimonfin.2019.102124
- Alessi, L., & Detken, C. (2018). Identifying excessive credit growth and leverage. Journal of Financial Stability, 35, 215–225. https://doi.org/10.1016/j.jfs.2017.06.005
- Allen, F., & Gu, X. (2018). The interplay between regulations and financial stability. Journal of Financial Services Research, 53(2), 233–248. https://doi.org/10.1007/s10693-018-0296-7
- Al-Shammari, N., & El-Sakka, M. (2018). Macroeconomic determinants of credit growth in OECD Countries. International Journal of Business, 23(3), 217–234. https://ijb.cyut.edu.tw/var/file/10/1010/img/864/V233-1.pdf
- Anwar, C. J. (2021). Heterogeneity effect of central bank independence on asset prices: Evidence from selected developing countries. Jurnal Ekonomi Malaysia, 55(2), 65–80. http://dx.doi.org/10.17576/JEM-2021-5502-6
- Anwar, C. J. (2022). Heterogeneity effect of central bank independence on inflation in developing countries. Global Journal of Emerging Market Economies, 15(1), 38–52. https://doi.org/10.1177/09749101221082049
- Anwar, C. J., & Suhendra, I. (2020). Monetary policy independence and bond yield in developing countries. The Journal of Asian Finance, Economics & Business, 7(11), 23–31. https://doi.org/10.13106/jafeb.2020.vol7.no11.023
- Arellano, M., & Bond, S. (1991). Some tests of specification for panel data: Monte Carlo evidence and an application to employment equations. The Review of Economic Studies, 58(2), 277–297. https://doi.org/10.2307/2297968
- Arteta, C., Kose, M. A., Stocker, M., & Taskin, T. (2018). Implications of negative interest rate policies: An early assessment. Pacific Economic Review, 23(1), 8–26. https://doi.org/10.1111/1468-0106.12249
- Bernanke, B. S. (2020). The new tools of monetary policy. The American Economic Review, 110(4), 943–983. https://doi.org/10.1257/aer.110.4.943
- Bernanke, B. S., & Gertler, M. (1995). Inside the black box: the credit channel of monetary policy transmission. Journal of Economic Perspectives, 9(4), 27–48. https://doi.org/10.1257/jep.9.4.27
- Blundell, R., & Bond, S. (1998). Initial conditions and moment restrictions in dynamic panel data models. Journal of Econometrics, 87(1), 115–143. https://doi.org/10.1016/S0304-4076(98)00009-8
- Boďa, M., & Zimková, E. (2021). Overcoming the loan-to-deposit ratio by a financial intermediation measure—A perspective instrument of financial stability policy. Journal of Policy Modeling, 43(5), 1051–1069. https://doi.org/10.1016/j.jpolmod.2021.03.012
- Chen, T. H., Lee, C. C., & Shen, C. H. (2022). Liquidity indicators, early warning signals in banks, and financial crises. The North American Journal of Economics & Finance, 62, 101732. https://doi.org/10.1016/j.najef.2022.101732
- Dang, V. (2019). The effects of loan growth on bank performance: Evidence from Vietnam. Management Science Letters, 9(6), 899–910. https://doi.org/10.5267/j.msl.2019.2.012
- De Leon, M. (2020). The impact of credit risk and macroeconomic factors on profitability: The case of the ASEAN banks. Banks and Bank Systems, 15(1), 21–29. https://doi.org/10.21511/bbs.15(1).2020.03
- Dell’ariccia, G., Rabanal, P., & Sandri, D. (2018). Unconventional monetary policies in the euro area, Japan, and the United Kingdom. Journal of Economic Perspectives, 32(4), 147–172. https://doi.org/10.1257/jep.32.4.147
- De Marco, F., Kneer, C., & Wieladek, T. (2021). The real effects of capital requirements and monetary policy: Evidence from the United Kingdom. Journal of Banking and Finance, 133, 106237. https://doi.org/10.1016/j.jbankfin.2021.106237
- Fahlenbrach, R., Prilmeier, R., & Stulz, R. M. (2018). Why does fast loan growth predict poor performance for banks? The Review of Financial Studies, 31(3), 1014–1063. https://doi.org/10.1093/rfs/hhx109
- Hassan, M. K., Khan, A., & Paltrinieri, A. (2019). Liquidity risk, credit risk and stability in Islamic and conventional banks. Research in International Business and Finance, 48, 17–31. https://doi.org/10.1016/j.ribaf.2018.10.006
- Iddrisu, A. A., & Alagidede, I. P. (2020). Revisiting interest rate and lending channels of monetary policy transmission in the light of theoretical prescriptions. Central Bank Review, 20(4), 183–192. https://doi.org/10.1016/j.cbrev.2020.09.002
- Innes, A. M. (2004). Credit and State Theories of Money, Cheltenham, Edward Elgar (pp. 50–78). https://doi.org/10.4337/9781843769842
- Ivanović, M. (2016). Determinants of credit growth: The case of Montenegro. Journal of Central Banking Theory and Practice, 5(2), 101–118. https://doi.org/10.1515/jcbtp-2016-0013
- Ivashina, V., Laeven, L., & Moral Benito, E. (2022). Loan types and the bank lending channel. Journal of Monetary Economics, 126, 171–187. https://doi.org/10.1016/j.jmoneco.2021.11.006
- Jiménez, G., Mian, A., Peydró, J. L., & Saurina, J. (2020). The real effects of the bank lending channel. Journal of Monetary Economics, 115, 162–179. https://doi.org/10.1016/j.jmoneco.2019.06.002
- Karamoy, H., & Tulung, J. E. (2020). The impact of banking risk on regional development banks in Indonesia. 15(2), 130–137. https://doi.org/10.21511/bbs.15(2).2020.12
- Kim, H., Batten, J. A., & Ryu, D. (2020). Financial crisis, bank diversification, and financial stability: OECD countries. International Review of Economics & Finance, 65, 94–104. https://doi.org/10.1016/j.iref.2019.08.009
- Maiti, M., Esson, I. A., & Vuković, D. (2020). The impact of interest rate on the demand for credit in Ghana. Journal of Public Affairs, 20(3), e2098. https://doi.org/10.1002/pa.2098
- Mishra, P., Montiel, P. J., & Spilimbergo, A. (2012). Monetary transmission in low-income countries: effectiveness and policy implications. IMF Economic Review, 60(2), 270–302. https://doi.org/10.1057/imfer.2012.7
- Miyajima, K. (2020). What influences bank lending in Saudi Arabia? Islamic Economic Studies, 27(2), 125–155. https://doi.org/10.1108/IES-07-2019-0018
- Modugu, K. P., & Dempere, J. (2022). Monetary policies and bank lending in developing countries: Evidence from Sub-Sahara Africa. Journal of Economics and Development, 24(3), 217–229. https://doi.org/10.1108/JED-09-2021-0144
- Molyneux, P., Reghezza, A., Thornton, J., & Xie, R. (2020). Did negative interest rates improve bank lending? Journal of Financial Services Research, 57(1), 51–68. https://doi.org/10.1007/s10693-019-00322-8
- Naiborhu, E. D. (2020). The lending channel of monetary policy in Indonesia. Journal of Asian Economics, 67, 101175. https://doi.org/10.1016/j.asieco.2020.101175
- Nguyen, T. T., & Dang, T. V., 2021, Credit growth determinants—the case of vietnamese commercial banks. In Data Science for Financial Econometrics (pp. 567–582). Springer. https://doi.org/10.1007/978-3-030-48853-6_38
- Oyebowale, A. Y. (2020). Determinants of bank lending in Nigeria. Global Journal of Emerging Market Economies, 12(3), 378–398. https://doi.org/10.1177/0974910120961573
- Ranaldo, A., Schaffner, P., & Vasios, M. (2021). Regulatory effects on short-term interest rates. Journal of Financial Economics, 141(2), 750–770. https://doi.org/10.1016/j.jfineco.2021.04.016
- Sabri, M. M. (2021). The role of monetary policy in activating the bank credit channel: Iraq case study. Revista Gestão Inovação e Tecnologias, 11(4), 4990–5009. https://doi.org/10.47059/revistageintec.v11i4.2517
- Saleh, I., & Abu Afifa, M. (2020). The effect of credit risk, liquidity risk and bank capital on bank profitability: Evidence from an emerging market. Cogent Economics & Finance, 8(1), 1814509. https://doi.org/10.1080/23322039.2020.1814509
- Shokr, M. A. (2020). Real interest rate, income and bank loans: Panel evidence from Egypt. Journal of Financial Economic Policy, 12(2), 227–243. https://doi.org/10.1108/JFEP-09-2018-0140
- Suhendra, I., & Anwar, C. J. (2021). The role of central bank rate on credit gap in Indonesia: A smooth transition regression approach. The Journal of Asian Finance, Economics & Business, 8(1), 833–840. https://doi.org/10.13106/jafeb
- Vo, X. V. (2018). Bank lending behavior in emerging markets. Finance Research Letters, 27, 129–134. https://doi.org/10.1016/j.frl.2018.02.011
- Wei, X., & Han, L. (2021). The impact of COVID-19 pandemic on transmission of monetary policy to financial markets. International Review of Financial Analysis, 74, 101705. https://doi.org/10.1016/j.irfa.2021.101705