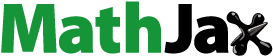
Abstract
Using appropriate agricultural mechanization services is critical to increasing agricultural productivity and ensuring food security. A large portion of Ethiopian farmers have no access to agricultural mechanization services, including tractors, combine harvesters, and threshers. There are several factors responsible for the limited use of agricultural mechanization services in Ethiopia. The objective of this study was to investigate the key factors that determine smallholder farmers’ decisions in hiring tractor and combine harvester services in the Debre Elias district of the Amhara region of Ethiopia. A multistage sampling technique was used to collect data from 133 respondents. Data collection techniques included structured interviews and focus group discussions. Descriptive statistics and a binary logistic model were employed to analyze the data. The findings showed that promotion and support from different organizations, including the government and development partners, are the main factors that influence farmers’ decisions to hire mechanization services. The results also revealed that economically active family labor, off-farm income, number of oxen, goal of farming, and institutional influence are the main factors that significantly influence farmers’ decisions about hiring tractor services in the study area. Harvesting labor costs and weather uncertainty were discovered to be the factors that positively and significantly influenced the farmers’ hiring decisions for combine harvester services. It is important to create awareness among farmers about agricultural mechanization services. Systematic support for both private machinery service providers and cooperative unions is needed to improve mechanization services for farmers in the area.
1. Introduction
Agriculture is the mainstay of the Ethiopian economy, contributing about 32.5% of the country’s gross domestic product (GDP) and more than 80% of its exports (National Bank of Ethiopia, Citation2021). Furthermore, it is one of the main employment sectors with about 80% of the country’s population depending on the agricultural sector for their livelihoods (World Bank, Citation2020). The sector is mainly dominated by smallholder farming systems, and about 74% of the country’s farmers are stallholder farmers with an average farm size of less than one hectare (FAO, Citation2018). The agriculture sector is expected to feed the country’s growing population. Producing adequate food for a rapidly growing population is a prime challenge. Rapid urbanization leads to increased market demand for agricultural products such as cereals, which require more labor than other crops (Berhane et al., Citation2017). Due to the increase in urbanization, agriculture is likely to continue to be affected by a labor shortage unless supported by timely technologies, including mechanization.
The agriculture sector in Ethiopia is characterized by low crop productivity levels below regional and international standards. Ethiopia is an agricultural country predominantly relying on draught animal power. Animal power is the main mode for plowing, threshing, and transportation in Ethiopia. Hand tools are mainly used in some southern and southwestern parts of the country, where most are infested by tsetse flies, making it hard for cattle to survive (Kelemu, Citation2015). A large portion of farmers have no access to agricultural mechanization services, including tractors, combine harvesters, and threshers (Deribe et al., Citation2022). Although efforts have been made to promote the use of agricultural mechanization services in rural areas, there has been little progress in addressing farmers’ demand. This shows agricultural mechanization has not yet been well practiced and used in the Ethiopian smallholders’ context.
There are different modes of production, inputs, and technologies that need to be applied in a very integrated manner to boost agricultural productivity, feed the growing population, and support the economic development of the country. Using appropriate agricultural mechanization technology is critical to increasing agricultural productivity and ensuring food security (Sayed et al., Citation2023). Agricultural mechanization deals with the use of any mechanical aid in agricultural production. Mechanization and good management can result in better timeliness in field operations, and on good soils, this can result in improved yields (Landers, Citation2000). Mechanization reduces drudgery and improves the timeliness and efficiency of farm operations (Mrema et al., Citation2018). Without proper mechanization strategies and their proper implementation, improving agricultural production is not possible (Pan et al., Citation2018). It is a key instrument to contribute to food security (Emami et al., Citation2018) and reduce harvest and post-harvest losses (Hengsdijk & Boer, Citation2017). Promoting the use of agricultural mechanization is a vehicle for positive rural transformation (van Loon et al., Citation2020).
Ethiopia employs less agricultural mechanization than the majority of sub-Saharan African countries (Ayele, Citation2022). Studies show that about 9% of Ethiopian farmers use mechanization services to either plough their land, harvest their output, or thresh their crops (Berhane et al., Citation2016). Interestingly, there is a large variation among regions and crop types in the access and utilization of mechanization services, ranging from none to full coverage (Deribe et al., Citation2022). With 11% using machines, the Oromia region has a higher rate of agricultural mechanization in Ethiopia. In other regions, the percentages hover around 7% and 9% (Berhane et al., Citation2016). There are several factors responsible for the limited use of agricultural mechanization services in Ethiopia. These include limited agricultural mechanization policies and policy narratives (Ayele, Citation2022), willingness to pay (Takele et al., Citation2018), capital, shortage of foreign currency, unavailability and expensiveness of maintenance, spare parts, and raw materials, and shortage of trained operators (Deribe et al., Citation2022). The mechanically operated agricultural activities in the Amhara region are only 4.35%, which is far below the national average (Deribe et al., Citation2022). Smallholder farmers plough two to six times per year depending on soil type, crop type, and weed infestation to improve the infiltration, minimize run-off and reduce evaporation of water from soil surface (Temesgen et al., Citation2008). Some farmers use hired equipment (mainly combine harvesters) from private service providers or unions for harvesting wheat. According to the Debre Elias district agriculture office (DEDAO), in the year 2020/21, about 400 ha of wheat land were plowed by four-wheel agricultural tractors (DEDAO, Citation2021). Often, combine harvesters migrate from other areas during the harvesting season. Oxen and labor shortages are becoming challenges in the district, and as a result, demand for ploughing, and harvesting, and threshing technologies is increasing.
To exploit economies of scale in the use of agricultural machinery and limitations in the financial capacity of farmers to own farm machinery, it is necessary to improve hiring arrangements to provide mechanization services to small-scale farmers. The development of a market for mechanization, including mechanization service provision through private and cooperative agents, to enhance smallholder access to mechanization and unleash human energy is an important way to improve the use of mechanization services by smallholder farmers (Ayele, Citation2022). Custom hiring services for agricultural machinery enable farmers to utilize the appropriate machinery and equipment for a defined period (UNESCAP, Citation2017). However, there is little information available about the key factors influencing hiring decisions for tractors and combine harvesters in the Amhara region’s wheat-producing areas. This paper is aimed at investigating the key factors affecting the hiring decisions of farmers for tractor and combine harvester services.
2. Methodology
2.1. Sample selection
Multistage sampling procedures were used to select individual interviewees. Both purposive and random sampling techniques were employed to select representative districts, villages, and respondents. Debre Elias district (Figure ) was purposely selected for the present study because it is one of the potential wheat-belt districts for using machinery services in the East Gojjam zone of the Amhara region (DEDAO, Citation2021). Using the reconnaissance survey, three villages, Yekegat, Guay, and Tija Goter, were purposefully chosen as potentially representing the district’s major mechanization service using villages. The total number of households in the district is estimated at 22,117 households. Out of which, the selected villages have 3,083 households. According to the report from the Debre Elias district office of agriculture, about 250 and 8,000 households, respectively, used tractor hiring and combine harvesting services in the year 2018/19. To select individual respondents, a random sampling technique was used. The district office of agriculture provided a list of households that used mechanization services, and thus it was used to select the households to interview. A total of 148 household farmers were selected using the formula suggested by Cochran (Citation1977).
Where:
n = designates the sample size
N = designates total population
e = designates maximum variability or margin error (for this study 8% is taken)
l = designates the probability of the event occurring
However, the information collected from 15 respondents was not as per the standard, so it was decided not to consider it in further analysis. Hence, the final data analysis and reporting were based on a total of 133 households randomly selected from the three villages.
2.2. Data collection
Both primary and secondary data were collected for the present study. Primary data were gathered from individual farmers and secondary data collected from publications of the government, non-governmental sources, and websites. Quantitative and qualitative data were collected from participants during individual interviews and focus group discussions (FGD). Face-to-face interviews were employed to gather information from individual farmers using a structured questionnaire. Before conducting the actual data collection from respondents, experienced experts from the areas of farming and agriculture mechanization were consulted. A pre-test was conducted with five farmers to check the suitability and validity of the questionnaire. Based on the feedback from experts and the pre-test, those questions that were not clear, ambiguous, out of context, or not relevant to the study were removed from the final questionnaire. The data were collected by well-trained interviewers. Verbal consent was obtained from participants prior to data collection. The data collected was checked on the spot by the first author to maintain the quality of the information and to take immediate corrective measures when necessary. FGD was carried out with eight participants to generate qualitative information.
2.3. Data analysis
Data analysis employed both descriptive and econometric procedures. Description of the variables and hypothesis are presented in Table . The descriptive analysis (means, percentages, and standard deviations) was used to summarize important socio-economic characteristics of the interviewed households. The econometric model was used to measure the significance level of the factors and their impact on farmer hiring decisions for mechanization services. The collected data were summarized and processed for analysis. The Statistical Package for Social Sciences (SPSS V21) was employed to analyze the quantitative data. Line-by-line examination and coding were used to summarize the FGDs’ transcripts.
Table 1. Definition of variables and hypothesis
2.4. Specification of the econometric model
A binary logistic model was used to estimate the relationship between factors and farmers’ decisions towards hiring agricultural mechanization services. This model predicts the probability that an individual with certain socio-economic characteristics and other determinants chooses one of the alternatives (Field, Citation2009; Gujarati, Citation2003). Following Gujarati (Citation2003), the logistic regression model form for the binary choice problem could be introduced as follows:
The model specification for the analysis is given as: Y=f (X1, X2, X3, X4, X5, X6, X7, … Xn)
Where Yi denotes the dependent variable, representing a hiring decision for the ith household. Farmers decision towards hiring a mechanization service (those who decide: 1 and those that do not:0), Xij constitute the independent variables in the study, β0 = constant term and βj=coefficient.
Pi is assumed to be the probability that decision is made to hire mechanization services and, therefore, 1-Pi represents the probability of not hiring mechanization services.
P[Y = 1] = Pi
P[Y = 0] = 1-Pi
The ratio Pi/1-Pi is known as the odds ratio in favor of hiring a mechanization service.
The logistic model applies the maximum likelihood estimation after transforming the dependent into a logit variable. The empirical mathematical model for estimations is formulated as follows:
Based on this empirical model, the effect of explanatory variables on farmer’s decision to hire mechanization services could be expressed through the following linear relationship:
The regression probability is:
Therefore, for estimation purpose, variable Y is defined in this study as:
Where:
Y = Hiring of mechanization services (0 = no decision, 1 = hiring decision)
X1 = Household’s gender (0=female, 1=male)
X2=Household’s head age (number)
X3=Farmer’s education (0 = illiterate, 1=literate including read and write)
X4 = Farming experience (years)
X5 = Household size (number)
X6 = Economically active labor in the household (number)
X7 = Farm size (in hectares)
X8 = Size of rented land (hectare)
X9 = Wheat land (in hectares)
X10 = Off-farm income (No = 0, Yes = 1)
X11 = Number of oxen (number)
X12 = Goal of farming (1 = Seed production, 0 = otherwise)
X13 = Hire tractor because of labor shortage (Yes = 1, No = 0)
X14 = Hire tractor because of better land preparation and faster operation (Yes = 1, No = 0)
X15 = Farmers hire services due to neighbor’s influence (Yes = 1, No = 0)
X16 = Farmers hire services due to institutional influence (Yes = 1, No = 0)
X17 = Farmers hire services due to brokers influence (Yes = 1, No = 0)
X18 = Hire combine harvester because of high labor cost (Yes = 1, No = 0)
X19 = Farmers hire due to uncertainty (Yes = 1, No = 0)
3. Results and discussion
3.1. Socio-economic characteristics of the respondents
The demographic characteristics of the respondents are presented in Table . About 81% of the respondents are males, and only 19% are females. The average age of the households interviewed was 42.9 (SD = 9.71) years old, indicating they are in the productive age. A recent study by Takele and GebreSelassie, (Citation2018) showed that the age of the household head negatively affected his or her willingness to use a tractor-hire service. Nearly two-thirds of the households are literate (at least, they can read and write). About 90% of the respondents have more than ten years of farming experience. Regarding the economically active family members of the households, half of them have up to two family members, and the remaining half have more than two members. Concerning the hiring of mechanization services by respondents, 58.6% of them hired combine harvesting services, and about 39.1% hired tractor ploughing services. Farmers hired combine harvesters in greater numbers than tractor ploughs, indicating that combine harvesting is far more practical in the survey area. The rate of customary hiring of the farm machines varies seasonally and from place to place (Abiy et al., Citation2021). One-fifth of the respondents hired both a tractor and a combine harvester, and nearly 22.6% of the respondents did not hire any of the mechanization services. In the study area, there were no tractor mechanization services for disking and planting.
Table 2. Demographic characteristics of the respondents
3.2. Farmers’ reasons for using mechanization services
Table displays the driving factors for farmers to start hiring mechanization services and their opinion towards the service fees. Promotion and support from different organizations, including the government and development partners, are the main factors for influencing farmers’ decisions to hire mechanization services, as explained by 64% of the respondents. Nearly 22% of the respondents said that they hired mechanization services based on the information they got from their fellow farmers. The contribution of brokers to help farmers make hiring decisions is also meaningful, as reported by 14% of the interviewed households. The key stakeholders in the rural community, including agricultural extension services, non-government organizations, and even farm machinery manufacturers, supply information to farmers for hiring decisions (Diao et al., Citation2016). Regarding the service fees for hired mechanization services, 98% of the respondents said that the fee is expensive for hiring tractor ploughing, and about 92% said the same for a hiring combine harvester. In general, farmers consider hiring harvesting services to be costly, although it is time and energy-saving and significantly reduces harvest losses.
Table 3. Perceptions and social factors
3.3. Cultural norms for operation days
Figure depicts the number of days respondents did not allow the operation of mechanization services in their own land due to religious factors. According to the findings, 40% of the interviewed households did not allow mechanization services to operate on their farmland between 5 and 8 days per month, 18% between 9 and 12 days, and 5% between 13 and 15 days per month. Concerning weekends, 29.3% of them did not support the operation both on Saturday and Sunday. During focus group discussions with farmers, they mentioned the negative effect of having a greater number of non-working days on their day-to-day farming activities. This is particularly serious at the critical cropping stage, where there is a need for intensive and timely farming activities such as weeding, rouging, de-tasselling etc. They also mentioned that farm machinery service providers are disappointed with too many off-working days in the area, and some of them stopped providing the service and moved their machines to places where they can work throughout the month.
3.4. Sources of mechanization services
Most of the mechanization service providers in the study area are private companies. The involvement of cooperative unions in providing mechanization services is low, with only 15% of households interviewed responding. Other service providers, such as government seed enterprises, are not providing mechanization services in the study area. As reported by participants during FGDs, the distance of the villages from the main road and the bad road conditions limit the availability of agricultural mechanization services in the study area.
3.5. Farmers’ perceptions for brokers in accessing mechanization service hiring
The perception of farmers towards brokers’ role in accessing mechanization services is different (Figure ). Half of the respondents said that brokers are not as important for this service. Nearly 40% of households claim brokers are responsible for expensive hiring service fees. On the other hand, only 10% of the respondents appreciated the contribution of brokers in making the hiring process easy and fast. Previous studies in the Arsi and Bale areas of the Oromia region confirmed the contribution of brokers in linking farmers with mechanised service providers. Brokers commonly assess the quantities to be harvested and the time when areas are ready to be harvested and then coordinate with mechanized service providers (Berhane et al., Citation2017).
3.6. Factors affecting farmers’ tractors service hiring decision
Results of the econometric model regarding the farmer’s decision to hire tractor service are reported in Table . Economically active family labour, off-farm income, the number of oxen, the goal of farming, and institutional influence was significantly contributed to farmers’ decision to hire tractor service. Active family labour was negative and significantly affected the decision of farmers to hire tractor services, b = −3.76, Wald X2 = 8.583, p < 0.01. This means that households with a larger number of economically active workers are less likely to hire a tractor machinery service. This is probably associated with the availability of sufficient labour in the family to manage the ploughing field operation. In other words, when other independent variables remain constant, for every unit increase in the number of economically active labour in the household, the odds of hiring tractor mechanization services decrease by 97.7%. The higher number of oxen in the family significantly and negatively affected farmers’ decision to hire tractor services. The more oxen a family has, the less interested they are in hiring tractor service for farming operations. For every increase in the number of oxen in the household, the odds of hiring a tractor mechanization service decrease by 77.9%.
Table 4. Factors affecting farmers’ tractor services hiring decision
On the contrary, the other three variables, namely off-farm income, the goal of farming, and institutional influence, positively and significantly affected farmers’ tractor service hiring decisions. Farm households that have additional income from other activities tend to spend much of their time on trading activities or engaging in employment opportunities and would prefer to hire tractor services for their land. Interestingly, the odds that households decide to hire tractor ploughing services are 163 times higher for farmers who have off-farm income. Farmers’ decisions to hire tractor services are influenced by additional income. A recent study from Nigeria revealed that the mean income of farmers hiring tractors was higher than that of non-tractor hiring farmers (Onomu & Aliber, Citation2021). Farmers whose goal of production is to produce seed tend to hire tractor services, believing that tractor ploughed fields are better to get a better yield. Other variables such as gender, age, educational level, farming experience, size of farmland, size of land rented, labor shortage, and brokers’ influence did not significantly influence the decision of farmers to hire tractor mechanization services.
3.7. Factors affecting farmers’ combine harvester service hiring decision
Results showed that only two variables (harvesting labor cost and weather uncertainty) were positively and significantly affecting farmers’ hiring decisions for combine harvester service (Table ). High labor costs during peak harvesting time positively and significantly affected the decision of farmers to hire combine harvester services at b = 4.978, Wald X2 = 4.595, p < 0.05. It was assumed that if there are relatively fewer agricultural laborers, a shortage will be created, and labor costs will become high. This increases farmers’ demands for agricultural machinery operations. The results showed that the odds in favor of hiring a combine harvester are 145 times higher for farmers who pointed to a high harvesting labor cost as a cause. Uncertainty due to weather factors also positively and significantly affects farmers’ decisions in hiring combine harvesters (b = 4.912, Wald X2 = 3.995, p < 0.05). Uncertainty about the weather was hypothesized to be a factor in the hiring decision for combine harvesters. The results of the model confirmed that farmers, in general, make hiring decisions to avoid crop loss due to unexpected rainfall. Due to unexpected weather factors, the odds of success in hiring a combine harvester are 136 times higher for farmers who use the service. The remaining proposed or tested variables, including gender, age, education level, farming experience, household size, farmland size, additional land rent, off-farm activities, the goal of farming, neighboring influence, and intestinal influence, do not significantly affect the decision of farmers in hiring combine harvesters.
Table 5. Factors affecting farmers’ combine harvesting services hiring decision
Institutional influence through the government extension system was statistically significant in influencing the tractor hiring decision positively. However, this variable was found to be statistically non-significant for the combine harvester hiring decision. The reason may be that little or no effort is required by extension workers to convince farmers since combine harvesters are well accepted and have a higher demand. However, for tractor service, there is a low uptake, and an institutional influence through government extension services plays a role in affecting the hiring decisions of farmers.
4. Conclusions and recommendations
The findings showed that institutional services, including agricultural extension services, significantly influence farmers’ decisions on hiring agricultural mechanization services. Extension’s personal knowledge regarding the efficiency of agricultural machinery matters a lot for the adoption of the services. Therefore, it is important for extension personnel to have the correct information concerning the services.
Personal factors such as an economically active labor force, resource factors, perception, social factors, and time factors explained the circumstances in which farmers decided to hire mechanization services. The existence of a greater number of economically active labor forces within the household has a negative influence on tractor hiring decisions. Other factors such as off-farm income, farm size, the number of oxen owned, the influence of extension personnel and systems, and the goal of farming showed a positive influence on the decision of farmers to hire tractor services. Labor cost and weather uncertainty were found to be the main factors that influence farmers’ decisions about hiring combine harvester services.
Most farmers got machinery services from private custom-hire operators, although they are few in number. Cooperative unions have a few machines that can only serve a small portion of the population. There is a lack of access, especially for tractor hiring services. Hence, it is recommended to develop the market for tractor and combine harvester mechanization services through private and cooperative agents. Moreover, including capacity-building measures, awareness-creation demonstrations on farmers’ fields, and financial credit access for hiring mechanization services should be considered.
Acknowledgments
The authors would like to thank the respondents for their participation and for providing the information during face-to-face interviews and focus group discussions. The authors also acknowledge the assistance of experts from the Debre Elias district.
Disclosure statement
No potential conflict of interest was reported by the author(s).
Additional information
Funding
References
- Abiy, S., Yonas, L., & Samrawit, K. (2021). Assessing the constraint and technical evaluation of custom hiring practices of agricultural machineries in major teff, wheat and barley growing areas of Ethiopia. Academic Research Journal of Agricultural Sciences and Research, 9(1), 9–16. https://doi.org/10.14662/ARJASR2020.570
- Ayele, S. (2022). The resurgence of agricultural mechanisation in Ethiopia: Rhetoric or real commitment? The Journal of Peasant Studies, 49(1), 137–157. https://doi.org/10.1080/03066150.2020.1847091
- Berhane, G., Dereje, M., Minten, B., & Tamru, S. (2017). The rapid – but from a low base – uptake of agricultural mechanization in Ethiopia: Patterns, implications and challenges. ESSP Working Paper 105. International Food Policy Research Institute (IFPRI). https://www.ifpri.org/publication/rapid-%E2%80%93-low-base-%E2%80%93-uptake-agricultural-mechanization-ethiopia-patterns-implications-and
- Berhane, G., Hirvonen, K., & Minten, B. (2016). Agricultural mechanization in Ethiopia: Evidence from the 2015 feed the future survey. International food policy research institute (IFPRI)-Ethiopia strategy support program (ESSP) research note 48. https://www.ifpri.org/publication/synopsis-agricultural-mechanization-ethiopia-evidence-2015-feed-future-survey
- Cochran, W. G. (1977). Sampling techniques (3rd ed.). John Wiley & Sons, Inc.
- DEDAO. (2021). Debre Elias District agriculture office 2021 annual report. Unpublished.
- Deribe, Y., Getnet, B., Kang, T. G., & Tesfaye, A. (2022). Benchmarking the Status of agricultural mechanization in Ethiopia. Research Report No. 133. Ethiopian Agricultural Research Institute (EIAR). https://papers.ssrn.com/sol3/papers.cfm?abstract_id=3968527
- Diao, X., Silver, J., & Takeshima, H. (2016). Agricultural mechanization and agricultural transformation. Background paper for African Transformation Report 2016: Transforming Africa’s agriculture. African Center for Economic Transformation (ACET) and Japan International Cooperation Agency Research institute (JICA-RI). https://www.jica.go.jp/jica-ri/publication/booksandreports/l75nbg0000004aet-att/l75nbg0000004aik.pdf
- Emami, M., Almassi, M., Bakhoda, H., & Kalantari, I. (2018). Agricultural mechanization, a key to food security in developing countries: Strategy formulating for Iran. Agriculture & Food Security, 7(24), 1–12. https://doi.org/10.1186/s40066-018-0176-2
- FAO. (2018). Small family farms country factsheet. Food and agriculture organization of the United Nations. http://www.fao.org/3/i8911en/I8911EN.pdf
- Field, A. (2009). Discovering statistics using SPSS (3rd ed.). SAGE Publication Ltd.
- Gujarati, D. N. (2003). Basic econometrics (4th ed.). McGraw-Hill.
- Hengsdijk, H., & Boer, W. J. (2017). Post-harvest management and post-harvest losses of cereals in Ethiopia. Food Security, 9(5), 945–958. https://doi.org/10.1007/s12571-017-0714-y
- Kelemu, F. (2015). Agricultural mechanization in Ethiopian: Experience, status and prospects. Ethiopian Journal of Agricultural Science, 25, 45–60. https://www.ajol.info/index.php/ejas/article/view/142997
- Landers, A. (2000). Farm machinery: Selection, investment and management. Farming Press.
- Mrema, G. C., Mpagalile, J., & Kienzle, J. (2018). Current status and future prospects of agricultural mechanization in Sub-Saharan Africa [SSA]. Agricultural Mechanization in Asia, Africa and Latin America, 49(2), 13–30. https://www.cabdirect.org/cabdirect/abstract/20183203421
- National Bank of Ethiopia. (2021). Annual report, Addis Ababa, Ethiopia. https://nbe.gov.et/annual-report/
- Onomu, A. R., & Aliber, M. (2021). Factors influencing smallholder farmers’ mechanization decisions in Nigeria: The case of tractor use in the north industrial revolution era. Asian Journal of Agriculture and Rural Development, 11(2), 199–209. https://doi.org/10.18488/journal.ajard.2021.112.199.209
- Pan, Y., Smith, S. C., & Sulaiman, M. (2018). Agricultural extension and technology adoption for food security: Evidence from Uganda. American Journal of Agricultural Economics, 100(4), 1012–1031. https://doi.org/10.1093/ajae/aay012
- Sayed, H. A. A., Ding, Q., Abdelhamid, M. A., Alele, J. O., Alkhaled, A. Y., & Refai, M. (2023). Application of machine learning to study the agricultural mechanization of wheat farms in Egypt. Agriculture, 13(1), 70. https://doi.org/10.3390/agriculture13010070
- Takele, A., GebreSelassie, Y. (2018). Socio-economic analysis of conditions for adoption of tractor hiring services among smallholder farmers, Northwestern Ethiopia. Cogent Food & Agriculture, 4(1), 1453978. https://doi.org/10.1080/23311932.2018.1453978
- Takele, A., GebreSelassie, Y., & Tekeste, S. (2018). Farmers’ willingness to pay for 2-wheel tractor hiring services in northwestern Ethiopia: A contingent valuation study. Asian Journal of Agriculture and Food Sciences, 6(6), 181–192. https://doi.org/10.24203/ajafs.v6i6.5592
- Temesgen, M., Rockstrom, J., Savenije, H. H. G., Hoogmoed, W. B., & Alemu, D. (2008). Determinants of tillage frequency among smallholder farmers in two semi-arid areas in Ethiopia. Physics and Chemistry of the Earth, Parts A/B/C, 33(1–2), 183–191. https://doi.org/10.1016/j.pce.2007.04.012
- UNESCAP. (2017). Promoting custom hiring of agricultural machines for climate resilient agriculture in Myanmar’s Dry Zone. Fact Sheet 21/2017. https://repository.unescap.org/handle/20.500.12870/4484
- van Loon, J., Wolteringa, L., Krupnikb, T. J., Baudronc, F., Boaa, M., & Govaertsa, B. (2020). Scaling agricultural mechanization services in smallholder farming systems: Case studies from sub-Saharan Africa, South Asia, and Latin America. Agricultural Systems, 180, 102792. https://doi.org/10.1016/j.agsy.2020.102792
- World Bank. (2020). Ethiopia regional poverty report: Promoting equitable growth for all regions. http://hdl.handle.net/10986/34805