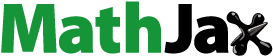
Abstract
Given that estimating the comprehensive and precise impacts of the COVID-19 crisis is challenging, this paper aims to quantify the overall impacts of the COVID-19 on Thai economy both at the macroeconomic and household levels. Our finding indicates that if government supports are not implemented, the country’s GDP could fall by 13.66 percent—the most important transmission channels of this severe impact coming from inbound and domestic tourism demand shocks. The pandemic has also significantly increased the level of poverty in Thailand. And those people facing the greatest risk of falling into poverty tend to be those living in urban areas, especially in metropolitan Bangkok, as well as those whose head of household is working in the tourism sector. In exploring the effectiveness of the mitigation measures implemented by the Thai government, our findings also show that such mitigation measures could successfully help lower the numbers of poor and almost poor people to below those of the Pre-COVID-19 era.
1. Introduction
Since the first report of a cluster of cases of viral pneumonia, which turned out to be COVID-19, in the People’s Republic of China (PRC) in late December 2019, followed by a report from Thailand of the first confirmed case outside the PRC, millions of people’s lives across the world have been affected by the COVID-19 pandemic (Ryan, Citation2021; Ryan & Nanda, Citation2022). The highly contagious nature of the virus and the lack of effective treatments have made this pandemic a global crisis, resulting in unprecedented economic and social consequences (Wang et al., Citation2021). In response to the increasing number of COVID-19 cases and related deaths, many countries all over the world have implemented non-pharmaceutical physical interventions to stop the spread of the virus, such as nationwide lockdowns, restrictions on public gatherings and movements, and restrictions on the operation of certain contact-intensive sectors. While these measures have slowed down the spread of the pandemic, they have also caused significant loss of income or, even worse, complete loss of jobs for many individuals and businesses (Ryan & Nanda, Citation2022; Wang et al., Citation2021).
Research shows that the impacts of the COVID-19 have been both massive and unequal across and within countries (Ryan & Nanda, Citation2022). National economies have experienced either single- or double-digit contractions depending on the number of infections, fatality rate, duration and stringency of measures to contain the spread of the pandemic, missed work and job losses, changes in consumer behavior, and resilience of particular economies and societies (Asian Development Bank, Citation2020; McKibbin & Fernando, Citation2020; Wren-Lewis, Citation2020). Severe income and job losses have been more common among lower-income population groups, low-skilled workers, low-education workers, informal workers, and workers in hard-hit sectors, especially in the tourism and hospitality sectors (Adams-Prassl et al., Citation2020; Crossley et al., Citation2021; Fana et al., Citation2020). As no surprise, the pandemic has plunged millions of people into poverty (Palomino et al., Citation2020).
To mitigate such impacts and revive their economies, many countries have introduced or adapted various forms of social safety nets as well as economic stimulus measures—cash transfer programs comprising the most widely used interventions by governments. By improving people’s wellbeing and livelihoods, these measures could support a country’s gradual economic and social recovery (Bayer et al., Citation2020; Boscá et al., Citation2021; Can et al., Citation2021).
While many studies have evaluated the impacts of the COVID-19 pandemic, none of them engages all the crucial aspects of such impacts concurrently. In other words, some studies focus only on the macroeconomic impacts of the pandemic, either in the absence or presence of impact-mitigating measures, but do not address the impacts at the household level whereas others focus merely on the household impacts of the pandemic, either in the absence or presence of impact-mitigating measures.
In addition, there are still several more challenges when it comes to estimating the impacts of the COVID-19 crisis. First, the COVID-19 pandemic has triggered a massive spike in uncertainty with regard to accurately identifying all of the economic transmission channels through which the pandemic shocks will adversely affect a modern economy with a complex web of interconnected parties. Second, impact-mitigating measures vary widely in terms of breadth, scope, target, and duration. Assessing their effects thus requires careful consideration. Last but not least is the critical practical challenge of assuring timeliness of data, without which impact evaluation, especially at the household or individual levels, can be seriously hindered and/or distorted. In this paper, we address these challenges. We aim to evaluate the overall impacts of COVID-19 by utilizing a top-down modeling approach in which a general equilibrium model is coupled with a microsimulation (Bourguignon et al., Citation2008).
With over 40 million tourists visiting each year (before the COVID-19 pandemic), Thailand is one of the world’s major tourist destinations and is ranked among the top 10 countries with the highest yearly tourist arrivals. Estimates of tourism revenue directly contributing to the GDP of 2.53 trillion baht in 2016, the equivalent to 17.7 percent of GDP. When including indirect travel and tourism receipts, contribution were estimated to be the equivalent of 19.3 percent of Thailand’s GDP (Sudsawasd et al., Citation2022). Thailand is one of perfect case study here due to a number of reasons, First Thailand was among the first countries in the world to be affected by restrictions on entry for foreign tourists. Immediate lock down should had huge impacts on the Thai economy in the overall.
Second, since businesses in Thailand (especially those tourism enterprises) experienced a drop in revenue (due to closures and lockdowns), yet the same debt and liquidity expenditures in both business operations and debt repayments had to be made. Therefore, many businesses needed to reduce their expenditures by reducing the number of hours worked and workers employed, thus causing some workers to be put out of work which created high unemployment which thereafter affect massive poverty and inequality (Rukumnuaykit et al., Citation2022).
Third, the Thai government responded boldly to mitigate the crisis. Timely and stringent containment measures introduced by the authorities successfully flattened the infection curve during most of 2020. Effective containment, along with a timely and multipronged policy package—comprising COVID-19 relief and fiscal stimulus amounting to about 10% of GDP.
Due to these three reasons, we aim to fully analyze the potential impacts of the COVID-19 pandemic and the associated COVID-19 relief and economic stimulus measures launched by the Thai government to discover impacts in terms of both economy-wide effects and household-level effects.
To evaluate the macroeconomic impacts in Thailand, we first employ a “top” model for this purpose, namely, the Global Trade Analysis Project (GTAP) model. This model is a multi-region, multisector, computable general equilibrium model that has been extensively used in the analysis of economic shocks due to a variety of factors, including pandemics and government policies. We augment the GTAP model by including global morbidity and mortality reports so that shocks are transmitted as realistically as possible through the changes in labor supply, production costs, consumption demand, and investment as a result of disruption of global value chains, restrictions on international mobility, and a redirection of demand away from activities that require proximity between people.
A vector of aggregate prices, wages, and employment variables from the top model—the linking aggregate variables—is then mapped with a “bottom” model that is constructed by using the latest pre-COVID-19 micro-household-level data to assess household income distribution and the country’s poverty level. To take into account the differing impact-mitigating measures, our paper also addresses the heterogeneity of impacts on different groups of households from different COVID-19 relief and economic stimulus measures by sorting them out according to who is eligible for them.
The rest of this paper is organized as follows. The next section reviews in detail prior works in the area of COVID-19 impacts assessment. In Section 3, we specify our empirical models along with crucial assumptions applied to examine the socioeconomic impacts of COVID-19 in Thailand. In Section 4 we highlight the main results for each scenario, and Section 5 concludes and provides recommendations.
2. Literature review
Prior studies employ a wide variety of models to quantify the impacts of the COVID-19 pandemic. Although these studies offer useful information on potential impacts, a holistic picture of such impacts and their practical extents, especially at the micro-level, is still incomplete and thus requires a better, deeper understanding, mostly due to the fact that the channels of influence are multiple and interconnected. Model selections together with crucial underlying assumptions about the impact of transmission channels of some selected flagship works are summarized as follows.
McKibbin and Fernando (Citation2020) estimate the aggregate economic costs by using a hybrid dynamic stochastic general equilibrium (DSGE) and computable general equilibrium (CGE) global model. They model COVID-19 as a negative shock to the labor supply, consumption spending, and financial markets, but as a positive shock to government expenditure, particularly stemming from health-related expenditures. Among seven different scenarios, they discover a reduction in global GDP of around $2.4 trillion in 2020 in the case of the most contained outbreak. Although the estimates of the fall in GDP for a number of large economies across the world are reported, those for small countries are absent. In addition, impacts from mitigating measures are not taken into account.
Can et al. (Citation2021) explore the effectiveness of macroeconomic recovery policies against the COVID-19 pandemic in Turkey by using a DSGE model. COVID-19 shock is introduced in the model as an autoregressive process that directly affects labor supply and aggregate demand as well as households’ consumption net exports, global interest rates, and inflation. The fiscal shocks include tax reductions or exemptions on consumption and wages, increasing public expenditure, and issuance of securities whereas the monetary shocks cover the cuts in policy interest rates, reserve requirements, and collateral-loan ratios. The results show that, in general, fiscal policy measures are more effective in alleviating adverse impacts of the COVID-19 shock. Nonetheless, other specific mitigating impacts that have actually been implemented by the Turkish government are not addressed in their paper.
Considered as one of the pioneering studies investigating the macroeconomic impacts of specific mitigating impacts in practice, Bayer et al. (Citation2020) simulate the effects of a lockdown by using a heterogeneous agent New Keynesian (HANK) model and quantify the impact of the U.S. government’s transfer payments to households under the Coronavirus Aid, Relief, and Economic Security (CARES) Act. They assume that a sizeable fraction of the labor force is more or less confined to their homes due to quarantine or, more generally, locked out of work during the expected lockdown duration of two months. In addition, a fraction of the aggregate capital stock and the goods of some sectors also become temporarily unavailable for production and consumption. Households are assumed to receive government transfers that replace 40% of their after-tax labor income capped at 50% of median income. For conditional transfer, households that lose income because they are put under quarantine are paid a lump-sum transfer equivalent of $600 per week. Meanwhile, a one-time payment of $1,200 to any adult in the U.S. population, except for households in the top 10% of the income distribution, is regarded as an unconditional transfer. According to the study, while the transfers could altogether reduce the output loss due to the pandemic by up to five percentage points, the conditional transfer is more directed to the unemployed, who have a high marginal propensity to consume and for which the multiplier is about six times as large as for the unconditional transfer multiplier.
The Asian Development Bank (Citation2020) not only estimated the potential economic impacts of the COVID-19 crisis and mitigating impacts announced by many countries around the world by using the GTAP model, but also calculated the poverty impacts at the household level. Their analysis incorporates three channels: (i) an increase in trade costs that affects the movement of people and inbound tourism, along with industries linked to global supply chains; (ii) a negative supply-side productivity shock that cuts wages and corporate earnings, leading to reductions in consumption and investment; and (iii) fiscal stimuli through various macroeconomic policy instruments. Their results show that the global economic impact of COVID-19 could reach $5.8 trillion (6.4% of global GDP) under a three-month containment scenario and $8.8 trillion (9.7% of global GDP) under a six-month containment scenario. Government policy responses such as direct income and revenue support could soften the COVID-19 impact by as much as 30%–40%, reducing the global economic loss to $4.1 trillion–$5.4 trillion (4.5%–5.9% of global GDP). By using the World Bank’s PovcalNet database based on the simple assumption that per capita consumption would fall by similar amounts for all households, the number of poor could increase by about 140 million, of which 56 million would fall into extreme poverty.
Lakner et al. (Citation2020) estimate the impacts of the COVID-19 on global poverty by using the PovcalNet database and growth projections from the World Bank’s Global Economic Prospects. A machine-learning algorithm is applied to determine the fraction of growth in GDP per capita that is passed through to income and consumption observed in household surveys. Holding within-country inequality unchanged and letting GDP per capita grow according to World Bank forecasts, their simulation suggests that the pandemic may have driven around 60 million people into extreme poverty in 2020. If the pandemic increased the Gini by 2% in all countries, more than 90 million may have been driven into extreme poverty in 2020. Their updated estimations in the subsequent analyses in January 2021 and June 2021, based on new growth estimates, still confirm that the pandemic reverses the downward trend in global poverty for the first time in a generation.
Laborde et al. (2020) examined the macroeconomic and poverty impacts from the COVID-19 crisis worldwide and regionally by using International Food Policy Research Institute’s global general equilibrium model and a global household database, called MIRAGRODEP and POVANA, respectively. The main channels of effect in their model include (i) income losses and demand shocks; (ii) food supply chain disruptions; (iii) consumer responses, such as hoarding, food waste, and dietary shifts; and (iv) policy responses where for the OECD countries, except for Mexico, Chile, Israel and Turkey, an economic stimulus package of, on average, 3.2% of GDP is introduced in the form of higher net income transfers from the government to the representative households. Their results reveal that, without social and economic mitigation measures such as fiscal stimulus and expansion of social safety nets in the global South, the COVID-19 crisis would result in a severe global recession with global GDP falling by 5% in 2020 and an increase in the number of people in poverty by about 150 million people, or 20% of current poverty levels. The poverty increase in rural areas is expected to be smaller than that in urban areas, partly because of the lower rate of transmission of the disease as well as the robustness of demand and supply for food relative to many other, more vulnerable sectors.
3. Methodology
This study aims to analyze the overall impacts of the coronavirus disease (COVID-19) on the Thai economy. The research framework is shown in Figure . Basically, the model employed is the Global Trade Analysis Project (GTAP) model, which is a Computable General Equilibrium (CGE) model widely used in the study of international trade. It is a multi-region and multi-sector CGE model, which is appropriate to be used especially when a shock event (e.g., a pandemic) affects multiple countries simultaneously and not necessarily the same. The results from the GTAP model can show the impact of the COVID-19 pandemic on prices, outputs, and macroeconomic variables. In particular, the results on input-factor prices and sectoral output prices can be linked to the micro-level data from the Thailand 2019 Household Socio-Economic Survey (SES) in the microsimulation to show the impact on household income and poverty and to provide a quantitative assessment of the effectiveness of government relief and stimulus measures. More detailed information about the model and shock scenario is discussed below.
3.1. The model
3.1.1. GTAP model
In this study, the CGE model employed is based on the standard GTAP model, which is a multi-country Computable General Equilibrium model. The structure of the model consists of behavioral equations which describe the function of each country’s economic system. The economic systems of different countries are linked together through import-export and international capital mobility. Equations were added to explain the behavior of the international transport sector.
Figure shows the standard structure of a country’s economy within the model. The model creates a virtual regional household to collect different incomes or revenues from private household, business, and government sector in order to simplify the model and reduce the burden of data compilation, such as direct taxes and direct transfers among these sectors. This aggregate income is then redistributed to private household, government, and the global bank.
The economic units within the model consist of producers, private households, governments and foreign sectors. Producers collect various factors of production from producers in other sectors (VDFA), imports (VIFA) and regional household (VOA) to produce goods and services. These goods and services are then sold to private household (VDPA), government (VDGA) and foreign sector through exports (VXMD) in addition to the demand for goods and services from domestic producers. Private household and government also consume foreign goods and services through imports (VIPA and VIGA). Regional household receive tax revenues from domestic producers, private household, and government (TAXES) and also from import and export activities (MTAX and XTAX).
Regional household spends income received from compensation for factors of production and taxes through private household consumption and government consumption (PRIVEXP and GOVEXP). The remainder is retained as savings (SAVE). The model assumes the existence of a global bank who collects savings from different economies around the world, and then are allocated into investments among different economies (NETINV) according to the rate of return of investment in each economy.
The model makes basic assumptions of perfect competition and constant returns to scale for production sector. Bilateral trades are linked together through the Armington assumption. The detailed structure of the GTAP model can be further studied in Hertel and Tsigas (Citation1997).
The GTAP database version 10 is used. It contains trading relations for 65 sectors and 141 countries/regions. This database relies on country-based input-output (I-O) tables and sets of behavioral parameters, such as consumer demand elasticities. It is noted that this study assumes in the model that capital can adjust freely across sectors within a country. The shock scenario used in this analysis is created by referring to the situation extant at the end of the second wave of COVID-19 infections in Thailand, ending around the last week of March 2021. As reported in Table , the attack rate and case-fatality rate used for the entire country are the actual rates as of 2 April 2021, as reported in the WHO Coronavirus (COVID-19) Dashboard. The data surprisingly show that the attack rate and the case-fatality rate due to the COVID-19 pandemic in Thailand are relatively low when compared to other countries.
Table 1. Assumptions regarding the attack rate and the case-fatality rate
For transmission channels of the economic impacts from the pandemic, this study follows the previous works of Lee and McKibbin (Citation2004), McKibbin and Sidorenko (Citation2006), and McKibbin and Fernando (Citation2020) by incorporating (i) the change in labor supply; (ii) the change in costs of production; (iii) the change in consumption demand; and (iv) the change in a country’s risk premium in the model. These four channels can account for both direct and indirect effects from the domestic shocks associated with mortality and morbidity that transmitted across countries and regions through the links of international trade and capital flows.
3.1.1.1. The change in labor supply
The outbreak of the COVID-19 pandemic affected the labor supply mainly in three aspects. First, the labor supply was permanently depleted due to the death of infected persons (mortality). Second, it was temporarily decreased as the infected persons had to stop working until they recovered (morbidity). Third, it was temporarily diminished due to absences from work of the relatives of the infected persons who took care of them. The estimation result of the change in labor supply of each country is shown in Table . As can be seen from this table, the estimated total annualized day loss for Thailand is at 0.0088 percent, largely due to the morbidity related to COVID-19. Nonetheless, the estimated number is low because Thailand has relatively low attack rate and case-fatality rate (as of 2 April 2021) when compared to most countries.
Table 2. Assumptions regarding the change in labor supply
3.1.1.2. The change in costs of production
The change in production costs of different sectors came from the fact that COVID-19 pandemic hindered some production activities that caused production costs to increase. The level of increase varies among sectors, depending on the dependency level on the production factors that come from sectors that are directly affected by the pandemic. These sectors include wholesale and retail trade, hotels and restaurants, land transport, water transport, air transport, and warehouse/support activities. Such dependency levels of a sector are calculated from the proportion of the value of input factors from these sectors to the value of all input factors used in that sector.
In addition, the increased level of production costs of each sector in a country is also assumed to be proportional to the mortality rate of that country. This assumption is the same as that used by McKibbin and Fernando (Citation2020). The change in production cost that is used as a benchmark is taken from the case of China’s SARS outbreak (McKibbin & Fernando, Citation2020), in which the 0.2 percent of mortality rate caused production costs to increase by 0.5 percent. Table shows the estimated increased cost in each group of production sectors.
Table 3. Percentage change in costs of production by sector and country
3.1.1.3. The change in consumption demand
Like the change in costs of production, the COVID-19 outbreak also caused the consumption demand to decrease, as some consumption activities became more difficult to pursue, in terms of the demand for both domestic and imported goods and services.
This study specifies the level of change in consumption demand for each country proportional to the mortality rate and the proportion of GDP of the country that comes from the sectors that were highly affected by the COVID-19 outbreak, namely: (i) wholesale and retail trade; (ii) hotels and restaurants; (iii) land transportation; (iv) water transportation; (v) air transportation; (vi) warehouse/support activities; and (vii) leisure and other services. This assumption is similar to that of the McKibbin and Fernando (Citation2020) study. The proportion of GDP from these service sectors by country is shown in Table . In the case of Thailand, it is calculated the GDP share from those sectors is around 28.6 percent which is relatively high when compared to other countries.
Table 4. GDP share from sectors that were directly affected by COVID-19
The magnitude of change in consumption demand from the SARS outbreak case study (McKibbin & Sidorenko, Citation2006) was used as a benchmark for calculating the change in consumption demand from the outbreak of COVID-19 in this study, i.e., a mortality rate of 0.2 percent that caused a 1.0 percent decrease in consumption demand. Table shows the assumption regarding the change in consumption demand.
Table 5. Assumptions regarding the change in consumption demand
3.1.1.4. The change in countries’ risk premiums
As with previous studies, this study considers how the COVID-19 pandemic could affect the risk premium of each country. For the method used for calculating the change in risk premium, this study used the data from country risk premiums between January 2020 to the end of June 2020 provided by Damodarn (Citation2020), and specifies that the change in risk premium during that period is a function of the case-fatality rate and the attack rate of COVID-19.
The estimation result of the linear regression (using Ordinary Least Square Method) obtained by using First-Difference Method (to control the effect of other factors that are constant in that period) was used to estimate the change in the countries’ risk premiums according to the case-fatality rate and attack rate of each country as shown in Table . For the case of Thailand, the estimated change in the country risk premium due to the COVID-19 is at 0.09 percent.
Table 6. Assumptions regarding the change (unexpected) in countries’ risk premiums
Since Thailand relies very much on its tourism sector, the shock in tourism demand as a result of the pandemic would seem to have a severe negative impact on the Thai economy. Unfortunately, under the current GTAP database, tourism flows are not yet fully integrated into the model. Therefore, this study attempts to construct the augmented GTAP model to capture the change in inbound and domestic tourism demand as the fifth transmission channel.
3.1.1.5. The change in inbound and domestic tourism demand
Because the GTAP data base does not isolate the tourism activities from other economic activities, this study therefore uses the following information, shown in Tables , to isolate tourism activities from other activities.
Value of inbound tourism income to that of the total exports of goods and services
Value of outbound tourism expenditure to that of total imports of goods and services
Value of domestic tourism expenditures to that of the final consumption
Table 7. Income and expenditure of international tourism by country
Table 8. Expenditure on domestic tourism to final consumption by country
The incomes and expenditures of international tourism are parts of exports and imports of goods and services of a country, respectively. In the GTAP model, as exports and imports of goods and services are specified as bilateral trade between countries, the incomes and expenditures of international tourism must also be specified in terms of bilateral trade.
Nonetheless, because this study was unable to find the information on bilateral international tourism incomes and expenditures, which covers the countries/groups of countries specified in this study, we used the data on the proportion of inbound tourism income to exports of goods and services, and the proportion of outbound tourism expenditures to imports of goods and services to construct a matrix of bilateral international tourism income and expenditure flows.
Creating such a matrix is an optimization problem with the objective to minimize the sum of squared difference between the total income and the total expenditure of international tourism by country obtained from the created matrix that obtained from the actual data (calculated from the proportion of international tourism expenditures based on the value of exports/imports of goods/services from the GTAP database). The constraints of this optimization problem are that the incomes and expenditures of international tourism of each country must not exceed the value of export and imports of goods/services in the tourism-related industry of that country (according to the GTAP database), respectively. In the case of Thailand, the total income and total expenditure of international tourism obtained from the created matrix is constrained to be equal to the total income and total expenses of the actual data.
This study used OpenSolver software to solve the above optimization problem. Table compares the total values of incomes and expenditures of international tourism of each country, obtained from the created matrix of bilateral flows of international tourism expenditures with those obtained from the actual data. The differences between these two datasets come from the inconsistency between the data on incomes and expenditures of international tourism and the data on exports and imports of goods and services in the GTAP database.
Table 9. International tourism income and international tourism expenditure by country (Unit: Million US$)
3.1.2. Microsimulation model
A microsimulation model is a simulation of the behavior of economic units at the micro level, for example, individual, household, or firm levels, where each economic unit has its own characteristics that reflect the actual conditions, such as income, education level, number of children, location, size of the firm, etc., without the need of data aggregation. The model database contains different types of economic units in proportion that can represent the actual economic condition. The model is therefore able to statistically infer what happens to the real economy as a whole and to a specific target group.
In this study, the analysis of the impacts of COVID-19 on household income and poverty utilized the household microsimulation model. Using a top-down approach, the GTAP model was linked to the microsimulation model through factor incomes classified by sector of production, and price of goods and services. Each household in the microsimulation model has its own utility function, each of which is assumed to be Cobb-Douglas function. The utility functions were calibrated by using the data of household expenditures from Household Socio-Economic Survey. For simplicity, this study assumes the income elasticity of household expenditure to equal one and no change in household expenditure patterns during the pandemic. The household problem can be shown as follows.
Subject to
Where is household utility,
is household expenditure,
is price of commodity i,
is price of commodity i,
is parameter
Solution to the above problem can be shown as the following.
To do the household microsimulation from the results of the GTAP model, we matched data from the 65 sectors of consumption and production in the GTAP database with the 261 categories of consumption and 441 categories of production in the Thailand 2019 Household Socio-Economic Survey. Then, the prices of input-factor (including unskilled labor, skilled labor, capital) and output in each sector between these two datasets were reconciled. Finally, the impact of a shock scenario on household income and on poverty can be assessed in Table .
Table 10. Assumptions regarding the changes in inbound and domestic tourism demand
3.2. COVID-19 relief and economic stimulus measures
To assess the impact as well as the effectiveness of the Thai government measures in response to the coronavirus pandemic, this study considers a set of COVID-19 relief and economic stimulus measures worth more than 23.35 billion US dollars approved from the start of pandemic until the end of second wave, as summarized in Table . The COVID-19 relief measures comprise six cash transfer programs, including cash handouts (e.g., We Stand Together), co-payments (e.g., Khon La Khrueng), etc. In addition, the Thai government also adopted an economic stimulus measure (We Travel Together) worth 0.70 billion US dollars aimed at promoting domestic tourism demands and activities to help revive the tourism sector and to boost economic recovery. Under the We Travel Together program, the government offered to subsidize the costs of hotel accommodation and airline tickets as well as other services, including food. It is noting that most of these COVID-19 relief and economic stimulus programs will be financed mainly from borrowing under a 1-trillion-baht (31.25 billion US dollar) program approved in April 2020.
Table 11. Policy responses through the end of second wave of COVID-19 infections in Thailand
In this study, all government’s relief measures are assumed to extend over a period of two years (24 months). Hence, the average monthly benefits, in terms of an individual’s income gains, are calculated by taking total benefits that each individual receives divided by 24. Everyone, without any exceptions, who is eligible for the relief measures is assumed to receive benefits. It is further assumed that there is no change in benefit recipients’ consumption patterns. And cash transfers received are used as part of their current consumption spending. Finally, the tourism stimulus measure is assumed to increase domestic consumption expenditure on goods or services produced domestically in Thailand’s tourism sector.
4. Simulation results
This section divides the study into four subsections. First, the macroeconomic results from the GTAP model are presented to indicate the overall impact of the COVID-19 pandemic on the Thai economy. In the second subsection, the impact on poverty based on the microsimulation results is discussed. Then, the findings on the macroeconomic impact of government support measures are presented. And lastly, the effectiveness of the Thai government measures in response to COVID-19 are assessed.
4.1. Impact of COVID-19 on the macro economy
Table presents simulation results indicating that COVID-19, at the end of second wave, had a negative impact on economic welfare in Thailand, measured by the equivalent variation (EV). As shown in the table, the decrease in EV is about 63,904 million US dollars based on a monetary value in the year of 2014. The real gross domestic product (GDP) also decreased by 13.66 percent. Inflation was reduced by 5.09 percent partly due to 21.14 percent lower demand for private consumption expenditure. Investment in Thailand slightly increased by 1.33 percent, since there were capital inflows from other countries, especially from those countries who experienced a greater fall in GDP, resulting in a lower rate of return on investment.
Table 12. Macroeconomic impact of the COVID-19 pandemic at the end of the second wave in Thailand (Unit: percent change)
Although the terms of trade slightly improved by 0.38 percent, COVID-19 still had a large negative impact on the trade balance (about 11,545 million US dollars), as the 18.46 percent decrease in exports was greater than 14.36 percent decrease in imports. Likewise, tax revenue was expected to decline by about 17.87 percent.
Table reports the effects according to the six transmission channels, as discussed in the previous section. In the case of Thailand, the most important transmission channels of the impact were from inbound and domestic tourism demand shocks. They accounted for 73.49 percent of total impact in real GDP. In particular, a reduction in inbound tourism alone accounted for 61.42 percent of the total impact. This finding highlights the importance that the tourism sector and tourism inflows have on the Thai economy.
Another important transmission channel that had large impact on the Thai economy was the change in a country’s risk premium since the COVID-19 pandemic intermediately raised the country’s risk premium. The simulation result shows that this channel accounted for 21.38 percent of the total impact in real GDP. For other transmission channels, including the change in labor supply, the change in costs of production, and the change in consumption demand, they all appear to have only small effects since Thailand had very low mortality and morbidity rates. Besides, the reduction in labor demand was greater than the reduction in labor supply from mortality and morbidity. The change in labor supply had, surprisingly, negligible impact in Thailand.
For the sectoral impact of the COVID-19 pandemic, the 15 largest output losses are reported in Table . As expected, tourism related sectors were hit hardest in terms of output decline. These sectors include accommodation, food and service activities (61.2 percent decrease), trade (22.8 percent decrease), and recreational and other services (41.4 percent decrease). The results indicate that these sectors were hit hardest by the pandemic due to the fall in inbound and domestic tourism demands.
Table 13. Sectoral impact of the COVID-19 pandemic at the end of the second wave in Thailand (the 15 largest output losses)
4.2. Impact of COVID-19 on poverty
Figure shows the overall impact of COVID-19 pandemic on poverty, as measured by the number of people whose monthly income put them below the poverty line in Thailand. At the end of second wave of COVID-19, the number of poor people was projected to increase from the baseline number in the year of 2019 (4.3 million people) to 9.4 million people (117.26 percent increase). And the new poverty rate was about 13.57. Within the group of poor people, the share of those “extremely poor” people, whose monthly incomes were below the poverty line by 20 percent, increased remarkably. The number of “extremely poor” people increased from 1.3 to 3.7 million people (184.61 percent increase). It is noted that the number of “almost poor” people, or those whose monthly incomes were above the poverty line but less than 20 percent, was projected to increase from 5.4 to 7.6 million people (40.74 percent increase), which is about 10.97 percent of the total population.
Figure 3. Impact of the COVID-19 pandemic on poverty in Thailand.
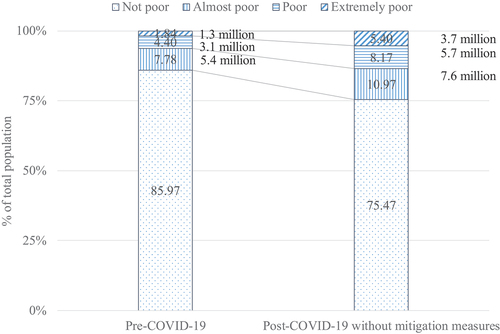
Figure shows the impact of the second wave of COVID-19 in Thailand on poverty classified by characteristics of the household head. We see that people who face the greatest risk of falling into poverty (more than a 200-percent change in the number of poor people) were those who lived in Bangkok (a remarkably high, 658.28 percent increase), those who had the head of household or a family member working in the tourism sector, those who rented their home, and those with their head of household with upper secondary and higher education. It is noteworthy that those people who lived in urban areas faced higher risks of poverty when compared with those who lived in rural areas.
Figure 4. Percent change in the number of poor people classified by characteristics of the household head.
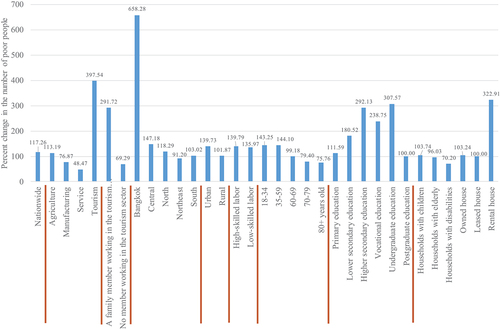
In summary, findings indicate that the COVID-19 pandemic increases the number of those below the poverty level in Thailand significantly, as the number of poor people more than doubled. In addition, the pandemic had diverse and heterogenous impacts on people. Hence, designing government policies in response to the pandemic is very important, especially with regard to those policies aimed at mitigating adverse impacts for different groups of people as well as the economy as a whole.
4.3. Macroeconomic impact of government support measures
As discussed in Section 3, one of the aims of this study is to assess the impact of COVID-19 government support measures on the Thai macro economy. Government support measures consist of two types: COVID-19 relief measures and tourism stimulus measures, amounting in total to 23.35 billion US dollars. Using the simulation results of the GTAP model, Table summarizes the changes in macroeconomic variables. In the table, columns (1) and (3) show the combined impact of the COVID-19 pandemic and government measures. And columns (2) and (4) show the “pure” impact of government measures.
Table 14. Macroeconomic impact of COVID-19 relief and tourism stimulus measures in Thailand (Unit: Percent change, unless otherwise indicated)
Although the combined impact of COVID-19 and government relief measures on the Thai economy remains negative, COVID-19 relief measures still produce a strongly positive “pure” impact on economic welfare and GDP. This study finds that welfare, measured by equivalent variation, improved by about 19,192 million US dollars. The levels of real GDP and private consumption increased by 4.02 percent and 13.98 percent, respectively. Due to the relief measures, tax revenue is estimated to increase by 4.58 percent. Terms of trade of the country are expected to improve by 0.28 percent. However, trade balance worsened by 13,800 million US dollars due to a higher growth rate in imports (4.59 percent increase) than in exports (1.03 percent decrease). It is noted that the decline in exports is partly because of an increase in domestic demand for outputs that would result in an increase in domestic prices and thereby a decrease in the country’s exports.
As for the “pure” impact of the government’s tourism stimulus measures, it has the same direction but in a smaller magnitude, as the smaller size of the government budget (0.70 billion US dollars vs. 22.65 billion US dollars). For instance, this study estimates that economic welfare increased by 1.623 billion US dollars and GDP by 0.35 percent. These increases are relatively small as compared to the simulation effects of government relief measures. However, according to the cost-effectiveness of government programs measured by the ratio of benefits (in terms of welfare gains) per dollar spent on the program, the tourism stimulus measures are shown to be more cost-effective government support programs since the benefits per dollar spent on the tourism stimulus measures at 2.32 are much higher than those of the relief measures at 0.847.
4.4. Government support measures and poverty alleviation
The effectiveness of government support programs to alleviate the impact of COVID-19 is assessed in this part of the paper. The microsimulation results (as in seen from Figure ) shows that the COVID-19 relief measures can reduce the number of poor people in Thailand from 9.4 million people to about 4 million people (57.44 percent decrease). And the number of almost poor people at the end of the second wave decreased from 7.6 million people to 5.5 million people (27.63 percent decrease), which is now about 10.97 percent of the total population. Government relief measures can lower the numbers of poor and almost poor people to below the baseline (before the COVID-19) levels in 2019 at 4.3 and 5.4 million people respectively. Hence, the government benefit programs can be considered, overall, as effective as measured by the size of poverty reduction if everyone has access to the relief measures designed for them.
Figure 5. Impact of the government’s relief measures on poverty in Thailand.
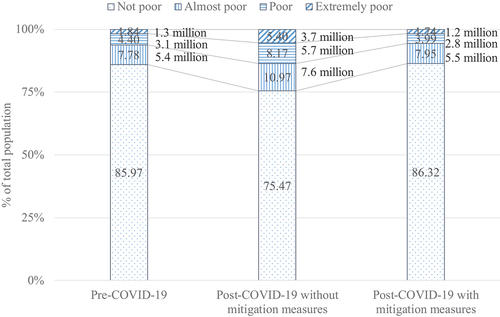
In spite of the government budget constraint, a set of across-the-board policy packages was purposely designed to urgently embrace as much population as possible regardless of each person’s genuine needs, resulting in an equality of helps but not an equity where more vulnerable people should be offered more to justify their needs. If one assumes that all government relief programs are aimed at the poor, the effectiveness of the government programs in terms of target efficiencies includes both horizontal efficiency, in which benefits should go to all the poor, and vertical target efficiency, in which benefits should go to only those who actually need them. Table shows that 87.07 percent of those who received the benefits were actually the non-poor. This finding demonstrates that there were serious inclusion errors in the government programs since a large majority of benefit recipients were not the target population. As for horizontal target efficiency, 16.50 percent of the poor population (around 1.56 million poor people) did not receive any financial assistance from government programs. They may not have had access or were able to fulfill eligibility criteria. This problem is generally known as exclusion error.
Table 15. Benefit recipients from COVID-19 relief measures
Table also provides information about the proportion of recipients receiving adequate benefits for two groups of people, the poor and non-poor. In this study, the level of benefits is calculated specific for each individual. It can be considered adequate when an individual receives average monthly income support payments from the government’s relief measures over a period of 2 years (from 2020 to 2021) greater than or equal to average monthly income loss due to the COVID-19 pandemic. It is shown that 52.65 percent of poor recipients received adequate support from the government to cover their loss of income from the COVID-19 pandemic. Still, a large number of poor (4.45 million people) were estimated to have received only insufficient support. This finding perhaps suggests the need for a better targeting of government relief measures.
Figure presents additional information about the proportion of poor people receiving adequate benefits from government relief measures, classified by characteristics of the household head. We see that a large proportion of poor people who received inadequate levels benefits lived in Bangkok, had a head of household or a family member working in the tourism sector, leased or rented their home, and had a head of household with an upper secondary or higher education. These groups of poor people face a high risk of long-term poverty and should be prioritized for receipt of government support.
Figure 6. Percent of poor people receiving adequate benefits by characteristics of the household head.
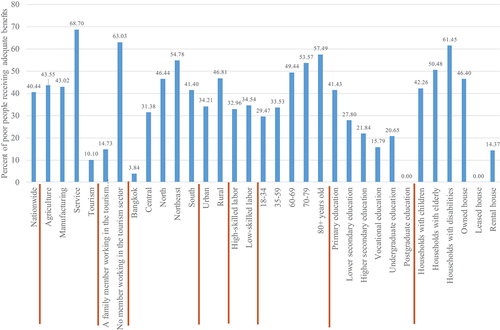
Finally, Table presents the average monthly loss of income per person and the average monthly total benefits per person from government support over a period of 24 months by income decline. As shown, at the end of the second wave of COVID-19, Thai people lost income, on average, around 1,355 baht per person per month whereas the average financial assistance from the government was around 540 baht per person per month. Hence, the average financial support from the government is seen to be less than a half of the average income loss per person. As for the distribution of benefits to cover income losses, the level of benefits is shown to increase with income up to the third decile and then starts to decrease with income. This contrasts with the amount of income losses, which appear to be progressive with income of all deciles. In addition, only people in the first three deciles received adequate financial support from the government programs to cover their lost income.
Table 16. Benefits from COVID-19 relief measures by income decile
5. Conclusion
While estimating the comprehensive and accurate impacts of the COVID-19 crisis is challenging, this paper aims to provide a full analysis of the potential impacts of the COVID-19 pandemic and the associated COVID-19 relief and economic stimulus measures on the Thai economy both at the macroeconomic and household levels by using the scenario-based top-down modeling approach in which the augmented Global Trade Analysis Project (GTAP) model is coupled with the microsimulation of the latest pre-COVID-19 micro-household-level data. Specifically, we adopt two scenarios: one with the absence of the COVID-19 relief and economic stimulus measures and the other with the presence of some selected flagship government supports.
In light of the actual worldwide COVID-19 morbidity and mortality reports as of April 2021, about which time the second wave of COVID-19 in Thailand ended, we can see that Thailand’s economy has been hit hard by the pandemic. We show here that if effective government support is not in place, the country’s GDP could severely fall (by 13.66 percent), as a result, mainly, of the most important transmission channels of the impact—inbound and domestic tourism demand shocks. In particular, a reduction in inbound tourism alone accounts for 61.42 percent of the total impact, highlighting the importance that the tourism sector and tourism inflows have on the Thai economy.
The pandemic has also significantly increased the number of people living in poverty in Thailand. Indeed, the number of poor people, in the absence of the government support, could more than double to 9.4 million people, among which 3.7 million people would experience extreme poverty. The COVID-19 crisis is hitting many households hard, but it does not affect them all equally. We find that people who face the greatest risk of falling into poverty are those who live in Bangkok, those who have their head of household or a family member working in the tourism sector, those who rent their home, and those whose head of household has upper secondary or higher education. And generally, people living in urban areas face higher risks of poverty than do those in rural areas.
We also explore the effectiveness of the mitigation measures implemented by the Thai government. According to the analysis, a set of six cash transfer programs and a tourism stimulus measure worth in total more than 23.35 billion US dollars produced a strong positive “pure” impact on the country’s GDP even though the combined impact of COVID-19 and these government relief measures on the Thai economy remains negative. Although the economic benefits accrued from the government’s tourism stimulus measure are relatively small, the tourism stimulus measure is shown to be more cost-effective than the other six government relief measures.
In terms of poverty reduction, the mitigation measures were found to decrease the numbers of poor and almost poor people to below the Pre-COVID-19 baseline levels in 2019, at 4.3 and 5.4 million people, respectively. Yet, a huge gap between reality and expectation still exists. Our analysis reveals that there are likely serious inclusion as well as exclusion errors in the government programs, as 87.07 percent of those who received benefits were the non-poor. And, indeed, 16.50 percent of the poor population did not receive any financial assistance from the government programs largely due to their failure to fulfil eligibility criteria. Moreover, a large number of poor received insufficient support from the government to cover their lost income during the pandemic, especially those who lived in Bangkok, those with their head of household or a family member working in the tourism sector, those without their own home, and those with a head of household with an upper secondary or higher education. These groups of poor people still face a high risk of long-term poverty and it is not too late to help such folks by pushing for government support programs.
Disclosure statement
No potential conflict of interest was reported by the author(s).
Additional information
Funding
Notes on contributors
Sasatra Sudsawasd
Sasatra Sudsawasd is Professor of Economics at Graduate School of Development Economics, National Institute of Development Administration in Bangkok, Thailand. He has been working in areas of International Trade, Tourism Economics, Public Finance, and Applied Economic Modelling. Professor Sasatra Sudsawasd received his doctorate in economics from Georgia State University (USA).
Taweechai Charoensedtasin
Taweechai Charoensedtasin is now Research Director of Institute of Future Studies for Development in Bangkok, Thailand. He has extensive working experience in different areas of Economic Development, Economic Forecasting, and Computable General Equilibrium (CGE) Model. Mr.Taweechai Charoensedsatis received his Bachelor Degree in Engineering and Master Degree in Economics both from Chulalongkorn University (Thailand.)
Nuttawut Laksanapanyakul
Nuttawut Laksanapanyakul is an independent consultant. He has been working tremendous researches and consultancy projects for both private and public sector. Mr.Nuttawut Laksanapanyakul received his Bachelor Degree in Engineering and Master Degree in Economics both from Chulalongkorn University (Thailand.)
Piriya Pholphirul
Piriya Pholphirul is Professor of Economics and Director of Center for Development Economics Studies, National Institute of Development Administration in Bangkok, Thailand. His expertise is Tourism Economics, Human Development, and Economics of Service Sector. Professor Piriya Pholphirul received his doctorate in economics from Georgia State University (USA).
References
- Adams-Prassl, A., Boneva, T., Golina, M., & Rauh, C. (2020). Inequality in the impact of the coronavirus shock: Evidence from real time surveys. Journal of Public Economics, 189, 1–29. https://doi.org/10.1016/j.jpubeco.2020.104245
- Asian Development Bank. (2020). An Updated assessment of the economic impact of COVID-19 ADB Briefs, No. 133.
- Bayer, C., Born, B., Luetticke, R., & Müller, G. J. (2020). The coronavirus stimulus package: How large is the transfer multiplier? CEPR Discussion Papers 14600, C.E.P.R. Discussion Papers.
- Boscá, J. E., Doménech, R., Ferri, J., García, J. R., & Ulloa, C. (2021). The stabilizing effects of economic policies in Spain in Times of COVID-19. Applied Economic Analysis, 29(85), 4–20. https://doi.org/10.1108/AEA-11-2020-0165
- Bourguignon, F., Pereira da Silva, L. A., & Bussolo, M., Pereira da Silva, L. A., Bourguignon, F., Bussolo, M. (2008). The Impact of MacroEconomic policies on poverty and income distribution: Macro-Micro evaluation techniques and tools. World Bank Publications. https://doi.org/10.1596/978-0-8213-5778-1
- Can, U., Can, Z. G., Bocuoglu, M. E., & Dogru, M. E. (2021). The effectiveness of the post-covid-19 recovery policies: Evidence from a simulated DSGE model for Turkey. Economic Analysis and Policy, 71, 694–708. https://doi.org/10.1016/j.eap.2021.07.006
- Crossley, T. F., Fisher, P., & Low, H. (2021). The heterogeneous and regressive consequences of COVID-19: Evidence from high quality panel data. Journal of Public Economics, 193, 1–11. https://doi.org/10.1016/j.jpubeco.2020.104334
- Damodarn, A. (2020). Country Risk: Determinants, measures and implications – the 2020 Edition. NYU Stern School of Business. updated on July 14, 2020.
- Fana, M., Pérez, S. T., & Fernández‑Macías, E. (2020). Employment impact of covid‑19 Crisis: From short term effects to long terms prospects. Journal of Industrial and Business Economics, 47(3), 391–410. https://doi.org/10.1007/s40812-020-00168-5
- Hertel, T. W., & Tsigas, M. E. (1997). Structure of GTAP. In T. W. Hertel (Ed.), Global trade analysis: Modeling and applications (pp. 13–73). Cambridge University Press. https://doi.org/10.1017/CBO9781139174688.003
- Lakner, C., Mahler, D. G., Negre, M., & Prydz, E. B. (2020). How much does reducing inequality matter for global poverty? Global poverty monitoring technical Note, No.13. The World Bank. https://doi.org/10.1596/33902
- Lee, J. W., & McKibbin, W. J. (2004). Globalization and disease: The case of SARS. Asian Economic Papers, 3(1), 113–131. https://doi.org/10.1162/1535351041747932
- McKibbin, W. J., & Fernando, R. (2020). The Global macroeconomic impacts of COVID-19: Seven Scenarios CAMA Working Papers 2020-19, Centre for Applied Macroeconomic Analysis, Crawford School of Public Policy, The Australian National University.
- McKibbin, W. J., & Sidorenko, A. A. (2006). Global macroeconomic consequences of pandemic Influenza CAMA Working Papers 2006-26, Centre for Applied Macroeconomic Analysis, Crawford School of Public Policy, The Australian National University.
- Palomino, J. C., Rodríguez, J. G., & Sebastian, R. (2020). Wage inequality and poverty effects of lockdown and social distancing in Europe. European Economic Review, 129, 1–25. https://doi.org/10.1016/j.euroecorev.2020.103564
- Rukumnuaykit, P., Pholphirul, P., & Kwanyou, A. (2022). Business survival in times of COVID-19: Empirical evidence from tourism enterprises in Thailand. Global Business Review, 097215092211160. https://doi.org/10.1177/097215092211160
- Ryan, M. J. (2021). COVID-19 (Vol. I & II). Routledge. https://doi.org/10.4324/9781003155911
- Ryan, M. J., & Nanda, S. (2022). COVID-19: social inequalities and human possibilities. Routledge. https://doi.org/10.4324/9781003178019
- Sudsawasd, S., Charoensedtasin, T., Laksanapanyakul, N., & Pholphirul, P. (2022). Pro-poor tourism and income distribution in the second-tier Provinces in Thailand. Area Development and Policy, 7(4), 404–426. https://doi.org/10.1080/23792949.2022.2032227
- Wang, C., Wang, D., Abbas, J., Duan, K., & Mubeen, R. (2021). Global financial crisis, smart lockdown strategies, and the covid-19 spillover impacts: A global perspective implications from Southeast Asia. Frontiers in Psychiatry, 12(September 2021), Article 643783. https://doi.org/10.3389/fpsyt.2021.643783
- Wren-Lewis, S. (2020). The economic effects of a pandemic. In R. Baldwin & B. W. DiMauro (Eds.), Economics in the Time of COVID-19, a VoxEU.Org book (pp. 109–112). CEPR Press.