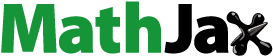
Abstract
The study examines the impact of non-communicable diseases (NCDs) and employment status in South Africa utilising the National Income Dynamics Study longitudinal data from 2008 to 2017. The Generalized Linear Latent and Mixed Methods (GLLAMM) were employed to fit the multinomial logit model with correlated random intercept over panel multinomial logit without random effects to control for unobserved heterogeneity between individuals or intercepts. The empirical results indicate that the significant impact of NCDs on employment status differs by gender. NCDs were found to be most threatening to women employment status. The odds of women being economically inactive in the labour market are highly associated with NCDs. Further, having multiple NCDs also significantly increases the women’s probability of being economically inactive population relative to being employed. The results highlight the necessity for undertaking a massive awareness campaign regarding the prevention and control of NCDs, especially among women.
1. Introduction
The available literature show that a country’s economic growth is highly characterised by increased labour force participation (Duval et al., Citation2010; Shahid, Citation2014). However, labour market statistics in South Africa show a high rate of economically inactive labour force. It is interesting to also note that the extended unemployment rate rose from 28% in 1990 to 37% in 2018 while the share of labour absorption rate fell from 56% in 1990 to 42% in 2018 (Ataguba, Citation2012; Statistics South Africa, Citation2019). Grossman (Citation1972) argues that poor health may reduce the amount of time an individual has for market activities and spend more hours on leisure, thus affecting the time allocation and reallocation among various employment status.
Research evidence suggest that individuals with ill-health are less likely to being employed in the labour market (van den Berg et al., Citation2010). Therefore, this study examines the impact of non-communicable diseases (NCDs) on employment status in South Africa. NCDs are known to be associated with mortalities, poor self-rated health as well as reduced social activity (Peasey et al., Citation2006).
It is evident that NCDs play an important role in the labour market exit through work disability (Kouwenhoven-Pasmooij et al., Citation2016). A number of available studies that looked at the impact of NCDs on labour market outcomes, especially diabetes, find that NCDs increase the chances of being unemployed and early retirement (Alavinia & Burdorf, Citation2008; Cai & Kalb, Citation2006; Schuring et al., Citation2007). It is also important to note that the majority of these studies were conducted in developed countries which have rich panel data sets to allow such type of analysis. In addition, these studies estimated employment status in a form of binary variable. It is possible for one to categorise and estimate the employment status as a multinomial choice variable, where the effect of NCD variables is allowed to differ across employment outcomes (economically inactive, unemployed, and employed). This approach can provide more comprehensive results, which can clearly show how NCDs affect employment status (Kouwenhoven-Pasmooij et al., Citation2016).
The previous studies that have been conducted in South Africa mostly examined socioeconomic and macroeconomic determinants of unemployment in South Africa (Vermeulen, Citation2017; Viljoen & Dunga, Citation2013). Given the magnitude of the rising rate of burden of NCDs in South Africa, it is of critical importance to understand how does NCDs affect employment status in the country. Unlike the previous studies, the current study seeks to analyse the effect of NCDs (diabetes, stroke and heart disease) on employment status in South Africa and how this effect differs between men and women which is an area that has not received significant attention. The importance of examining the effects of NCDs on employment status at gender level emanates from the effect of NCDs on employment status being gender specific. In addition, the study determined the effect of multi-morbidityFootnote1 on employment in South Africa. This analysis is particularly important in light of the efforts to enable individuals with NCDS to remain in the labour market for longer periods.
The findings from the study highlight that NCDs influence the employment status of women far more than that of men. Women with NCDs are found to be more likely to be economically inactive in the labour market. This issue can help health policymakers in targeting the most affected gender group for health promotion. The study is organised as follows: following the introduction, the second section focuses on reviewed relevant studies. The third section presents and discusses the methodology which has been utilised to analyse the link between the variables of interest. The presentation of results and conclusion to the study are presented in sections 4 and 5, respectively.
2. Literature review
The effect of health on employment status among older workers has been discussed extensively in the literature. The available studies show that as workers age, they are also likely to be more prone to chronic health conditions (like diabetes, stroke and heart attacks), injury and disability (Bound et al., Citation1999; Cai & Cong, Citation2009; Zucchelli et al., Citation2012). However, in terms of academic studies related to chronic health conditions and employment status, there are few that have been undertaken at international level (Chatterji et al., Citation2017).
Of the available studies, using a dataset of 14,000 households from New Jersey in the US, Wilson (Citation2001) examined the effect of chronic diseases on the probability of employment. The authors employed the probit regression model and the results revealed that individuals with chronic illnesses have a lower probability of employment. In addition, Wilson (Citation2001) argues that chronic diseases in New Jersey explain the large gap in employment probability for those with multiple chronic conditions.
In another study, Schofield et al. (Citation2013) assessed the association between multiple chronic conditions and labour force participation among people aged 45–64, using the logistic regression model. The authors found that having a chronic condition is associated with lower labour force participation and that having multiple conditions is associated with even lower labour force participation. This was also found to be consistent with findings of Virtanen, Junlart and Hammarström (Citation2013), who reaveled that there is a strong association between poor self-rated health and prolonged unemployment. This implies that chronic health conditions do have a significant impact on labour force participation.
Focusing on Denmark, Finland, Iceland and Norway, Lindbohm et al. (Citation2014) examined the link between early retirement and non-employment after breast cancer, controlling for comorbidity using multinomial logit model. The authors found that comorbidity is associated with increased probability of early retirement and other non-employment (due to unemployment, subsidized employment or being a homemaker).
There are studies that have emphasised that diabetes does have a significant impact on employment. Of these studies, González-González and Wong (Citation2014) examined the relationship between chronic diseases and labour force participation of individuals aged 50 years and older in Mexico using the logistic regression model. The findings show that the impact of chronic diseases on employment varies by gender, the negative impact of diabetes and hypertension on employment was significant in both men and women, while heart disease and stroke showed significant effect on employment status of men.
In another study still on Mexico, Seuring, Goryakin and Suhrcke (Citation2015) also analysed the effect of diabetes on employment of working age (15 and over) using the bivariate probit model to control for endogeneity of diabetes. The result from the standard probit model revealed that having diabetes significantly reduces employment probabilities for both men and women. Further analysis showed that diabetes mainly affects the employment probability of individuals over 44 and also has a greater effect on the poor than on the well-off. This was found to be contrary to Seuring et al. (Citation2015) who found that diabetes is exogenous and it does not have a significant effect on employment. The authors concluded that accounting for endogeneity resulted in an under-estimation of the effect of diabetes on employment.
In the United States of America, Chatterji et al. (Citation2017), utilising the longitudinal data from the 1992–2010 Health and Retirement Study in US to determine the effect of diabetes on exiting labour market among older Americans, reports that diabetes is associated with an increased probability of exiting the labour market. This analysis relies on standard probit regression model and does not use methods that allow one to account for the dynamic nature of the relationship between the variables of interest.
Consistent with Chatterji et al. (Citation2017), Ward (Citation2015) employed the multivariate regression analysis to assess the association between multiple chronic conditions and employment of US adults aged 18–64. Chronic diseases examined under this study were cancer, hypertension, coronary heart disease, stroke, obstructive pulmonary disease, asthma, diabetes, arthritis, hepatitis and failing kidneys. Empirical results revealed that adults with just one chronic condition had an increased probability of employment compared with individuals with multiple conditions. The main findings of the study suggest that multiple chronic conditions are a barrier to employment.
In a study on European countries, Kouwenhoven-Pasmooij et al. (Citation2016) analysed the relationship between cardiovascular diseases or diabetes and exit from paid employment, unemployment and early retirement using the multinomial regression model. The study found no significant effect of NCDs related to the employed as against the unemployed and strong positive association between cardiovascular diseases and early retirement.
The review of literature does indicate that there are several studies that have been done on the subject. However, the focus has been on developed countries and not much in developing countries where the prevalence of NCDs is growing rapidly. However, it is important to note that many of the previous studies have focused solely on one particular disease (Lindbohm et al., Citation2014, Goryakin and Suhrcke, Citation2015; Kouwenhoven-Pasmooij et al., Citation2016; Chatterji et al., Citation2017), while some studies carried out focused on general health status (Virtanen et al., Citation2013). It is also important to note that most of the studies reviewed did not consider the problem of endogeneity and heterogeneity. These are likely to be present in NCDs and labour market outcome models. All these points are likely to have resulted in biased and inconsistent results. Furthermore, in the case of South Africa, there are no specific studies of this nature to the best of our knowledge. Many of the available studies on labour market outcomes have focused largely on either the determinants of labour market outcomes in the country, focusing on the conventional variables of unemployment, but have not specifically examined how different types of NCDs might influence labour market outcomes in the country. This becomes important in the case of South Africa, given that communities in this country rely heavily on income from the labour market while at the same time experiencing an increasing burden of NCDs. It is also important to determine the differential effect of a variety of diseases on labour market outcomes which is the focus of the current study.
3. Data and methods
This study utilised five waves of balanced panel data of the National Income Dynamics Study (NIDS) from 2008 to 2018. The surveys use a two-stage stratified cluster sampling design. In the first stage, 400 primary sampling units in 53 districts of all 9 South African provinces were selected from Statistics South Africa’s 2003 master sample of 3000 PSUs. Private households were randomly selected within each sampled PSU and individuals from the selected households were then interviewed.Footnote2 Panel weights were assigned to observations to consider attrition bias and survey design bias. Around 57% of the original sample have been reinterviewed in all waves of the NIDS. In determining the final sample to be used, all the females and males that were not followed across the five waves were dropped (N = 3502, 2900, respectively). The analysis was restricted to individuals between the age of 20 in wave 1 and not older than 64 years in wave 5. Although the working age population is defined by the International Labour Organisation as individuals aged 15–64 years, this study excludes younger respondents who were mainly students at the time of analysis as this might cause bias estimates (Lawana et al., Citation2020; Nwosu & Woolard, Citation2017). The final analysed sample comprised of 3325 and 1501 working age females and males, respectively.
The dependant variable is employment status which is a categorical variable, and it has three categories that consists of employed, unemployed, and economically inactive individuals. The study combined individuals reported “being unemployed and actively searching for a job in the last four weeks” with individuals reported “unemployed and not actively searching for a job in the last four weeks” due to the small sample size of individuals reported being unemployed but not searching for job.
The main independent variable is non-communicable diseases (diabetes, stroke, heart diseases). Non-communicable diseases are included in the model because they affect the health status of individuals and health is a form of human capital (Grossman, Citation1972). NCD is the major independent variable in the employment status model. NCDs can affect employment status of an individual thereby affecting employability in certain types of employment (Schultz, Citation1961; Becker, Citation1962; Mushkin, Citation1962). The measures of NCDs used in this study are self-reported by the participants to the survey. The NCD dummy variables were created from the question in the survey to identify the occurrence of the diseases whereby participants were asked if they had ever been diagnosed with diabetes, stroke or heart diseases.
The analysis looked at differential impact of having an individual NCD compared with the impact of having multi-morbidity on employment status. Multi-morbidity is defined as the coexistence of two or more NCDs. It is a binary variable with a value of one if the individual reported that he/she was diagnosed with more than one NCD, be it diabetes and stroke or/and heart disease, and 0 if otherwise. Four NCD (diabetes, stroke, heart diseases and high blood pressure) variables were used to construct multimorbidity variable. It is expected that the probability of multi-morbidity will reduce the probability of being employed and increase the probability of being unemployed and other inactive situations. The impact of multi-morbidity on employment status will be much higher than the impact of having one NCDs on employment status. This is consistent with Ward (Citation2015). Other control variables of interest include individual characteristics (marital status, education, age and non-labour income), household characteristic (household size), and geographical characteristics (area of residence that is urban, rural or farm).
4. Regression models
The examination of different types of NCDs on employment status is underpinned by Grossman theory of demand for health and the dynamic labour supply model. Access to employment is an important labour market outcome. Health status can be an important determinant of access to employment. Health status contributes to an individual’s probability of participating in various types of employment by influencing total healthy time which individuals have for market and non-market activities (Grossman, Citation1972). Health status, in turn, is determined by socioeconomic, demographic and geographical factors. In addition, other factors that directly influence access to employment include socioeconomic, demographic and geographical factors.
This section presents the models utilised in estimating the impact of NCDs on employment status in South Africa. The model has three categories of employment status: employed (j = 1), unemployed (j = 2) and economically inactive (j = 3). The model is specified as follows:
where j is a categorical measure of employment status; NCD is a measure of specific non-communicable diseases (i.e., diabetes, stroke, and heart diseases) for individual i and X is vector of explanatory variables that affect employment status such as demographics (age, gender, marital status, and race); socioeconomics (education, non-labour income, and household size) and geographical factors for individual, ε is an error term and β are coefficients to be estimated.
The multinomial logit model was employed to analyse the link between the variables of interest. The multinomial logit is the most common model used in the literature for a dependent or outcome variable with more than two categories (nominal outcome). As an extension of the binary logistic regression for outcome variables with more than two categories, the multinomial logit regression model consists of a set of independent binary logit equations with each modelling the binary logit between the selected reference outcome category and one other outcome category (Roessler et al., Citation2015).
The model has one major limitation that has been recognised in the literature that relate to the assumption of independence of irrelevant alternatives (IIA). The multinomial logit model has errors which are independent among the dependant variable choices and identically distributed according to the type-1 extreme value distribution (Kropko, Citation2008). In other words, this means that the choice of one category is not related to another choice of category (i.e. dependant variable). This assumption can be tested using the Hausman-McFadden test. When IIA is a false assumption, the estimates of multinomial logit are biased and inconsistent. However, what is good about multinomial logit estimates is that the odds ratio only depends on the coefficient of interpreted outcome category, meaning that the odds ratio is fixed to additions or deletions of other outcome categories.
As this study used five waves of observations, it is appropriate to include random intercept to account for unobserved heterogeneity or spurious dependence between individuals. This heterogeneity may occur because individuals can make several choices that may not be independent and hence the probabilities of each category for the same individual will share the same unobservable random effects. The parameters will be biased if unobserved heterogeneity is not accounted for. This requires a more advanced estimation model than just pooled multinomial logit without random effects. The inclusion of random intercept in the multinomial logit model partially relaxes the IIA assumption (Grilli & Rampichini, Citation2007; Zhu et al., Citation2010). Hence, the study employed generalised linear latent and mixed model (GLLAMM) to fit the multinomial logit model with correlated random intercept to control for unobserved heterogeneity between individuals or categories. Therefore, REMNL had several advantages; these include being able to account for unobserved heterogeneity, considering the correlation in the error term and it is more computational time compared to other longitudinal data methods for discrete variables. Due to the nature of variables used in the study, the multinomial random effects model is employed. Employment state and NCD variables were time invariant in most waves and according to the literature, the suitable model for time invariant variables is the random effects (Bell et al., Citation2019)
To describe the REMNL, suppose that individual i is faced with j different choices of employment status at time t. The individual receives level of utility at each alternative and chooses the category that maximises the utility. The probability of choosing employment category (Pr) in model j = 1, 2, 3 is related to a set of observed characteristics Xit which vary between individuals and over time and unobserved individual effects (εi) that are time constant. This multinomial logit observation can be presented as
where βj is the intercept for each employment status and βk is the set of coefficients to be estimated, following the standard assumption that εi is identically and independently distributed over individuals and it follows a multivariate normal distribution of unobserved heterogeneity. Hence, the sample likelihood function (L) of multinomial logit with random intercepts can be expressed as
The log likelihood function (LL) for the estimation is specified as
where f(ε) represents the population distribution of random effects, dijt = 1 if individual i chooses category j at time t and zero otherwise. The model is identified if the coefficient vector (β) and unobserved heterogeneity term (ε) of one category are set to zero. The major problem in estimating Equationequation 33
3 is that the analytical solution for integral part of the model cannot be obtained. “In this regard, the random effects are assumed to have a multivariate normal distribution and the marginal distribution can be found only after integrating out these random effects” (Drikvandi et al., Citation2017, p5). This requires some form of numerical integration. There are various simulation and quadratic techniques suggested in the literature such as adaptive Gaussian Quadrature (AGQ), Monte Carlo Simulation, Laplace Approximation, Taylor series approximation, and Gauss Hermie quadrature to solve this problem (Cameron & Trivedi, Citation2009; Haan & Uhlendorff, Citation2006; Hartzel et al., Citation2001; Rabe-Hesketh et al., Citation2004; Train, Citation2009). However, in estimating the numerical integration of Equationequation 3
3
3 , AGQ is recommended as the most computationally efficient method than other quadrature techniques. Moreover, AGQ technique is the preferred method for longitudinal data because the number of quadrature points required to approximate the integral effectively is much lower than for ordinary quadrature (Haynes et al., Citation2008). GLLAMM with AGQ techniques is an extension of the generalized linear model because it incorporates both fixed and random effects.
In this study, employed was chosen as a reference or base outcome category. The unknown parameters are jointly estimated using the maximum likelihood (ML) approached in which values of the coefficients are selected which makes the observed results most likely (Cizek & Fitzgerald, Citation1999).
The multinomial logit coefficient is then expressed as the odds ratio (Expβ). The odds ratios or coefficients are interpreted as change of likelihood of an outcome category relative to that of base outcome category for a unit change in the value of independent variable. In addition, a coefficient which is equal to 1.00 indicates that there is no change in the odds of being in one category of the outcome measure versus the reference category; coefficients greater than 1.00 indicate that the odds of being in one category of outcome measure relative to reference category for the unit chance in some explanatory variables increases and coefficients less than 1.00 indicate that the odds of being in one category of the outcome measure relative to reference outcome category for the unit change on some explanatory variable decreases (Cizek & Fitzgerald, Citation1999; Roessler et al., Citation2015).
The empirical equations were fitted for men and women separately because of differences on employment patterns and differences on chronic health conditions.
To select the best fit model, Bayesian Information Criterion (BIC) and Akaike Information Criterion (AIC) were utilised in the study with the hypothesis that the lower AIC and BIC values were more preferable as they would result in a more robust model (Vrieze, Citation2012). AIC and BIC indicate that model with low values is more robust that the alternative model with high values.
4.1. Results and discussions
4.1.1. Descriptive statistics
Table presents the descriptive statistics of variables used in the analysis. The data come with sets of weights. These are intended to correct the sample for non-response, attrition and overall representativity. We observe that the sample percent is altered by weights in a way that makes the sample look closer to the national South African population throughout the waves. Therefore, we incorporated panel weights into all the analyses presented in this paper. The descriptives shows that 18% and 36% of men and women, respectively, were not economically active in the labour force. Also, over 65% of males reported being employed with only 48% of females reported being employed in the study. The percentage of individuals reported being unemployed was less than a quarter of the sample. More women than men significantly reported being unemployed.
Table 1. Descriptive statistics
A higher percentage of women than men reported specific NCDs. While less than 5% of men reported having specific individual NCDs (diabetes, stroke, and heart), approximately 4% of women reported having diabetes. In addition, when it comes to multimorbidity as well, more women (0.72.%) than men (0.11%) reported having multimorbidity; however, the difference is not statistically significant. According to the NIDS data, the prevalence of NCDs in South Africa among individuals between the ages of 20–64 is very low compared to what has been documented in the literature (2013–2017 Strategic plan for the prevention of NCD). According to World Health Organisation (Citation2018), in 2016, the total mortality due to NCDs was 51%; for instance, cardiovascular diseases accounted to 19% of overall deaths and diabetes resulted to 7% of overall deaths in the country.
The dominant population race of the sample reported in the study was African (88% men and 84% for women, respectively). Majority of men and women in the sample reported having secondary level of education (32% men and 36% for women). Those with matric and tertiary education stood at 45% and 36% for both men and women, respectively. The proportion of individuals reported having no formal education were few for both men and women. The mean age of men was 41 years while that of women was 42 years. A smaller fraction of women in the sample reported to be single or never married (37%) compared to 44% of men. Similarly, a larger percentage of men in the sample reported to be living in the urban areas (65%) compared to (62%) of the women.
Almost the same average of household size reported by both men and women (4 and 5, respectively). More women (56%) in the sample reported having non-labour income compared to that of men (12%).
Table presents the distribution of employment status categories by individuals reported having specific NCDs and multiple NCDs by gender. Regardless of whether they are men or women, among the healthiest, the highest percentage of the population is employed or participating in the labour market than among those reported poor health conditions or NCDs. NCDs in the labour market are more prevalent to women. It seems there is a possible negative association between NCDs and employment status (i.e., specifically employed category) more especially females reported having more than one disease. More females either reported one specific NCD or not were economically inactive in the labour force. For example, approximately 49% of women reported having diabetes were not economically active in the labour market, while only 28% of men in the sample reported having same disease. Only 30% of women reported in the sample being employed while having diagnosed with multiple NCDs, though higher proportion of males reported having multiple NCDs were employed.
Table 2. Distribution of employment status among individuals who reported having an NCDs, by gender
4.2. Empirical estimation results and discussions
4.2.1. Multinomial logit regressions
The study estimated a robust panel multinomial logit model without random effects on the impact of NCDs on employment status in South Africa. However, given the panel nature of the data used, an unobserved heterogeneity is suspected to exist between individuals and across different constraints categories in results presented in Table . Hence, the study further estimated multinomial logit model with random effects.
This section presents the maximum likelihood model estimated from the multinomial logit regression model with an emphasis on the impact of NCDs on probability of economically inactive, and unemployed relative to participating in paid employment. The base category represents those who reported being employed.
The estimated results for panel multinomial logit with random effects are reported in Tables , respectively, in odds ratios. The interpretation of results presented along with diagnostic and robust checks are based on the random effects multinomial logit. The BIC and AIC values from the panel multinomial logit without random effects are reported in Tables for comparison purposes.
Table 3. Effects of NCDs on employment status (GLLAMM)
Table 4. Effect of multi-morbidity on employment status (GLLAMM)
The unexplained variance in the economically inactive category and correlation between two categories is captured by random effects at individual level (Table ). The Wald test confirmed that the null hypothesis that all coefficients are simultaneous equal to zero except the intercept term is rejected at one percent of confidence interval and this confirms the theoretical predictions of the above model. Table shows odds ratios and confidence intervals for economically inactive and expanded unemployed (discouraged work seekers and strictly unemployed), respectively. Given that the analysis was disaggregated by gender, it was found that a significant influence of the different NCDs on employment status varies by gender. Men’s choice of employment status is significantly influenced by heart diseases only. The men’s odds of being unemployed are highly associated with heart diseases. The likelihood of men being unemployed when reported heart diseases is 83% (95% confidence (CI), 1.00–3.33) higher than those without heart diseases, while employment status of women is significantly influenced by all estimated NCDs. These results are consistent with Zhang et al. (Citation2009), Harris (Citation2009), Pelkowski and Berger (Citation2004) and González-González and Wong (Citation2014) who found evidence that participation in the labour market or employment status varies by gender. González-González and Wong (Citation2014) found that women’s employment status is not influenced by heart diseases whereas it has a negative impact on men’s employment. However, in this current study, it is found that women with heart diseases are also significantly more likely to be economically inactive in the labour market (OR:1.25; 95% CI:1.02–1.58).
Incident of having one NCD is found to be the most threatening factors in the South African labour market for only women in this study. For instance, the findings show that NCDs are associated with the positive probability of women being economically inactive relative to employed. The results indicate that the magnitude impact of stroke on women is significantly higher than that of diabetes and heart conditions. For women, stroke raises the likelihood of being economically inactive by 1.8 times relative to the likelihood of being employed (95% CI, 0.88–2.78).
The study finds that the impact of diabetes on economically inactive relative to employed is only statistically significant for females. These findings contradict with those of Chatterji et al. (Citation2017) who investigate the effect of diabetes on labour market exit using longitudinal data from 1992 to 2010 health and retirement study in US. The authors found that diabetes is associated with increased probability of exiting the labour market for men, and the association was not significant for women. This result can be explained by the differences in the economically inactive used in both studies. Chatterji et al. (Citation2017) examined exit from labour market after diagnosis of diabetes while this current study does not determine whether the individuals were economically active before diagnosis.
The results also indicate insignificant impact of NCDs on unemployment relative to employment for women. For males, the odds of being unemployed relative to employed is statistically significant with an incidence of heart diseases. These results regarding the association between NCDs and unemployment are in line with other studies focusing on diabetes, heart diseases and stroke on exit via unemployment (Kouwenhoven-Pasmooij et al. (Citation2016). Several studies have reported statistically significant effect of combined categories of NCDs or self-reported poor health on unemployment (Robroek et al., Citation2013; Schuring et al., Citation2007; Virtanen et al., Citation2013). However, comparing the current findings with those from other studies should be done cautiously, because the analysis of this study is disaggregated by gender. The insignificant impact of NCDs on unemployment may be due to endogeneity. This means that unemployment is likely to contribute to NCDs, and being unemployed can cause individuals to develop stress, high blood pressure, heart diseases or diabetes. Therefore, a model that considers endogeneity bias that arise from NCD variables is required.
4.3. Effects of multiple NCDs on employment status
The estimated effect of multi-morbidity is as expected for women, whilst that of odds of being unemployed when reported multi-morbidity is not significant for women. Having the multi-morbidity of chronic conditions and individual chronic condition differ in the sense that single disease can be easily managed while multiple NCDs takes time to manage and requires many medical consultation visits. The results indicate no significant difference of having individual NCDs with those having multi-morbidity of employment status.
The results show that a combination of more than one NCDs (diabetes, stroke, and/or heart) increases the odds women being economically inactive relative to being employed. The likelihood of economically inactive in the labour market among women with multi-morbidity is 2.49 times (95% CI 0.88–6.98) more than those without multi-morbidity. For men, however, the effect of multiple NCDs on odds of economically inactive relative to employed is different. The findings indicate that there are less chances of men with multiple NCDs to be economically inactive relative to being employed in the South African labour market. Further, the results indicate that having one NCD does not significantly influence the allocation of individuals into various employment status. These results imply that having an additional NCD will cause the number of those that are economically inactive to decrease by 25%. These findings contradict with the results from previous studies such as Schofield et al. (Citation2013), Smith et al. (Citation2014) and Ward (Citation2015) which all indicated that a combination of NCDs was associated with a greater probability than the separate effect of each chronic condition independently on employment outcomes.
This study does not provide evidence that the impact of multi-morbidity on employment status is higher than that of individual NCD for men. Contrary to Ward (Citation2015), the author finds that having multi-morbidity reduces the probability of employment relative to economically inactive.
The positive and large magnitude impact of stroke on economically inactive population relative to working for women may be attributed to the fact that most of wage employment in South Africa involves a lot of labour-intensive jobs, especially the manufacturing sector which requires workers to be fit for industrial work. In addition, working for wage jobs in South Africa is non-flexible time; they require employees to work for minimum of 8 hours per day and standard working week. Whereas individuals with NCDs may require flexible working schedules depending on the type and severity of chronic diseases. Therefore, the chances of getting treatment and doctor’s visit check-ups might not be available for individuals with these diseases.
The data used have number of limitations to be considered; while this study accounted for unobserved heterogeneity, the estimates may have been affected by the unobserved time invariant of covariates. Reverse causality, where employment status can have effect on the propensity to develop or be diagnosed with certain non-communicable diseases, may have a role on the estimates. Previous studies that have looked at this particular direction of causality have not found strong evidence of the effect of employment status on NCDs onset. For example, Schaller and Stevens (Citation2015) estimated the short-rum effects of jobless on health conditions in the United States. The authors found little evidence of the increasing diabetes onset after job loss and the results found the impact of employment status on heart diseases was not significant. However, these studies are done in high-income countries.
The small individual response rate on the question of being diagnosed with NCDs is found to be a big challenge and the proportion of employment status does not correspond with the reported national figures on labour force participation. Additionally, large percentage of the included respondents at wave 1 were lost to follow up. However, in the analysis between respondents and non-respondents, no major differences were found in non-communicable measures and employment variables. The data employed in the study are based on self-reported NCDs which may under-report the true prevalence of NCD cases in the country.
To address these limitations, there are number of steps for further research that might be taken. The result of this study suggests the need for qualitative research like interviews or focus groups with individuals with non-communicable diseases, employers and human resource managers. This could provide in-depth information on the needs of individuals with NCDs in the labour market and to examine why females with NCDs are more likely not to be active in the labour market than their male counterparts. In addition, there are important factors that have a significant influence on employment status that are controlled in the study like illness, employment rate, additional chronic conditions (those related to both mental health and physical health) that could be considered in the future research. Also, an alternative analytical method may be taken that could yield additional information on the impact of non-communicable diseases in employment status.
5. Conclusion
Using longitudinal data from the National Income Dynamic Study, the study examined the impact of NCDs on employment status in South Africa. The overarching result is that the NCDs significantly impact on women employment status. The study finds no significant differences by gender on the impact of NCDs on employment status. When controlling for having multi-morbidity, it also increased the probability of being economically inactive relative to employment but only statistically significant for females. No evidence was found on the difference between the magnitude of having individual NCDs and having multi-morbidity. Regarding unemployment, the results indicate no evidence of its association with NCDs in all the models. The findings of this study imply that NCDs constraint individuals especially women from being economically active in the labour market. Therefore, there is a need for government to expand health promotion initiatives to manage and reduce the spread of NCD prevalence so as to improve employment rate and labour force participation in the country.
Acknowledgments
The financial assistance of the National Institute for the Humanities and Social Sciences, in collaboration with the South African Humanities Deans Association towards this research is hereby acknowledged. Opinions expressed and conclusions arrived at are those of the authors and are not necessarily to be attributed to the NIHSS and SAHUDA.
Disclosure statement
No potential conflict of interest was reported by the author(s).
Additional information
Funding
Notes
1. Multi-morbidity is defined as an occurrence of more than one NCDs reported by individual (i.e. diabetes and/or stroke and/or heart diseases and/or high blood pressure).
2. A detailed description of the dataset is available at www.nids.uct.ac.za
References
- Alavinia, S. M., & Burdorf, A. (2008). Unemployment and retirement and ill-health: A cross sectional analysis across European countries. International Archives of Occupational and Environmental Health, 82(1), 39–18. https://doi.org/10.1007/s00420-008-0304-6
- Ataguba, J. E. (2012). Distributional impact of health care financing in South Africa [ Unpublished PhD]. University of Cape Town.
- Becker, G. S. (1962). Investment in human capital: A theoretical analysis. The Journal of Political Economy, 70(5, Part 2), 9–49. https://doi.org/10.1086/258724
- Bell, A., Fairbrother, M., & Jones, K. (2019). Fixed and random effects models: Making an informed choice. Quality & Quantity, 53(2), 1051–1074. https://doi.org/10.1007/s11135-018-0802-x
- Bound, J., Schoenbaum, M., Stinebrickner, T., & Waidmann, T. (1999). The dynamic effects of health on the labor force transitions of older workers. Labour Economics, 6(2), 179–202. https://doi.org/10.1016/S0927-5371(99)00015-9
- Cai, L., & Cong, C. (2009). Effects of health and chronic diseases on labour force participation of older working‐age Australians. Australian Economic Papers, 48(2), 166–182.
- Cai, L., & Kalb, G. (2006). Health status and labour force participation: Evidence from Australia. Health Economics, 15(3), 241–261. https://doi.org/10.1002/hec.1053
- Cameron, A. C., & Trivedi, P. K. (2009). Microeconometrics using stata (Vol. 5). Stata press.
- Chatterji, P., Joo, H., & Lahiri, K. (2017). Diabetes and labor market exits: Evidence from the Health & Retirement Study (HRS). The Journal of the Economics of Ageing, 9, 100–110. https://doi.org/10.1016/j.jeoa.2016.08.005
- Cizek, G. J., & Fitzgerald, S. M. (1999). An introduction to logistic regression. Measurement and Evaluation in Counseling and Development, 31(4), 223–245.
- Drikvandi, R., Verbeke, G., & Molenberghs, G. (2017). Diagnosing misspecification of the random‐effects distribution in mixed models. Biometrics, 73(1), 63–71. https://doi.org/10.1111/biom.12551
- Duval, R., Eris, M., & Furceri, D. (2010). Labour force participation hysteresis in industrial countries: Evidence and causes ℵ.
- González-González, C., & Wong, R. (2014). Health impact: Longitudinal analysis of employment at middle and old age in Mexico. Population Papers, 20(81), 89–120.
- Grilli, L., & Rampichini, C. (2007). A multilevel multinomial logit model for the analysis of graduates’ skills. Statistical Methods and Applications, 16(3), 381–393. https://doi.org/10.1007/s10260-006-0039-z
- Grossman, M. (1972). On the concept of health capital and the demand for health. Journal of Political Economy, 80(2), 223–255. https://doi.org/10.1086/259880
- Haan, P., & Uhlendorff, A. (2006). Estimation of multinomial logit models with unobserved heterogeneity using maximum simulated likelihood. The Stata Journal, 6(2), 229–245. https://doi.org/10.1177/1536867X0600600205
- Harris, A. (2009). Diabetes, cardiovascular disease and labour force participation in Australia: An endogenous multivariate probit analysis of clinical prevalence data. Economic Record, 85(271), 472–484. https://doi.org/10.1111/j.1475-4932.2009.00572.x
- Hartzel, J., Agresti, A., & Caffo, B. (2001). Multinomial logit random effects models. Statistical Modelling, 1(2), 81–102. https://doi.org/10.1177/1471082X0100100201
- Haynes, M., Western, M., Yu, L., & Spellak, M. (2008). Analysing nominal data from a panel survey: Employment transitions of Australian women. Proceedings of the 103rd Annual Meeting of the American Sociological Association, 1-4 August 2008, Boston, USA.
- Kouwenhoven-Pasmooij, T. A., Burdorf, A., Roos-Hesselink, J. W., Hunink, M. G. M., & Robroek, S. J. W. (2016). Cardiovascular disease, diabetes and early exit from paid employment in Europe; the impact of work-related factors. International Journal of Cardiology, 215, 332–337. https://doi.org/10.1016/j.ijcard.2016.04.090
- Kropko, J. (2008). Choosing between multinomial logit and multinomial probit models for analysis of unordered choice data.
- Lawana, N., Booysen, F., Tsegaye, A., Kapingura, F. M., & Hongoro, C. (2020). Lifestyle risk factors, non-communicable diseases and labour force participation in South Africa. Development Southern Africa, 37(3), 446–461. https://doi.org/10.1080/0376835X.2019.1678459
- Lindbohm, M. L., Kuosma, E., Taskila, T., Hietanen, P., Carlsen, K., Gudbergsson, S., & Gunnarsdottir, H. (2014). Early retirement and non‐employment after breast cancer. Psycho‐Oncology, 23(6), 634–641. https://doi.org/10.1002/pon.3459
- Mushkin, S. J. (1962). Health as an Investment. Journal of Political Economy, 70(5, Part 2), 129–157. https://doi.org/10.1086/258730
- Nwosu, C. O., & Woolard, I. (2017). The impact of health on labour force participation in South Africa. South African Journal of Economics, 85(4), 481–490. https://doi.org/10.1111/saje.12163
- Peasey, A., Bobak, M., Kubinova, R., Malyutina, S., Pajak, A., Tamosiunas, A., Pikhart, H., Nicholson, A., & Marmot, M. (2006). Determinants of cardiovascular disease and other non-communicable diseases in Central and Eastern Europe: Rationale and design of the HAPIEE study. BMC Public Health, 6(1), 255. https://doi.org/10.1186/1471-2458-6-255
- Pelkowski, J. M., & Berger, M. C. (2004). The impact of health on employment, wages, and hours worked over the life cycle. The Quarterly Review of Economics & Finance, 44(1), 102–121. https://doi.org/10.1016/j.qref.2003.08.002
- Rabe-Hesketh, S., Skrondal, A., & Pickles, A. (2004). GLLAMM manual. UC Berkeley division of biostatistics working paper series. University of California, Berkeley.
- Robroek, S. J., Schuring, M., Croezen, S., Stattin, M., & Burdorf, A. (2013). Poor health, unhealthy behaviors, and unfavorable work characteristics influence pathways of exit from paid employment among older workers in Europe: A four-year follow-up study. Scandinavian Journal of Work, Environment & Health, 39(2), 125–133. https://doi.org/10.5271/sjweh.3319
- Roessler, R. T., Rumrill Jr, P. D. B., Li, J., & Leslie, M. J. (2015). Predictors of differential employment statuses of adults with multiple sclerosis. Journal of Vocational Rehabilitation, 42(2), 141–152. https://doi.org/10.3233/JVR-150731
- Schaller, J., & Stevens, A. H. (2015). Short-run effects of job loss on health conditions, health insurance, and health care utilization. Journal of Health Economics, 43, 190–203. https://doi.org/10.1016/j.jhealeco.2015.07.003
- Schofield, D. J., Callander, E. J., Shrestha, R. N., Passey, M. E., Percival, R., & Kelly, S. J. (2013). Multiple chronic health conditions and their link with labour force participation and economic status. PloS one, 8(11), e79108.
- Schultz, T. H. (1961). Investment in human capital. The American Economics Review, 51, 1–17.
- Schuring, M., Burdorf, L., Kunst, A., & Mackenbach, J. (2007). The effects of ill health on entering and maintaining paid employment: Evidence in European countries. Journal of Epidemiology and Community Health, 61(7), 597–604. https://doi.org/10.1136/jech.2006.047456
- Seuring, T., Goryakin, Y., & Suhrcke, M. (2015). The impact of diabetes on employment in Mexico. Economics & Human Biology, 18, 85–100.
- Shahid, M. (2014). Impact of labour force participation on economic growth in Pakistan. Journal of Economics & Sustainable Development, 5(11), 89–93.
- Smith, P., Chen, C., Mustard, C., Bielecky, A., Beaton, D., & Ibrahim, S. (2014). Examining the relationship between chronic conditions, multi-morbidity and labour market participation in Canada: 2000–2005. Ageing and Society, 34(10), 1730–1748. https://doi.org/10.1017/S0144686X13000457
- Statistics South Africa. (2019). Quartely labour force survey. South Africa http://www.statssa.gov.za/publications/P0211/P02111stQuarter2019.pdf
- Train, K. E. (2009). Discrete choice methods with simulation. Cambridge university press.
- van den Berg, T., Schuring, M., Avendano, M., Mackenbach, J., & Burdorf, A. (2010). The impact of ill health on exit from paid employment in Europe among older workers. Occupational and Environmental Medicine, 67(12), 845–852. https://doi.org/10.1136/oem.2009.051730
- Vermeulen, J. C. (2017). Inflation and unemployment in South Africa: Is the Phillips curve still dead? Southern African Business Review, 21(1), 20–54.
- Viljoen, D. J., & Dunga, S. H. (2013). Determining the factors that influence female Unemployment in a South African township. International Journal of Social Sciences & Humanity Studies, 5(1), 63–72.
- Virtanen, P., Janlert, U., & Hammarström, A. (2013). Health status and health behaviour as predictors of the occurrence of unemployment and prolonged unemployment. Public Health, 127(1), 46–52. https://doi.org/10.1016/j.puhe.2012.10.016
- Vrieze, S. I. (2012). Model selection and psychological theory: A discussion of the differences between the Akaike information criterion (AIC) and the Bayesian information criterion (BIC). Psychological Methods, 17(2), 228–243. https://doi.org/10.1037/a0027127
- Ward, B. W. (2015). Multiple chronic conditions and labor force outcomes: A population study of U.S. Adults. American Journal of Industrial Medicine, 58(9), 943–954. https://doi.org/10.1002/ajim.22439
- Wilson, S. S. (2001). Work and the accommodation of chronic illness: A re-examination of the health-labour supply relationship. Applied Economics, 33: 1(9), 139–1 156. https://doi.org/10.1080/00036840010004527
- World Health Organisation. (2018). Non-communicable Diseases Country Profiles 2018. https://doi.org/10.16/j.jad.2010.09.007
- Zhang, X., Zhao, X., & Harris, A. (2009). Chronic diseases and labour force participation in Australia. Journal of Health Economics, 28(1), 91–108. https://doi.org/10.1016/j.jhealeco.2008.08.001
- Zhu, C. W., Livote, E. E., Ross, J. S., & Penrod, J. D. (2010). A random effects multinomial logit analysis of using Medicare and VA healthcare among veterans with dementia. Home Health Care Services Quarterly, 29(2), 91–104. https://doi.org/10.1080/01621424.2010.493771
- Zucchelli, E., Harris, M. N., & Zhao, X. (2012). Ill-health and transitions to part-time work and self-employment among older workers. Available at SSRN 2269449.
Appendices
Table A1. Effects of NCDs on employment status (panel mlogit)
Table A2. Effects of multiple NCDs on employment status (panel mlogit)